Introduction to Experiments in Psychology PDF
Document Details
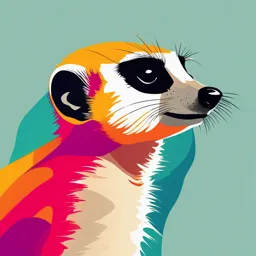
Uploaded by AdulatorySpinel1384
University of Cape Town
Tags
Summary
This document provides an introduction to experiments in psychology. It covers the history of research methods, the purpose of experiments, and the concept of causality. It examines the relationship between correlation and causation, highlighting the difference between the two. It also explores various research designs and discusses the importance of controlling variables in experiments.
Full Transcript
Introduction to experiments A brief history of research methods in Psychology As early as 1909, Robert Sessions Woodworth was giving his students copies of a mimeographed handout called “Problems and Methods in Psychology” When it was finally published in book form as Experimental Psychology in 193...
Introduction to experiments A brief history of research methods in Psychology As early as 1909, Robert Sessions Woodworth was giving his students copies of a mimeographed handout called “Problems and Methods in Psychology” When it was finally published in book form as Experimental Psychology in 1938, the publishers’ announcement merely said, ‘The Bible is out’. The purpose of the experiment: - is to demonstrate causality – to test casual relationships between variables. - Experiments are designed to manipulate one or independent variables and observe the effect on dependent variables, with the goal of establishing causality. For this to happen, there are 3 requirements: Cause must precede effect in time - This means that the independent variable must be manipulated or occur before the dependent variable is measured. In an experiment, researchers ensure this by controlling the timing of the manipulation and then observing the effect afterward. Cause and effect must be empirically correlated with one another - There must be a statistical association between the cause and the effect. This means that changes in the independent variable should be systematically associated with changes in the dependent variable. In other words, as the cause varies, the effect must vary as well. The relationship between cause and effect cannot be explained in terms of a third variable - Researchers must ensure that the observed relationship between the cause and effect is not due to a third, confounding variable. Confounding variables can falsely create or obscure the appearance of a relationship between the cause and effect. In a well-designed experiment, random assignment and control of extraneous factors are used to eliminate the influence of third variables. Should the cause be both necessary and sufficient to explain the effect? Causality and Correlation Correlation Causality - Definition: Correlation describes a - Definition: Causality indicates that statistical relationship between one event is the result of the two variables. When one variable occurrence of the other event; changes, the other tends to there is a cause-and-effect change in a specific direction. relationship. - Example: There is a positive - Example: Smoking causes an correlation between ice cream increase in the risk of lung cancer. sales and temperatures. As Here, smoking is the cause, and temperatures rise, ice cream sales lung cancer is the effect. tend to increase. - Key Point: Causation implies - Key Point: Correlation does not correlation, but the reverse is not imply causation. Just because two necessarily true. variables are correlated does not mean one causes the other. Why Correlation Doesn’t Imply Causation Third Variable Problem: A third factor might be influencing both variables. A third variable, also known as a confound, might explain the relationship between the two correlated variables. This third factor could be influencing both variables simultaneously, creating a false appearance of causality between them. For example, higher temperatures can increase both ice cream sales and the number of people swimming, which might lead to more drownings. Directionality Problem: It can be unclear which variable is the cause and which is the effect. For instance, does stress cause poor sleep, or does poor sleep cause stress? Coincidence: Sometimes, variables may be correlated purely by chance, especially when large datasets or numerous variables are involved. This phenomenon is known as a spurious correlation—where the relationship exists only due to random coincidence. Research Designs Correlational Research: Observes variables without manipulating them to identify relationships. Experimental Research: Manipulates one variable to see if it causes changes in another, helping to establish causation. Aspect Correlational Research Experimental Research Purpose Identify relationships between Establish causality variables Control of No manipulation; observe Manipulates variables, controls for Variables natural occurrences confounds Causality Cannot establish causality Can establish causality Research Observational, surveys, archival Controlled, randomized design Design data Advantages Useful when manipulation is not Provides strong evidence for cause- possible, large datasets and-effect Limitations Cannot infer causation, May lack generalizability, ethical confounding variables limitations In summary, correlational research is great for identifying associations between variables in natural settings, but it falls short of determining cause-and-effect. Experimental research, on the other hand, is designed to establish causal relationships, but it often requires more control, and its results may not always generalize to real- world situations. Features of an experiment In an experiment, one of the key features is the manipulation and control of the independent variables. These are the variables that the researcher manipulates to see how they affect the dependent variable, which is the outcome or response being measured. Independent Variables An independent variable is the factor that the researcher actively changes or controls