Exposure to Natural Hazard Events and Disaster Risk Reduction Policies - PDF
Document Details
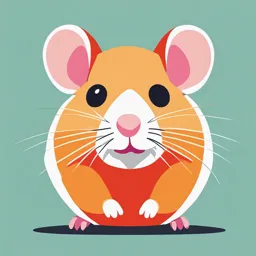
Uploaded by InstrumentalBluebell
Loyola Marymount University, Stanford University
2021
Daniel Nohrstedt
Tags
Summary
This study investigates the relationship between natural hazard events and policy changes for disaster risk reduction in 85 countries over eight years. The results indicate that event frequency and severity are generally not associated with improved disaster risk reduction policies, controlling for income levels and the types of hazards. However, variability in policy progress exists among countries, despite similar levels of hazard exposure.
Full Transcript
ARTICLE https://doi.org/10.1038/s41467-020-20435-2 OPEN Exposure to natural hazard events unassociated with policy change for improved disaster risk reduction 1234567890():,; Daniel Nohrstedt 1,2 ✉, Maurizio Mazzoleni2,3, Charles F. Parker 1,2 & Giuliano Di Baldassarre 2,3 Natural hazard events prov...
ARTICLE https://doi.org/10.1038/s41467-020-20435-2 OPEN Exposure to natural hazard events unassociated with policy change for improved disaster risk reduction 1234567890():,; Daniel Nohrstedt 1,2 ✉, Maurizio Mazzoleni2,3, Charles F. Parker 1,2 & Giuliano Di Baldassarre 2,3 Natural hazard events provide opportunities for policy change to enhance disaster risk reduction (DRR), yet it remains unclear whether these events actually fulfill this transformative role around the world. Here, we investigate relationships between the frequency (number of events) and severity (fatalities, economic losses, and affected people) of natural hazards and DRR policy change in 85 countries over eight years. Our results show that frequency and severity factors are generally unassociated with improved DRR policy when controlling for income-levels, differences in starting policy values, and hazard event types. This is a robust result that accounts for event frequency and different hazard severity indicators, four baseline periods estimating hazard impacts, and multiple policy indicators. Although we show that natural hazards are unassociated with improved DRR policy globally, the study unveils variability in policy progress between countries experiencing similar levels of hazard frequency and severity. 1 Department of Government, Uppsala University, 75120 Uppsala, Sweden. 2 Centre of Natural Hazards and Disaster Science (CNDS), c/o Department of Earth Sciences, Uppsala University, 75236 Uppsala, Sweden. 3 Department of Earth Sciences, Uppsala University, 75236 Uppsala, Sweden. ✉email: daniel. [email protected] NATURE COMMUNICATIONS | (2021)12:193 | https://doi.org/10.1038/s41467-020-20435-2 | www.nature.com/naturecommunications 1 ARTICLE F NATURE COMMUNICATIONS | https://doi.org/10.1038/s41467-020-20435-2 loods, wildfires, storms, and other natural hazard events interact with societal vulnerability to cause human casualties, property damage, and economic loss. Reducing disaster losses is directly linked to the achievement of the sustainable development goals (SDGs), including targets to build resilience and reduce vulnerability to climate-related extreme events (SDG1, target 1.5) and to reduce losses from these events in terms of deaths, people affected, and economic impacts (SDG11, target 11.5)1,2. While much research has been directed at understanding variability in hazard losses related to income, geography, and level of democracy3–5, few studies have yet examined natural hazards as drivers of policy change to reduce disaster risk around the world6. When the Hyogo Framework for Action (HFA) was adopted in 2005, it marked an important milestone in the development of an international policy regime to support countries in their efforts to reduce the risk from natural hazards. Coordinated through the United Nations Office for Disaster Risk Reduction (UNISDR, currently UNDRR), the HFA for the first time provided a set of attainable policy actions for enhancing legal and institutional resources and creating enabling environments for stakeholder collaboration in the pursuit of disaster risk reduction (DRR)7. Generally, such policy directives have a low chance of succeeding because of well-known rigidities characterizing policymaking, including inertia, path dependency, and incrementalism8. Due to these hurdles, the dominant theoretical explanation for policy change has been the occurrence of external shocks, including extreme natural hazard events. Cataclysmic events can spur policy change by raising public consciousness, redistributing political resources among policy actors, and providing impetus and legitimacy to governmental action9. Policy change has been attributed to repeated events that gradually build pressure for reform or high-magnitude events whose social and economic impacts cannot be ignored by policymakers10,11. This perspective, here labeled the “disaster-reform hypothesis,” is well established in several research fields, figuring prominently in public policy12, environmental management13, resilience14, and adaptation, transformation, and transitions15,16 in the pursuit of sustainable development17,18. Other studies suggest that extreme hazard events are unlikely to trigger major policy change. For instance, research19,20 has shown that countries regularly exposed to large-scale natural hazard events tend to devote resources to recovery at the cost of developing proactive DRR policies. Hazard events can also spark political contestation and become portrayed as policy failures, encumbering impartial diagnosis, learning, and system improvement, and can incentivize political and bureaucratic leaders to restore order, which may reaffirm pre-existing policies21. Despite these competing insights and expectations, systematic empirical research investigating the policy effects of natural hazards has been sparse. Prior work primarily draws from single or small-N case studies