Cognitive Psychology (8th Edition) PDF
Document Details
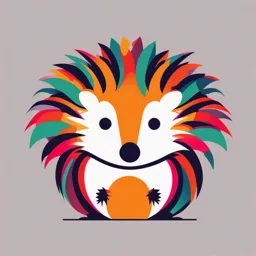
Uploaded by ChivalrousToucan3503
2020
Michael W. Eysenck & Mark T. Keane
Tags
Summary
This book is an eighth edition student handbook on cognitive psychology. It covers topics like inductive and deductive reasoning, and hypothesis testing. The authors are Michael W. Eysenck and Mark T. Keane.
Full Transcript
Eighth edition published 2020 by Routledge 2 Park Square, Milton Park, Abingdon, Oxon OX14 4RN and by Routledge 52 Vanderbilt Avenue, New York, NY 10017 Routledge is an imprint of the Taylor & Francis Group, an informa business © 2020 Michael W. Eysenck and Mark T. Keane The right of Michael W. Eyse...
Eighth edition published 2020 by Routledge 2 Park Square, Milton Park, Abingdon, Oxon OX14 4RN and by Routledge 52 Vanderbilt Avenue, New York, NY 10017 Routledge is an imprint of the Taylor & Francis Group, an informa business © 2020 Michael W. Eysenck and Mark T. Keane The right of Michael W. Eysenck and Mark T. Keane to be identified as authors of this work has been asserted by them in accordance with sections 77 and 78 of the Copyright, Designs and Patents Act 1988. All rights reserved. No part of this book may be reprinted or reproduced or utilised in any form or by any electronic, mechanical, or other means, now known or hereafter invented, including photocopying and recording, or in any information storage or retrieval system, without permission in writing from the publishers. Trademark notice: Product or corporate names may be trademarks or registered trademarks, and are used only for identification and explanation without intent to infringe. First edition published by Lawrence Erlbaum Associates 1984 Seventh edition Published by Routledge 2015 Every effort has been made to contact copyright-holders. Please advise the publisher of any errors or omissions, and these will be corrected in subsequent editions. British Library Cataloguing-in-Publication Data A catalogue record for this book is available from the British Library Library of Congress Cataloging-in-Publication Data A catalog record has been requested for this book ISBN: 978-1-13848-221-0 (hbk) ISBN: 978-1-13848-223-4 (pbk) ISBN: 978-1-35105-851-3 (ebk) Typeset in Times New Roman by Servis Filmsetting Ltd, Stockport, Cheshire Visit the companion website: www.routledge.com/cw/eysenck. 9781138482210_COGNITIVE_PSYCHOLOGY_PRE_CHAP_1.indd 6 28/02/20 2:15 PM Chapter 14 Reasoning and hypothesis testing INTRODUCTION KEY TERMS Inductive reasoning Forming generalisations (that may be probable but are not certain) from examples or sample phenomena; see deductive reasoning. Deductive reasoning Reasoning to a conclusion from a set of premises or statements where that conclusion follows necessarily from the assumption the premises are true; see inductive reasoning. For hundreds of years, philosophers have distinguished between two kinds of reasoning. One is inductive reasoning, which involves drawing general conclusions from premises (statements) referring to particular instances. A key feature of inductive reasoning is that the conclusions of inductively valid arguments are probably (but not necessarily) true. The philosopher Bertrand Russell provided the following example. A turkey might use inductive reasoning to draw the conclusion “Each day I am fed”, because that has always been the case in the past. However, there is no certainty that the turkey will be fed tomorrow. Indeed, if tomorrow is Christmas Eve, it is likely to be proven false. Scientists very often use inductive reasoning similarly to Russell’s hypothetical turkey. A psychologist may find across numerous experiments that reinforcement (reward) is needed for learning. This might lead them to use inductive reasoning to propose the hypothesis that reinforcement is essential for learning. This conclusion is not necessarily true because future experiments may not replicate past ones. The other kind of reasoning identified by philosophers is deductive reasoning. Deductive reasoning allows us to draw conclusions that are definitely or certainly valid provided other statements are assumed to be true. For example, the conclusion Tom is taller than Harry is necessarily true if we assume Tom is taller than Dick and Dick is taller than Harry. Deductive-reasoning problems owe their origins to formal logic. An important issue is whether the distinction between inductive and deductive reasoning is as clear-cut in practice as it appears above. There is increasing evidence that similar processes are involved in both cases. For example, Stephens et al. (2018) asked participants to evaluate identical sets of arguments after receiving inductive- or deductive-reasoning instructions. With the former instructions, participants decided whether the conclusion was plausible, strong or likely to be true. With the latter instructions, they decided whether the conclusion was necessarily true. 9781138482210_COGNITIVE_PSYCHOLOGY_PART_4.indd 666 28/02/20 4:14 PM 667 Reasoning and hypothesis testing Stephens et al. (2018) found participants used the same processes whether instructed to reason inductively or deductively. The only major difference was that greater argument strength was required to decide that a conclusion was necessarily true (deductive condition) than to decide it was strong or likely to be true. The wide chasm between the artificial, logic-driven, deductive-reasoning tasks traditionally used in the laboratory and everyday reasoning in the form of argumentation has led to a rapid increase in research on informal reasoning. Informal reasoning (discussed later, pp. 694–701) is based on our knowledge and experience rather than logic. It is a form of inductive reasoning that resembles our everyday reasoning. A major consequence of this shift in research is that it is increasingly accepted that reasoning processes often resemble those used in judgement and decision-making. For example, the Bayesian approach, according to which our subjective probabilities (i.e., that X is dishonest) are adjusted in the light of new information, plays a prominent role in theorising about judgements (see Chapter 13). In a similar fashion, the Bayesian approach is increasingly applied to reasoning (Navarrete and Mandel, 2016). KEY TERMS Informal reasoning A form of reasoning based on one’s relevant knowledge and experience rather than logic. Falsification Proposing hypotheses and then trying to falsify them by experimental tests; the logically correct means by which science should work, according to Popper (1968). HYPOTHESIS TESTING Karl Popper (1968) distinguished between confirmation and falsification. Confirmation involves the attempt to obtain evidence confirming or supporting one’s hypothesis. In contrast, falsification involves the attempt to falsify hypotheses by experimental tests. Popper claimed we cannot achieve confirmation via hypothesis testing. Even if all the available evidence supports a hypothesis, future evidence may disprove it. He argued falsifiability (the potential for falsification) separates scientific from u nscientific activities such as religion or pseudo-science (e.g., psychoanalysis). According