in an experiment to observe its effect on the dependent variable. The IV is considered the "cause" in the cause-and-effect relationship being tested. Creating experimental conditions or comparisons that are under the direct control of the researcher. To test the effect of an independent variable, there must be at least two levels or conditions of that variable. These levels allow the researcher to compare different conditions and assess whether changes in the IV lead to changes in the dependent variable. Independent variables can be manipulated (actively changed by the researcher) or subject variables (pre-existing traits of participants). These types of IVs allow researchers to investigate how different factors influence behaviour and outcomes, while the controlled experimental design helps ensure that any observed effects are due to the manipulation of the IV, not external factors. Must have a minimum of two levels May be: Manipulated - Manipulated Variables: These are variables that the or subject researcher actively manipulates to create different conditions. variables The levels of the IV are directly controlled by the researcher. - Subject Variables: These are characteristics or traits of participants that are not manipulated but are naturally occurring, such as age, gender, or personality traits. While subject variables cannot be manipulated, they can still be used as independent variables by grouping participants based on these characteristics. Situational - features of the environment that participants may encounter - Researchers manipulate situational variables to see how changes in the environment affect behaviour. Task - variations in the task given to participants - Different versions of a task are used to test how task features influence performance. Instructional - variations in the instructions given - Different instructions may lead to different behaviours or performance outcomes. Controlling extraneous variables Extraneous variables are factors other than the independent variable (IV) that may influence the dependent variable (DV). If these variables are not controlled, they can lead to confounding, making it difficult or impossible to determine whether changes in the DV are due to the IV or some other factor. Not of interest to the researcher - Extraneous variables are variables that are not part of the hypothesis being tested. They are not of interest to the researcher, yet they can still affect the outcome of the experiment. These variables could come from a variety of sources, such as characteristics of the participants, the environment, or how the experiment is conducted. Must be controlled, otherwise it leads to confounding - If extraneous variables are not controlled, they can lead to confounding. Confounding occurs when an extraneous variable changes systematically along with the independent variable, making it hard to determine whether the observed effect is truly due to the IV or the confounding variable. We need to control anything that’s not of interest to us (i.e., we need to eliminate any “third variable” explanations) How to control extraneous variables: Random Randomly assigning participants to different experimental Assignment conditions helps ensure that individual differences (such as motivation, intelligence, or prior knowledge) are equally distributed across conditions. This reduces the likelihood that extraneous variables will systematically vary with the independent variable. Holding Variables Researchers can choose to hold certain variables constant Constant across all experimental conditions to prevent them from influencing the outcome. Counterbalancing When order effects (e.g., fatigue or practice effects) could influence the dependent variable, counterbalancing can be used. This involves varying the order in which participants experience conditions to control for these extraneous influences. Matching Groups Researchers can match participants on certain characteristics that could become confounds (e.g., age, gender, socioeconomic status) and then assign them to experimental conditions. This way, the extraneous variable is balanced across groups. Using Control Control groups provide a baseline for comparison. The control Groups group doesn’t receive the experimental treatment, which helps to isolate the effect of the independent variable by comparing it to participants who didn’t receive the manipulation. Measuring dependent variables Behaviours that are measured in the study In an experiment, measuring dependent variables (DVs) is a key part of the research process. The dependent variable is the outcome or behaviour that researchers measure to determine the effect of the independent variable (IV). The accuracy and credibility of the experiment hinge on how well the dependent variable is defined, measured, and interpreted. Must be defined precisely The dependent variable must be defined with precision so that it is clear exactly what is being measured and how it will be measured. This is referred to as the operational definition of the dependent variable. The operational definition specifies the exact procedures or criteria used to measure the behaviour or outcome, which ensures that the variable can be observed and quantified consistently across participants and conditions. The credibility of an experiment depends on (amongst other things) the operational definition of the measured outcomes The credibility of the experiment depends on how accurately and consistently the dependent variable is measured. Researchers aim to ensure that their measurement of the DV is reliable, valid, sensitive, and free from bias. Reliability of The measurement of the dependent variable must be reliable, Measurement meaning it should produce consistent results under similar conditions. If the same experiment were conducted again, the measurement should yield similar outcomes. Validity of The measurement must also be valid, meaning that it accurately Measurement measures what it is supposed to measure. Sensitivity of The