Quantitative Notes - Research Methods in Psychology II - University of Cape Town PDF
Document Details
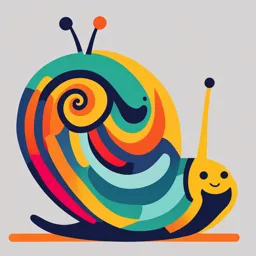
Uploaded by UnbeatableAlexandrite
University of Cape Town
Rain Coslett
Tags
Summary
These notes cover quantitative research methods in psychology, focusing on topics like causality, validity, experimental design, and specific types of research. The document is a collection of lecture notes. The notes come from the University of Cape Town.
Full Transcript
lOMoARcPSD|5507874 Quantitative Notes Research Methods in Psychology II (University of Cape Town) StuDocu is not sponsored or endorsed by any college or university Downloaded by Rain Coslett ([email protected]) ...
lOMoARcPSD|5507874 Quantitative Notes Research Methods in Psychology II (University of Cape Town) StuDocu is not sponsored or endorsed by any college or university Downloaded by Rain Coslett ([email protected]) lOMoARcPSD|5507874 Quantitative Research [email protected] Prescribed Readings: 1. Terre Blanche et al., Ch. 3, 7,8: 1. Causality 2. Validity 3. Control problems 2. Wilson & MacLean (on Vula), Ch 7: 1. Single-factor designs 2. Factorial designs 3. Terre Blanche et al., Ch. 8: 1. Correlational research 4. Wilson & MacLean, Ch 8 (on Vula): 1. Quasi-experimental designs 5. Terre Blanche et al., Ch 18 1. Programme evaluation 6. Leary Ch 14 (on Vula): 1. Small N designs 7. Terre Blanche et al., Ch 7: 1. Survey research 8. Terre Blanche et al., Ch 4: 1. Ethics in research Types of research: descriptive; relational; comparative (experimental and quasi-experimental) Causality A Brief History of Research Methods in Psychology As early as 1909, Robert Sessions Woodworth was giving his students copies of a mimeographed handout called “Problems and Methods in Psychology” Downloaded by Rain Coslett ([email protected]) lOMoARcPSD|5507874 When it was finally published in book form as Experimental Psychology in 1938, the publishers’ announcement merely said, ‘The Bible is out’. The Purpose of the Experiment: To demonstrate causality For this to happen, there are 3 requirements (NB): 1. The cause must precede the effect in time (i.e. temporal precedence) 2. Cause and effect must be empirically correlated with one another (i.e. covariance – as one changes, so does the other) 3. The relationship between cause and effect cannot be explained in terms of a third variable. No other explanation. Should the cause be both necessary and sufficient to explain the effect? Do you have to have the cause to get the effect, and is only the cause enough? (e.g. frustration causing aggression) The Features of an Experiment Establishing independent variables What the researcher controls Need to create experimental conditions or comparisons that are under the direct control of the researcher Controlling extraneous variables Not of interest to the researcher Must be controlled, otherwise it leads to confounding Measuring dependent variables Behaviors that are measured in the study Must be defined precisely Hold everything constant except for the independent variable, that which is of interest to the researcher. Independent Variables Must have a minimum of two levels (e.g. eats chocolate/doesn’t eat chocolate) Independent variables may be: Manipulated (controlled by the experimenter) or subject variables (inherent in the participants, can’t be manipulated e.g. learning disabilities) Downloaded by Rain Coslett ([email protected]) lOMoARcPSD|5507874 Situational variables – features of the environment that participants may encounter (e.g. noise or silence) Task variables– variations in the task given to participants (e.g. easy race maze / difficult rat maze) Instructional variables – variations in the instructions given (e.g. visualize something / recite something) Controlling Extraneous Variables We need to control anything that’s not of interest to us (i.e., we need to eliminate any “third variable” explanations) e.g. experimenter effect: when the researcher gives subtle clues about the way in which they expect the subjects of a study to respond Demand characteristics: certain features of the research setting that can impact the findings. Measuring the Dependent Variable The credibility of an experiment depends on (amongst other things) the operational definition of the measured outcomes - Operational definition = how the construct is defined in terms of the specific operations, measurement instruments, or procedures through which it can be observed Validity in Experimental Research 1. Statistical conclusion validity 2. Construct validity 3. External validity 4. Internal validity 1: Statistical Conclusion Validity The degree to which conclusions about the relationship among variables based on the data are correct Do the correct analysis, without violating any assumptions Determine your hypotheses and primary outcome variables (preferably) before the data has been collected