IS4242 Intelligent Systems & Techniques Churn Management PDF
Document Details
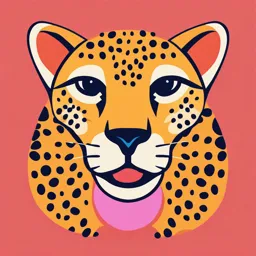
Uploaded by DexterousFern6890
National University of Singapore
Aditya Karanam
Tags
Summary
This document is a lecture on Churn Management, particularly targeting topics relevant to intelligent systems and techniques. The presentation details factors causing churn, its management techniques, and the use of neural networks in predicting churn.
Full Transcript
IS4242 INTELLIGENT SYSTEMS & TECHNIQUES L8 – Churn Management Aditya Karanam © Copyright National University of Singapore. All Rights Reserved...
IS4242 INTELLIGENT SYSTEMS & TECHNIQUES L8 – Churn Management Aditya Karanam © Copyright National University of Singapore. All Rights Reserved. Announcements ▸ The group project will be released today ‣ Due: 5-Nov, 11:59 PM (~1 month) ‣ Peer reviews are used for grading, please do not sabotage your teammates ▸ No lecture, only tutorial (at 4 pm) on 22nd October ‣ The recorded video of lecture will be posted on Canvas ▸ Midterm Feedback will be released tomorrow ‣ Please provide your input by Saturday IS4242 (Aditya Karanam) 2 In the coming weeks… ▸ Customer Churn Management (Week - 8) ▸ Social Media Analytics (Weeks – 9, 10) ‣ Targeting customers is good, but we also need to address their individual issues ▸ Competitor Analysis (Week – 11) ▸ Fairness in AI (Week – 12) ▸ Tutorials: MLP + Deep Neural Networks (week-9, 10) and Graph Analytics (Week – 11), Revision on Week – 12. IS4242 (Aditya Karanam) 3 In this class… ▸ Churn Management ‣ Types of Churn ‣ Involuntary and Voluntary (deliberate and incidental) ‣ Factors Causing Churn ‣ Customer satisfaction, switching costs, etc. ‣ Managing Churn ‣ Untargeted and targeted (reactive and proactive) ▸ Predicting churn ‣ Neural networks IS4242 (Aditya Karanam) 4 Customer Churn ▸ Customers can leave and not naturally return without significant re-acquisition costs ▸ At the firm level, churn is the percentage of the firm’s customer base that leaves in a given time period ▸ At a customer level, churn refers to the probability that a customer leaves the firm at a given point in time ‣ Churn is therefore 1 minus the retention rate: 𝑐 = 1 − 𝑟 IS4242 (Aditya Karanam) 5 Customer Churn ▸ Churn management focuses on the retention component of the customer life time value (LTV) ∞ 𝑚 𝑡 r𝑡 ∞ 𝑚𝑡 (1−𝑐)𝑡 𝐿𝑇𝑉 = σ𝑡=0 = σ𝑡=0 (1+𝛿)𝑡 (1+𝛿)𝑡 ▸ Churn is of a significant concern to a firm in an industry, especially in the digital world ‣ Example: In App Store, apps released in 2009 were abandoned after two years on average. However, apps released in 2014 and 2015 were abandoned within three months on average IS4242 (Aditya Karanam) https://techcrunch.com/2016/06/21/the-apple-app-store-graveyard/ 6 Types of Churn ▸ There are two major types of customer churn: “voluntary” and “involuntary.” baby products ▸ Involuntary churn: company deciding to terminate the relationship, typically because of poor payment history ▸ Voluntary churn: customer decides to terminate the relationship ‣ “Deliberate” voluntary churn: customer is dissatisfied or has received a better competitive offer ‣ “Incidental” voluntary churn: customer no longer needs the product or has moved to a location where the company does not offer service IS4242 (Aditya Karanam) 7 Major Factors Causing Churns ▸ Customer satisfaction ▸ Switching costs ▸ Network Effects ▸ Competition