operational definition of the dependent variable should be the Measure sensitive enough to detect any changes caused by the independent variable. Avoiding The measurement process should be free from bias, meaning that it Measurement should not systematically favour one outcome over another. Bias Researchers need to ensure that the way they measure the dependent variable doesn’t unintentionally influence the results. Precise definitions and measurements of the DV help ensure that the results of the experiment are valid, can be replicated by other researchers, and lead to meaningful and accurate conclusions about the relationship between the independent and dependent variables. Validity Statistical conclusion validity Do the correct analysis, without violating any assumptions Correct analysis refers to choosing the appropriate statistical test based on the type of data, the design of the study, and the research question. Different tests have different requirements (assumptions), and violating these assumptions can lead to inaccurate results. Common statistical assumptions: - Normality: Many statistical tests assume that the data are normally distributed. - Homogeneity of variance: Some tests assume that different groups have equal variances. - Independence: Observations must be independent of each other in many tests. Report all analyses, even the ones you don’t like Transparency in reporting is crucial to ensure that the statistical conclusions are valid. This means that researchers should report all the analyses they performed, not just the ones that support their hypotheses. Selectively reporting only favourable results, often called "p-hacking", is unethical and can lead to misleading conclusions. Don’t go fishing (Type I error – the more the analyses, the higher the chance of a false positive) Type I error occurs when a researcher incorrectly rejects the null hypothesis, meaning they find an effect or relationship when there isn’t one (a false positive). The more analyses you conduct on the same dataset, the higher the risk of making a Type I error. This is sometimes referred to as "going fishing" or "data dredging"—running multiple tests in search of any significant result. - The problem: The more tests you conduct, the greater the chance you’ll find something significant purely by chance. Without correcting for multiple comparisons, you increase the likelihood of falsely claiming an effect (inflating Type I errors). Make sure your measures are reliable, so that if there is an effect you can find it (otherwise you run the risk of Type II error – a false negative) Type II error occurs when a researcher fails to reject the null hypothesis, meaning they miss an effect that is actually present (a false negative). One of the main causes of Type II errors is using unreliable measures. If the dependent variable is not measured reliably, even if there is a real effect, the study may not be able to detect it. How to Maintain Statistical Conclusion Validity: 1. Correctly Choose and Apply Statistical Tests: o Use the appropriate statistical tests based on your research design and data type (e.g., t-tests, ANOVA, regression, etc.). o Make sure the test assumptions are met (e.g., normal distribution, equal variances, independence). 2. Report All Analyses: o Be transparent and report all your analyses, not just the significant ones. This helps ensure the credibility of your findings. 3. Avoid Type I Errors (False Positives): o Don’t run unnecessary or excessive analyses without justification. o Adjust for multiple comparisons when conducting many tests (e.g., Bonferroni correction). 4. Avoid Type II Errors (False Negatives): o Ensure that your measures are reliable and that your study is adequately powered to detect effects. Poor measures and small sample sizes increase the risk of missing true effects. 5. Use Reliable Measures: o Make sure that your dependent variable and other measurements are reliable. If the measurements are not consistent and dependable, the conclusions drawn from them may be flawed. 6. Ensure Adequate Sample Size: o Small sample sizes reduce the power of a study, increasing the risk of Type II errors (missing a true effect). Adequate sample sizes enhance the likelihood of detecting real differences or relationships. Construct validity Construct validity refers to how well a test, measure, or experiment actually measures the concept or construct it claims to measure. In psychological research, constructs like intelligence, anxiety, or motivation are abstract, and researchers use specific measures (e.g., tests, surveys, or observational tools) to quantify them. Construct validity ensures that these measures accurately represent the theoretical construct they are intended to assess. Does a test truly measure what it purports to measure? Construct validity addresses whether the test or measure is truly capturing the underlying concept (construct) it is designed to assess. It’s not just about measuring something but about measuring the right thing. Are your operational definitions adequate? For construct validity to be high, the operational definition of the construct must be adequate and reflect the concept accurately. External validity External validity refers to the extent to which the results of a study can be generalized to other populations, settings, times, and contexts beyond the specific conditions of the experiment. High external validity means that the findings can be applied broadly. Generalizability: - Other populations - The extent to which the results can be generalized to the broader population. If the sample is not representative of the larger population, external validity may be compromised. - Other environments - The extent to which the study's conditions mimic real- world settings. If an experiment takes place in a highly controlled lab setting, the results may not reflect what happens in real-world environments. - Other times - The extent to which findings apply across different times. If a study is conducted during