Report all analyses, even the ones you don’t like Don’t go fishing (Type I error – the more the tests/analyses, the higher the chance of a false positive) Downloaded by Rain Coslett ([email protected]) lOMoARcPSD|5507874 Make sure your measures are reliable, so that if there is an effect you can find it (otherwise you run the risk of Type II error – a false negative) 2: Construct Validity Definition: the extent to which a measure of a construct is empirically related to other measures with which it is theoretically associated Does a test truly measure what it purports to measure? Are your operational definitions adequate? - Discriminant evidence: measures that aren’t theoretically related aren’t empirically related either - Convergent evidence is the opposite 3: External Validity The degree to which the findings / conclusions can be generalized beyond the confines of the design and study setting. Generalisability to: Other populations (e.g. males/females): the sampling can limit the study’s generalizability Other environments (many studies are performed in labs). Ecological validity: can the findings be generalized to real-life situations? Other times 4: Internal Validity The degree to which a study is methodologically sound and confound-free (designed well; no third variables) Degree to which we can be sure that the dependent variable changed as a result of the independent variable. The findings follow directly and unproblematically from the research design Concerns the research design and validity of the conclusions. Pre-Post Studies: the dependent variable is measured; there’s an intervention; the dependent variable is measured again. Test anxiety could also be decreasing through natural development (getting used to it). Only choosing 1st years means this is likely to be the case (selection bias). Can’t assume that all changes of the DV are due to the intervention: Downloaded by Rain Coslett ([email protected]) lOMoARcPSD|5507874 NB: Threats to internal validity: History: anything else that happens during the study that could affect the outcome of the study (e.g. the tests were changed, the participants watched something that changed them changes the DV) Maturation: natural development / independent change in the participants (getting better or worse at something) Testing: reactive effects to participating in the study. The initial test/measurement may change the DV or how the participants behave. The experimenter may suggest something that increases or decreases the DV. Includes priming, demand characteristics and experimenter effects. Instrumentation: the measurement / test is unreliable or problematic in some way Selection bias: non-random group assignment: groups are different in an important way. Experimental group and control group should be the same. Need to get a large enough sample, then randomize (most relevant in experimental groups) Attrition (mortality): non-random participant drop-out. They may share particular attributes, thus skewing he results. Regression to the mean: scores tend to average out. Data that is initially extremely higher or lower than the mean will likely be closer to the mean if it is measured a second time (or vice versa) Control problems in experimental research Experimental design: strongest internal validity (history, maturation, testing effect, selection bias are controlled for) - Instrumentation effect is also controlled for unless the measurement/test varies significantly between measures - Regression to the mean is controlled for if the two groups were drawn from the same sample - Attrition can still be an issue Two basic experimental designs: 1. Between-subject designs 2. Within-subjects (repeated measures): 1: Between-subject designs: AKA independent measures. Each person takes part in only one condition of the research Most commonly used type Downloaded by Rain Coslett ([email protected]) lOMoARcPSD|5507874 Used when the IV is a subject variable (e.g., gender; extrovert/introvert; marital status) - Also used when experience gained in one level would make it impossible to participate in another level Problem: making the groups equivalent Two ways to try to have equivalent groups: Random assignment and matching a. Random Assignment / randomisation Goal: to take individual factors that could bias the study, and spread them evenly throughout the different groups Randomness isn’t a guarantee Works best with large numbers b. Matching (not a true experimental design) Not an experimental design because the groups are no longer random: opens up room for bias. Not ideal, because there may be other factors effecting the DV that aren’t recognised Matching participants across groups who share similar factors that correlates with and is therefore expected to affect the DV Useful when you only have a small number of participants available (e.g. if the phenomena is rare), and/or can’t use random assignment. Make sure