Psychology of Thinking (2nd Edition) PDF
Document Details
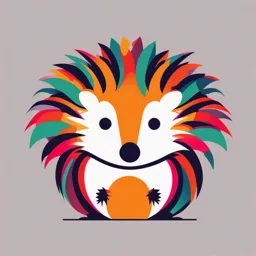
Uploaded by ChivalrousToucan3503
John Paul Minda
Tags
Summary
This is a psychology textbook, 2nd edition by John Paul Minda, covering reasoning, decision-making, and problem-solving. It explains inductive reasoning and generalization, and explores related phenomena.
Full Transcript
6 Inference and Induction At a fundamental level, when we think about “thinking”, we are often considering cognitive actions like figuring out a problem, or trying to predict how someone will react, or relying on our knowledge and existing conceptual representations to make sense of and interpret ne...
6 Inference and Induction At a fundamental level, when we think about “thinking”, we are often considering cognitive actions like figuring out a problem, or trying to predict how someone will react, or relying on our knowledge and existing conceptual representations to make sense of and interpret new events. Another way of describing this behaviour is that we rely on our past experiences to predict what might happen in the future in similar circumstances. In previous chapters I have given the example of shopping at a farmers’ market, so let's consider that example again. When I go to the farmers’ market, I rely on my past experiences to help me predict what to expect the next time I go. I expect there to be vendors, produce, other shoppers, and prepared food. If I pick up a butternut squash, I can predict what it will look like on the inside based on my prior knowledge of similar butternut squashes that I have purchased and processed in the past. The entire experience is one that relies heavily on prior knowledge to make predictions. The entire experience is one that is made predictable and manageable on the basis of prior knowledge. The inductions and predictions all seem straightforward and the process happens so quickly that we do not realize the power inherent in what we are doing. We are using the past to make predictions about the future. And usually these predictions come true so often that we don't even notice. And when our predictions don't come true, we often ignore or downplay evidence to the contrary. This is the power of inductive reasoning: induction is about predicting the future. Objectives On completing this chapter you should be able to achieve the following: Understand the basic definitions of induction, inference, and generalization. Be able to explain why some philosophers and psychologists believed that induction is a problem and why is it difficult to explain. Be familiar with the basic phenomena of inductive reasoning, how people behave in experimental settings, and with logical fallacies related to inductive reasoning. Understand and explain the two primary theories of inductive reasoning: similarity coverage and feature coverage. The Role of Induction in Thinking Inferences and conclusions Induction or inductive reasoning is a process that we use for many common activities and behaviours. For one, we use induction to make inferences. Inferences are conclusions based on the available or observable evidence. These conclusions might be used to make a prediction about a specific event. For example, I used to get telephone calls from telemarketers between the hours of 4:00 pm and 7:00 pm. (I don't get these calls as much anymore, primarily because I no longer have an old fashioned “land line” telephone.) Those hours between 4:00 pm and 7:00 pm would be a time when many but not all people are home from work or school, making and/or eating supper. When the phone would ring at that time, I usually made an inference or prediction that the caller was just trying to sell something, so I rarely picked up the call. Because this had happened in the past, I made enough observations to draw a reasonable conclusion about who would be on the phone. On the other side, the telemarketing company was relying on their evidence to make an inductive inference that I would be at my home phone between 4:00 pm and 7:00 pm. We were both making inductive inferences. Generalization Induction is also used when we make generalizations. A generalization is also an inductive conclusion, but rather than describing a specific prediction, as in the previous examples, generalization is a broad conclusion about a whole class or group of things. These generalizations inform the conclusions we make, and those conclusions affect our behaviour. If you see the sun rise every day, this will lead to a generalization that the sun always rises, and that generalization allows you to predict something about the sunrise tomorrow and the next day. If you enjoy a really good espresso at a particular café several times in a row, you will probably begin to form a generalization about that café and that will affect your expectations. On the other hand, if you had a bad dinner at a restaurant, you might form a general impression of its poor quality and that would affect your predictions about future meals and would reduce the likelihood that you would want to eat there. You are using your past experience to generate a mental representation, the generalization, that you will use to guide your behaviour. We also form generalizations about people based on our experience with one or more individuals. For example, let's consider the thinking behind football rivalries. In the UK, there are many fierce rivalries between football clubs in the premier league. Some of the rivalries are based on history of play and others are rivalries based on fan experience. Imagine a person who supports Arsenal FC and suppose that they have had less than positive experiences with some Chelsea FC fans (or the other way around: I'm not picking sides). Based on a few of those negative interactions, the Arsenal fan might form a negative generalization about Chelsea fans. That generalization may be based on limited evidence or even indirect evidence. This is the basis of many stereotypes and prejudices. One of the reasons that stereotypes are so difficult to overcome is that they arise from the basic cognitive processes that underlie all generalizations and inferences. In the examples above, whether or not the conclusion was made about a specific telemarketing call or a general conclusion about Arsenal or Chelsea fans, the evidence was specific. Inductive reasoning involves making specific observations and then drawing conclusions from that available evidence. It seems that we make inferences all the time. If you call a restaurant to place an order for a pick-up/delivery/takeaway, you make a basic inference that the food you order will be ready for you to collect. When the driver in front of you puts on his turn signal, you make an inference that he will turn left or right. We rely on induction to make inferences about how people will behave and react to what we say. We rely on induction to make inferences about how to use new ingredients when cooking dinner. Young children rely on induction when they pick up an object and learn about how size predicts the weight of objects. Parents make inductions when they predict how their young children might behave after a short nap or a long nap. The list is extensive because induction is such a critical aspect to the psychology of thinking. In summary, we rely on inductive reasoning to discover something new by thinking. How Induction Works Induction is central to our thinking. And as a result, philosophers and psychologists have been thinking about and studying induction for centuries. Let's look at the history of induction as an area of study. This history is fascinating because it's full