a specific time period, the results may not apply to other times or eras. Internal validity Internal validity refers to the degree to which a study can establish a cause-and-effect relationship between the independent and dependent variables, without interference from extraneous or confounding variables. A study with high internal validity ensures that the results can confidently be attributed to the manipulation of the independent variable, rather than other factors. The degree to which a study is methodologically sound and confound-free Factors Affecting Internal Validity: 1. Control of Confounding Variables: Researchers must control for other variables that might influence the dependent variable. This is done through random assignment, holding variables constant, or using control groups. o Example: If you're studying the effect of sleep on memory performance, internal validity ensures that differences in memory are due to sleep conditions, not other factors like caffeine consumption or stress levels. 2. Random Assignment: Randomly assigning participants to different experimental groups helps ensure that the groups are comparable at the start of the experiment, which strengthens internal validity. o Example: Randomly assigning participants to a "sleep-deprived" group or a "well-rested" group helps ensure that differences in memory performance are due to sleep conditions rather than pre-existing differences between participants. 3. Eliminating Confounds: Confounding variables are factors that may co-vary with the independent variable and affect the dependent variable, thus distorting the results. o Example: If the sleep-deprived group also tends to have higher anxiety levels, the anxiety (a confounding variable) could explain differences in memory performance rather than sleep alone. 4. Temporal Precedence: The cause (independent variable) must precede the effect (dependent variable) in time. This ensures the proper direction of causality. o Example: Ensuring that sleep deprivation occurs before memory performance is tested to establish that sleep is affecting memory, not the other way around. 5. No Alternative Explanations: High internal validity means that other possible explanations for the results have been ruled out. o Example: If sleep deprivation is manipulated carefully and other influences like diet, stress, or medication are controlled, the researcher can conclude that sleep causes changes in memory performance. Comparing Internal and External Validity Aspect Internal Validity External Validity Focus Establishing a cause-and- Generalizing findings to effect relationship within broader contexts outside the study the study Main Concern Whether changes in the Whether the results apply dependent variable are to other populations, due to the independent settings, or times variable (control of confounding variables) Threats Confounding variables, Non-representative selection bias, maturation, samples, artificial settings, history, demand limited ecological or characteristics population validity Question Asked "Is the study "Can the study findings be methodologically sound?" generalized?" Importance in Critical for ensuring that Important for ensuring that Experiments the observed effects are the findings apply to the real and not due to outside real world, beyond the influences specific conditions of the study Pre-Post Studies How to go about experiments or studies Pretest → Treatment → Post-test Aspect Details Structure of a 1. Pretest: Measure the dependent variable before treatment. Pre-Post Study 2. Treatment: Apply the independent variable (the intervention). 3. Post-test: Measure the dependent variable after treatment. Steps to 1. Identify Research Question: Define the independent and Conduct a Pre- dependent variables. Post Study 2. Recruit Participants: Gather a suitable sample. 3. Pretest: Administer baseline measurements. 4. Administer Treatment: Implement the intervention. 5. Post-test: Measure outcomes again. 6. Analyze Data: Compare pretest and post-test results. Controlling for - Control Groups: Use randomized or no-treatment control groups Confounds to compare results. - Repeated Testing: Monitor for maturation, history effects, and testing effects. - Regression to the Mean: Account for natural fluctuations in performance. Advantages - Provides a baseline for comparison. - Each participant acts as their own control, reducing individual differences. - Simple and widely applicable design. Disadvantages - Lack of control for confounding variables without a control group. - Potential testing effects from repeated measurements. - Maturation and history effects can confound results. Threats to internal validity History - refers to external events that happen during the course of a study and could influence participants' responses or outcomes. - It threatens internal validity because the change in the dependent variable might be due to the external event, not the experimental manipulation. Maturation - the process where participants naturally change over time, regardless of the experimental treatment. This could include aging, gaining experience, or growing fatigued during the study. - It threatens internal validity by making it hard to determine whether changes in the dependent variable are due to the treatment or simply due to the passage of time. Testing - The effect of taking a test may influence subsequent test results. For instance, participants may improve on a post- test simply because they became familiar with the test format, not because of any treatment effect. - It undermines internal validity by conflating practice effects with actual changes caused by the treatment. Instrumentation - refers to changes in measurement tools or procedures over the course of a study. For example, if a test administrator becomes more lenient over time, the scores could be inflated, having a reliable or valid instrument - This poses a threat to internal validity because changes in the measurement, rather than the experimental