and has concluded that some hazard events trigger significant policy change while others reinforce the status quo and even increase the vulnerability of communities22–24. A few systematic studies25–29 have analyzed the influence of past natural hazards on damage reduction as a proxy for adaptation and also reported mixed results. Comparative studies have documented socio-political effects of natural hazards, but this work is limited to a few phenomena, including civil and political unrest11,30, armed conflict31, political regime change23, elections32, community memory33, and climate change discourse34. However, while the impact of natural hazard events on policymaking, specifically policy change, is extensively theorized and contested in the scientific literature, conclusive empirical evidence is lacking regarding the relationship from a global perspective. This lack of knowledge is unfortunate, given 2 that the frequency and intensity of some of these events are likely to increase in a changing climate20. This study aims to empirically explore if frequency (number of events) and severity factors (fatalities, people affected, and economic losses) of natural hazards influence national-level changes in DRR policy. To test this, we combine data on 85 countries collected from the HFA regime with disaster event data and explore whether the frequency or severity of natural hazard events have influenced national-level DRR policymaking worldwide. Data were retrieved from four evaluation cycles in the HFA covering the 2007–2015 period, focusing on countries’ selfreported progress on a scale from 1 (minor achievement) to 5 (comprehensive achievement), in implementing 22 key activities detailed by the HFA. Activities were listed under five priority for action (PFA) areas, specifying measures and principles for enhancing disaster resilience (Supplementary Table 1). Since the data is self-reported, we performed a validation test for two selected countries with the highest policy change scores (Swaziland and Chile), including assessing their HFA progress scores and level of policy change (see “Methods”; Supplementary Results). DRR policy change was measured as changes in the average of all five PFAs combined and for each PFA separately. These data were combined with information retrieved from the International Disaster Database (EM-DAT)35, which is the most widely used global natural hazard events database capturing the date, location, and impacts in terms of economic losses and the number of persons killed, injured, and affected36. Given the observation that the nature and severity of past hazard events can influence policy learning37, hazard frequency and severity measures were normalized in relation to long-term country averages in four baseline periods. The analysis also considered that the frequency and severity of natural hazard events are relative to countries’ experience of past events at different time scales. Finally, we controlled for income levels, differences in PFA values reported in the first two evaluation cycles, and hazard event types as potential confounding variables. Results The HFA data provide a valuable opportunity to measure policy change through clearly defined starting conditions (average progress scores in the first two HFA evaluation cycles) based on concrete policy efforts for longitudinal cross-country comparison38. For each country, DRR policy change was measured as the difference between the average HFA progress scores in the five PFAs between two main periods in 2007–2011 and 2011–2015 (Eqs. (1) and (2), “Methods”). DRR policy change and relationships with natural hazard events. Regarding patterns of DRR policy change, measured by changes in average PFA values, we find that policies in PFA3, which cover the use of knowledge, innovation, and education, changed the most over the study period (0.25 on average). The second largest change occurred in PFA4, which addresses the reduction of underlying risk factors (0.22) followed by PFA2, which deals with disaster risk and early warning (0.19). Policies in PFA1, which concern DRR as a national and local priority, including institutional conditions for implementation, and, PFA5, which focuses on measures to strengthen preparedness, changed the least (0.15 and 0.16, respectively). The majority of the countries (n = 63, 74%) reported positive policy change (a shift from lower progress scores in 2007–2011 to higher scores in 2011–2015—defined here as “improved” DRR policy), 6 (7%) were defined as status quo (same scores in both periods), whereas NATURE COMMUNICATIONS | (2021)12:193 | https://doi.org/10.1038/s41467-020-20435-2 | www.nature.com/naturecommunications NATURE COMMUNICATIONS | https://doi.org/10.1038/s41467-020-20435-2 17 (20%) had negative changes (a decline from higher to lower scores). Next, we compared these policy changes in the 2011–2015 period with natural hazard event frequency and severity measures in the 2007–2011 period. Figure 1 plots policy changes by country in relation to fatalities (Fig. 1a), affected people (Fig. 1b), economic losses (Fig. 1c), and the number of events (Fig. 1d). Average PFA changes refer to aggregated measures of change in the five PFAs in the 2007–2015 period (Eqs. (1) and (2), “Methods”). Severity and frequency measures are normalized in relation to the 30-year baseline period, i.e., estimating whether frequency and severity measures in the study period were above or below average in a 30-year period between 1980 and 2011. Here severity and frequency values >1 (x-axis) indicate that countries in the 2007–2011 period experienced hazard events that were more severe and/or more frequent compared to average frequency/ severity in the 1980–2011 period (see “Methods”). Figure 1 classifies countries by income levels to account for possible wealth effects, particularly the assertion that lower-income countries generally have less ability to adapt to