of paradoxes and quandries and many of these ideas are still relevant today. Hume's problem of induction In the era of the Scottish Enlightenment, the philosopher David Hume considered induction to be one of the greatest problems for philosophers to solve. Unlike deductive logic, which I discuss in Chapter 7 and many contemporary philosophers believed could be explained by formal, mathematical operations, induction seemed to defy this. Hume gave a description of what he called the problem of induction. As we discussed, induction is essentially the act of relying on past experience to make inferences and conclusions about the future. Hume was concerned that this was a circular argument. The reason was as follows: induction works because we assume that the future will resemble the past in some way. We must have confidence in our judgements about the future. Hume claimed that this only worked because the future has always resembled the past … in the past. To say that the future has always resembled the past, in the past, might strike you as unnecessarily confusing. But what this means is that your inductions and conclusions were probably correct in the past. You might be able to recall inductions and conclusions that you made yesterday, two weeks ago, or two months ago, that turned out to be true. As a concrete example, if you were at a farmers’ market yesterday, and you made an inference about what the inside of a hubbard squash would look like, and your prediction was later confirmed, you could say that yesterday, the future resembled the past. The problem with this, according to Hume, is that we cannot use these past inductive successes to predict future inductive successes. We simply cannot know if the future will resemble the past. In other words, it is impossible to know if your inductions will work in the future as well as they worked in the past without resorting to the circular argument of using induction. Just because your inductive inferences worked yesterday, two weeks ago, two months ago, does not guarantee that they will work now, tomorrow, or two weeks from now. Induction is based on the understanding that the future will resemble the past, but we only have information about how well this has worked in the past. To make this assumption requires the acceptance of a circular premise. In essence, we are relying on induction to explain induction. By now, your head might hurt from considering all the past futures and future pasts, and you would be right. Hume concluded that from a strictly formal standpoint, induction cannot work. But it does work. Humans do rely on induction. This is why Hume considered induction a problem. There is no way for it to work logically, and yet we do it all the time. We rely on induction because we need to. Hume suggested that the reason we rely on induction is that we have a “habit” of assuming that the future will resemble the past. In a modern context, we might not use the term “habit”, but instead would argue that our cognitive system is designed to track regularities in the world and make conclusions and predictions on the basis of those regularities. Let's consider some of the fundamental mechanisms that allow induction to work. Basic learning mechanisms All cognitive systems, intelligent systems, and non-human animals rely on the fundamental processes of associative learning. There is nothing controversial about this claim. The basic process of classical conditioning provides a simple mechanism for how inductions might work. In classical conditioning, the organism learns an association between two stimuli that frequently co-occur. In Chapter 2 on similarity, we discussed the example of a cat that learns the association between the sound of the can of food opening and the subsequent presentation of her favourite food. The cat has learned that the sound of the can being opened always occurs right before the food. Although we tend to talk about it as a conditioned response, it is also fair to discuss this as a simple inductive inference. The cat doesn't have to consider whether or not it is reasonable that the future will resemble the past; she simply makes the inference and acts on the conditioned response. In other words, the cat makes a prediction and generates an expectation. Stimulus generalization Another conceptual advantage of relating induction to basic learning theory is that we can also talk about the role of similarity and stimulus generalization. Consider a straightforward example of operant conditioning. Operant conditioning, somewhat different from classical conditioning, is characterized by the organism learning the connection between a stimulus and a response. We can imagine a rat in a Skinner box learning to press a lever in response to the presentation of a colour. If a red light goes on and the rat presses the lever, it receives reinforcement in the form of rat food. If the blue light goes on and the rat presses the lever, it receives no reinforcement. Not surprisingly, the rat learns pretty quickly that it needs to press the lever only when the red light comes on. We can argue that the rat has learned to make inductive inferences about the presentation of food following various lights or even different colours. However, the rat can do more than just make a simple inference. The rat can also generalize. If you were to present this rat with a new colour that was slightly different from the original colour that it was trained on, it would probably still press the lever. Its rate of pressing might decrease, however. You would also find that the rate would decrease as a function of this similarity. The more similar the new colour is to the training colour, the higher the rate of lever pressing (Figure 6.1). This decrease is known as a generalization gradient. So pervasive is this generalization gradient that Roger Shepard referred to it as the universal law of stimulus generalization (Shepard, 1987). Not only is the rat making what amounts to an inductive inference about the relationship between lights and food, it is also making these inductive generalizations in accordance with the similarity between the current state of affairs and previously encountered instances. Figure 6.1 An example of a typical generalization gradient. The animal (a rat, for example) will press the lever vigorously when it sees a specific colour (the middle grey). The maximum lever press rate corresponds to the peak. The rat also presses the lever when colours that are similar to that colour are presented, but the rate of lever pressing drops off considerably as a function of decreasing similarity. What we see is that there is something fundamental and universal about generalizing to new stimuli as a function of how similar they are to previously experienced stimuli. This has implications for understanding induction. First, it strongly suggests that Hume was right: we do have a habit to behave as if the future will always resemble the past and this tendency is seen in many organisms. Second, our tendency to base predictions about the future on similarity to past events should also obey this universal law of stimulus generalization. If one's past experiences are very similar to the present situation, then inferences have a high likelihood of being accurate. As the similarity between the present situation and past experiences decreases, we might expect these predictions to have a lower probability of being accurate. Goodman's problem of induction Although stimulus association and generalization seem to explain how induction might work at the most basic level, there are still some conceptual problems with induction. According