there is some reasonable way of measuring participants on the matching variable. e.g. comparing reading skills between people with and without brain lesions: match participants according to education etc (things that may affect the DV) 2: Within-Subject Designs AKA repeated measures. Each person takes part in all conditions of the research Problem: participating in one condition might affect behaviour in another condition (order/sequencing effects) Advantages: Need fewer people No problems with equivalent groups Reduces error variance, since you have no between-condition individual difference – so it gives more statistical power to find an effect if there is one Downloaded by Rain Coslett ([email protected]) lOMoARcPSD|5507874 Problems – sequence or order: Practice effects (includes learning, habituation, sensitization, adaptation, and fatigue) Carryover effects – previous condition effects behaviour in subsequent condition (does it matter if condition A comes before condition B?) Try to fix using counterbalancing. Single-Factor Designs Vocabulary: “Factor” – the independent variable “Level” – number of “states” of the IV you are testing Example: If you are studying test anxiety, then divide your group into “high test anxiety” and “low test anxiety”. “Test anxiety” is the factor, and “high” and “low” are the two levels (or conditions). Independent Groups, 1-Factor: Example: Blakemore and Cooper (1970) raise two-week-old cats in two visual environments: one see only horizontal stripes, others see only vertical stripes. Factor = type of visual environment Levels = horizontal stripes / vertical stripes Analysis of data = t-test for independent groups Matched Groups, 1-Factor: Blagrove (1996): Matched participants on their normal sleep duration. Will sleep-deprived people be influenced by misleading questions? Factor = amount of sleep Levels = adequate sleep / inadequate sleep Analysis = t-test for dependent groups Non-equivalent Groups, 1-Factor Non-equivalent groups: when matching AND random assignment is infeasible. Knepper, Obrzut & Copeland (1983): Are gifted children good at social and emotional problem- solving, compared with average-IQ children? Factor = IQ Levels = Average IQ / above-average IQ Downloaded by Rain Coslett ([email protected]) lOMoARcPSD|5507874 Analysis = t-test for independent groups Within-Subjects, 1-Factor Lee & Aronson (1974): Will children shift their balance to moving visual stimuli as if their balance has shifted? Factor = visual stimuli Levels = forwards / backwards Analysis = t-test for dependent groups Multilevel Designs: All the previous designs have included only 2 levels. Adding more levels adds more sensitivity and can show the true relationship between variables. Can be within or between subject designs Between-subjects, measuring only 2 levels: Measuring 4 levels shows a different relationship: Factorial Designs Factorial designs have more than one independent variable Multifactorial designs allow us to look at interaction effects Downloaded by Rain Coslett ([email protected]) lOMoARcPSD|5507874 A closer approximation of real-world settings, where independent variables don’t exist alone. All levels of each independent variables are combined with all levels of the other independent variables. Simplest design: 2x2 factorial design 4 conditions 2-Factor with 3 levels 2x3 design: a 2-factor study with 3 levels of one factor and 2 of the other. 6 separate conditions. Two kinds of results: Main effects and interaction effects Main effects are exclusively due to only one independent variable or another (the effect of one of your independent variables on the dependent variable) In general, there is one main effect for every independent variable in a study Example: Word-finding (DV) and sleep-deprivation and caffeine-deprivation (factors / IVs). 4 conditions. Can look at effect of caffeine OR the effect of sleep Interaction effects: when the effect of one IV variable depends on the level of another IV. A statistical interaction occurs when the effect of one independent variable on the dependent variable changes depending on the level of another independent variable. Example: Word-finding depends on sleep-deprivation and caffeine-deprivation. Example: an interaction with no main effects Godden & Baddeley (1975): Is learning context-dependent? Four conditions: Learn on land – recall on land Learn on land – recall under water Learn under water – recall on land Learn under water – recall under water No real difference between land and underwater (i.e. no main effects). But learning and recalling within the same environments (2 of the 4 conditions) was more effective. Downloaded by Rain Coslett ([email protected]) lOMoARcPSD|5507874 S1 S6 It’s possible to combine between-subjects and within-subjects manipulations in a S2 S7 single experiment. High self- Low self- S3 S8 efficacy efficacy S4 S9 Looming 2.6 4.5 Example: Mixed factorial with counterbalancing S5 S10 Counterbalancing is used to fix the order / sequencing effect Retreating 2.2S11 2.7 S16 Riskind & Maddux (1993): looking at how self-efficacy relates to fear S12 S17 S13 S18 Between-subjects variable: High self-efficacy vs. low self-efficacy S14 S19 Within-subjects variable: “Looming” (participants saw a video of a spider either S15 S20 coming towards them or moving away) DV: Fear But the ordering mattered: whether participants saw the spider moving towards them first or second - Fixed using counterbalancing: randomly assigned people to getting either order of the within-subjects variable The sample would split into two groups experimental (A) and control (B). For example, group 1 does ‘A’ then ‘B,’ group 2 does ‘B’ then ‘A’ this is to eliminate order effects. Although order effects occur for each participant, because they occur equally in both groups, they balance each other out in the results. Interaction effect between ‘looming’ and ‘low self-efficacy’ Number of participants needed: Remember that the number of participants needed goes up as your number of conditions increases: - 2x2 between subjects: 5 per group, 20 in total - 2 x 2 within subjects: 5 per group, 5 in total (same people in each group) - 2 x 2, mixed design (between and within subjects): 5 per group x 2 groups, so 10 in total Between subject designs require the highest numbers of subjects: Correlational Research Two broad disciplines of scientific psychology (according to Cronbach): Correlational: Concerned with studying individual differences and investigating the relationship between naturally-occurring variables Experimental: Not usually interested in individual differences, but in minimising these differences. Looks at groups and similarities within them. Variables are assigned by the experimenter. Correlation and regression Downloaded by Rain Coslett ([email protected]) lOMoARcPSD|5507874 Correlation identifies an association between two naturally-occurring variables (co-relation) - There is a positive association between number of hours studied per week, and marks at the end of the year Regression: used to make predictions when strong correlations exist - Y = a + bX - a = y intercept, b = slope - y = the dependent variable, x = independent variable - e.g. Marks = error + b(hours studied) Need to know conditions for causality (correlation doesn’t equal causality): - covariation: they vary together - temporal precedence: one comes before the other - elimination of possible 3rd variables Correlational research and causality Two specific problems: 1. Which comes first? (Directionality/temporal precedence) Does watching violent TV lead to aggression in children? Or do already aggressive children prefer to watch violent TV? 2. Third variables Violent parents may expose their children to more violent media Not in the test: One solution to the directionality problem: cross-lagging Eron, Huesman, Lefkowitz, & Walder (1972): Does watching violent TV cause aggression? Measured preference for watching violent TV programmes Measured peer ratings of aggression 875 third grade students Returned 10 years later, measured the same variables Cross-lagged results: Downloaded by Rain Coslett ([email protected]) lOMoARcPSD|5507874 Not in the test: Caution: design can’t be inferred from the statistics Comparing introverts and extraverts on their level of obedience to authority: 1. Administer tests of introversion/extraversion and obedience of authority; correlate the two 2. Administer tests of introversion/extraversion; select 25% most introverted and extraverted; put them in an obedience situation and measure obedience; use a t-test or 1-way ANOVA. So why do we use correlational research? Practicality: - Some variables can’t be randomly assigned (gender, age, personality variables) Some research is conducted with prediction in mind - E.g., predicting why certain people will do well on the job Ethical grounds - Can’t randomly assign to brain damage or to experiencing childhood abuse etc Places where correlational research is used Psychometrics (test development) - Testing reliability: split-half, test-retest. Must be highly correlated to indicate that they halves measure the same construct. - Testing validity: criterion validity. The ability of the measure to predict an outcome. Research in Personality and Abnormal Psychology (almost all is correlational: can’t assign people to having mental health problems or certain personalities) Studying the nature-nurture controversy (correlation between particular genes/environment and outcomes) Any cross-sectional study (e.g. correlation between exercise and stress levels) – can’t infer causality Downloaded by Rain Coslett ([email protected]) lOMoARcPSD|5507874 In summary: Correlational research: Contributes a great deal to psychology, often when experimental procedures cannot be used With modern, sophisticated