extreme events39–41. Based on these results, we finally highlight pairs of two candidate cases per factor for future comparison and in-depth study. We find that both the level and variability of policy change— independent of exposure to natural hazard events—is lower on average among high-income countries (average PFA change = 0.12, standard deviation = 0.23) compared to countries at lowerincome levels. Although there are examples of outlier cases, represented by high-income countries with high average PFA changes (for example, Chile, average PFA change = 0.85, Fig. 1) as well as low-income countries with relatively small or negative average PFA changes (for example, Togo, average PFA change = −1.21, Fig. 1), Fig. 1 shows that most high-income countries are located close to the status quo threshold indicating policy stability (i.e., average PFA change = 0, Fig. 1a–d). This stands in contrast to countries at lower-income levels, which displayed more substantial PFA changes on average and greater variability in terms of standard deviation values (low income = 0.19, SD = 0.56; lower–middle income = 0.26, SD = 0.48; upper–middle income = 0.17. SD = 0.45). However, given that policy change is measured as the difference between progress scores on the five-point scale, these differences between income levels are relatively negligible. Results reported in Fig. 1 rely on a relatively simple measure of DRR policy change, based on the average progress of all five PFAs combined. Furthermore, these results are based on the 30-year baseline for normalizing frequency and severity and thus do not show results based on other baselines. To explore potential differences across individual PFAs, Fig. 2 relates average changes in each PFA to different levels of hazard severity (Fig. 2a–c) and frequency (Fig. 2d) on an aggregated level. We also show results for these policy changes in relation to all four baseline periods (1970 [40 years], 1980 [30 years], 1990 [20 years], and 2000 [10 years]). By comparing results across the four baselines, we are able to examine whether policy impacts of natural hazard events depend on different measures of frequency and severity, respectively. Negative difference values in Fig. 2 (y-axis) indicate that countries exposed to a greater number or more severe events relative to the long-term country averages reported lower levels of policy change than countries with fewer and less severe events. Conversely, positive difference values indicate higher policy change levels in the former group of countries compared to the latter (Eq. (4), “Methods”). Figure 2 shows, contrary to the disaster-reform hypothesis, that an increase in hazard frequency and severity is generally followed by less policy change. To the extent there was change, it tended to be negative. In fact, countries experiencing more frequent and severe hazard events reported lower levels of DRR policy change ARTICLE compared to countries with fewer and less severe events. This result is also depicted in Fig. 1, which illustrates that several countries with high frequency (Fig. 1d) and severity scores (Fig. 1a–c) had low or even negative policy change values. For instance, we find several examples of countries that experienced economic losses higher than the long-term average (Fig. 1c) but reported PFA change close to 0, among them Mexico, Vietnam, Australia, Cuba, Japan, Romania, Malaysia, New Zealand, and Thailand. As shown in Fig. 2, the pattern is relatively similar across the five PFAs and the four baseline periods, yet some interesting variations can be noted. The five PFAs display relatively similar differences between the two groups of countries in relation to fatalities (Fig. 2a), affected people (Fig. 2b), and the number of events (Fig. 2d). In comparison, there is more variance between the five PFAs in relation to economic losses (Fig. 2c). It is shown here that hazard events causing greater economic losses are, in fact, associated with lower levels of policy change compared with events causing less economic loss. This suggests that the positive relationship between economic losses and policy change reported in some case studies37,42 does not apply globally when focusing on DRR policy. The pattern regarding policy impacts of economic losses, however, is most pronounced for PFA3 involving the use of knowledge, innovation, and education in support of safety and resilience (difference values below −0.2 for all baseline periods, Fig. 2c). This is in contrast to policies in PFA1 (DRR as a national and local priority) and PFA5 (measures to strengthen preparedness), which changed more after hazard events with greater economic losses compared to events generating less economic damage. Results are similar for affected people (Fig. 2b). Yet, for both of these factors, the results vary depending on which baseline periods are used to normalize hazard frequency and severity. The predominance of negative values suggests that the average level of policy change has been lower in countries facing more frequent and severe hazard events than countries with fewer and less severe hazards. Testing the robustness of these results, we first conducted correlation analyses for all measures, including separate analyses of policy change in relation to different combinations of hazard event types (Supplementary Table 2). Results confirmed that the relationship between hazard events and policy change is very weak and slightly negative across hazard frequency and the three severity measures. Only a few significant differences were found across income levels and hazard event types (results based on 30year baseline). Next, we controlled for cross-country variance in HFA starting values (i.e., that countries reported different initial progress scores in any of the first two HFA evaluation cycles) by calculating the average PFA change ratio between 2011–2015 and 2007–2011 over the average PFA change in 2007–2011 (Eq. (3), “Methods”). Analyses with the normalized PFA measure did not generate different results compared to average PFA changes (Fig. 1 and Supplementary Fig. 2) and PFA difference scores (Fig. 2 and Supplementary Fig. 3). Thus, we find that differences in initial PFA scores did not influence the relationship between natural hazard exposure and policy change within the study period. Country cases. Although the study shows that no relationship exists between natural hazard events and DRR policy change in our sample of 85 countries, we can still observe considerable differences when comparing across individual countries. These differences provide opportunities to strategically select countries for in-depth comparisons. Different selection logics are possible, and Fig. 3 exemplifies two of the alternatives. To reiterate, since hazard severity and frequency depend on countries’ previous NATURE COMMUNICATIONS | (2021)12:193 | https://doi.org/10.1038/s41467-020-20435-2 | www.nature.com/naturecommunications 3 ARTICLE 4 NATURE COMMUNICATIONS | https://doi.org/10.1038/s41467-020-20435-2 NATURE COMMUNICATIONS | (2021)12:193 | https://doi.org/10.1038/s41467-020-20435-2 | www.nature.com/naturecommunications NATURE COMMUNICATIONS | https://doi.org/10.1038/s41467-020-20435-2 ARTICLE Fig. 1 Average aggregated policy changes in relation to natural hazard event frequency and severity measures. Plots demonstrating the relationship between fatalities (a), affected people (b), economic loss (c), number of events (d), and average changes in aggregated HFA PFAs by income levels in the World Bank’s fiscal year 2015 (red = low income; orange = lower–middle income; yellow = upper–middle income; blue = high income). Country acronyms are provided in the Source Data file. Frequency and severity measures are normalized against the 30-year country baseline. Normalized index ≤1 indicates that hazards are less or equally frequent and severe as the 30-year baseline long-term average. Conversely, normalized index values >1 represent more frequent and severe events than the 30-year baseline. Frequency and severity scales have been shortened for readability, to the effect that some countries are excluded from a–d. Countries not shown in a: Chile (CHL, normalized fatality score = 2.54), Australia (AUS, 2.94), Japan (JPN, 3.27), and Samoa (WSM, 4.13); b: Uruguay (URY, normalized affected people score = 6.26) and Macedonia (MKD, 4.90); c: Chile (CHL, normalized economic loss score = 5.47), New Zealand (NZL, 7.68), Thailand (THA, 8.06), and Malaysia (MYS, 5.18); d: Turks and Caicos Islands (TCA, normalized number of events score = 2.0). Source Data are provided as a Source data file. Fig. 2 Differences in policy changes in relation to levels of hazard event severity and frequency. Plot comparing changes in average values of the Hyogo Framework for Action Priority for Action areas (PFA), indicating self-reported achievement measures for enhancing disaster resilience. Policy changes are displayed for fatalities (a), affected people (b), economic losses (c), and frequency (d) and are estimated as the difference of the average PFA values of the countries that experienced normalized hazard measures >1 (higher frequency/intensity than in the four baseline periods) and the average PFA values of the countries in which the normalized hazard measures is 1 indicate that countries in the 2007–2011 period experienced more severe and/or more frequent hazards than average over the baseline period. Utilizing this approach, we analyzed the data in relation to four baselines periods as benchmarks to test the sensitivity of the statistical results for different hazard events occurring in a period spanning four decades. Figure 2 compares results across all four baselines to discern potential effects from using different time periods that normalize each measure. In other parts of the study (Figs. 1 and 3), we present results based on the 30-year baseline, which represents a reasonable compromise between excluding older events data with potential reliability issues75 and ensuring a sufficiently large sample of historical events. Estimation of policy change. To generate DRR policy change scores for each country and measurement period, we first calculated the difference between the average of the 22 core indicators within the five PFA areas as: National versus local- and regional-level policy action. Our results concerning national-level DRR policies should be contrasted with the potential effects of hazard events on local and regional level policy action69. Studies show that transformations after natural hazards often take place locally, emanating from adaptive efforts by households and civil society groups without the involvement of public actors17. External evaluations of the HFA framework, however, suggest that the regime produced limited local impact70. Although local policy responses are indirectly accounted for in our study (local priorities and risk assessments are acknowledged within PFA1 and PFA2, see Supplementary Table 1), this is an area where more work is warranted. Regional-level policymaking can also be studied in relation to hazard events. Useful reference cases include, e.g., the development of sustainable flood prevention policy within the European Union in the wake of catastrophic floods in 200271 and the development of the Indian Ocean Tsunami Warning and Mitigation System after the 2004 tsunami72. where p is each specific PFA area, c is the country, and t1 and t2 are the four HFA evaluation cycles divided into two periods: 2007–2011 (evaluation cycles 1 and 2 combined) and 2011–2015 (evaluation cycles 3 and 4). Aggregated PFA values were calculated as the average value of policy goals in the HFA evaluation cycle periods 2007–2009 and 2009–2011 for t1 and periods 2011–2013 and 2013–2015 for t2. Next, we estimated the aggregated change of PFAs for each country (Fig. 1) as: Time frame. The