treatment, could explain the results. Selection bias - If participants are not randomly assigned to groups, differences between the groups at the outset can affect the outcomes. For example, if one group has higher motivation levels from the start, this could confound the results. Random assignment and large sample. Bigger sample means better randomisation. - This threatens internal validity as differences between groups may be due to pre-existing differences rather than the treatment itself. Attrition - refers to participants dropping out of the study over time. If (morality) the participants who leave differ systematically from those who remain, it could bias the results. - It threatens both internal and external validity. Internally, it can skew the results if those who drop out differ in key ways from those who remain. Externally, it reduces the generalizability of the findings. Regression to - Extreme scores tend to move toward the average upon the mean retesting. For example, if participants are selected based on extremely high or low scores, their post-test scores may be less extreme simply due to statistical regression. - This threatens internal validity because changes in the dependent variable might be due to statistical regression rather than the experimental treatment. Control problems In experimental research Two basic designs: - Between-subjects: each person takes part in only one condition of the research o Problem: creating equivalent groups - Within-subjects (repeated measures): each person takes part in all conditions of the research o Problem: participating in one condition might affect behaviour in another condition (sequencing effects) Try to make sure that the only difference between the two groups in an experiment is the difference you as the researcher provide Aspect Between-Subjects Design Within-Subjects (Repeated Measures) Design Definition Each participant is assigned Each participant experiences all to only one condition of the conditions of the experiment. experiment. Goal To compare differences To compare differences within the between groups. same group. Equivalence Requires random Each participant serves as their own of Groups assignment to create control, reducing individual equivalent groups. differences. Advantages - Simplicity in design and - Reduces variability since the same analysis. participants are tested in all - Avoids carryover effects conditions. from previous conditions. - Requires fewer participants for the same statistical power. Challenges - Potential for group - Risk of carryover or sequencing differences due to random effects (e.g., practice effects, fatigue, assignment (selection bias). learning). - Requires larger sample - Requires counterbalancing to sizes to achieve statistical mitigate these effects. power. Example Testing a new medication Testing a new teaching method where where one group receives all students are taught using both the drug and another traditional and innovative methods in receives a placebo. different sessions. Ensure that the only Ensure that any observed differences difference between groups is are solely due to the treatment the treatment provided. condition and not influenced by prior conditions. Between-Subjects designs Used when the IV is a subject variable (e.g., extrovert/introvert; marital status) When experience gained in one level would make it impossible to participate in another level Two ways to try to have equivalent groups: - Random assignment - Matching Random assignment Goal: to take individual factors that could bias the study, and spread them evenly throughout the different groups of Ss Isn’t a guarantee Works best with large numbers Matching (not an experimental design!) Useful when you only have a small number of participants available, and/or can’t use random assignment Choose a matching variable that correlates with the DV (i.e., is expected to affect the outcome in some way) Make sure there is some reasonable way of measuring participants on the matching variable Within-Subjects Designs Advantages Disadvantages Need fewer people Practice effects No problems with equivalent groups Fatigue effects Reduces error variance, since you have Carryover effects (does it matter if no between-condition individual condition A comes before condition B?) difference – so it gives more statistical power to find an effect if there is one Single-factor designs Single-factor designs are experimental designs that focus on one independent variable, referred to as a "factor," and investigate its effects on a dependent variable. Single-factor designs allow researchers to systematically explore how variations in a single independent variable affect a dependent variable, making it a fundamental approach in experimental research. Vocabulary - “Factor” – This term denotes the independent variable being tested in the experiment. It is the variable that the researcher manipulates to observe its effect on the outcome. - “Level” – This refers to the different variations or states of the factor that are being tested. Each level represents a specific condition or treatment within the factor. - Example: o If you are studying test anxiety, then divide your group into “high test anxiety” and “low test anxiety”. “Test anxiety” is the factor, and “high” and “low” are the two levels (or conditions). - Another way of thinking of it is that levels are the variations within the factor Independent groups, 1-factor Example: Blakemore and Cooper (1970) raise two week old cats in two visual environments: one see only horizontal stripes, others see only vertical stripes. - Factor - visual environment - Levels - vertical stripes & horizontal stripes Analysis of data? - t-test for independent groups Matched groups, 1 factor Blagrove (1996): Will sleep-deprived people be influenced by misleading questions? - Factor – sleep deprivation - Levels - 21 hours or 43 hours Analysis? - T-test for dependent groups 1 factor, nonequivalent groups Knepper, Obrzut & Copeland (1983): Are gifted children good at