statistical procedures, more complex questions about cause and effect can be addressed than in the past Much correlational research takes place outside the laboratory – for instance, quasi- experimental research and programme evaluation Quasi-Experimental Designs An experiment is… Where participants are randomly assigned To more than one condition. AKA a randomized controlled trial. Definition of quasi-experimental designs: Quasi-experimental designs are those in which participants cannot be randomly assigned to conditions Or A quasi-experiment exists whenever causal conclusions cannot be drawn because there is less than complete control over the variables in the study Don’t have random assignment and the conditions aren’t equivalent, but they resemble experiments in other respects (have an IV). Participants are assigned to groups according to a characteristic they already possess (age, gender, IQ) which is the IV. Need to try manually equating the groups or eliminating other differences as explanations for observed effects. Advantage of quasi-experimental designs: Increased external validity - Experiments are held under carefully controlled, artificial environments that may not generalise outside of these settings (i.e. in the real world) - Quasi-experiments are held under naturally occurring conditions Very useful for policy decisions / evaluation Disadvantages Downloaded by Rain Coslett ([email protected]) lOMoARcPSD|5507874 As the quasi-independent variable isn’t completely under control and there’s random assignment or control group, the research is much more vulnerable to threats of internal validity, specifically: - History - Maturation - Selection bias - Regression to the mean Two types of quasi-experimental designs to focus on: 1. Non-equivalent control group designs 2. Interrupted time series designs T = treatment / intervention 1. Non-equivalent control group designs - Purpose: evaluate effectiveness of a treatment programme by including a time series component along with a “control group” that’s not exposed to the intervention - Sample size = 2 - The 2 groups differ, they’re not randomly assigned. Validity therefore compromised. Therefore important to try match the groups as closely as possible prior to the study. Also vulnerable to regression to the mean and maturation. - Measure the two groups before and after the intervention 2. Interrupted time-series design - Purpose: to observe behaviour over time prior to and immediately after introducing an intervention - Advantage: allows for the evaluation of trends - Often used in policy or media evaluation - E.g.: measuring the effect of an anti-smoking campaign - Need a lot of data points to actually understand the effect of the intervention. Important to measure the DV before as well as after the treatment: Downloaded by Rain Coslett ([email protected]) lOMoARcPSD|5507874 Example: effect of an incentive plan on worker productivity - Tracked productivity for 4 years before the intervention - Tracked productivity for a further 6 years after - Systematically evaluated possible threats to internal validity to verify the effectiveness of the treatment Small N designs Very similar to time-series designs. Both use very small sample sizes. Used often in early days of psychology (e.g. studying oneself) Advantages of using small n designs: Sometimes used because potential subjects are hard to find (e.g. studying rare disorders) Grouped data can lead to misleading results (experiments average out results). Small n designs are more interested in individual differences - Averaged-out results from group designs may not accurately portray the response of any particular participant (e.g. USA women have an average of 2.09 children) - Group means may have no counterpart in the behavior of individual participants - Group means may obscure the fact the IV affected some participants but not others Often more than one individual is studied (usually 3-8), but their results are analyzed separately and rarely averaged. Single-participant researchers study intraparticipant variance: variability in an individual’s behavior within the same situation on different occasions. Small N designs in applied behavioural research Showing that the behaviour of a single individual changes as a result of the treatment, punishment or reinforcement. This requires: 1. The target behaviour must be operationally defined in terms of easily recordable events (usually frequency) 2. Establish a baseline level of responding against which the effects of the programme can be assessed (there’s a period of no intervention) 3. Introduce the treatment and continue monitoring Downloaded by Rain Coslett ([email protected]) lOMoARcPSD|5507874 Some types of small N designs 1. Withdrawal/reversal designs 2. Multiple baselines designs 1: Withdrawal/reversal designs The simplest is the A-B design (A = baseline / before the intervention, B = during