time period covered here excludes policy changes undertaken prior to the first HFA evaluation cycle in 2007–2009. Many countries adopted policies before the HFA was initiated to reduce disaster risks with a focus on prevention. Therefore, it is possible that some countries entered the HFA Averaging was employed to cope with the fact that not all countries submitted national progress reports for each evaluation cycle. Thus, if a country completed only one evaluation cycle, we calculated the PFA value based on the scores from that cycle. In order to filter the influence of the PFA value in the evaluation cycles 8 p p ΔPFApc ¼ PFAc ðt2 Þ PFAc ðt1 Þ; ΔPFAc ¼ 5 1X ΔPFApc : 5 p¼1 NATURE COMMUNICATIONS | (2021)12:193 | https://doi.org/10.1038/s41467-020-20435-2 | www.nature.com/naturecommunications ð1Þ ð2Þ ARTICLE NATURE COMMUNICATIONS | https://doi.org/10.1038/s41467-020-20435-2 periods 2007–2011, we calculated a normalized PFA change as: p ΔPFApc ¼ p PFAc ðt2 Þ PFAc ðt1 Þ : ð3Þ p PFAc ðt1 Þ Analysis. Once policy change scores and hazard measures were calculated for the different periods, we filtered the countries that were in both datasets to compile a unique, consistent dataset. The dataset included countries that: (a) completed at least one of the two evaluation cycles in the first HFA period (t1) and one cycle in the second period (t2) (n = 94) and (b) experienced hazard events in the 2007–2011 period (n = 85). Nine countries (Anguilla, Armenia, Bahrain, British Virgin Islands, Finland, Monaco, Saint Kitts and Nevis, Seychelles, and Sweden) with valid PFA change scores, but no recorded hazard events within the exposure period, were excluded for the final sample of 85 countries. Due to missing data in the EMDAT database, the sample size differs regarding hazard event frequency (n = 85), fatalities (n = 81), people affected (n = 84), and economic damage (n = 59). Then, in addition to aggregating PFA scores combining all PFAs (Eq. (2) and Fig. 1), we also considered separated scores for the five PFAs and aggregated the results over the 85 countries (Fig. 2). We divided the countries in the sample into two subsamples representing, first, countries that experienced higher hazard frequency/ intensity than the baseline period (normalized hazard measures >1), and second, countries that experienced lower frequency/intensity (normalized hazard measures 1 H 1 and H < 1, respectively. Given the exploratory nature of our study, we used a Mann–Kendall non-parametric statistical trend test to calculate the Theil–Sen estimator and p values between each normalized severity index and average PFA changes for the different baseline periods (Supplementary Table 2). All Theil–Sen estimators were within the range −1.19 to 0.34, with an average value of −0.08 and a standard deviation of 0.27, and only six of them were statistically significant (p ≤ 0.05). It is worth noting that the highest variability of the Theil–Sen estimator was found when analyzing the number of events, while the smallest variation was observed for the affected people. One limitation of this method is that there might be other (confounding) variables that are potentially obfuscating the result but are not accounted for in our analysis. This suggests that future studies should explore more complex relationships with econometric or causal inference methods. Case study method for validating country DRR progress scores. We conducted an in-depth examination of two cases to investigate whether the HFA scores and the score changes between the HFA evaluation cycles were consistent with how DRR work in the selected cases had been described in the scientific literature. The examination procedure consisted of five steps. First, we selected two cases with the highest PFA change scores in the dataset: Swaziland (average PFA change score = 1.55) and Chile (average PFA change score = 0.85). The procedure for estimating policy change is detailed in “Methods” (Eqs. (1) and (2)), and final PFA change scores for all countries are reported in the Source data file. Second, we identified the PFA core indicators (Supplementary information, Table 1) that changed the most between the two periods in each country, respectively. As specified in Eq. (1), these changes were calculated based on the differences in average PFA progress scores in the first two HFA evaluation cycles and the last two cycles. However, Swaziland and Chile submitted reports in two of the four evaluation cycles, which means that the estimation of policy change was based on two evaluation cycles in both cases: in Swaziland 2007–2009 [cycle 1] → 2011–2013 [cycle 3], in Chile 2009–2011 [cycle 2] → 2011–2013 [cycle 3]. The Swaziland case included four core indicators that changed from a reported progress score of 1 in the first period to 4 in the second period. In Chile, the two core indicators that changed the most—from 2 to 4 and from 2 to 5, respectively—were included. Third, we reviewed the substantive qualitative descriptions provided by each country in the HFA reports to justify the reported scores for each core indicator. By doing so, we were able to access more detailed information about concrete actions taken (or not taken) that were cited by the reports as justifications for the scores given. These descriptions were then compared with evidence reported in the scientific literature. Fourth, to find relevant literature, we searched for previous studies addressing the issues covered by each core indicator. Specifically, we searched Google Scholar (searches conducted between May 4 and June 5, 2020) using different search strings and reviewed the first 50 studies listed. For example, to identify case studies of issues associated with core indicator 1.4 (A national multi-sectoral platform for DRR is functioning) in Swaziland, we used the following search string: “Swaziland+disaster risk reduction+platform.” In some instances, we also replaced key terms with related