to Popper, scientists should focus on falsification. In fact, as discussed later, they often seek confirmatory rather than disconfirmatory evidence when testing their hypotheses. It has also been claimed the same excessive focus on confirmatory evidence is found in laboratory studies on hypothesis testing – research to which we now turn. Wason’s 2-4-6 task Wason (1960) devised a much-researched hypothesis-testing task (see Evans, 2016, for a review). Participants were told three numbers 2-4-6 conformed to a simple relational rule. Their task was to generate sets of three numbers and provide reasons for generating each set. After each choice, the experimenter indicated whether the set of numbers conformed to the experimenter’s rule. Here is the rule: “Three numbers in ascending order of magnitude.” The participants could announce what they believed to be the rule on any trial and were told whether it was correct. The rule sounds (very) simple. However, only 21% of university students were correct with their first attempt (Wason, 1960). In spite of the emphasis in the literature on the poor levels of performance with the 2-4-6 task, Wason found 72% of participants eventually solved it. 9781138482210_COGNITIVE_PSYCHOLOGY_PART_4.indd 667 28/02/20 4:14 PM 668 KEY TERM Confirmation bias In hypothesis testing, seeking evidence that supports one’s beliefs. Thinking and reasoning Why is performance so poor? One explanation focuses on confirmation bias – most people seek information confirming their hypothesis. For example, participants whose original hypothesis or rule is that the second number is twice the first, and the third number is three times the first number often generate sets of numbers (test triples) consistent with that hypothesis (e.g., 6-12-18; 50-100-150). Wason assumed participants produced test triples conforming to their current hypothesis. However, this is an oversimplification (Evans, 2016). It is true that participants mostly produce positive or confirmatory tests conforming to their hypothesis and expected to receive positive feedback (conforms to the rule). However, participants sometimes produce negative tests, not conforming to their hypothesis, where they expect to receive negative feedback (does not conform to the rule). There are two types of negative tests (Evans, 2016): (1) (2) those (e.g., 12-8-4) where participants expect to receive the answer “No” and which are therefore confirmatory; those (e.g., 1-4-9) where participants expect to receive the answer “Yes” and which are therefore disconfirmatory. Findings Participants typically engage in very few falsification attempts on the 2-4-6 task and have a low success rate. Tweney et al. (1980) enhanced performance by telling participants the experimenter had two rules in mind and they had to identify both. One rule generated DAX triples and the other MED triples. They were also told 2-4-6 was a DAX triple. After generating each test triple, participants were informed whether the set fitted the DAX or MED rule. The DAX rule was any three numbers in ascending order and the MED rule covered all other sets of numbers. Over 50% of participants produced the correct answer on their first attempt (much higher than with the standard problem). Of importance, participants could identify the DAX rule by using positive testing to confirm the MED rule, and so they did not have to try to disconfirm the DAX rule. Gale and Ball (2012) carried out a study resembling that of Tweney et al. (1980). They always used 2-4-6 as an example of a DAX triple, but the example of a MED triple was 6-4-2 or 4-4-4. Success in identifying the DAX rule was much greater when the MED example was 6-4-2 (75%) rather than 4-4-4 (23%). The greatest difference between solvers and non-solvers of the DAX rule was the number of descending triples they produced. This indicates the importance of participants focusing on the ascending/descending dimension, which was difficult to do when the MED example was 4-4-4. Cowley and Byrne (2005) argued people show confirmation bias because they are loath to abandon their initial hypothesis. They suggested people might be much better at managing to falsify a given incorrect hypothesis if told it was someone else’s. As predicted, 62% of participants abandoned the other person’s hypothesis compared to only 25% who abandoned their 9781138482210_COGNITIVE_PSYCHOLOGY_PART_4.indd 668 28/02/20 4:14 PM Reasoning and hypothesis testing 669 own hypothesis. Cowley (2015) extended these findings. Participants were told the hypothesis “even numbers ascending in two” was theirs or that of an imaginary participant Peter. They generated more negative falsifying tests when the hypothesis was Peter’s rather than their own (32% vs 7%, respectively). In another experiment by Cowley (2015), all participants were told Peter’s hypothesis was “even numbers ascending in twos”. In two conditions, they were also told another participant, James, had a different explicit hypothesis (“any ascending numbers”) or implicit hypothesis (“something else”). In the third, condition, there was no mention of James. There were three key findings. First, there were numerous falsifying test triples (positive + negative tests) in all conditions (percentages between 43% and 54%; predominantly negative falsifying tests). Second, Peter’s incorrect hypothesis was rejected by nearly 80% of participants. Third, the correct rule was discovered by 50% of participants given James’ explicit hypothesis and 31% given his implicit hypothesis. In sum, “Negative falsifying is possible more often than the literature has ever shown... falsification is sufficient to announce that a hypothesis is untrue, but an explicit alternative hypothesis that explains the falsifying result is necessary for truth discovery” (Cowley, 2015, pp. 32–33). Performance on the 2-4-6 task involves separable processes of hypothesis generation and hypothesis testing. Most research has focused on the latter, but Cherubini et al. (2005) focused on hypothesis generation. They argued participants try to preserve as much of the information contained in the example triple (i.e., 2-4-6) as possible in their initial hypothesis, making this hypothesis much more specific than the general rule. Cherubini et al. (2005) presented participants with two initial triples exemplifying the rule. When these triples inhibited the generation of a very specific rule (e.g., 6-8-10; 9-14-15), participants generated more general hypotheses than when the two triples were consistent with a specific rule (e.g., 6-8-10; 16-18-20). The success rate was much higher in the former condition (70% vs 30%). Theoretical analysis Most hypotheses are sparse or narrow (applying to under half the possible entities in any given domain: Navarro & Perfors, 2011). For example, Perfors and Navarro (2009) asked people to generate all the rules and hypotheses applying to numbers in a given domain (numbers 1 to 1,000). The key finding was that 83% of the rules (e.g., two-digit numbers; prime numbers) applied to fewer than 20% of the numbers. With sparse hypotheses, positive testing is optimal “because there are so many ways to be wrong and so few to be right”. In such circumstances, the learner will discover “the world has a bias towards saying ‘no’, and asking for ‘yes’ is the best way to overcome it” (Perfors & Navarro, 2009, p. 2746). Thus, positive testing is typically successful. In contrast, the 2-4-6 task penalises positive testing because the target rule is so general. 