to Hume, induction may be a habit, but it is difficult to explain in logical terms without resorting to some kind of circular argument. Hume's concern was not so much with how induction worked, but rather that it seemed to be difficult to describe philosophically. Nelson Goodman, the twentieth-century philosopher, raised a very similar concern, but the example is somewhat more compelling and possibly more difficult to resolve (Goodman, 1983). Goodman's example is as follows. Imagine that you are an emerald examiner (I know it is not really a thing, but just pretend that it is). Every emerald you have seen so far has been green. So we can say that “All emeralds are green”. By ascribing to emeralds the property of green what we are really saying is that all emeralds that have been seen are green and all emeralds that have not yet been seen are also green. Thus, “emeralds are green” predicts that the very next emerald you will pick up will be green. This inductive inference is made with confidence because we have seen consistent evidence that it is true. Figure 6.2 This is a schematic of Nelson Goodman's example of the concepts of green and grue emeralds. As can be seen, all the emeralds set up and seen so far are simultaneously green and grue, and so both properties are true. Emeralds that have not yet been seen are to the right of the black line. Each property makes a different prediction about the subsequent emeralds to be examined. But there is a problem with this. Consider an alternative property called grue. And that if you say that “All emeralds are grue”, it means that all the emeralds you have seen so far are green and all the emeralds that have not yet been seen are blue: green emeralds in the past, but blue emeralds from this moment forward. Yes, this sounds a little ridiculous, but Goodman's paradox is that at any given time this property of grue is true. Both properties are true given the evidence of green emeralds. I've illustrated this in Figure 6.2. Notice that the past experience (green emeralds) is identical for both properties. Goodman's suggestion is that both of these properties, green and grue, can be simultaneously true, given the available evidence. It is possible that all the emeralds are green, and it is also possible that all emeralds are grue and that you've seen green-coloured ones but not the blue-coloured ones yet. But these properties also make opposite predictions about what colour the next emerald you pick up will be. If green is true, then the next emerald will be green. If grue is true, then the next emerald will be blue. And since both are true, a clear prediction cannot really be made. And yet, of course, we all predict that the next emerald will be green. Why? This is the problem of induction. Viewed in this way, induction is a problem because the available evidence can support many different and contradictory conclusions. Entrenchment and natural kinds With the earlier problem of induction defined by David Hume, the solution was straightforward. Hume stated that we have a habit of making inductions. And our current understanding of learning theory suggests that we naturally generalize. Goodman's problem of induction is more subtle because it assumes we do have this habit. If we have a habit to make inductions, how do we choose which one of the two possible inductions to make in the emerald example? A possible solution is that some ideas, descriptors, and concepts are entrenched and thus more likely to be the source of our inductions. Entrenchment means that a term or a property has a history of usage within a culture or language. And as we discussed earlier in Chapter 5, there is still considerable evidence that language can influence and direct our thinking. In the emerald example, green is an entrenched term. Green is a term that we can use to describe many things. It is a basic colour term in English. It has a history of usage within our language of being used to describe many different categories of things. And so it is the useful property to make predictions from, and about. By saying that a collection of things (emeralds) is green, we can describe all the things. Grue, on the other hand, is not entrenched. There is no history of usage and no general property of grue outside the emeralds that were grue yesterday and blue tomorrow. Unlike green, grue is not a basic colour term and does not apply to whole categories. Goodman argued that we can only make reliable inductions from entrenched terms and from coherent categories, and from natural kinds. The philosopher W.V.O Quine, in his essay “Natural kinds” (Quine, 1969), argued that natural kinds are natural groupings of entities that possess similar properties, much like what we have referred to earlier in Chapter 4 as a family resemblance concept (Rosch & Mervis, 1975). Quine suggested that objects form a kind only if they have properties that can be projected to all the members. For example, an apple is a natural kind. This is a natural grouping, and what we know about apples can be projected to other apples. “Not apple” is not a natural kind because the category is simply too broad to be projectable. This grouping consists of everything in the universe that is not an apple. Quine argued that all humans make use of natural kinds. Reliable inductions come from natural kinds. Granny Smith apples and Gala apples are pretty similar to each other and belong to the same natural kind concept. Anything you know about Granny Smith apples can be projected to Gala apples with some confidence, and vice versa. The same would not be true of Gala apples and a red ball. True, they may be similar to each other on the surface, but they do not form a natural kind. Whatever you learn about the Gala apple, can't be projected to the red ball. Quine's notion of a natural kind suggests a solution for Nelson Goodman's problem of induction. Quine pointed out that green is a natural property and that green emeralds are a natural kind. Because of this, the property of green can be projected to all possible emeralds. Grue, being arbitrary in nature, is not a natural kind and cannot be extended to all possible members. In other words, green emeralds form a kind via similarity; grue emeralds do not. Green emeralds are a coherent category, whereas grue emeralds are not. Green and grue might both be technically true, but only one of them is a coherent category, and natural kind, and a group with a consistent perceptual feature. And so we are able to make inductions about green emeralds and do not consider making inductions about grue emeralds. Categorical Induction If we consider the research discussed above, we can first conclude that most organisms have a tendency to display stimulus generalization. This can be as simple as basic conditioning or generalizing about a group of people. Second, basic stimulus generalization is sensitive to the similarity between the current stimulus and mental representations of previously experienced stimuli. Third, we have shown in other chapters that concepts and categories are often held together by similarity. As a result, a productive way to investigate inductive reasoning is to consider that inductions are often based on concepts and categories. This is known in the literature as categorical induction. By assuming that induction is categorical, we make an assumption that there is a systematic way in which the past influences future behaviour. The past influences the present and judgements about the future as as function of conceptual structure. The structure of an induction task For the present purposes, we can define categorical induction