Agricultural Statistics (PDF)
Document Details
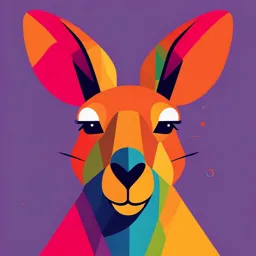
Uploaded by ObservantCarbon
Manila Review Institute, Inc.
2024
Tags
Summary
This document provides review materials for agricultural statistics. It covers definitions of key terms, data classification, presentation methods, frequency distributions, and graphical representations. The document was created by Manila Review Institute, Inc. in 2024.
Full Transcript
Philippine Copyright 2024 by MANILA REVIEW INSTITUTE, INC. 3/F Consuelo Building, 929 Nicanor Reyes St. (formerly Morayta), Manila Tel. No. 8-736-MRII (6744)...
Philippine Copyright 2024 by MANILA REVIEW INSTITUTE, INC. 3/F Consuelo Building, 929 Nicanor Reyes St. (formerly Morayta), Manila Tel. No. 8-736-MRII (6744) www.manilareviewinstitute.com All rights reserved. These handouts/review materials or portions thereof may not be reproduced in any form whatsoever without written permission from MRII. AGRICULTURAL AND BIOSYSTEMS ENGINEERING AGRICULTURAL STATISTICS STATISTICS – is a branch of mathematics that deals with the techniques in collecting, analyzing, interpreting and drawing conclusions from a given data POPULATION or UNIVERSE - this term refers to the totality of all actual or conceivable objects of a certain class under consideration, or the total number of experimental units under consideration. SAMPLE - this represents a finite number of objects obtained from the population. RANDOM SAMPLE - an object, number, or item drawn from the population objectively such that all objects in the population have equal chances of being selected. PARAMETER – is a property or characteristic of the population. It is seldom measured directly if the population is exceedingly large but often can be estimated from samples taken from the population. STATISTIC – is a property of a sample which is used to estimate the characteristics of the population. CLASSIFICATION OF DATA: Based on source a. primary data – gathered directly by the researcher through either of the following: experiment, personal interview, questionnaire, actual measurements, direct observations b. Secondary data – data obtained from other sources Based on form a.raw – unprocessed data b.derived data WAYS OF COLLECTING DATA: 1. By complete enumeration or census 2. By sampling ADVANTAGES OF SAMPLING OVER CENSUS: 1. It is more economical 3. It covers a greater scope 2. It saves time and effort 4. It is more reliable DATA PRESENTATION – is the process of arranging data in a compact form to facilitate computations and comparisons. METHODS OF DATA PRESENTATION 1. Through tables 2. Through graphs or charts Page 2 of 10 COMMONLY USED GRAPHS/CHARTS 1. Line graph – useful in comparing data in a time series (e.g. monthly sales, increase in enrolment). 2. Bar graph and column graph – an excellent toll for purposes of comparison (e.g. weekly growth of broilers with different dietary intake). 3. Pie chart – is a useful tool in showing how a whole is divided into parts (e.g. breakdown of expenses for food, clothing, rents, bills, recreation, transportation, etc.) 4. Pictorial chart – is the most effective if the data is qualitative rather than quantitative. GROUPING DATA – a manner of organizing a large set of data to make it easier to manage and analyze. FREQUENCY DISTRIBUTION - is a systematic and organized presentation of raw data into classes of appropriate size showing the corresponding frequency of observations in each class. CLASS INTERVAL (i) - is the difference between upper and lower boundaries of a class or of two successive midpoints. CLASS LIMIT (cl) - the pair of numbers written in the column of classes of a frequency distribution and used in tallying the original observations into their various classes. CLASS BOUNDARIES - are the true limits of the class. CLASS MARK (x) - or sometimes called the mid-x or midpoint is the middle value of the class. It is determined by adding the lower class limit to the upper class limit and dividing it by 2. FREQUENCY (f) - the number of items belonging into a particular class of a frequency distribution. CUMULATIVE FREQUENCY – for a certain class, the cumulative frequency refers to the sum of all frequencies either from the lowest or highest class up to the class considered. FREQUENCY DISTRIBUTION TABLE – is a tabular arrangement that shows the frequency of occurrence of the observed data in the different classes. GRAPHICAL REPRESENTATION OF A FREQUENCY DISTRIBUTION: 1. HISTOGRAM - a graph of the frequency distribution in which the frequency of any class interval is represented by a rectangle erected with the interval as a base and with a height proportional to the observed frequency. STEPS IN CONSTRUCTING A HISTOGRAM: 1. Draw a horizontal line to represent scores and a vertical line to represent frequencies. 2. Upper class boundaries are written along the horizontal base line and the frequencies scale along the vertical. 3. For each class interval the corresponding frequency is plotted and a horizontal line drawn the full length of the interval. 