9781138482210_COGNITIVE_PSYCHOLOGY_PART_4.indd 669 28/02/20 4:14 PM 670 Thinking and reasoning Evaluation Wason’s 2-4-6 task has been “the classic test-bed reasoning task for investigations of hypothesis falsification for over forty years” (Cowley, 2015, p. 2), with research having clarified the strengths and limitations of human inductive reasoning. The processes involved in the 2-4-6 task are of relevance to understanding scientists’ hypothesis testing. What are the limitations of Wason’s approach? First, his task differs from real-life hypothesis testing. Participants given the 2-4-6 task receive immediate accurate feedback but are not told why the numbers they produced attracted a “yes” or “no” response. In the real world (e.g., scientists testing hypotheses), the feedback is much more informative, but is often delayed in time and sometimes inaccurate. Second, the correct rule or hypothesis in the 2-4-6 task (three numbers in ascending order of magnitude) is very general because it applies to a fairly high proportion of sets of three numbers. In contrast, most rules or hypotheses apply to only a smallish proportion of possible objects or events. Positive testing works poorly on the 2-4-6 task but not with most other forms of hypothesis testing. Third, Wason argued most people show confirmation bias and find a falsification approach very hard to use. However, there is much less confirmation bias but more evidence of falsification when testing someone else’s hypothesis (Cowley, 2015; Cowley & Byrne, 2005). This is consistent with scientists’ behaviour. For example, at a conference in 1977 on the levels-of-processing approach to memory (see Chapter 6), nearly all the research presented identified limitations with that approach. Hypothesis testing: simulated and real research environments According to Popper (1968), a crucial feature of all truly scientific theories is falsifiability. Scientists should focus on falsification rather than confirmation because the latter cannot be achieved. His arguments possess merit but are oversimplified (see below). Suppose a scientist obtains findings apparently inconsistent with their hypothesis. The findings may mean the hypothesis is incorrect. However, they may reflect problems with the experimental design or the accuracy of the data. Of relevance, a recent study (Open Science Collaboration, 2015; see Chapter 1) found that attempts to replicate 100 findings in psychology were successful only 36% of the time. Dunbar (1993) found evidence of confirmation bias using a simulated research environment. Participants had to explain how genes are controlled by other genes using a computer-based molecular genetics laboratory. This problem is so difficult that those solving it in real life (Jacques Monod and François Jacob) received the Nobel prize! The participants were led to focus on the hypothesis that the gene control was by activation whereas it was actually by inhibition. Participants who simply sought data consistent with their activation hypothesis failed to solve the problem. In contrast, the 20% of participants solving the problem tried to explain the discrepant findings. Most participants started with the general hypothesis that activation was the key 9781138482210_COGNITIVE_PSYCHOLOGY_PART_4.indd 670 28/02/20 4:14 PM Reasoning and hypothesis testing 671 IN THE REAL WORLD: HYPOTHESIS TESTING BY SCIENTISTS What actually happens in real-life science? Uchino et al. (2010) analysed numerous research articles in psychology. The great majority (77%) sought confirmation by testing the hypothesis favoured by the researcher(s) and 91% supported an existing theory. Only 22% discussed other hypotheses. These findings suggest, “Someone must be wrong: either scientists are going about their business incorrectly or Popper was mistaken about how science progresses” (Sanbonmatsu et al., 2015, p. 2). Sanbonmatsu et al. (2015) proposed a solution based on a distinction between absolute or universal hypotheses and non-absolute hypotheses. Absolute hypotheses claim a given phenomenon always occurs, whereas non-absolute hypotheses claim a phenomenon occurs only in some conditions. Popper (1968) assumed scientific theories are absolute or universal. On that assumption, the optimal approach involves falsification or disconfirmation – a single negative observation would disprove an absolute theory. In contrast, a confirmatory approach is generally more informative than a disconfirmatory one with non-absolute hypotheses. What do scientists actually do? Sanbonmatsu et al. (2015) found 96% of researchers in psychology indicated their research was mostly driven by non-absolute hypotheses. Nearly all (96%) said they generally used a confirmatory approach. With absolute hypotheses, 81% of researchers would use a disconfirmatory approach. With non-absolute hypotheses, in contrast, only 9% would use a disconfirmatory approach with 91% favouring a confirmatory approach. Feist (2008) argued a useful heuristic in science is “confirm early – disconfirm late”. Scientists should initially seek confirmatory evidence for a theory; when they have such evidence, they should focus more on disconfirming the theory and discovering its breadth of application. Eighty-three percent of scientists were most likely to use a confirmatory approach early in a research programme, and 87% were most likely to use a disconfirmatory approach subsequently (Sanbonmatsu et al., 2015). In sum, Popper adopted an excessively black-and-white approach. The reality is messy: research rarely provides a definitive falsification of a theory. Instead, theories are modified as their limitations become increasingly apparent (Lakatos, 1978). Scientists’ strategies when engaged in hypothesis testing approximate to maximising the informativeness of the evidence obtained. Thus, describing their typical approach as “confirmation bias” (see Glossary) is misleading. Kane and Webster (2013) suggested using the term “confirmation heuristic” to refer to appropriate focus on a confirmation strategy, limiting the use of “confirmation bias” to situations where scientists refuse to use the disconfirmatory strategy even when optimal. controlling process, focusing on one gene after another as the potential activator. Only after every activation hypothesis had been disconfirmed did some participants focus on explaining data inconsistent with activation hypotheses. Confirmation bias: analysis and interpretation We have seen scientists generally adopt a confirmatory approach during hypothesis testing. We now focus on confirmation bias when scientists analyse and interpret their findings (see Nuzzo, 2015, for a review). Fugelsang et al. (2004) studied professional scientists working on issues in molecular biology relating to how genes control and promote replication 9781138482210_COGNITIVE_PSYCHOLOGY_PART_4.indd 