as the process by which people arrive at a conclusion or a statement of confidence about whether a conclusion category has some feature or predicate after being told that one or more premise categories possess that feature or predicate. This is just like our ongoing example about winter squashes. If you learn that a winter squash has fibres and large seeds inside, and then learn that the hubbard squash is a also winter squash, you use your knowledge about the category of winter squash and the features that are typical of that category to make the inductive inference. In this way, the conceptual structure of past knowledge influences your prediction that the hubbard squash will also have seeds. In many of the examples I discuss below, the induction is made in the form of an argument. The argument is a statement which contains one or more premises that support a conclusion. A premise is a statement of fact about something, someone, or a whole class. The premise contains predicates, which can be things and properties. In most of the examples, the predicates are properties or features that are common to the category members. The inductive argument also contains a conclusion statement. The conclusion is the actual inductive inference, and it usually concerns the possible projection of a predicate to some conclusion, object or category. In an inductive argument, participants would be asked to decide whether or not they agreed with the conclusion. They might be asked to consider two arguments and decide which of the two is stronger. For example, consider the inductive argument below, which first appeared in work by Sloman and Lagnado (2005). Argument Premise: Boys use GABA as a neurotransmitter Conclusion: Therefore girls use GABA as a neurotransmitter. The first statement about boys using GABA as a neurotransmitter is a premise. Boys are a category and the phrase “use GABA as a neurotransmitter” is a predicate. How strongly do you feel about this conclusion? Part of how you assess the strength has to do with whether or not you think girls are sufficiently similar to boys. In this instance, you would probably agree that they are pretty similar with respect to neurobiology, and therefore you would endorse the conclusion. When you were answering this question, you may wonder what GABA is beyond being a neurotransmitter. You may not have actually known what it is at all and may not have known whether or not it is present in boys and girls. So the actual answer to this conclusion is probably unknown. The statement is designed this way for a reason, though. The categorical induction statement works because it asks you to infer a property based on category similarity, rather than on retrieving the property from your semantic memory. Thus, in the example above, GABA is a blank predicate (Rips, 1975). It is a predicate because it is the property we wish to project. But it is blank because we do not assume to know the answer. It is plausible, but not immediately known. And because you cannot rely on your factual knowledge about GABA as a neurotransmitter, you will have to make an inductive inference on the basis of your knowledge about the categories (boys and girls in this case). Thus, the blank predicate is crucial in inductive inference research because it forces the participant to rely on categorical knowledge and induction, rather than on the retrieval of a fact from semantic memory. Research using this paradigm has described several strong phenomena regarding how people make categorical inductions. These include inductions and conclusions about specific cases and inductions and conclusions about whole categories. In most cases, the strength of the argument is dependent upon how similar the premise (or premises) is (or are) to the conclusion. And categorical structure plays a role as well. When we have evidence that a feature is associated with a member of a category that is highly typical, we tend to trust other inductions as well. Using this basic paradigm, we can investigate some general phenomena about categorical induction. Let's look at a few of these. Keep in mind that these phenomena tell us about how induction works, and by extension how concepts, categories, and similarity influence thinking and behaviour. Premise similarity For example, if the facts and features in the premise and the conclusion are similar to each other, are from similar categories, or are from the same category, inductive inferences can be made confidently. This is referred to as premise-conclusion similarity. According to Osherson et al. (1990), arguments are strong to the extent that the categories in the premises are similar to the categories in the conclusion. We are more likely to make inductive inferences between similar premise and conclusion categories. For example, consider the following two arguments (from Osherson et al., 1990): Argument 1 Premise: Robins have a high concentration of potassium in their bones. Conclusion: Sparrows have a high concentration of potassium in their bones. Argument 2 Premise: Ostriches have a high concentration of potassium in their bones. Conclusion: Sparrows have a high concentration of potassium in their bones. In this example, the high concentration of potassium in their bones is the blank predicate. This is the new property we are making an inductive inference about. Argument 1 should seem stronger, and in empirical studies research participants find this to be a stronger argument (Osherson et al., 1990). The reason is that robins and sparrows are fairly similar to each other; ostriches and sparrows are not very similar. The low similarity between the ostrich and the sparrow is evident on the surface, as is the high similarity between the robin and the sparrow. We assume that if the robin and the sparrow share observable features, they may also share nonobservable features like the concentration of potassium in the bones. Premise typicality The example above emphasized the role of similarity between the premise and the conclusion, but in the strong similarity case you may have also noticed that the robin is a very typical category exemplar. For all intents and purposes, the robin is one of the most typical of all birds. And remember that typical exemplars share many features with other category members. Typical category members have a strong family resemblance with other category members. And they can also be said to cover a wide area of the category space. What is true about robins is true of many exemplars in the bird category. Premise typicality can affect inductions about the whole category. For example, consider the following set of arguments: Argument 1 Premise: Robins have a high concentration of potassium in their bones. Conclusion: All birds have a high concentration of potassium in their bones. Argument 2 Premise: Penguins have a high concentration of potassium in their bones. Conclusion: All birds have a high concentration of potassium in their bones. In this case, you might agree that the first argument seems stronger. It is easier to draw a conclusion about all birds when you are reasoning from a typical bird like a robin, which covers much of the bird category, than from a very atypical bird like a penguin, which does not cover very much of this category. If we know that a penguin is not very typical – it possesses many unique features and does not cover very much of the bird category – we are not likely to project additional penguin features onto the rest of the category. We know that many penguin features do not transfer to the rest of the category. Premise diversity The preceding example suggests a strong role for