the intervention) Can’t be too hasty in deciding that the intervention caused the change in behaviour: there could have been a 3rd variable. Therefore, the intervention should be withdrawn to see whether the behaviour changes again. Other threats to internal validity include maturation, testing, and history. Can also have more complex withdrawal/reversal designs: A-B-A or A-B-A-B – allows for more robust conclusions Withdrawal design case study: 2: Multiple baseline designs Used when a withdrawal design is not feasible (when the intervention/treatment changes something in the participants that can’t be reversed or when medical reasons forbid the withdrawal of a treatment) Multiple baselines approach uses a varying time schedule that allows the researcher to determine if the application of treatment is truly influencing the change in behaviour. We might vary the length of time in the initial baseline determination and then apply the treatment to determine if the change in behaviour corresponds with the introduction of treatment. Multiple baselines across subjects: Downloaded by Rain Coslett ([email protected]) lOMoARcPSD|5507874 The start of treatment conditions is staggered (started at different times) across individuals. Because treatment is started at different times we can conclude that changes are due to the treatment rather than to a chance factor. Evaluating small N designs What about external validity? Small N designs don’t tend to have very good external validity due to their small sample sizes. Generalizability is limited to the representivity of the sample. What about statistical significance? Can’t always be sure of it What about interactions? Difficult to explore because interventions are usually introduced one at a time. What about studies that don’t rely on frequency of response? Ethical issues regarding ABA designs: is it ethical to withdraw a potentially helpful treatment from a trouble patient to assure the researcher that it is in fact effective? (e.g. anti- depressants for suicidal patients The causal inferences one can draw from single participant designs are often weak, and the effects are of questionable generalizability, but such studies can provide indirect, anecdotal evidence that particular effects can be obtained. Downloaded by Rain Coslett ([email protected]) lOMoARcPSD|5507874 Surveys Two important aspects: - Sampling - Structuring the questions The sample is not drawn directly from the population, but from sampling frames (i.e. the form in which the sample becomes available to the researcher) Probability sampling and random selection Random selection: each person has an equal chance of being chosen to be in the sample Used whenever you want to learn something specific about an identifiable group of individuals (want to be able to generalize back to the population) We need a representative sample in order to generalise about the population, otherwise the sample is biased For a sampling method to be considered probability sampling, it must utilize some form of random selection. In other words, researchers must set up some process or procedure that ensures, with confidence, that the different units in their sample population have equal probabilities of being chosen. 1. Simple random sampling: Like “putting names in a hat” Each element has exactly the same chance of being selected, and the selection of each element was independent of the selection of a previous one. Creates samples that are highly representative of the population Effective and practical, except: - When you want to look at systematic features of the population (e.g. when you want to do research into gender differences but there are more women than men in a class) - When the population is very large 2. Stratified sampling: Used when populations consist of non-overlapping sub-groups/strata e.g. age, socio- economic status The researcher divides the entire population into different subgroups or strata (if it isn’t already divided), then randomly selects the final subjects from the different strata using some form of probability sampling. Downloaded by Rain Coslett ([email protected]) lOMoARcPSD|5507874 Proportionate stratified sampling: proportions of important subgroups in the population are represented precisely in the sample. The same proportion of individuals that can be found in the population are also in the sample. 3. Cluster sampling: Used when studying large populations and a sampling frame isn’t available The population is divided into more manageable groups of elements called clusters A “cluster” is a sampling unit (a class, a school etc) within which one samples randomly Non-probability sampling Any kind of sampling where selection of element isn’t determined by the statistical principle of randomness. Can be used when generalisability is not the goal, but rather studying the relationship between two variables is (e.g. stress and memory). Useful for testing theories about processes considered universal. 