671 28/02/20 4:14 PM 672 Thinking and reasoning in bacteria, parasites and viruses. Of 417 experimental results, over half (223) were inconsistent with the scientists’ predictions. They responded to 88% of these inconsistent findings by blaming problems with their methods. In only 12% of cases did the scientists modify their theories. Thus, the scientists showed considerable reluctance to change their original theoretical position. Approximately two-thirds of the inconsistent findings were followed up, generally by changing the methods used. In 55% of cases, the inconsistent findings were replicated. The scientists’ reactions were very different this time – in 61% of cases, they changed their theoretical assumptions. How defensible was the scientists’ behaviour? Note that almost half the inconsistent findings were not replicated when a second study was carried out. Thus, it was reasonable for the scientists to avoid prematurely accepting possibly spurious findings. Overall, these findings suggest the scientists’ exhibited only a modest tendency towards confirmation bias. John et al. (2012) asked over 2,000 psychologists to provide anonymous information about their questionable research practices. There was substantial evidence of such practices. For example, John et al. estimated 78% of respondents had selectively reported studies that “worked”, 62% had excluded data (typically those inconsistent with their hypotheses), and 36% had stopped data collection after achieving the desired result. Here are two other examples of confirmation bias. First, Bakker and Wicherts (2011) found in an analysis of statistical analyses in research journals that over 10% of p values were incorrect. In the great majority of cases where such errors changed the statistical significance of the results, the change was from non-significant to significant. Second, researchers often expect their meta-analyses (see Glossary) to support their existing hypotheses. As Watt and Kennedy (2017, p. 1) argued, “Decisions about studies to be included [in a meta-analysis], statistical analyses, and moderating factors are made after the analysts know the outcomes of the studies. These retrospective decisions provide high potential for [confirmation] bias.” How can we reduce confirmation bias in the analysis and interpretation of data? Several answers have been proposed (Hamlin, 2017). First, more openness or transparency is required by experimenters so other researchers can see precisely what has been done. Second, there is blind analysis – all relevant statistical analyses are completed before the experimenter(s) is aware of the outcomes of such analyses. Third, and most importantly, there is pre-registration – experimenters announce the rationale, hypotheses, design and proposed methods of data analysis before conducting a piece of research. DEDUCTIVE REASONING In deductive reasoning, conclusions can be drawn with certainty. In this section, we will mostly consider conditional and syllogistic reasoning problems based on traditional logic. In the next section, we consider general theories of deductive reasoning. As we will see, theory and research increasingly focus on the non-logical strategies and processes used when people solve deductive-reasoning problems. 9781138482210_COGNITIVE_PSYCHOLOGY_PART_4.indd 672 28/02/20 4:14 PM 673 Reasoning and hypothesis testing Conditional reasoning KEY TERM Conditional reasoning (basically, reasoning with “if”) had its origins in Conditional reasoning A form of deductive reasoning based on if... then propositions. propositional logic, in which logical operators such as or, and, if... then, if and only if are included in sentences or propositions. In this system, symbols represent sentences and logical operators are applied to them to reach conclusions. Thus, we might use P to stand for the proposition “It is raining” and Q to stand for “Nancy gets wet”, and then use the logical operator if... then to relate these two propositions: if P then Q. The meanings of words and propositions in propositional logic differ from their natural language meanings. For example, propositions can have only one of two truth values: true or false. If P stands for “It is raining”, then P is true (in which case it is raining) or P is false (it is not raining). Propositional logic does not admit any uncertainty about the truth of P, such as when it is so misty you could almost call it raining. Many people produce incorrect answers when given certain conditional-reasoning problems. Consider the following (affirmation of the consequent): Premises If Nancy is angry, then I am upset. I am upset. Conclusion Therefore, Nancy is angry. Interactive exercise: Conditional reasoning Many people accept the above conclusion as valid. However, it is not valid because I may be upset for some other reason (e.g., my football team has lost). Here is another problem in conditional reasoning: Premises If it is raining, then Nancy gets wet. It is raining. Conclusion Nancy gets wet. This conclusion is valid. It illustrates the rule of inference known as modus ponens: “If P, then Q” and also given “P”, we can validly infer Q. Another major rule of inference is modus tollens: from the premise “If P, then Q” and the premise “Q is false”, the conclusion “P is false” necessarily follows. Here is an example: Premises If it is raining, then Nancy gets wet. Nancy does not get wet. Conclusion It is not raining. 9781138482210_COGNITIVE_PSYCHOLOGY_PART_4.indd 673 28/02/20 4:14 PM 674 Thinking and reasoning People consistently perform much better with modus ponens than modus tollens: many people argue incorrectly that the conclusion to the above problem is invalid. Another inference involves denial of the antecedent: Premises If it is raining, then Nancy gets wet. It is not raining. Conclusion Therefore, Nancy does not get wet. Many people argue the above conclusion is valid although it is invalid. It does not have to be raining for Nancy to get wet (e.g., she might have jumped into a swimming pool). Traditionally, research on conditional reasoning was limited in three ways. First, unlike everyday life, it focused on disinterested reasoning (goals and preferences are irrelevant). For example, denial of the antecedent is invalid in traditional logic. In natural language, however, “If P, then Q” often means “If and only if P, then Q”. If someone says to you “If you mow the lawn, I will give you five dollars”, you are likely to interpret it to imply, “If you don’t mow the lawn, I won’t give you five dollars.” Second, traditional research typically involved instructions indicating that background knowledge was irrelevant. Nowadays, participants are generally not instructed to disregard their relevant knowledge with conditional-reasoning problems. Third, traditional research required participants to draw definite conclusions (true or false). In contrast, participants nowadays are often asked to assess the probability of the conclusion being true. This change is desirable because we often assign probabilities to conclusions in everyday life (Singmann et al., 2016). Theories Here we briefly discuss theories of conditional reasoning. More general theories of deductive reasoning are discussed later. Klauer et al. (2010) proposed a dual-source model of conditional reasoning. There is a knowledge-based process influenced by premise content where the subjective probability of the conclusion depends on individuals’ relevant know ledge. There is also a form-based process influenced only by the form of the premises. Verschueren et al. (2005) also proposed a dual-process model (other more general dual-process models are discussed later, pp. 683–690). They focused on individual differences in conditional reasoning more than Klauer et al. (2010). Some reasoners use a relatively complex counter example strategy: a conclusion is considered invalid if the reasoner can find a counterexample to it (this process is discussed later in the section on mental models, pp. 681–683). The other process is an intuitive statistical strategy based on probabilistic reasoning triggered by relevant knowledge. 