2. FREQUENCY POLYGON - is the polygon formed by connecting the tops of a series or ordinates whose lengths are proportional to the various frequencies and whose abscissas correspond to the variable value of the distribution. STEPS IN CONSTRUCTING A FREQUENCY POLYGON: 1. To construct a frequency polygon, plot the mid-values as an abscissa and the corresponding frequencies as ordinates. 2. Connect these plotted points by line segments. 3. Start the line segment from the origin and close it to the end of the scale. 3. CUMULATIVE FREQUENCY POLYGON - the cumulative frequency polygon is the graphical presentation of the cumulative frequency distribution. STEPS IN CONSTRUCTING A CUMULATIVE FREQUENCY POLYGON: 1. The cumulative frequency (F) (abscissa) are plotted against the upper class boundaries (ordinaries) and the points are joined by straight lines. 2. The polygon should always start from zero (origin) at the lower class boundary. 3. The general shape always slopping upward to the right and usually steepest near the center. 4. OGIVE - a continuous cumulative frequency curve approximated by the cumulative frequency polygon. Just as a histogram and frequency polygon can often be approximately fitted by a smooth curve, so the corresponding cumulative frequency polygon can be fitted approximately by smooth ogives. In short, an ogive is a smooth curve approximated from the cumulative frequency polygon. Page 3 of 10 5. FREQUENCY CURVES - the smooth curve obtained from the histogram as the size of the sample increases indefinitely. It may be regarded as the frequency curves of the parent population from which the sample is taken as in a random sample. TYPES OF FREQUENCY CURVES: 1. SYMETRICAL OR BELL-SHAPED - are characterized by the fact that observations equidistant from the central for maximum have the same frequency. 2. SKEWED TO THE RIGHT ( positive skewness) - the curve is said to be skewed to the right, if its longer tail is on the right- hand side of the mode. 3. SKEWED TO THE LEFT ( Negative skewness) 4. J-SHAPED OR REVERSE J-SHAPED - a frequency curve that a maxima occurs at one end. 5. U-SHAPED - a frequency curve that has a maxima at both ends. 6. BIMODAL - a frequency curve that has two maxima. (Two modes) 7. MULTIMODAL - a frequency curve that has more than two maxima. ( more than 2 modes). MEASURES OF CENTRAL TENDENCY : The so-called measures of central tendency are distinguished by the fact that they seek to determine some central value of the distribution. A number of such measures are available, each measure based on a different interpretation of what is meant by the most frequent characteristic value of distribution. No one of these measures is consistently better than the others. The best, or the most appropriate measure of a particular problem depends on the nature of the data and of the distribution. A working knowledge of the properties of the various possible measures is therefore essential for their proper use. ARITHMETIC MEAN - is the sum of all the observations divided by their number. MEDIAN, (Md) - is the central value of the distribution, and it divides the distribution into two equal parts. MODE, (Mo) - is the value that occurs most frequently in the distribution or the most common among the observations. It is employed when the most typical value of a distribution is desired. It is the most meaningful measure of central tendency in the case of strongly skewed or non-normal distribution, as it then provides the best indication of the point of heaviest concentration, though a distribution has only one mean and one median, it may have several modes, depending upon the number of peaks or concentration. Unimodal (one mode), Bimodal (two modes) and Multimodal (more than two modes). MEASURES OF DISPERSION: A measure of central tendency locates a point of concentration, but tell us nothing about the degree of concentration or about the matter in which the observations are dispersed throughout the distribution. Knowledge of the dispersion is important not only for its own sake but also because it enables us to evaluate the reliability of a measure of central tendency as a true measure of concentration. Of the many possible measures of dispersion, only five are in wide general use today - the standard deviation (Sx), Mean absolute deviation (MAD), range (R), quartile deviation (Q), and percentile range (10P90). THE STANDARD DEVIATION, SX Definition : The sum of squares of the differences between the observations and the mean value divided by the number of observations is known as the variance or mean square. The square root of the variance is the standard deviation, also known as the root mean square. 