1. Convenience sampling: Requesting volunteers from a group of available people who meet the general requirements of the study (e.g. UCT’s SRPP program). Only need to be available and willing to participate. 2. Purposive sampling: Recruiting a specific type of person (can overlap with convenience sampling). E.g. women who are economic migrants. Sampling is directed towards elements of interest. Purposive sampling depends not only on availability and willingness to participate, but that the cases are typical of the population group being researched 3. Snowball sampling: When you start off with purposive sampling, then ask the participants to recruit anyone they know who matches the inclusion criteria of the study. Gradually accumulating a large enough sample through contacts and references Survey methods Interviews Advantages: Comprehensive, yields lots of information Downloaded by Rain Coslett ([email protected]) lOMoARcPSD|5507874 Usually don’t get problems with unclear information or missing data Disadvantages: People refuse to be interviewed, can’t be found, interviewer refuses to go there Cost, logistics Interviewer bias (e.g., cross-race bias) Written surveys Use either open or closed questions Often use Likert-like scales Can be sent through the post or online or administered in a group setting Phone surveys Use random digit dialing (to avoid missing unlisted numbers) Does exclude that portion of the population that doesn’t have a phone Non-response rate can be high NB: Evaluating survey research Was the sampling frame biased? Did it leave out certain groups? Was there a social desirability bias? Participants worry about how they look in the eyes of the researcher. E.g. parents unlikely to admit they do (or how often they do) spank their child. Problems with questions: - Ambiguity. E.g. “Visiting relatives can be fun.” - 2-part questions. E.g. “Is it wrong for female patrons in bars to swear and to buy drinks for men they don’t know?” - Framing of the question. E.g. “Do you prefer your hamburgers flame-broiled or fried?” VS “Do you prefer a hamburger that is grilled on a hot stainless steel grill or cooked by passing the raw meat through an open gas flame?” Observational Research Types of observational research: 1. Case studies 2. Naturalistic observation Downloaded by Rain Coslett ([email protected]) lOMoARcPSD|5507874 3. Participant observation 4. Archival research Case studies A detailed, descriptive study of a single individual, group or event (i.e. case) There’s a tension in psychology between the idiographic and nomothetic approaches Idiographic = individual. Nomothetic = the group. Case studies studying the individual are most common, but researchers can sometimes perform case studies of groups. The data can come from a variety of sources such as observation, interviews, news reports, and archival records Value of the case study: Source of ideas and hypotheses (e.g. Freud and his clients, Piaget and his own children theories) Source for developing therapy techniques Permit the study of rare phenomena (hard to find enough people for a group) Can provide a counterfactual for notions considered universally applicable (e.g. in criminal profiling) They have persuasive and motivational value (in the advertising industry) Limitations of the case study They’re virtually useless in providing evidence to test theories, as the case study occurred in an uncontrolled environment. No matter how plausible the explanation offered, alternative explanations cannot be ruled out (internal validity). The heavy reliance on anecdotal information makes for the possibility of quite biased presentation. There’s no relevant comparison information and control over other variables. Lack of generalisability to other individuals or situations. Observer bias: in behavioral science, the researcher is often the participant’s psychotherapist. There’s therefore no way of determining the reliability or validity of the researcher’s observations or interpretations. Additionally, the researcher often has a stake in the outcome of the investigation self-fulfilling prophecies. Case study example: stage hypnosis Crawford et al. (1992): 22 university students who had participated in a stage hypnosis show Downloaded by Rain Coslett ([email protected]) lOMoARcPSD|5507874 Enjoyable for 14, but: - 8 found could not remember what they had done on stage - 5 believed the hypnotist had total control over what they did - 1 likened the amnesia to an alcoholic blackout Naturalistic + participant observation The attempt to study normal behaviours of people or animals as they act in their everyday environments. The chief method of anthropologists and wildlife researchers The researcher… - Could be hidden (e.g., behind a one-way mirror; in a bird-hide) - Could just sit on a bench in a strategic location - Could just analyse a