9781138482210_COGNITIVE_PSYCHOLOGY_PART_4.indd 674 28/02/20 4:14 PM Reasoning and hypothesis testing 675 Findings Singmann et al. (2016) tested the dual-source model using many conditional-reasoning problems. For each problem, participants rated the likelihood of the conclusion being true on a probability scale running from 0% to 100%. Knowledge-based processes were more important than form-based ones. Overall, the model accounted very well for participants’ performance. De Neys et al. (2005) obtained evidence relevant to Verschueren et al.’s (2005) dual-process model. On some trials, participants were presented with few or many counterexamples conflicting with valid conclusions (modus ponens and modus tollens). According to classical logic, these counterexamples should have been ignored. In fact, however, participants were more likely to decide wrongly the conclusions were invalid when there were many counterexamples. Markovits et al. (2013) tested the dual-process model using problems involving affirmation of the consequent (where the conclusion is invalid). Here are two examples: (1) (2) If a rock is thrown at a window, then the window will break. A window is broken. Therefore, a rock was thrown at the window. If a finger is cut, then it will bleed. A finger is bleeding. Therefore, the finger was cut. Reasoners using the statistical strategy were influenced by the fact that the subjective probability that “If a finger is bleeding, it was cut” is greater than the probability that “If a window is broken, it was broken by a rock”. As a result, such reasoners accepted the invalid conclusion more often in problem (2) than (1). In contrast, reasoners using the counterexample strategy accepted the conclusion if no counterexample came to mind. They also accepted the invalid conclusion more often in problem (2). This was because it was easier to find counterexamples with respect to the conclusion of problem (1) (the window might have been broken by several objects other than a rock) than the conclusion of problem (2). According to the model, the counterexample strategy is more cognitively demanding than the statistical strategy. Accordingly, Markovits et al. (2013) predicted it would be used less often when participants had limited time. This prediction was supported: that strategy was used on 49% of trials with unlimited time but only 1.7% of trials with limited time. Markovits et al. (2017) gave their participants modus ponens inferences which are always valid. Each problem was presented with additional information indicating the relative strength of evidence supporting the inference (50%; 75%; 99%; or 100%). Markovits et al. compared groups of participants previously identified as using counterexample or statistical strategies. Markovits et al. (2017) found clear differences between the two groups (see Figure 14.1). Statistical reasoners were strongly influenced by relative strength when deciding whether to accept modus ponens inferences. In contrast, counterexample reasoners showed a sharp reduction in acceptance when some evidence failed to support the inference (e.g., the 99% and 75% conditions). These findings were as predicted. 9781138482210_COGNITIVE_PSYCHOLOGY_PART_4.indd 675 28/02/20 4:14 PM 676 Thinking and reasoning Mean number of MP inferences accepted Figure 14.1 Mean number of MP (modus ponens) inferences accepted (out of 3) as a function of relative strength of the evidence and strategy. From Markovits et al. (2017). 3 2.5 2 1.5 Counterexample 1 Statistical 0.5 0 100% 99% 75% 50% Relative strength Summary Research on conditional reasoning has become much more realistic. For example, reasoners are encouraged to use their relevant knowledge on reasoning tasks. They also assign probabilities to the correctness of conclusions rather than simply deciding conclusions are valid or invalid. Theoretically, it is assumed reasoners are influenced by the form of the premises. More importantly, however, their relevant knowledge and experience lead them to engage in probabilistic reasoning (dual-source model) or to try to find counterexamples to the stated conclusion (dual-process model). Wason selection task The Wason selection task has been studied intensively by researchers interested in deductive reasoning. However, it is more accurately described as a task involving hypothesis testing using a conditional rule. Research activity: In the standard version of the task, four cards lie on a table (R, G, 2, 7; Deductive reasoning see Figure 14.2). Each card has a letter on one side and a number on the other, and there is a rule applying to the four cards (e.g., “If there is an R on one side of the card, then there is a 2 on the other side of the card”). The participants’ task is to select only those cards needing to be turned over to decide whether or not the rule is correct. What is your solution? Most people select the R and 2 cards. If you did the same, you are wrong! You need to see whether any cards fail to obey the rule when turned over. From this perspective, the 2 card is irrelevant: if there Figure 14.2 is an R on the other side, this indicates only The Wason selection task. Rule: If there is an R on one side of the card, then there is a 2 on the other. that the rule might be correct. If there is any 9781138482210_COGNITIVE_PSYCHOLOGY_PART_4.indd 676 28/02/20 4:14 PM 677 Reasoning and hypothesis testing other letter on the other side, we have discovered nothing about the rule’s validity. The correct answer is to select the R and 7 cards, an answer given by only about 10% of university students. The 7 is necessary because it would definitely disprove the rule if it had an R on the other side. How can we explain performance on the Wason selection task? Several factors are involved. First, performance is worse with abstract versions of the task (as above) compared to concrete versions referring to everyday events (e.g., “Every time I travel to Manchester, I travel by train”). Ragni et al. (2018) found in a meta-analysis that the percentage of correct answers increased from 7% with abstract versions to 21% with concrete versions. Second, there is matching bias (the tendency to select cards matching the items named in the rule). Thompson et