concepts (e.g., “coordination” instead of “platform,” and “DRR” instead of “disaster risk reduction”). In this step, we excluded studies that made references to the HFA reports as a data source. Peer-reviewed journal articles, book chapters, Ph.D. dissertations, and published research reports were included. In the last step, we compared the qualitative descriptions from the HFA reports with accounts derived from the literature to establish whether these were consistent. As a way to minimize the risk of confirmation bias, we searched for evidence in the literature that both corroborated the descriptions and evidence that pointed toward an alternative interpretation. The results of these validation tests are detailed in the Supplementary information (section 3) along with references to the literature used. Reporting summary. Further information on research design is available in the Nature Research Reporting Summary linked to this article. Data availability Natural hazard events data, including the number of events, fatalities, economic loss, and people affected, are accessible through the International Disaster Database (EM-DAT), administrated by the Centre for Research on the Epidemiology of Disasters (CRED), https://www.emdat.be. EM-DAT data are freely available but accessible by a data request. Data on national-level DRR progress can be freely downloaded through the Hyogo Framework for Action (HFA) National Progress Query Tool, https://www. preventionweb.net/applications/hfa/qbnhfa/home. Data on country income levels underlying Figs. 1 and 3 were derived from the World Bank, https://datahelpdesk. worldbank.org/knowledgebase/articles/906519-world-bank-country-and-lending-groups. All analyses in this work were performed with the MATLAB software version R2019b. In particular, the statistical analyses were carried out by using the MATLAB function ktaub available at: https://se.mathworks.com/matlabcentral/fileexchange/11190-mann-kendalltau-b-with-sen-s-method-enhanced. Source data are provided with this paper. Code availability The custom code and mathematical algorithm generated for this study have been deposited in the following public repository: https://www.statsvet.uu.se/research/ trampoline/data-repository/. Received: 20 December 2019; Accepted: 27 November 2020; References 1. 2. 3. 4. 5. 6. 7. 8. 9. 10. 11. 12. 13. 14. 15. 16. 17. Pielke, R. Tracking progress on the economic costs of disasters under the indicators of the sustainable development goals. Environ. Hazards 18, 1–6 (2019). McCaughey, J. W., Daly, P., Mundir, I., Mahdi, S. & Patt, A. Socio-economic consequences of post-disaster reconstruction in hazard-exposed areas. Nat. Sustain. 1, 38–43 (2018). Raschky, P. A. Institutions and the losses from natural disasters. Nat. Hazards Earth Syst. Sci. 8, 627–634 (2008). Kahn, M. E. The death toll from natural disasters: the role of income, geography, and institutions. Rev. Econ. Stat. 87, 271–284 (2005). Kousky, C. Informing climate adaptation: a review of the economic costs of natural disasters. Energy Econ. 46, 576–592 (2014). Jongman, B. et al. Declining vulnerability to river floods and the global benefits of adaptation. Proc. Natl Acad. Sci. USA 112, E2271–E2280 (2015). United Nations Office for Disaster Risk Reduction (UNISDR). Implementation of the Hyogo Framework for Action - summary of reports 2007-2013. https://www.unisdr.org/we/inform/publications/32916 (2013). Baumgartner, F. R. & Jones, B. D. Agenda dynamics and policy subsystems. J. Polit. 53, 1044–1074 (1991). Adger, N., Arnell, N. W. & Tompkins, E. L. Successful adaptation to climate change across scales. Glob. Environ. Chang. 15, 77–86 (2005). Albala-Bertrand, J. M. Natural disaster situations and growth: a macroeconomic model for sudden disaster impacts. World Dev. 21, 1417–1434 (1993). Drury, A. C. & Olson, R. S. Disasters and political unrest: an empirical investigation. J. Contingencies Cris. Manag. 6, 153–161 (1998). Birkland, T. A. Lessons of Disaster: Policy Change after Catastrophic Events (Georgetown University Press, 2006). Michaels, S., Goucher, N. P. & McCarthy, D. Policy windows, policy change, and organizational learning: watersheds in the evolution of watershed management. Environ. Manag. 38, 983–992 (2006). Folke, C. et al. Resilience thinking: integrating resilience, adaptability and transformability. Ecol. Soc. 15, art20 (2010). Burch, S., Shaw, A., Dale, A. & Robinson, J. Triggering transformative change: a development path approach to climate change response in communities. Clim. Pol. 14, 467–487 (2014). O’Brien, K. Global environmental change II: from adaptation to deliberate transformation. Prog. Hum. Geogr. 36, 667–676 (2012). Gibson, T. D. et al. Pathways for transformation: disaster risk management to enhance resilience to extreme events. J. Extrem. Events 03, 1671002 (2016). NATURE COMMUNICATIONS | (2021)12:193 | https://doi.org/10.1038/s41467-020-20435-2 | www.nature.com/naturecommunications 9 ARTICLE NATURE COMMUNICATIONS | https://doi.org/10.1038/s41467-020-20435-2 18. Becker, S. L. & Reusser, D. E. Disasters as opportunities for social change: using the multi-level perspective to consider the barriers to disaster-related transitions. Int. J. Disaster Risk Reduct. 18, 75–88 (2016). 19. Thomalla, F., Downing, T., Spanger-Siegfried, E., Han, G. & Rockström, J. Reducing hazard vulnerability: towards a common approach between disaster risk reduction and climate adaptation. Disasters 30, 39–48 (2006). 20. Field, C. B. Managing the Risks of Extreme Events and Disasters to Advance Climate Change Adaptation: Special Report of the Intergovernmental Panel on Climate Change (Cambridge University Press, 2012). 21. Boin, A. & Hart, P. ’t Public leadership in times of crisis: mission impossible? Public Adm. Rev. 63, 544–553 (2003). 22. Birkmann, J. et al. Extreme events and disasters: a window of opportunity for change? Analysis of organizational, institutional and political changes, formal and informal responses after mega-disasters. Nat. Hazards 55, 637–655 (2010). 