video - Participant observation: join the group being observed E.g. The theory of cognitive dissonance emerged out of participant observation. Researcher “joined” a cult and wrote a book about it. Issues with this study: - Joined under false pretences - Gave the other cult members the feeling that their ideas may be correct, because 7 other people (the researchers) had joined them Evaluating observational methods Absence of control: - Conclusions about causality cannot be drawn Can serve the purpose of falsification (show that what are considered universal laws aren’t actually) Observer bias: - Allowing preconceived ideas to colour one’s interpretations of one’s observations Subject reactivity - Subjects may react to the researcher being present, taking notes etc Example: observations of touching Hall & Veccia (1990): Men and women in public places: who initiates the touching? Are there gender differences in the type of touching? Are there age differences? Downloaded by Rain Coslett ([email protected]) lOMoARcPSD|5507874 Mixed-sex and same-sex touching in a variety of public places (airports, subways, malls) in Boston Handheld tape recorder and a timer that beeped every 10s Archival research Archival data range from public information such as census data, court records, genealogical data, etc. through more private information such as credit histories, medical records, educational records, and diaries. Evaluating archival research Advantages: Unlimited information available; the only limitation is the researcher’s creativity No subject reactivity (as you’re not working with actual participants) Can converge with lab research, thereby increasing external validity (testing whether lab- generated ideas correspond with archival data i.e. the real world) Disadvantages: Because it already exists, some piece may be missing or may not be representative Experimenter bias: selecting only those records that support one’s hypothesis Ethics in Psychological Research The start of formalising ethics The Tuskegee syphilis study - Black men with syphilis living in a poor area were studied. They were told they were being treated (when they weren’t) and the study was continued even after effective treatment was developed - Study started in the 1930’s but was only made public in 1968 Led to: The Belmont Report: Ethical Principles and Guidelines for the Protection of Human Subjects of Research (1979) NB: Three fundamental principles: 1. Beneficence: maximise benefits and minimise risks (benefits should outweigh the risks) Downloaded by Rain Coslett ([email protected]) lOMoARcPSD|5507874 2. Autonomy: people are treated as capable of making decisions about whether or not to participate in research (adults give informed consent, children give assent). Confidentiality is also important. 3. Justice: fairness (fairness about carrying the risks/burdens of the research; fairness about receiving the benefits). People should receive what is due to them. Participants should be treated with fairness at all stages of the research (includes fair selection and providing care/support distress/harmed participants). Need to be able to define and explain the above. Deception may only be used when the study is not harmful, there are no other alternatives, and the research is important. Participants must be debriefed afterwards. The IRB and Ethics codes Institutional Review Board – generic name Draws on: The American Psychological Association Ethics Code (see www.apa.org/ethics) The Helsinki Declaration of the World Medical Association NB: Informed consent Informed consent deals mostly with autonomy. Participants need to understand what they’re signing up for. Knowledge - Understanding the nature of the experiment (time required etc.), the alternatives available, and the potential risks and benefits Volition - Participants must provide their consent free from constraint or duress (can’t be forced), and may revoke their consent at any time (NB when working with children, prisoners etc.) - “Thank you” gifts or potential benefits shouldn’t be so big that they make participants feel that they can’t drop out. Participation needs to be entirely voluntary. Competence - The individual’s ability to make a well-reasoned decision and to give consent meaningfully - People in comas, people with intellectual disabilities Consent needs to be formalized in writing unless the risks of harm are very low. Consent forms Downloaded by Rain Coslett ([email protected]) lOMoARcPSD|5507874 - Overview - Description of procedures - Risks and inconveniences - Benefits - Costs and economic considerations - Confidentiality - Alternative treatments - Voluntary participation - Questions and further information - Signature lines Other ethical issues in research Data fraud Allocation of credit i.e. plagiarism. Stealing someone else’s work/ideas Sharing of materials and data Downloaded by Rain Coslett ([email protected])