al. (2013a) obtained strong evidence for matching bias on the selection task. In addition, cards named in the rule were selected faster than other cards and produced greater feelings of rightness. Third, the logical solution to the Wason selection task conflicts with everyday life (Oaksford, 1997). According to formal logic, we should test the rule “All swans are white” by searching for swans and non-white birds. However, this would be extremely time-consuming because only a few birds are swans and the overwhelming majority of birds are non-white. It would be preferable to adopt a probabilistic approach based on the likely probabilities of different kinds of events or objects. The problem of testing the above rule resembles the Wason selection task, which has the form “If p, then q”. We should choose q cards (e.g., 2) when the expected probability of q is low but not-q cards when q’s expected probability is high to maximise information gain. As predicted, far more q cards were selected when the percentage of q cards was low (17%) than when it was high (83%) (Oaksford et al., 1997). Fourth, motivation is important. People are more likely to select the potentially falsifying card (7 in the original version of the task) if motivated to disprove the rule. Dawson et al. (2002) gave some participants the rule that individuals high in emotionality lability experience an early death. The four cards showed high emotional lability, low emotional lability, early death and late death, with the correct answer involving selecting the first and last cards. Of participants led to believe they had high emotional lability (and so motivated to disprove the rule), 38% solved the problem (versus 9% of control participants). Motivation is also involved with deontic rules (rules concerned with obligation or permission). Sperber and Girotto (2002) used a deontic rule relating to cheating. Paolo must decide whether he is being cheated when buying things through the internet: the answer is to select the “item paid for” and “item not received” cards (selected by 68% of participants). This unusually high level of performance was achieved because the motivation to detect cheating led participants to select the “item not received” card. In a meta-analysis, Ragni et al. (2018) found the correct answer was selected by 61% of participants with deontic versions but 7% using abstract versions. Marrero et al. (2016) proposed a general motivational approach accounting for the above findings. Individuals concerned about potential 9781138482210_COGNITIVE_PSYCHOLOGY_PART_4.indd 677 KEY TERMS Matching bias The tendency on the Wason selection task to select cards matching the items explicitly mentioned in the rule. Deontic rules Rules relating to obligation and permissibility. 28/02/20 4:14 PM 678 KEY TERMS Syllogism A type of problem used in deductive reasoning; there are two statements or premises and a conclusion that may or may not follow logically from the premises. Belief bias In syllogistic reasoning, the tendency to accept invalid but believable conclusions and reject valid but unbelievable ones. Thinking and reasoning costs focus on disconfirming evidence whereas those concerned about potential benefits focus on confirming evidence. Fifth, Ragni et al. (2018) evaluated 15 theories of relevance to Wason’s selection task. In a large-scale meta-analysis, they found Johnson-Laird’s (1983) mental model theory (discussed shortly, pp. 681–683) best predicted performance. In essence, this theory assumes that selections on Wason’s selection task depend on two processes: (1) (2) There is an intuitive process producing selections matching the reasoners’ hypothesis (e.g., selection of R in the version of the task shown in Figure 14.2). There is a more deliberate process producing selections of potential counterexamples to the hypothesis (e.g., selection of 7 in the same version). The extent to which reasoners search for counterexamples depends on factors discussed above including task content, instructions and so on. Syllogistic reasoning Syllogistic reasoning has been studied for over 2,000 years. A syllogism consists of two premises or statements followed by a conclusion. Here is an example: “All A are B; all B are C. Therefore, all A are C”. A syllogism contains three items (A, B and C), with one (B) occurring in both premises. The premises and conclusion all contain one of the following quantifiers: all; some; no; and some... not. When presented with a syllogism, you must decide whether the conclusion is valid assuming the premises are valid. The validity (or otherwise) of the conclusion depends only on whether it follows logically from the premises – the conclusion’s truth or falsity in the real world is irrelevant. Consider the following example: Premises All children are obedient. All girl guides are children. Conclusion Therefore, all girl guides are obedient. The conclusion follows logically from the premises. Thus, it is valid regardless of your views about children’s obedience. Findings Various biases cause errors in syllogistic reasoning. Of special importance is belief bias, the tendency to accept invalid conclusions as valid if believable and to reject valid (but unbelievable) conclusions as invalid (theoretical explanations are discussed later). Klauer et al. (2000) investigated belief bias thoroughly. The conclusions of half their syllogisms were believable (e.g., “Some fish are not trout”) whereas the others were unbelievable (e.g., “Some trout are not fish”). Half the syllogisms were valid 9781138482210_COGNITIVE_PSYCHOLOGY_PART_4.indd 678 28/02/20 4:14 PM Reasoning and hypothesis testing 679 and half invalid; however, some participants were told only one-sixth of the syllogisms were valid whereas others were told fivesixths were. What did Klauer et al. (2000) find? First, there was a base-rate effect: syllogistic reasoning performance was influenced by the perceived probability of syllogisms being valid (see Figure 14.3). Second, there was strong evidence for belief bias. Third, there was a belief-by-logic interaction. Performance on syllogisms with valid conclusions was better when those conclusions were believ able, whereas performance on syllogisms with invalid conclusions was worse when those conclusions were believable. In sum, reasoners’ performance was influenced by factors irrelevant to logic. Stupple and Ball (2008) found with syllogistic reasoning that unbelievable premises were processed more slowly than believable ones. This finding suggests people experienced conflict between their beliefs and what they were asked to assume and resolving this conflict was time-consuming. Some problems in syllogistic reasoning occur because of differences in the meanings of expressions in formal logic and everyday life. For example, we often assume “All As are Bs” means “All Bs are As” and “Some As are not Bs” means “Some Bs are not As”. Ceraso and Provitera (1971) spelled out such premises unambiguously (e.g., “All As are Bs, but some Bs are not As”). This greatly enhanced reasoning performance. In similar fashion, “some” means “some but not all” in everyday usage but “at least one and possibly Figure 14.3 Percentage acceptance of conclusions as a function of all” in formal logic. Schmidt and Thompson perceived base rate validity (low vs. high), believability of (2008) found syllogistic reasoning improved conclusions and validity of conclusions. when the meaning of “some” in formal logic Based on data in Klauer et al. (2000). © American Psychological Association. was made explicit. Finally, people’s syllogistic reasoning performance is influenced by whether the conclusion matches the premises in surface or superficial features. Here is an example of matching: no A are not B; no B are not C; therefore, no C are not A; and an example of non-matching: all A are B; all B are C; therefore no A are not C. In spite of the irrelevance of matching vs non-matching to formal logic, people are more likely to accept conclusions matching the premises (Stupple et al., 2013). 