typicality, because typical exemplars cover a broad range of category exemplars. But there are other things that can affect the coverage as well. For example, the premise diversity effect comes about when several premises are dissimilar to each other. Not completely unrelated, of course, but dissimilar and still in the same category. When being presented with two dissimilar premises from the same category, it can enhance the coverage within that category. For example, consider the two arguments below: Argument 1 Premise: Lions and hamsters have a high concentration of potassium in their bones. Conclusion: Therefore, all mammals have a high concentration of potassium in their bones. Argument 2 Premise: Lions and tigers have a high concentration of potassium in their bones. Conclusion: Therefore, all mammals have a high concentration of potassium in their bones. Looking at both of these arguments, it should seem that statement one is a stronger argument. Indeed, subjects tend to choose arguments like this as being stronger (Heit & Hahn, 2001; Lopez, 1995). The reason is that lions and hamsters are very different from each other, but they are still members of the same superordinate category of mammals. If something as different and distinct as a lion and a hamster have something in common, then we are likely to infer that all members of the superordinate category of mammal have the same property. On the other hand, lions and tigers are quite similar in that both are big cats, both appear in the zoo in similar environments, and they co-occur in speech and printed text very often. In short, they are not very different from each other. And because of that we are less likely to project the property of potassium in the bones to all mammals and are more likely to think that this is a property of big cats, or cats in general, but not all mammals. The diversity affect comes about because the diverse premises cover a significant portion of the superordinate category. The inclusion fallacy Sometimes, the tendency to rely on similarity when making inductions even produces fallacious conclusions. One example is known as the inclusion fallacy (Shafir et al., 1990). In general, we tend to prefer conclusions in which there is a strong similarity relation between the premise and the conclusion category. We tend to discount conclusions for which there is not a strong similarity relation between the premise and the conclusion. Usually, this tendency leads to correct inductions, but occasionally it can lead to false inductions. Take a look at the statements below and think about which one seems like a stronger argument. Argument 1 Premise: Robins have sesamoid bones. Conclusion: Therefore, all birds have sesamoid bones. Argument 2 Premise: Robins have sesamoid bones. Conclusion: Therefore, ostriches have sesamoid bones. It is easy to agree that the first argument seems stronger. Robins are very typical members of the bird category and we know they share many properties with other members of the bird category. And so it seems reasonable to conclude that if robins possess sesamoid bones, so too do all other birds. Most people find the second statement to be less compelling. Robins are typical, but ostriches are not. We know that robins and ostriches differ in many ways, so we are less willing to project the property of sesamoid bones from robins to ostriches. So this probably strikes us as reasonable and fair. And not as a fallacy. But it is a fallacy and another example of how our intuitions seem to guide us to made inferences that seem obvious but may not be correct. The reason why this is a fallacy is that all ostriches are included in the “all birds” statement. In other words, if we are willing to accept Argument 1 that a property present in robins is also present in all birds, then that inference includes ostriches already. It cannot be the case that a single member of the “all birds” category is a less compelling argument than all birds. If we are willing to project the property to an entire category, it is not correct to assume that specific members of that entire category do not have that property. Otherwise, we should not be willing to accept the first argument. But most people find the first argument to be more compelling because of the strong similarity of robins to other birds. Robins possesses features that are common to many other birds. We recognize that similarity and judge the inference to all birds accordingly. The atypicality of the ostrich undermines the argument. People are likely to use similarity relations rather than category inclusion when making these kinds of arguments. So similarity seems to be the stronger predictor of inferences. Category membership is important, but featural overlap may be even more important (Osherson et al., 1990; Shafir et al., 1990; Sloman, 1993, 2005). Causal factors Sometimes, we make inferences based on our understanding of how the world works. These might not be based on category inclusiveness or even similarity and feature overlap. Instead, causal relationships may play a role. Consider the following two arguments (Lo et al., 2002): Argument 1 Premise 1: House cats can carry the floxum parasite. Premise 2: Field mice can carry the floxum parasite. Conclusion: Therefore, all mammals can carry the floxum parasite. Argument 2 Premise 1: House cats can carry the floxum parasite. Premise 2: Tigers can carry the floxum parasite. Conclusion: Therefore, all mammals can carry the floxum parasite. Both of these arguments are fairly strong. And the first statement might seem to be an example of the diversity effect, because the two premises mention category members that are fairly diverse – house cats and field mice cover a fair amount of the mammal category. House cats and tigers are also diverse, but they are members of the same near superordinate category of cats. So a strict coverage model should predict that the first argument is stronger. However, people (children specifically) tend to find the first argument to be weaker (Lo et al., 2002). The reason is that most of us recognize why house cats and field mice might carry the same parasite. If the mice carry the parasite, and the cats catch the parasite from the mice while hunting, it suggests a causal link rather than a categorical link. This causal link is specific and idiosyncratic to this house cat/field mice relationship. As a result, it may not conform to Quine's notion of a natural kind. We are less likely to project the property of the parasite to all mammals on the basis of this evidence. Thus, the alternative argument concerning house cats and tigers seems stronger because there is no causal link between house cats and tigers. There is only a biological link between the two. Sometimes, this causal effect arises even when the same terms are used. A study by Doug Medin and colleagues asked subjects to consider a variety of premises (Medin et al., 2003). They found that when the order of presentation highlighted a causal relationship, people preferred that argument over the same terms when the order did not highlight the causal relationship: Statement 1 Premise: Gazelles contain the protein retinum. Conclusion: Therefore, lions contain the protein retinum. Statement 2 Premise: Lions contain the protein retinum. Conclusion: Therefore, gazelles contain the protein retinum. People generally prefer statements like the first one and rate that to be the stronger argument. In that statement, the order of terms highlights a plausible causal link. Gazelles have this protein, and so lions may also have this protein because they often hunt and eat gazelles. They ingest the