IS4242 (Aditya Karanam) 8 Customer Satisfaction ▸ More satisfied customers should be less likely to churn, i.e., have higher lifetime durations ‣ “Fit-to-needs” is an important consideration in customer satisfaction ▸ Product Customization can increase satisfaction ‣ One-size-fits-all approach to services marketing often leads to lack of fit and results in lower satisfaction and churn ▸ Strong promotional incentives may lead to lower satisfaction ‣ Maybe due to the acquisition of the wrong customers, i.e., customers for whom this is not their best choice. IS4242 (Aditya Karanam) 9 Customer Satisfaction ▸ Information source used in choosing the product can influence churn ‣ By influencing customer expectations and perceived “fit-to-needs” ▸ Customers are less likely to churn if they use experiential information in making their choices ‣ More accurate expectations and more knowledgeable choice ▸ Information from external sources such as advertisements is associated with more churn ‣ It may not be accurate with one’s own experience IS4242 (Aditya Karanam) 10 Switching Costs ▸ Switching costs, or the lack of them, are another reason for churn. ‣ If it does not cost the customer much to switch to a competitor, the customer is more likely to churn. ‣ Switching costs can take two forms – psychological and physical ▸ Psychological switching costs: how a brand or a product makes the consumer feel ‣ Examples: Association with the brand name, perception of customer service, etc. ▸ Physical switching costs include real inconveniences due to switching. ‣ Example: To terminate a Singtel mobile line early one must pay termination fees, prior written notice of 4 days, etc. IS4242 (Aditya Karanam) 11 Switching Costs: Experiment ▸ An interesting natural experiment on physical switching costs occurred in the wireless telephone industry, related to “number portability.” ▸ Before the fall of 2003 customers who switched carriers would have to change their telephone numbers as well. ▸ As of the fall of 2003, customers were allowed to retain their old numbers. ▸ 22% of cell phone users were interested in switching carriers if they could retain their current phone number ‣ Only 9% were interested in churning if they could not keep the number IS4242 (Aditya Karanam) 12 Network Effects ▸ Network effects: Consumer prefers a product that a lot of other users also prefer ‣ Telephone is valuable only when a lot of customers also use the telephone ‣ Similar phenomenon for Facebook, Dropbox, etc. ▸ Network effects influence consumer choice, while switching costs create consumer lock-in IS4242 (Aditya Karanam) 13 Competition ▸ Competitive offers and opportunities are considered to be the major cause of churn ▸ Competition can come from both within and outside the industry or product category ‣ Google (Waymo) is a competitor BMW ‣ Online Bankers may worry about regular bankers ‣ Dial-up ISP providers may worry about Broadband internet services ‣ When broadband providers offered significant internet speed dail-up ISPs had a significant churn IS4242 (Aditya Karanam) 14 Competition ▸ However, there is little empirical verification on this effect due to several factors ▸ Companies often have little direct information on competitive offers ▸ More important, difficult to identify competition! ‣ Usual suspects: Companies listed in the Security and Exachange Commission (SEC) filings ‣ Facebook argues that TikTok