social and emotional problem-solving, compared with average-IQ children? - Factor - IQ - Levels - gifted or average IQ Groups cannot be matched or randomly assigned here Gifted = 2 standard deviations on the right of the mean (on a normal distribution curve) Analysis - t-test for independent groups Aspect Independent Matched Groups Nonequivalent Groups 1-Factor 1-Factor Design Groups 1-Factor Design Design Definition Participants are Participants are Participants are randomly assigned paired based on a assigned to to different levels of characteristic, then different levels of the independent randomly assigned the independent variable, with each to different levels of variable without participant in only the independent random one condition. variable. assignment. Advantages - Reduces - Controls for - Practical and carryover effects. confounding easier to - Minimizes bias variables through implement in real- through random matching. world settings. assignment. - Increases group - Useful for equivalency. exploratory research. Disadvantages - Requires larger - More complex and - Higher risk of sample sizes for time-consuming to confounding due to equivalent groups. implement. pre-existing group - Individual - May require differences. differences may smaller sample - Limited causal confound results. sizes, reducing conclusions. power. 1-factor, within-Ss Lee & Aronson (1974): Will children shift their balance to moving visual stimuli as if their balance has shifted? - Factor – visual stimuli - Levels – Forwards and Backwards Analysis - t-test for dependent groups 1-Factor, Within-Subjects Design (also known as repeated measures design) is an experimental research approach where all participants are exposed to every level of the independent variable. This design focuses on a single independent variable (the "factor") that has multiple levels or conditions, and each participant experiences all of those conditions. Key Characteristics: 1. Single Factor: The design involves one independent variable. For example, if you're studying the effect of different types of music (classical, rock, and no music) on concentration levels, "type of music" is the single factor. 2. Within-Subjects: Every participant takes part in all conditions of the experiment. Continuing with the music example, each participant would complete a concentration task under classical music, rock music, and no music, allowing for direct comparisons of their performance across different conditions. Advantages: Control Over Individual Differences: Since each participant serves as their own control, this design helps eliminate variability caused by individual differences. This makes it easier to detect the effects of the independent variable because the same individuals are tested under all conditions. Requires Fewer Participants: Because each participant provides data for multiple conditions, researchers can achieve statistical power with a smaller sample size compared to designs where participants are only assigned to one condition. Disadvantages: Carryover Effects: One significant challenge is that the experience of one condition can influence how a participant responds to subsequent conditions. For example, if a participant listens to classical music first, it may affect how they perform when they switch to rock music. This is known as a sequencing effect. Order Effects: The order in which conditions are presented can also impact results. For example, if all participants first experience classical music, they might perform differently in the subsequent conditions simply because they are getting fatigued or accustomed to the task, not necessarily because of the music itself. Mitigating Issues: Researchers often use techniques such as counterbalancing, where the order of conditions is varied among participants. This helps to minimize the impact of order effects and ensure that the findings are more robust. Between-Ss, Multilevel designs Study looked at how much one can remember when there are no associations (e.g. if I say dog, you can picture a dog, however, if I mention something you have no association to, you will not picture anything). What if Ebbinghaus had measured at t=0 and t=7? Factorial designs Factorial designs are experimental research designs that investigate the effects of two or more independent variables (factors) simultaneously. Each factor can have multiple levels, allowing researchers to examine not only the main effects of each factor but also the interaction effects between them. A 2-factor study with 3 levels of one factor and 2 of the other is a 3x2 design; it has 6 conditions: FACTOR B (IV) Level 1 Level 2 Level 1 FACTOR A (IV) Level 2 Level 3 Explanation of a 2-Factor Design: In a 2-factor study: There are two independent variables being tested. Each factor can have a different number of levels. For example, if you have: Factor A with 3 levels (e.g., low, medium, high) Factor B with 2 levels (e.g., treatment and control) This results in a 3x2 design. The notation 3x2 indicates: 3 levels of the first factor (A) 2 levels of the second factor (B) Total Conditions: To determine the total number of experimental conditions (or groups), you multiply the number of levels of each factor: 3 (levels of Factor A) × 2 (levels of Factor B) = 6 conditions Example Here we are looking at test performance. Specifically the effect of test anxiety in males and females on test performance. So, 2 IVs here: test anxiety level, and gender. This means we have 2 factors Test anxiety has 3 levels (low, medium, high), and gender for this example has 2 levels (male, female) Please note the 6 separate conditions. A condition is a subgroup created when we have different factors, e.g. males with high anxiety would be one of the conditions here. Two kinds of results Main effects Main effects refer to the individual impact of each independent variable on the dependent variable. In a factorial design, each factor can have a main effect that indicates how changes in that factor influence the