protein from the gazelle. Mentioning gazelles first and lions second highlights this causal link. The second statement still mentions the same terms, but in a different order. When you read that lions contain this protein, and you are asked to infer that gazelles may also contain it, the causal explanation is downplayed and you are likely to reason from category membership. It is not a bad argument, but without the additional causal information, it is not as strong as the first one. Unlike the previous example, in this case the causal link strengthens the argument because we are not making conclusions about all members of a category. Category coherence Inductions can be made from concepts and categories on the basis of similarity between premises and the conclusion, but the character of the concept also plays a role. One of the ways to see this is to consider the role of category coherence. The coherence of a category is related to how well the entities in the category seem to go together. For example, police officer seems to be a fairly coherent category. We expect there to be a high degree of similarity among people who join the police force and we might expect to them to share features, traits, and behaviours. Upon hearing that someone is a police officer, we might feel confident in our predictions about how they might act and behave. But not all categories are that coherent. For example, restaurant waiter might seem much less coherent. Compared to police officer, there is probably more diversity in this category and more possible reasons why people would have the job. Maybe there is more variability in appearance and behaviour. As a result, we are less likely to predict how people might act or behave as a function of their being waiters. In other words, there may be a coherence effect in categorical induction in which we prefer to reason from the most coherent category available. This was studied directly by Andrea Patalano and her colleagues (Patalano et al., 2006). In their research, they considered social/occupational categories that were higher or lower in coherence. In order to make this determination, they first asked a group of research participants to rate categories on a construct called entitativity, which is a measure that takes into account how members of a category are expected to be alike, how informative it is to know that something is a member of a category, and whether or not members of a category might possess an inherent essence. Categories that are high in entitativity are thought to be highly coherent. Patalano et al. found that categories like soldier, feminist supporter, and minister were all highly coherent, whereas matchbook collector, country clerk, and limousine driver were low in coherence. The researchers then carried out an induction task in which people were asked to make predictions about people who were members of more than one categories. For example, imagine that the following information is true: Premise: 80% of feminist supporters prefer Coca-Cola to Pepsi. Premise: 80% of waiters prefer Pepsi to Coca-Cola. Premise: Chris is a feminist supporter and a waiter. Conclusions: What beverage does Chris prefer, Coca-Cola or Pepsi? People were asked to make an indictive decision and rate their confidence. They found that people in their experiments preferred to make inductions about the more coherent categories. That is, in the example above, people say that Chris was likely to prefer Coca-Cola. Because people view feminist supporter as the more coherent category, they preferred to base their inductions on that category. Theoretical Accounts of Categorical Induction The similarity-coverage model Several theoretical accounts have been developed to deal with the kinds of facts shown above. The first is the similarity-coverage model of Osherson and colleagues (Osherson et al., 1990; Shafir et al., 1990). This theory assumes that inductive inferences are made on the basis of a similarity between premise and conclusion, and the degree of coverage that premises have over the lowest-level category that includes all of the premises and the conclusion. This theory accounts for the natural tendency to project an object's attributes to other similar objects and to similar categories. It is also a natural tendency to activate the superordinate category when a premise is stated. That is, when given information about apples and pears, the fruit category can be assumed to be automatically activated. The similarity-coverage model accounts for things like the premise and conclusion similarity effect, the typicality effects, and the diversity effects. However, the similarity-coverage model in its basic form may have difficulties accounting for effects like the inclusion fallacy. In the inclusion fallacy, people tend to prefer similarity relations over category membership. The feature coverage theory The feature coverage theory (Sloman, 1993, 2005; Sloman et al., 1998) is similar to the similarity-coverage model in that it emphasizes premise and conclusion similarity. It differs from the similarity-coverage model in that it downplays the role of category inclusion. In other words, it reduces the role of similarity and category coverage and replaces it with the notion of feature coverage. When a premise and a conclusion are similar to each other, they share many features and thus there is a high degree of feature coverage. When two dissimilar premises are used, as in the diversity effect, they do not tend to share many features with each other, and thus there are more features activated overall, which strengthens the conclusion. Formally, this is equivalent to claiming category coverage, but the feature coverage theory explains how and why the category coverage effect works. It works by activating more features, and those features are eligible to be projected onto the conclusion category. The feature coverage theory handles the inclusion fallacy fairly well. Because it doesn't make the assumption that subjects will rely on category membership, it tends to predict that inductions will be strong on the basis of shared features. In the inclusion fallacy, “robin” activates several common bird features. We know that robin is typical, and so we are willing to agree with the conclusion that if robins have some new feature, it is probable that all birds have this feature. The feature coverage model predicts that we will not prefer the second argument when we are projecting features from robins to ostriches. Although technically still a logical fallacy, the feature coverage model explains why we prefer the first statement. There is very little feature overlap between ostriches and robins as birds. Induction in Real-World Scenarios Given how important induction is to thinking, it is worth looking at some examples of inductive reasoning in cases outside the laboratory. The specific examples we gave above demonstrate some of the key effects related to categorical induction and inductive reasoning in general. But they are also somewhat circumscribed and designed to illustrate and elicit these specific effects. It is also worth examining some cases of categorical induction in more naturalistic settings. As a starting point, let's consider a study by Medin et al. (1997). This paper will also be discussed in greater detail in Chapter 11 in the context of expertise. Although this study was conducted in the laboratory, the materials used were more naturalistic. In this case, the researchers were interested in reasoning and categorization in a very specific expert population: tree experts. They defined three kinds of expert: expert botanical taxonomists, who