is their competitor, but the US Federal Trade Commission (FTC) argues that there are no competitors for Facebook ‣ More on this in week - 11 not 11 is 12 IS4242 (Aditya Karanam) 15 Reducing or Managing Churn ▸ Two major approaches to reducing churn: targeted or untargeted ▸ Untargeted approaches try to increase customer satisfaction or increase customer-switching costs ‣ Involves improving the overall product, mass advertising, or loyalty programs. ▸ Targeted approaches: identify customers most likely to churn and attempt to “rescue that customer.” ‣ Reactive or Proactive in nature IS4242 (Aditya Karanam) 16 Reactive Churn Management ▸ Reactive approaches wait for the customer to identify him/herself as a likely churner ‣ For example: Customer has called to cancel the service, the company now takes a corrective action ▸ Perfect prediction, or at least near-perfect in identifying churners ‣ Hence, the company can afford a significant incentive to keep the customer. ▸ This behavior may “train” the customer to call whenever they have a competing offer, expecting the company to match or exceed that offer IS4242 (Aditya Karanam) 17 Proactive Churn management ▸ A proactive approach identifies, in advance, customers most likely to churn ‣ Management uses predictive models to identify would-be churners ▸ Imperfect predictive accuracy – depending on the quality of the churn model ‣ Company can’t spend as much money per incentive as in a reactive program, since some of it may be wasted. ▸ Another potential concern for proactive churn management programs is that they might stimulate non-would-be churners to contemplate churning IS4242 (Aditya Karanam) 18 Proactive Churn management ▸ The customer had a “latent need” to churn that was identified by the predictive model ▸ The proactive contact enabled the customer to recognize that need. ▸ Some proactive programs have been found to increase churn rather than decrease it! ‣ E.g: Encouraging customer to switch to cost-minimizing plans led to more churn (Ascarza et al. 2016) ▸ Important to highly accurate predictive models to circumvent these challenges IS4242 (Aditya Karanam) 19 Predicting Churn: Neural Networks © Copyright National University of Singapore. All Rights Reserved. 20 Churn ▸ Let 𝑌 be a random variable representing whether a customer leaves the company ▸ 𝑃(𝑌 = 1) > 𝑃(𝑌 = 0) P(Y=1) P(Y=1) = > 1(odds ratio) P(Y=0) 1− P(Y=1) P(Y=1) log( ) > 0 (log-odds ratio) 1− P(Y=1) IS4242 (Aditya Karanam) 21 From Linear Models to Neural Models ▸ We can model the problem using logistic regression ‣ To get better prediction accuracy, we will use a Neural Network model ‣ Referred to as Multi-layer Perceptron or Feed Forward Neural Networks ▸ A neuron can be viewed as a generalization of a linear model ▸ Neural networks are combinations of neurons, they are versatile and powerful learning machines IS4242 (Aditya Karanam) 22 From Linear Models to Neural Models ▸ In logistic regression, the probability of label y = 1 for a feature vector x: 𝑒 𝛽0 +𝛽1 𝑥1 +𝛽2 𝑥2 + …+𝛽𝑝 𝑥𝑝 𝑒 𝜷𝑿 1 𝑃 𝑦 = 1 𝑋, 𝛽 = 𝛽0 +𝛽1 𝑥1 +𝛽2 𝑥2 + …+𝛽𝑝 𝑥𝑝 = = 1+𝑒 1+𝑒 𝜷𝑿 1+𝑒 −𝜷𝑿 ‣ Where 𝑿 = 1 𝑥1 𝑥2 … 𝑥𝑝 ; 𝜷 = [𝛽0 𝛽1 𝛽2 … 𝛽𝑝 ] (row vectors) 𝑝 ‣ Dot Product: 𝜷𝑿 = σ𝑗=0 𝑥𝑗 𝛽𝑗 , where 𝑥0 = 1 IS4242 (Aditya Karanam) 23 Neuron ▸ Let us call the coefficients 𝜷 as weights and denote them by 𝑾 ‣ 𝑾 = [𝑤0 𝑤1 𝑤2 … 𝑤𝑝 ] ‣ The intercept 𝛽0 becomes 𝑤0 and is called bias. 