9781138482210_COGNITIVE_PSYCHOLOGY_PART_4.indd 679 28/02/20 4:14 PM 680 Thinking and reasoning IN THE REAL WORLD: INVALID DEDUCTIVE REASONING So far we have focused on laboratory-based examples of invalid deductive reasoning. However, such reasoning is also very common in everyday life. Here are a few examples starting with the politician’s syllogism, which is along the following lines: Premises We must do something to save the country. Our policy is something. Conclusion Our policy will save the country. Invalid conditional reasoning occurs frequently in everyday life. Earlier we discussed the logical fallacy known as denial of the antecedent. Here is a real-world example: Premises If you have got nothing to hide, you have nothing to fear. You have nothing to hide. Conclusion You have nothing to fear. The above argument is invalid because it implies that people are only interested in privacy because they have something to hide. In fact, of course, we all have a basic human right to privacy. Ironically, the authorities who argue strongly in favour of greater surveillance of the public are often notoriously reluctant to provide information about their own activities! Finally, we consider an everyday example of the logical fallacy known as affirmation of the consequent (discussed earlier, p. 673). Premises If the Earth’s climate altered throughout pre-human history, this was due to natural climate change. The Earth’s climate is currently altering. Conclusion Natural climate change is occurring currently. This is clearly an invalid argument. The fact that past climate change was not due to humans does not necessarily mean that current climate change is not due to human intervention. In sum, many groups in society (e.g. politicians; climate change deniers) are strongly motivated to persuade us of the rightness of their beliefs. This often leads them to engage in invalid forms of reasoning. The take-home message is that we all need to be sceptical and vigilant when exposed to their arguments. THEORIES OF “DEDUCTIVE” REASONING Here we will focus on two very influential theoretical approaches to deductive reasoning. First, there is Johnson-Laird’s mental model theory, which represents a relatively “traditional” approach. Second, we turn our 9781138482210_COGNITIVE_PSYCHOLOGY_PART_4.indd 680 28/02/20 4:14 PM 681 Reasoning and hypothesis testing attention to the increasingly popular dual-process approach. The word deductive in the title of this section has been put in quotation marks to indicate that individuals presented with deductive-reasoning problems often fail to use deductive processes when trying to solve them. Mental models Johnson-Laird (e.g., 1983; Johnson-Laird et al., 2018) argues that reasoning involves constructing mental models. What is a mental model? According to Johnson-Laird et al. (2015, p. 202), a mental model is “an iconic representation of a possibility that depicts only those clauses in a compound assertion that are true. The mental models of a disjunction, ‘A or B but not both’ accordingly represent two possibilities: possibly (A) and possibly (B)”. It is iconic because its structure corresponds to what it represents. Here is a concrete example of a mental model: KEY TERMS Mental models An internal representation of some possible situation or event in the world having the same structure as that situation or event. Principle of truth The notion that assertions are represented by forming mental models concerning what is true while ignoring what is false. Premises The lamp is on the right of the pad. The book is on the left of the pad. The clock is in front of the book. The vase is in front of the lamp. Conclusion The clock is to the left of the vase. According to Johnson-Laird (1983), people use the information contained in the premises to construct a mental model like this: book pad clock lamp vase The conclusion the clock is to the left of the vase clearly follows from the mental model. The fact we cannot construct a mental model consistent with the premises (but inconsistent with the conclusions) (i.e., we cannot construct a counterexample) indicates the model is valid. Here are the theory’s main assumptions: A mental model describing the given situation is constructed and the conclusions that follow are generated. An attempt is made to construct alternative models to falsify the conclusion by finding counterexamples to the conclusion. If a c ounterexample model is not found, the conclusion is deemed valid. The construction of mental models involves the limited resources of working memory (see Chapter 6). Reasoning problems requiring the construction of several mental models are harder than those requiring only one mental model because the former impose greater demands on working memory. The principle of truth: “Mental models represent what is true, but not what is false” (Khemlani & Johnson-Laird, 2017, p. 16). This minimises demands on working memory. 9781138482210_COGNITIVE_PSYCHOLOGY_PART_4.indd 681 28/02/20 4:14 PM 688 KEY TERM Meta-reasoning Monitoring processes that influence the time, effort and strategies used during reasoning and problem solving. Thinking and reasoning (1) (2) The more intelligent reasoners had greater difficulty resolving conflict when providing belief-based responses rather than logic-based responses. The less intelligent reasoners exhibited the opposite pattern. These findings suggest more intelligent individuals generate logic-based responses faster than belief-based ones, whereas less intelligent individuals generate belief-based responses faster. What conclusions should we draw? First, rather than arguing beliefbased responses involve fast Type 1 processing whereas logic-based responses involve slow Type 2 processing, we need to consider individual differences. Second, “If responses (belief- or logic-based) can be generated either quickly and effortlessly or slowly and deliberately, perhaps these responses merely differ on a single dimension, namely, complexity” (Newman et al., 2017, p. 1165). What causes Type 2 processing? What determines whether reasoners’ responses are based on analytic (Type 2) processing or whether they reflect only intuitive (Type 1) processes? The theories discussed above address this issue. Traditional serial models assume that Type 2 processes monitor the output of Type 