were all university faculty members with expertise in the classification of trees; landscape designers and architects, who were all experts in how trees should be planted and cared for; and city arborists and park maintenance personnel, who were all experts in the care of trees. Although all of these groups were experts, they all have specific goals in mind with respect to their expertise. The researchers predicted that these groups would classify trees with respect to these expert level goals, and might also display systematic differences in inductive reasoning. Subjects were asked to examine cards that had the name of a specific tree on it. They were then asked to sort those cards into as many groups or as few groups as they thought reasonable based on how the trees went together. One of the key findings, which will be discussed in Chapter 11 on expertise, is that the subjects tended to sort the trees into categories as a function of what kind of expert they were. Botanists sorted the trees primarily according to scientific taxonomy. Landscape architects and arborists chose more idiosyncratic sorting strategies, sometimes based on the specific goals they might have when planting trees. In other words, if you work with trees from a practical standpoint, your natural tendency might be to group them into categories like “weed tree” and “trees needing space”. These are understandable functional groupings, but may not reflect the best grouping for categorical induction. Certainly, a category of trees that may be treated as weeds is not the same kind of natural kind, according to Quine (1969), that “Japanese Maple” would be. Interestingly, when the same subjects were asked to make inductions about specific trees, Medin et al. (1997) created a series of forced-choice triads that pitted functional grouping against taxonomy. Subjects were then asked an induction question along the lines of “Suppose a new disease was discovered that affected the [target tree]. Would you be likely to see this disease in [choice A] or [choice B]?” On some trials, one of the choices might reflect the thematic or goal-oriented choice and the other would reflect a taxonomic choice. Not surprisingly, expert taxonomists tended to make inductions in accordance with the taxonomic category. Maintenance workers tended to reason in accordance with the genus level and folk taxonomy. For those cases when there might be a conflict between folk taxonomy and scientific taxonomy, maintenance workers often relied on their own goal-oriented classifications. In contrast, landscape architects relied on reasoning strategy that did not reflect their initial goal-oriented strategy for sorting the trees. Landscape architects tended to be quite flexible in their understanding of trees and also tended to make inductions on the basis of scientific taxonomy. In other words, although the landscapers sorted trees into goaloriented categories, it did not seem to undermine their understanding of natural kind-based induction. Representativeness heuristic Categorical induction is commonplace in social interactions. For example, the well-known representativeness heuristic occurs when people base their predictions about things, on the prototype of the category for which that thing is a member. The idea is that if valuable knowledge exists in our understanding of basic categories, then we tend to project the category's properties to individual members of the category. This is entirely within the scope of our current understanding of categorical induction. If we assume something is true of a category, we assume it can be true of individual members. We also know that sometimes individual category members may not resemble the prototype or the overall family resemblance. Even in a category with a strong family resemblance structure, the very definition of family resemblance assumes that there may be category members that differ on a number of features from other category members and the prototype. The most well-known examples are those described by Kahneman and Tversky (Kahneman & Tversky, 1973; Shafir et al., 1990; Tversky & Kahneman, 1983). One particularly famous example is the case of “Linda the bank teller”. It illustrates not only the effect of representativeness, but also an effect known as the conjunction fallacy. In this task, subjects are given a description and are asked to choose one of two options that reflect a conclusion about the person. For example: “Linda is 31 years old, single, outspoken, and very bright. She majored in philosophy. As a student, she was deeply concerned with issues of discrimination and social justice, and also participated in anti-nuclear demonstrations”. Which is more probable? Linda is a bank teller. Linda is a bank teller and active in the feminist movement. Not surprisingly, in the original study subjects chose option 2 (Tversky & Kahneman, 1983). The reason they chose option 2 was that she is representative of what many people considered to be the “feminist movement”. Yes, it is possible she is a bank teller as well, but we probably don't have a strong, coherent representation of that category. Think about this problem in reverse and you will see its connection to some of the earlier premise and conclusion statements we studied. To say that Linda is a bank teller suggests she is a member of a large and maybe not very distinct category. To say that she is a member of a bank teller category and the feminist movement suggests she is a member of a smaller, more distinct category. We tend to prefer that relationship, and project the properties consistent with the feminist movement on to Linda. The reason this is a fallacy is that both options include membership in the bank teller category. Formally, it cannot be the case that she is more likely to be a member of two categories (the conjunction) than either one of those single categories. In other words, the probability of being a member of one category is greater than the probability of being in the conjunction of two of them. And yet, because of our understanding of representativeness and a tendency to rely on that heuristic, we all prefer the second option. We tend to rely on feature coverage and similarity. Consider another well-known example, again from Kahneman and Tversky – the “engineer lawyer problem”. The original version appeared in 1973, and has appeared in other studies in other forms many times since then (Kahneman & Tversky, 1973). In the original and fundamental version, subjects were given some base rate information about how many people in a group of 100 were engineers and how many were lawyers. In one case, subjects were told to assume that of the 100, 30 were engineers and 70 were lawyers. In other words, there was a 70% base rate of lawyers in this group. They were then given the description of a person who was sampled at random from the group of 100: “Jack is a 45-year-old man. He is married and has four children. He is generally conservative, careful, and ambitious. He shows no interest in political and social issues and spends most of his free time on his many hobbies, which include home carpentry, sailing, and mathematical puzzles”. Subjects were then asked to estimate the probability that Jack was a lawyer or an engineer. If subjects were paying attention to base rates and category membership exclusively, they should have estimated the likelihood of Jack being an engineer at 30%. After all, most of the people in this group were lawyers, and any randomly sampled person should have a 70% probability of being a lawyer. Clearly, Jack does not conform to the representativeness of lawyers, and this