𝑒 𝑤0 +𝑤1 𝑥1 +𝑤2 𝑥2 + …+𝑤𝑝 𝑥𝑝 𝑒 𝑊𝑿 1 𝑃 𝑦 = 1 𝑋, 𝑊 = 𝑤0 +𝑤1 𝑥1 +𝑤2 𝑥2 + …+𝑤𝑝 𝑥𝑝 = = 1+𝑒 1+𝑒 𝑊𝑿 1+𝑒 −𝑾𝑿 1 ▸ Logistic or sigmoid function: 𝜎 𝑧 = , where 𝑧 = 𝑾𝑿 1+𝑒 −𝒛 ‣ Note: 𝑾𝑿 is the dot product IS4242 (Aditya Karanam) 24 Linear Models to Neural Networks: Steps ▸ Take input feature vector X = [1 𝑥1 𝑥2 … 𝑥𝑝 ] with an additional 1 to include bias in next step ▸ Dot product with weight vector 𝑾 = 𝑤0 𝑤1 𝑤2 … 𝑤𝑝 to get σ𝑝𝑗=0 𝑤𝑗 𝑥𝑗 = 𝑾X 1 ▸ Apply sigmoid function to the dot product to get the class label 1+𝑒 −𝑾𝑿 IS4242 (Aditya Karanam) 25 Graphical Representation ▸ Take input feature vector X = [1 𝑥1 𝑥2 … 𝑥𝑝 ] with an additional 1 to include bias in next step 𝑝 ▸ Dot product with weight vector 𝑾 = 𝑤0 𝑤1 𝑤2 … 𝑤𝑝 to get σ𝑗=0 𝑤𝑗 𝑥𝑗 = 𝑾X 1 ▸ Apply sigmoid function to the dot product 1+𝑒 −𝑾𝑿 to get the class label IS4242 (Aditya Karanam) 26 Generalization 1: Activation Function ▸ 𝑓 – Activation function that can be chosen to determine the type of output IS4242 (Aditya Karanam) 27 Activation Functions ▸ An activation function is typically a non-linear transformation. ▸ Examples: ‣ Sigmoid: squashes output to [0, 1] ‣ Tanh: squashes output to [-1, 1] ‣ ReLU: zero for negative inputs, identity for positive. 𝑓 𝑧 = max(0, 𝑧) IS4242 (Aditya Karanam) 28 Generalization - 2 ▸ Neurons can be combined to form layered architectures ▸ Each hidden layer is a (non-linear) transformation of the inputs: can be viewed as a (non-linear) feature transformation. Hidden layer: intermediate results, Edges: Weights (except final output) IS4242 (Aditya Karanam) 29 Layers: Composition of Functions ▸ Input: X = [1, 𝑥1 , 𝑥2 , … , 𝑥𝑝 ] ▸ Outputs of layer 1 = Inputs of layer 2: 𝐟 = [f1 𝒘𝒇𝟏𝑿 , f2 𝒘𝒇𝟐 𝑿 , f3 𝒘𝒇𝟑 𝑿 , f4 𝒘𝒇𝟒𝑿 ] ▸ Outputs of layer 2 = Inputs of layer 3: 𝐠 = [g1 𝒘𝒈𝟏 𝐟 , g 2 𝒘𝒈𝟐 𝐟 , 𝑔3 𝒘𝒈𝟑 𝐟 ] ▸ Final output: y = h(𝒘𝒉𝒈) IS4242 (Aditya Karanam) 30 Generalization – 3: Loss Function ▸ Also called cost function or objective. ▸ The function we want to optimize (minimize/maximize) to train our model, i.e., obtain values of weights in the network. ▸ Recall the objectives for these models: ‣ Logistic Regression: Likelihood ‣ 𝐿 𝛽 = 𝑃 𝑌 𝑋, 𝛽 = ς𝑛 𝑖=1 𝑝 𝑦𝑖 = ς𝑛 𝑖=1 𝜎 𝛽𝑋 𝑦𝑖 (1 − 𝜎 𝛽𝑋 )1−𝑦𝑖 ‣ Linear Regression: Squared Error: σ𝑛𝑖=1(𝑦𝑖 −𝑦 𝑖 ) 2 IS4242 (Aditya Karanam) 31 Loss Function ▸ We use cross entropy as a loss function for binary classification ‣ Compares two discrete distribution ‣ 𝐻 𝑝, 𝑞 = − σ𝑏 𝑝 𝑏 log(𝑞(𝑏)) ‣ Summation is over possible values (𝑝(𝑏), 𝑞(𝑏) are probabilities of 𝑏) ▸ For binary classification, 𝑝 denotes true label and 𝑞 denotes predicted label, ‣ Example: Logistic Regression ‣ 𝐻 𝑝, 𝑞 = − σ𝑖 𝑝𝑖 log 𝑞𝑖 = − σ𝑖 𝑦𝑖 log 𝑦ෝ𝑖 + (1 − 𝑦𝑖 ) log 1 − 𝑦ෝ𝑖 = − σ𝑖 𝑦𝑖 log 𝜎 𝛽𝑋𝑖 + 1 − 𝑦𝑖 log 1 − 𝜎 𝛽𝑋𝑖 = −log(𝐿(𝛽)) ▸ Maximizing likelihood = Minimizing cross-entropy loss ▸ Can be generalized to multi-class classification (b takes on more than 2 values). IS4242 (Aditya Karanam) 32 Loss Functions ▸ Regression: Mean Squared Error ▸ Classification: Cross Entropy ▸ Many other loss functions based on the task at hand IS4242 (Aditya Karanam) 33 Neural Networks ▸ Neurons: a transformation of inputs; exact transformation depends on the choice of activation function ▸ Network Architecture: a combination of neurons, that creates more complex non-linear features ▸ Loss function: determined by task, the function we want to optimize to train the network IS4242 (Aditya Karanam) 34 Neural Networks: Training ▸ Training: computing the weights. ‣ Gradient Descent! ‣ A differentiable loss function allows us to calculate gradients easily. IS4242 (Aditya Karanam) 35 Gradient Descent ▸ Algorithm to find the local minimum of a loss function: 𝑓(𝑊), 𝑤: weights (𝑡) Notation: superscript t denotes 𝑡 𝑡ℎ iteration ▸ Initialize 𝑊 for iteration 𝑡 = 0 ▸ Repeat until convergence: 𝑊 (𝑡+1) = 𝑊 (𝑡) − 𝛼∇𝑓(𝑊 (𝑡) ) ‣ ∇𝑓(𝑤 (𝑡) ): The gradient points along the direction in which the function increases most rapidly ‣ −∇𝑓 𝑤 𝑡 : Direction of the steepest descent ‣ 𝛼: step size or learning rate IS4242 (Aditya Karanam) 36 Gradient Descent ▸ Initialize 𝑊 (𝑡) for iteration 𝑡 = 0 ▸ Repeat until convergence: 𝑊 (𝑡+1) = 𝑊 (𝑡) − 𝛼∇𝑓(𝑊 𝑡 ) IS4242 (Aditya Karanam) 37 Gradient Descent: Initialization ▸ Initialize 𝑊 (𝑡) for iteration 𝑡 = 0 ▸ Repeat until convergence: 𝑊 (𝑡+1) = 𝑊 (𝑡) − 𝛼∇𝑓(𝑊 (𝑡) ) ▸ Initialization Matters! IS4242 (Aditya Karanam) 38 Gradient Descent: Learning Rate ▸ Too small: convergence is too slow ▸ Too large: may overshoot the minimum IS4242 (Aditya Karanam) 39 Different Variants of Gradient Descent ▸ 𝑤 (𝑡+1) = 𝑤 (𝑡) − 𝛼∇𝑓(𝑤 (𝑡) ) ▸ In gradient descent: gradients can be computed using entire training data ‣ Accurate but really slow ▸ In stochastic gradient descent: using one training datapoint (in SGD) in each iteration ‣ Faster, but less accurate ▸ Intermediate strategy: compute gradients using a batch of training data points. IS4242 (Aditya Karanam) 40 Training ▸ Compute the weights through gradient descent ▸ Layer wise propagation of information in Neural Networks ▸ Updating all the weights in all layers can be done efficiently though repeated use of chain rule: Backpropagation algorithm IS4242 (Aditya Karanam) 41 Backpropagation: Intuition ▸ 𝑓 = 𝑥 + 𝑦 𝑧 is a function of three variables ‣ 𝑓 = 𝑞 × 𝑧 ,𝑞 = 𝑥 + 𝑦 𝜕𝑓 𝜕𝑓 𝜕𝑞 𝜕𝑓 𝜕𝑓 𝜕𝑞 ▸ From chain rule: = , = 𝜕𝑥 𝜕𝑞 𝜕𝑥 𝜕𝑦 𝜕𝑞 𝜕𝑦 ▸ Inputs: 𝑥 = −2; 𝑦 = 5; 𝑧 = − 4 ▸ Forward pass: ‣ 𝑞 = 𝑥 + 𝑦 = 3, Computational graph ‣ 𝑓 = 𝑞 × 𝑧 = -1 ▸ Backward pass: 𝜕𝑓 𝜕𝑓 ‣ Second Layer: = 𝑧 = −4, =𝑞=3 𝜕𝑞 𝜕𝑧 𝜕𝑓 𝜕𝑓 𝜕𝑞 𝜕𝑓 𝜕𝑓 𝜕𝑞 ‣ First Layer: = = −4 ∗ 1 = −4, = = −4 ∗ 1 = −4 𝜕𝑥 𝜕𝑞 𝜕𝑥 𝜕𝑦 𝜕𝑞 𝜕𝑦 IS4242 (Aditya Karanam) 42 Backpropagation ▸ Libraries like TensorFlow and PyTorch can very efficiently perform backpropagation using tensors and Automatic Differentiation tools ▸ Values are passed around as tensors (a generalization of matrices to higher dimensions) ▸ Automatic Differentiation: a set of tools to compute derivatives of functions in computational fluid dynamics, engineering design optimization and machine learning. ▸ We will use PyTorch in our class IS4242 (Aditya Karanam) 43 Neural Networks: Summary ▸ Neural Network Architecture ‣ Different layers of neurons ‣ Different types of activation functions ▸ Loss Function: Determined by the task ▸ Training: Backpropagation using gradient descent ‣ Learning rate and initialization matter IS4242 (Aditya Karanam) 44 References ▸ Churn management: ‣ Robert C. Blattberg, Byung-Do Kim, Scott A. Neslin, (2010). Database Marketing: Analyzing and Managing Customers. (Chp. 24) ‣ Ascarza et al. (2016) The Perils of Proactive Churn Prevention Using Plan Recommendations: Evidence from a Field Experiment, http://dx.doi.org/10.1509/jmr.13.0483 ▸ Neural Networks: ‣ http://cs231n.github.io ‣ http://neuralnetworksanddeeplearning.com/index.html ‣ http://www.deeplearningbook.org IS4242 (Aditya Karanam) 45 Thank You © Copyright National University of Singapore. All Rights Reserved.