1 processes and it is this monitoring process that determines whether reasoning performance is based on Type 2 processes. In similar fashion, many parallel models assume Type 2 reasoning is triggered when conflict monitoring (involving Type 2 processing) leads to conflict detection. What is puzzling about all these theories is that, “They assume that Type 2 processing is effectively caused by itself” (Pennycook et al., 2015, p. 36). Ackerman and Thompson (2017, p. 607) provided a detailed account of meta-reasoning: “The processes that monitor the progress of our reasoning and problem-solving activities and regulate the time and effort devoted to them” (see Figure 14.6). Monitoring processes assess the probability of success before, during and after performing a reasoning task. The most important monitoring feature is the feeling of rightness: “the degree to which the first solution that comes to mind feels right” (p. 608). Only when this feeling is weak do reasoners engage in substantial Type 2 or analytic processing. Thompson et al. (2011) studied the role of feeling-of-rightness ratings on the use of Type 2 processes with syllogistic and conditional-reasoning tasks. Participants provided an initial answer immediately after reading each problem (intuitive, or Type 1, answer) followed by an assessment of that answer’s correctness (feeling of rightness). Participants then had unlimited time to reconsider their initial answer and provide a final analytic, or Type 2, answer. As predicted, participants spent longer reconsidering their intuitive answer and were more likely to change it when they had low feelings of rightness. What determines feeling-of-rightness ratings? Thompson et al. (2013b) addressed this issue in a study on syllogistic reasoning. Participants produced the first response that came to mind, provided a feeling-of-rightness rating, and then produced a slower, more deliberate response. Feeling-of-rightness 9781138482210_COGNITIVE_PSYCHOLOGY_PART_4.indd 688 28/02/20 4:14 PM Reasoning and hypothesis testing Reasoning Timeline Meta-reasoning Identifiy components and goal Meta-cognitive monitoring Meta-cognitive control Assessment of knowledge and strategies Think? Search memory? Change strategy? Stop? Initial judgement of solvability Engage in solving? Give up? Feeling of rightness Provide the initial response? Reconsider? Intermediate confidence Provide current response? Try another strategy? Generate an initial, autonomous, response 689 Figure 14.6 The approximate time courses of reasoning and meta-reasoning processes during reasoning and problem solving. From Ackerman & Thompson (2017). Analytic processing Answer choice Final confidence Feeling of error Final judgement of solvability Provide chosen answer? “Don’t know”? Seek help? ratings were higher when the first response was produced rapidly rather than slowly, indicating the importance of response fluency. Most research indicates response fluency is a fallible measure of response accuracy. For example, people give higher feeling-of-rightness ratings to reasoning problems having familiar rather than unfamiliar content even when the former problems are harder (Ackerman & Thompson, 2017). Evaluation What are the strengths of the contemporary dual-process approach? First, dual-process theories have become increasingly popular and wide-ranging. For example, they provide explanations for syllogistic reasoning and conditional reasoning (Verschueren et al, 2005, discussed earlier, pp. 674–675). In addition, such theories account for findings in problem solving (see Chapter 12), judgement and decision-making (see Chapter 13). Second, “Dual-process theory... provides a valuable high-level framework within which more specific and testable models can be developed” (Evans, 2018, p. 163). Third, there have been increasingly sophisticated attempts to clarify the relationship between Type 1 and Type 2 processes (e.g., whether they are used serially or in parallel). Fourth, we have an enhanced understanding of meta-reasoning processes (especially those involved in monitoring). Fifth, recent theory and research are starting to take account of the flexibility of processing on reasoning problems due to the precise form of the problem and individual differences (Thompson et al., 2018). What are the limitations with the dual-process approach? First, the processes used by reasoners vary depending on their abilities and preferences, 9781138482210_COGNITIVE_PSYCHOLOGY_PART_4.indd 689 28/02/20 4:14 PM 690 Thinking and reasoning their motivation and their task requirements. Melnikoff and Bargh (2018; see Chapter 13) identified two ways many dual-process theories are oversimplified: (1) they often imply that Type 1 processes are “bad” and errorprone, whereas Type 2 processes are “good”; and (2) they assume many cognitive processes can be assigned to just two types. Second, “The absence of a clear and general definition of a Type 1 or Type 2 response does create difficulty for researchers wishing to test [dual-process] theories” (Evans, 2018, p. 163). For example, it is often assumed theoretically that fast responses reflect Type 1 processing and are error-prone whereas slow responses reflect Type 2 processing and are generally accurate. However, we have discussed various studies disconfirming those assumptions. Third, there has been a rapid increase in the findings that require to be explained theoretically, and theories have not kept pace with this increase. For example, meta-reasoning often plays an important role in influencing reasoners’ processing strategies and performance. As yet, however, no theorists have integrated meta-reasoning processes into a comprehensive dual-process theory of reasoning. BRAIN SYSTEMS IN REASONING Interactive feature: Primal Pictures’ 3D atlas of the brain In recent years, there has been increased research designed to identify the brain regions associated with deductive reasoning. Prado et al. (2011) reported a meta-analytic review of 28 neuroimaging studies on deductive reasoning (see Figure 14.7). They obtained evidence for a core brain system centred in the left hemisphere involving frontal and parietal areas. Specific brain areas activated during deductive reasoning included the inferior frontal gyrus, the medial frontal gyrus, the precentral gyrus and the basal ganglia. Coetzee and Monti (2018) reported findings broadly consistent with those of Prado et al. (2011). They used fMRI to assess brain activation while participants performed deductive-reasoning tasks. There were two key findings. First, core regions (left rostrolateral cortex, in BA10; medial prefrontal cortex, in BA8) were more strongly activated with complex (rather than) simple deductive reasoning. Second, the main language areas Figure 14.7 Brain regions most consistently activated across 28 studies of deductive reasoning. PG = precentral gyrus; MFG = middle frontal gyrus; PPC = posterior parietal cortex; IFG = inferior frontal gyrus; BG = basal ganglia; MeFG = medial frontal gyrus. From Prado et al. (2011). © Massachusetts Institute of Technology, by permission of the MIT Press. 9781138482210_COGNITIVE_PSYCHOLOGY_PART_4.indd 690 28/02/20 4:14 PM