description reads to us like a stereotypical engineer. Bear in mind that this study was carried out in the early 1970s; our understanding of engineers and lawyers has probably changed somewhat over the intervening decades. But the main point here is that the subjects seemed to have ignored the given base rate of information and focussed instead on features. Jack possesses features that are representative of engineers and thus we overestimate the likelihood of him being an engineer. When I present this study in a lecture, nearly everyone who has not seen the study before is incredulous. I typically get comments like “but he is obviously an engineer”, or “yes, we realize the base rate is 70% lawyer, but for any individual it's the features that matter!” And these students are right. A common criticism of this paradigm is that people rely on many sources of information to make judgements and inferences about things. We predict or project features on the basis of category membership, typicality, representativeness, shared features, causal relations, etc. Furthermore, other researchers have suggested that our reliance on representativeness versus base rates can be affected by the way the problem is framed. In cases where the features are stereotypical, subjects tend to rely on representativeness. But in other cases where the features might be less extreme or stereotypical, or where sampling is explicitly random, people may rely on base rates more often (Gigerenzer et al., 1988). Even Daniel Kahneman has more recently suggested both pros and cons for representativeness. He points out that representativeness has many virtues. The intuitive impressions that one makes via rapid access to category-level information are more accurate than chance alone. As an example, Kahneman suggests that, on most occasions, people who act friendly actually are friendly. And that people with a PhD are more likely to subscribe to the New York Times than people with a high school diploma only. These are facts and they also conform to representativeness (Kahneman, 2011). Still, Kahneman also argues that representativeness can lead people to make erroneous inferences. One of the biggest problems is that because the influence of category-level information is so strong, we may overlook other, more rational sources of information. Consider again Kahneman's example of the New York Times and education level. If you see someone on a subway in New York City reading the New York Times, which alternative is more probable? She has a PhD or she does not have a college degree? Although we might agree that having a PhD is representative of readers of the New York Times, it would not be a rational inference given the general base rate of New York Times readership in New York City. So although the representativeness is based in fact and may often be a helpful and unavoidable inductive heuristic, it still can result in biases and errors. If we examine our own behaviour, we might see examples of representativeness all around. For example, we may know that close to 50% of all initial marriages end in divorce. But we certainly would not assume that half of our friends will get divorced, especially if they seem happy. Furthermore, if one's own marriage is happy and enjoyable, one would not predict a 50% failure rate. For example, my wife and I have been married for twenty years and we have an enjoyable, happy relationship. As a result, I do not assume that our marriage has a 50% chance of ending in a divorce. My personal knowledge overrides this base rate statistic. In other words, I believe my own marriage is representative of a happy marriage that is not likely to end. Representativeness affects many of our choices and decisions. We often choose things based on what they remind us of, or on what category they are representative of. I might go to the wine shop and pick out a new wine based on its attractive packaging. The attractive bottle is representative of a quality product. And I would do this even if I knew that there was a low base rate of wines that I would enjoy. Of course, we apply representativeness heuristics in ways far less benign than the lawyer and engineer example. Representativeness is also at the heart of many destructive and negative racial and ethnic stereotypes. The base rate of people who follow the Muslim religion and that also engage in terrorist activity is incredibly low (almost no one is a terrorist, regardless of religion or ethnicity). Nevertheless, many people, and even many elected government officials, tend to overestimate the connection between Muslims and terrorism. This is clearly an example of the representativeness heuristic in action and suggests a reasoning error. Summary Inductive logic and inference are cornerstones of human thinking. As was discussed early in this chapter, the tendency to make inferences is rooted in fairly primitive associative mechanisms. Inferential behaviour can be observed in non-human species – rats, birds, primates, etc. It would be safe to argue that all living organisms make inferences. An inference allows an organism to generate expectations, make predictions, and learn from past experience. This is not only a central aspect of human thought, it is also a central aspect of thought in general. Induction is in many ways what keeps us from living in an absolute present. The topic covered in this chapter connects with topics covered earlier. The idea of categorical induction relies not only on the formation of concepts and categories, which was covered in Chapter 4, but also on the general principle of similarity, covered in Chapter 2. As a general rule, the more similar the current situation or event is to some previously experienced situation or event, the easier it is to make inferences, and the more likely we are to trust those inferences and inductions. In Chapter 5, one aspect of language processing we discussed was the tendency to need to generate expectations when hearing or reading a sentence. For the most part, our linguistic inferences help us to reduce the inherent ambiguity in language, even though it occasionally produces a false inference, such as the “garden path sentence”. The psychology of inferential reasoning suggests that many of our internal representations are structured and designed in order to facilitate inductive inference. In many ways, this is the main function of a concept. As with other aspects of human thinking, the design features of our conceptual representation system, similarity, and inferential prediction system allow us to behave adaptively and to make predictions with a minimal amount of computation and maximize benefits. In many cases, we rely on cognitive shortcuts like the representativeness heuristic. This heuristic is adaptive but occasionally produces errors in reasoning. Subsequent chapters, specifically Chapters 8–10, will explore these kinds of heuristics in greater detail. Questions to Think About Why do we rely so strongly on categories and concepts when we engage in induction? Is this something specific to inductive reasoning or is this more representative of higher-order thinking in general? Take a look at your own thinking and examine whether or not you rely on heuristics like representativeness. Do you find that you tend to base inferences and predictions on concepts you are familiar with? Do you tend to judge people and things according to the categories you think they are most representative of? Do you think that Hume's concerns about induction still hold? Is it a problem for our understanding of thinking that a formal explanation of induction is based on circular premises?