outcome. Example: Word-finding and sleep-deprivation and caffeine-deprivation. Can look at effect, and at the effect of sleep of caffeine - DV = word finding - 2 Factors (Sleep-deprivation; Caffeine-deprivation) - 2 Levels (Yes/No) - 4 Conditions Interactions Interactions occur when the effect of one independent variable on the dependent variable depends on the level of another independent variable. In other words, an interaction indicates that the impact of one factor changes depending on the level of the other factor. Interaction effects: when the effect of one IV variable depends on the level of another IV. Example: Word-finding depends on sleep-deprivation and caffeine-deprivation. Here we see no difference because all the averages are 9. However, when you look at the interactions, you find that participants remembered more words when they were both caffeine and sleep deprived (average = 10), and when they were deprived of neither sleep nor caffeine (also average of 10). Example: An interaction with no main effects Godden & Baddeley (1975): Is learning context-dependent? Four conditions: - Learn on land – recall on land - Learn on land – recall under water - Learn under water – recall on land - Learn under water – recall under water Results in In terms of inferential stats, there are no real differences numbers between the main effects averages, i.e. the overall averages. However, we see that recall on land was better when they learned on land and recalled on land (compared to learning on land and recalling underwater). Similarly, recall was better underwater when they learned underwater. So, learning is somewhat context dependent, because there is an interaction between where the material is learned and where it is recalled. Results in bar graph Results in line So here learning on land has no real advantage unless one has graph to recall on land as well (that is the interaction). If the main effects were significant, then learning on land would be an advantage, or recalling underwater would have an advantage (which is not quite the case). Mixed factorial with counterbalancing A mixed factorial design is a type of research study that combines two different types of designs: 1. Between-Subjects Design: In this part, different groups of people experience different levels of the independent variable. For example, one group might play a game with low difficulty, while another group plays the same game with high difficulty. 2. Within-Subjects Design: In this part, the same group of people experiences all levels of the independent variable. For example, those same players might try both the low and high difficulty of the game at different times. Riskind & Maddux (1993): Between-Ss variable: High self-efficacy vs. low self-efficacy Within-Ss variable: “Looming” DV: Fear Self-efficacy dependent on tasks Low self-efficacy = tied to a chair with newspaper being far High self-efficacy = not tied to the chair and so could reach the newspaper Results Values are average fear ratings (self- report) Number of participants needed Remember that the number of participants needed goes up as your number of conditions increases. 2X2, between-Ss 2X2, within-Ss 2X2, mixed design S1 S6 S1 S1 S1 S1 S2 S7 S2 S2 S2 S2 S3 S8 S3 S3 S3 S3 S4 S9 S4 S4 S4 S4 S5 S10 S5 S5 S5 S5 S11 S16 S1 S1 S6 S6 S12 S17 S2 S2 S7 S7 S13 S18 S3 S3 S8 S8 S14 S19 S4 S4 S9 S9 S15 S20 S5 S5 S10 S10 Number of participants can limit the complexity we can (safely) have in a study Correlational research Correlational research is a type of study in psychology that examines the relationship between two or more variables to see if they are associated or related in some way. It does not involve manipulation of variables or establishing causation; instead, it simply looks at how changes in one variable correspond to changes in another. Correlational research is a type of study in psychology that examines the relationship between two or more variables to see if they are associated or related in some way. It does not involve manipulation of variables or establishing causation; instead, it simply looks at how changes in one variable correspond to changes in another. Key Points about Correlational Research 1. Relationship Exploration: Correlational research seeks to identify whether there is a statistical relationship between variables. For instance, researchers might explore whether higher levels of stress are associated with lower academic performance. 2. Correlation Coefficient: The strength and direction of the relationship between variables are typically quantified using a correlation coefficient, which ranges from -1 to +1. A positive value indicates a direct relationship (as one variable increases, so does the other), while a negative value indicates an inverse relationship (as one variable increases, the other decreases). 3. No Causation: It’s important to remember that correlation does not imply causation. Just because two variables are correlated does not mean that one causes the other. For example, while there might be a correlation between ice cream sales and drowning incidents in summer, it doesn’t mean that ice cream sales cause drownings; both are influenced by the warm weather. Two disciplines of scientific psychology There was a rift between correlational and experimental psychology, but Cronbach helped to smooth it out a bit. Correlational Concerned with studying individual differences and psychology investigating the relationship between naturally occurring variables - Focus on individual differences - Investigating natural relationships - No manipulation Experimental Not usually interested in individual differences, but in psychology minimising these - Minimizing individual differences - Focus on Cause and Effect - Controlled Environment Correlation and regression Correlation is a statistical method used to determine if there is an association or relationship between two variables. It tells us how changes in one variable might relate to changes in another. Correlation identifies an association