23. Pelling, M. & Dill, K. Disaster politics: tipping points for change in the adaptation of sociopolitical regimes. Prog. Hum. Geogr. 34, 21–37 (2010). 24. Passerini, E. Disasters as agents of social change in recovery and reconstruction. Nat. Hazards Rev. 1, 67–72 (2000). 25. Onuma, H., Shin, K. J. & Managi, S. Reduction of future disaster damages by learning from disaster experiences. Nat. Hazards 87, 1435–1452 (2017). 26. Hsiang, S. M. & Narita, D. Adaptation to cyclone risk: evidence from the global cross-section. Climate Change Econ. 3, 1250011 (2012). 27. Neumayer, E., Plümper, T. & Barthel, F. The political economy of natural disaster damage. Glob. Environ. Chang. 24, 8–19 (2014). 28. Keefer, P., Neumayer, E. & Plümper, T. Earthquake propensity and the politics of mortality prevention. World Dev. 39, 1530–1541 (2011). 29. Anbarci, N., Escaleras, M. & Register, C. A. Earthquake fatalities: the interaction of nature and political economy. J. Public Econ. 89, 1907–1933 (2005). 30. Nardulli, P. F., Peyton, B. & Bajjalieh, J. Climate change and civil unrest. J. Confl. Resolut. 59, 310–335 (2015). 31. Mach, K. J. et al. Climate as a risk factor for armed conflict. Nature 571, 193–197 (2019). 32. Gasper, J. T. & Reeves, A. Make it rain? Retrospection and the attentive electorate in the context of natural disasters. Am. J. Pol. Sci. 55, 340–355 (2011). 33. Fanta, V., Šálek, M. & Sklenicka, P. How long do floods throughout the millennium remain in the collective memory? Nat. Commun. 10, 1105 (2019). 34. Boudet, H., Giordono, L., Zanocco, C., Satein, H. & Whitley, H. Event attribution and partisanship shape local discussion of climate change after extreme weather. Nat. Clim. Chang. 10, 69–76 (2020). 35. Centre for research on the epidemiology of disasters (CRED). The International Disaster Database–EM-DAT. https://www.emdat.be/ (2019). 36. Peduzzi, P. et al. Global trends in tropical cyclone risk. Nat. Clim. Chang. 2, 289–294 (2012). 37. Brody, S. D., Zahran, S., Highfield, W. E., Bernhardt, S. P. & Vedlitz, A. Policy learning for flood mitigation: a longitudinal assessment of the community rating system in Florida. Risk Anal. 29, 912–929 (2009). 38. Dupuis, J. & Biesbroek, R. Comparing apples and oranges: the dependent variable problem in comparing and evaluating climate change adaptation policies. Glob. Environ. Chang. 23, 1476–1487 (2013). 39. Fankhauser, S. & McDermott, T. K. J. Understanding the adaptation deficit: why are poor countries more vulnerable to climate events than rich countries? Glob. Environ. Chang. 27, 9–18 (2014). 40. Yohe, G. & Tol, R. S. J. Indicators for social and economic coping capacity moving toward a working definition of adaptive capacity. Glob. Environ. Chang. 12, 25–40 (2002). 41. Schumacher, I. & Strobl, E. Economic development and losses due to natural disasters: the role of hazard exposure. Ecol. Econ. 72, 97–105 (2011). 42. Johnson, C. L., Tunstall, S. M. & Penning-Rowsell, E. C. Floods as catalysts for policy change: historical lessons from England and Wales. Int. J. Water Resour. Dev. 21, 561–575 (2005). 43. Koivisto, J. E. & Nohrstedt, D. A policymaking perspective on disaster risk reduction in Mozambique. Environ. Hazards 16, 210–227 (2017). 44. Delaney, P. & Shrader, E. Gender and post-disaster reconstruction: the case of hurricane Mitch in Honduras and Nicaragua. https://www. humanitarianlibrary.org/resource/gender-and-post-disaster-reconstructioncase-hurricane-mitch-honduras-and-nicaragua-0 (2000). 45. Schipper, E. L. F. Meeting at the crossroads?: exploring the linkages between climate change adaptation and disaster risk reduction. Clim. Dev. 1, 16–30 (2009). 46. Meseguer, C. Policy learning, policy diffusion, and the making of a new order. Ann. Am. Acad. Pol. Soc. Sci. 598, 67–82 (2005). 47. Skidmore, M. & Toya, H. Do natural disasters promote long-run growth? Econ. Inq. 40, 664–687 (2002). 48. Nohrstedt, D. & Weible, C. M. The logic of policy change after crisis: proximity and subsystem interaction. Risk Hazards Cris. Public Pol. 1, 1–32 (2010). 10 49. Boin, A., ’t Hart, P. & McConnell, A. Crisis exploitation: political and policy impacts of framing contests. J. Eur. Public Pol. 16, 81–106 (2009). 50. May, P. J. Reconsidering policy design: policies and publics. J. Public Pol. 11, 187 (1991). 51. Crow, D. A., Albright, E. A. & Koebele, E. The role of coalitions in disaster policymaking. Disasters https://doi.org/10.1111/disa.12396 (2019). 52. Boin, A., McConnell, A. & ’t Hart, P. Governing after Crisis: The Politics of Investigation, Accountability and Learning (Cambridge University Press, 2008). 53. Peters, B. G., Jordan, A. & Tosun, J. Over-reaction and under-reaction in climate policy: an institutional analysis. J. Environ. Pol. Plan 19, 612–624 (2017). 54. Birkland, T. A. & Warnement, M. K. in Disaster and Development (eds Kapucu, N. & Liou, K. T.) 39–60 (Springer, 2014). 55. Puddington, A. et al. Freedom in the World 2014: The Annual Survey of Political Rights and Civil Liberties (Rowman & Littlefield, 2015). 56. Jones, S., Oven, K. J., Manyena, B. & Aryal, K. Governance struggles and policy processes in disaster risk reduction: a case study from Nepal. Geoforum 57, 78–90 (2014). 57. Mirza, M. M. Q. Climate change and extreme weather events: can developing countries adapt? Clim. Pol. 3, 233–248 (2003). 58. Füssel, H.-M. Adaptation planning for climate change: concepts, assessment approaches, and key lessons. Sustain. Sci. 2, 265–275 (2007). 59. Burton, I., Huq, S., Lim, B., Pilifosova, O. & Schipper, E. L. From impacts assessment to adaptation priorities: the shaping of adaptation policy. Clim. Pol. 2, 145–159 (2002). 60. Birkland, T. in Oxford Research Encyclopedia of Natural Hazard Science 1–26 (Oxford University Press, 2016). 61. Messerli, P. et al. Expansion of sustainability science needed for the SDGs. Nat. Sustain. 