between two variables (co- relation). Positive Correlation This means that as one variable increases, the other variable also increases. For example, if you study more hours per week, your marks at the end of the year tend to go up. Negative Correlation This means that as one variable increases, the other variable decreases. For example, if you spend more time playing video games, your marks might go down. Correlation Coefficient: - The strength of the correlation is often measured using a correlation coefficient, which ranges from -1 to +1. - A coefficient closer to +1 indicates a strong positive correlation, while a coefficient closer to -1 indicates a strong negative correlation. A coefficient around 0 suggests no correlation. There is a positive association between number of hours studied per week, and marks at the end of the year Regression: used to make predictions when strong correlations exist. Regression is a statistical technique used to predict the value of one variable based on the value of another variable. It goes a step further than correlation by not only showing the relationship but also providing a formula to make predictions. The general form of a simple linear regression equation is: Y = a + bX Y is the dependent variable (the one you want to predict, like marks). X is the independent variable (the one you use for prediction, like hours studied). a is the intercept (the predicted value of Y when X is zero). b is the slope (how much Y changes for a one-unit change in X). Marks = error + b(hours studied) … Y = a + bX Correlation allows us to predict through regression While correlation identifies the relationship between variables, regression uses that relationship to make predictions. If you find a strong correlation, you can confidently use regression to estimate outcomes based on the independent variable. Correlational research and causality Two specific problems: o Which comes first? (Directionality) o Does watching violent TV lead to aggression in children? - Or do already aggressive children prefer to watch violent TV? Third variables So, you can talk about association, but you cannot use language that implies causation. One solution to the directionality problem Cross-lagging In quantitative research, directionality problems arise when it's unclear whether one variable causes changes in another or vice versa. This is a common issue in correlational studies where the relationship between two variables is established, but the direction of the relationship is uncertain. Cross-lagged analysis is a statistical technique used to address these directionality problems. It helps researchers determine the direction of causal influence between variables over time. Here's how it works: 1. Collect data over multiple time points: Instead of measuring the variables at just one point, the researcher measures them at multiple time points (e.g., time 1 and time 2). 2. Cross-lagged correlations: The technique looks at the correlation between one variable at an earlier time (say, time 1) and the other variable at a later time (say, time 2), and vice versa. This creates two "cross-lagged" paths: a. Variable A at time 1 → Variable B at time 2 b. Variable B at time 1 → Variable A at time 2 3. Compare the strength of the cross-lagged effects: By examining which cross- lagged path is stronger, researchers can infer which variable is more likely to influence the other over time. If the correlation from A at time 1 to B at time 2 is stronger than B at time 1 to A at time 2, then it's likely that A influences B. This method allows researchers to make more confident claims about the direction of causality between variables, helping to clarify potential causal relationships in correlational data. Caution: you can’t infer design from the stats Statistical analysis alone cannot tell you about the quality or structure of the research design. In other words, just because you have statistical results (e.g., significant correlations, p-values, or effect sizes), you can't automatically make conclusions about the research methodology, causality, or the overall validity of the study. Statistical Just because a relationship between variables is statistically significance significant doesn’t mean one causes the other. The design (e.g., doesn’t imply experimental, longitudinal, or correlational) is what allows causality researchers to make causal inferences, not the statistics themselves. Poor design If the research design is flawed (e.g., confounding variables are not can lead to controlled, samples are not representative, or the timing of data misleading collection is inappropriate), the statistical results may still appear stats strong but may not be trustworthy or meaningful. Design Different designs (e.g., experimental vs. observational) lead to dictates the different types of inferences. Experimental designs allow for stronger type of causal claims, while observational studies typically cannot. The conclusions stats from either design might be similar, but only an experiment would allow you to infer cause and effect, for example. So why do we do it? Practicality: - Some variables can’t be randomly assigned (gender, age, personality variables) Some research is conducted with prediction in mind - E.g., predicting why certain people will do well on the job Ethical grounds - Can’t randomly assign to brain damage Four places where correlational research is used - Psychological testing o Reliability: split-half, test-retest o Validity: criterion validity - Research in Personality and Abnormal Psychology - Studying the nature-nurture controversy - Any cross-sectional study Summary Correlational research: - Contributes a great deal to psychology, often when experimental procedures cannot be used - With modern, sophisticated statistical procedures, more complex questions about cause and effect can be addressed than in the past - Much correlational research takes place outside the laboratory – for instance, quasi-experimental research and programme evaluation