2, 892–894 (2019). 62. United Nations Office for Disaster Risk Reduction (UNISDR). Progress and challenges in disaster risk reduction: a contribution towards the development of policy indicators for the post-2015 framework for disaster risk reduction. https://www.unisdr.org/we/inform/publications/40967 (2014). 63. Stone, D. Learning lessons and transferring policy across time, space and disciplines. Politics 19, 51–59 (1999). 64. Scolobig, A., Prior, T., Schröter, D., Jörin, J. & Patt, A. Towards people-centred approaches for effective disaster risk management: balancing rhetoric with reality. Int. J. Disaster Risk Reduct. 12, 202–212 (2015). 65. Tall, A., Patt, A. G. & Fritz, S. Reducing vulnerability to hydro-meteorological extremes in Africa. A qualitative assessment of national climate disaster management policies: accounting for heterogeneity. Weather Clim. Extrem. 1, 4–16 (2013). 66. Burton, I. et al. in Managing the Risks of Extreme Events and Disasters to Advance Climate Change Adaptation: A Special Report of Working Groups I and II of the Intergovernmental Panel on Climate Change (eds Field, C. B. & Barros, V.) 393–435 (Cambridge University Press, New York, 2012). 67. United Nations Office for Disaster Risk Reduction (UNISDR). Indicators of Progress: Guidance on Measuring the Reduction of Disaster Risks and the Implementation of the Hyogo Framework of Action (UNISDR, 2008). 68. Aitsi-Selmi, A., Blanchard, K. & Murray, V. Ensuring science is useful, usable and used in global disaster risk reduction and sustainable development: a view through the Sendai framework lens. Palgrave Commun. 2, 1–9 (2016). 69. Gray, C. L. & Mueller, V. Natural disasters and population mobility in Bangladesh. Proc. Natl Acad. Sci. USA 109, 6000–6005 (2012). 70. Global Network of Civil Society Organisations for Disaster Reduction. Views from the frontline. https://www.gndr.org/programmes/views-from-thefrontline/vfl-2013.html (2013). 71. Dworak, T. & Görlach, B. Flood risk management in europe - the development of a common eu policy. Int. J. River Basin Manag. 3, 97–103 (2005). 72. Thomalla, F. & Larsen, R. K. Resilience in the context of tsunami early warning systems and community disaster preparedness in the Indian Ocean Region. Environ. Hazards 9, 249–265 (2010). 73. Formetta, G. & Feyen, L. Empirical evidence of declining global vulnerability to climate-related hazards. Glob. Environ. Chang. 57, 101920 (2019). 74. Voss, M. & Wagner, K. Learning from (small) disasters. Nat. Hazards 55, 657–669 (2010). 75. Gall, M. et al. When do losses count? Bull. Am. Meteorol. Soc. 90, 799–810 (2009). 76. Tanoue, M., Hirabayashi, Y. & Ikeuchi, H. Global-scale river flood vulnerability in the last 50 years. Sci. Rep. 6, 36021 (2016). Acknowledgements We thank the International Disaster Database (EM-DAT) team for granting access to natural hazard events data, Beatriz Quesada Montano for assistance with initial data preparation, and Jacob Hileman for comments on the main text. This work was NATURE COMMUNICATIONS | (2021)12:193 | https://doi.org/10.1038/s41467-020-20435-2 | www.nature.com/naturecommunications NATURE COMMUNICATIONS | https://doi.org/10.1038/s41467-020-20435-2 conducted as part of a project entitled “The transformative potential of extreme weather events (TRAMPOLINE)” supported by the Swedish Research Council (grant No. 201803977 to all authors). All authors are grateful for the support from the Centre of Natural Hazards and Disaster Science (CNDS). Additional support was received from the Swedish Research Council FORMAS within the ERA-NET project STEEP-Streams (to M. M.) and the European Research Council (ERC), which provided funding for G.D.B.’s project entitled “HydroSocialExtremes: Uncovering the Mutual Shaping of Hydrological Extremes and Society,” H2020 Excellent Science, Consolidator Grant No. 771678. Author contributions All authors designed the study. M.M. developed the custom code and conducted data analyses with G.D.B. D.N. was the lead author, C.F.P., G.D.B., and M.M contributed to the writing of the text. All authors contributed to the analysis and interpretation of results, reviewed the manuscript, and gave approval for publication. Funding Open Access funding provided by Uppsala University. Competing interests The authors declare no competing interests. Additional information Supplementary information is available for this paper at https://doi.org/10.1038/s41467020-20435-2. ARTICLE Correspondence and requests for materials should be addressed to D.N. Peer review information Nature Communications thanks Jörn Birkmann and the other anonymous reviewer(s) for their contribution to the peer review of this work. Peer reviewer reports are available. Reprints and permission information is available at http://www.nature.com/reprints Publisher’s note Springer Nature remains neutral with regard to jurisdictional claims in published maps and institutional affiliations. Open Access This article is licensed under a Creative Commons Attribution 4.0 International License, which permits use, sharing, adaptation, distribution and reproduction in any medium or format, as long as you give appropriate credit to the original author(s) and the source, provide a link to the Creative Commons license, and indicate if changes were made. The images or other third party material in this article are included in the article’s Creative Commons license, unless indicated otherwise in a credit line to the material. If material is not included in the article’s Creative Commons license and your intended use is not permitted by statutory regulation or exceeds the permitted use, you will need to obtain permission directly from the copyright holder. To view a copy of this license, visit http://creativecommons.org/ licenses/by/4.0/. © The Author(s) 2021 NATURE COMMUNICATIONS | (2021)12:193 | https://doi.org/10.1038/s41467-020-20435-2 | www.nature.com/naturecommunications 11