1. For ungrouped data: a) Sx = (x - x)2 n b) Sx = x2 n Sometimes, in order to have a better estimate of the standard deviation of a population from which the sample data is taken, the denominators in the above formulates (a), (b), & (c) are replaced by n-1 for samples less than 30. For large values of n (n 30) there is practically no difference between the two formulas. MEAN ABSOLUTE DEVIATION, M.A.D. - is the sum of the absolute value of the difference between the observations and the mean value divided by the number of observations. RANGE (R) - is the length of an interval which covers the highest and the lowest value of the observations. QUARTILE DEVIATION (Q) - is the measure to be used when the median is used as the average. Page 4 of 10 If a set of observations are arranged in order of magnitude and divided into four equal parts, the points of division are called quartiles. The first quartile, Q1 is the smallest in magnitude. The second quartile, Q2 is the same as the median. The third quartile, Q3 is the largest in the inter-quartile range, from Q1 to Q3 , this includes half of the observations. One half of this range denoted by Q is called the Quartile Deviation, or the Semi Quartile range. In symbols: Q = Q3 - Q1 2 1. For Ungrouped Data: If a set of X1, X2, X3,.... , Xn observations (arranged in an array ) are represented by a line segment and are to be divided by 4 equal parts, the points of division are called quartiles. In symbol, Q1 Q2 Q3 L ___________________________________H 50% The first quartile, Q1 is that value of x for which F = N/4 that is, 1/4 of all the observations n the distribution are smaller in value than Q1. Second quartile, Q2 , is equal to the median which divides the distribution into two equal parts. Formula: See Median Third Quartile, Q3, is that value of x for which F is equal to 3N/4, so that 75% of the values are below and 25% are above the O1 and Q3 or considering Q1 /- Q3. as an average, it is follows that the range (Q1 /- Q3/- Q) will include 50% of the middle values 2 (10-90) Percentile Range, 10P90 - is defined as the difference between the 10th and the 90th percentile in the distribution. In symbol, 10P90 = P90 - P10 where : 10P90 = percentile range P90 = 90th percentile P10 = 10th percentile COMPARISON OF DISTRIBUTION The Coefficient of Variation , V : The standard deviation is an absolute measure of dispersion, being an original unit, and does not permit comparisons, to be made of the dispersions of various distributions that are in different scales or units. The coefficient variation, being the ratio for the standard deviation to the mean is an abstract measure of dispersion that facilitates comparison of variations among different groups. The greater is the dispersion of the distribution, to the higher is the value of the standard deviation relative to that of the mean. Hence, the relative dispersion of a number of distribution may be determined simply by comparing the values of their coefficient of variation. Formula : Where : V = Sx/X V = coefficient of variation Sx = standard deviation of distribution X = mean of the distribution or average Example : Suppose the average annual sales of all filling station in city A are P/32,000 with a standard deviation of P/8,000 and that the annual sales of all filling stations in city B are P/16,000 with a standard deviation of P/4500. VA = 8,000 = 0.25 32,000 VB = 4,500 = 0.28 16,000 Since VA is less that VB, we may conclude that the sale of filling station in city B are more variable, i.e.. less consistent from stores in city A. The smaller the coefficient of variation, the less dispersed is the distribution. THE SHAPE COEFFICIENT: The shape of a distribution can be described by two coefficients. 1. SKEWNESS - to measure the degree of departure from symmetry. A symmetrical distribution are skewed either to the right positive or to the left (negative). A right-skewed distribution is usually characterized by the fact that its longer tail is on the right band side of the mode, i.e., most of the observation are the dispersed to the right of the mode. Similarly, a left-skewed distribution usually has its longer tail on the left hand-side of the mode. Page 5 of 10 2. KURTOSIS (K) - is a Greek word referring to the relative height (peakness) of a distribution. A distribution is said to be MESOKURTIC (K = 3) if it has the so-called "normal" kurtosis; PLATYKURTIC (K < 3) if its peak is abnormally flat, and LEPTOKURTIC (K > 3) if its peak is abnormally high. LINEAR CORRELATION & REGRESSION In the previous section, distribution of only one variable was discussed. Now, we are going to discuss a bivariate (two variables) distribution. There are instances when one variable increases as the other variable also increases. However, there are also instances when one variable decreases while the other one increases. When two variable behaves in a manner such that a change in one affects the value of the other, they are said to be correlated. One of the major objectives in a many statistical researches is to establish relationships or degree of association between two sets of variables. The purpose of determining the degree of association between two variables without considering the cause and effect of such a relationship is a problem in CORRELATION. While the purpose of estimating or predicting the average value of one variable in terms of the other variable is a problem in REGRESSION. TESTS OF STATISTICAL HYPOTHESES A. DEFINITIONS: 1. STATISTICAL HYPOTHESIS - is an assumption concerning a specific characteristics of some population 2. TEST OF STATISTICAL HYPOTHESIS - is a procedure for deciding whether to accept or reject the hypothesis. B. TWO TYPES OF ERRORS: In the test of statistical hypothesis, if the hypothesis is true but rejected base on the result of the analysis Type I Error is committed. On the other hand, if the hypothesis is false but accepted, Type II Error is committed. C. LEVEL OF SIGNIFICANCE: A test of a statistical hypothesis is made only in terms of probability statement. The probability that a researcher wants to risk of committing a type I Error at which the test is being conducted is called the Level of significance which is denoted by (alpha). Usually, the probabilities used are 0.05 and 0.01 to determine the critical region. The choice of the level of significance depends on the researcher and it is usually chosen before the data are available. STEPS IN THE TEST OF STATISTICAL HYPOTHESIS: 1. Statement of the Null hypothesis 2. State the level of significance 3. Determine the critical region a. Region of ACCEPTANCE b. Region of REJECTION 4. Compute the Statistic from the data obtained experimentally 5. ACCEPT or REJECT the hypothesis depending on whether the Statistics is outside or inside the CRITICAL REGION. HOW TO DERIVE RESULTS 1. If the experimental value is less than the tabular value at 5% level of significance the result indicates INSIGNIFICANCE. 2. If the experimental value is equal or greater than the tabular value at 5% level of significance but less than the tabular value at 1% level of significance the RESULT indicates SIGNIFICANCE. 3. If the experimental value is equal or greater than the tabular value at 1% level of significance the RESULT indicates Highly SIGNIFICANCE. HOW TO DRAW CONCLUSION 1. If the RESULT indicates insignificance, we conclude that there is no significant difference between the effects of the treatments. 2. If the RESULT indicates significance or highly significance, we conclude that there is a significant difference between the effects of the Treatments ELEMENTS OF FIELD EXPERIMENTS 1. Treatment 2. Replication 3. Error control COMPLETELY RANDOMIZED DESIGN The CRD is used when there is no factor that may cause bias in the result of the experiment Page 6 of 10 RANDOMIZED COMPLETE BLOCK DESIGN: This design is used when there is a factor that may possibly cause bias (such as fertility of the soil) in the conduct of the experiment. The area can be divided into blocks such that the plots within the blocks have the same fertility level. A - RANDOMIZATION Divide the area into blocks depending on the number of replications (no. of blocks) desired. Then, each block is subdivided into experimental plots corresponding to the number of treatments. Randomization in this design has one restriction; that all the treatments must appear once in each block, so that to assign the treatments each plot; randomization must be done separately for each block. For example, an experiment involving 5 treatments (A, B, C, D, and E) to be replicated five times. Divide the area into 5 blocks and each block is subdivided into 5 experimental plots. Randomization procedures similar to those discussed in-CRD can be used to assign treatments to plots in each block. LATIN SQUARE DESIGN: The Latin Square Design is usually used only for experiment involving four to eight treatments. The most important characteristics of this design is its capacity to stratify the experimental area along two directions instead of only one as with the RBD. Another important feature of this design, is that, no same treatment be on the row or on the same column. RANDOMIZATION This design has two restrictions on randomization to construct a Latin Square, select a Latin Square plan depending on the number of treatments, followed by the randomization among columns and among rows. For example, an experiment involving 5 treatments (A, B, C, and E ). Divide the area into plots of 5 rows and 5 columns. Then assign the treatments in plots at random: a. Select a 5 x 5 Latin Square Plan: A B C D E B C D E A C D E A B D E A B C E A B C D SPLIT-PLOT DESIGN: This design is used when the precise information on one factor and on the interaction of this factor with a second but to forgo such precision on the other factor is desired. And when the practical limit for plot size is much larger in one factor compared with the other. The most important feature of this design is that the sub-plot treatments are not randomized over the block but only over the main plots, and the main treatments are randomized in the large blocks. A- RANDOMIZATION: The randomization is a split-plot design is done by assigning the main treatments in the main plots and the s ub-treatments are assigned to the sub-plots at random. There are actually, two separate randomization processes, one for the main plots and another for the sub-plots. For example, a fractorial experiment with 3 main treatments and 4 sub-treatments, arranged in 5 blocks. a. Divide the experimental area into 5 blocks of equal size. Those blocks are subdivided into 3 main plots. b. Assign the main treatments at random in the main plots for all the blocks. c. Divide each of the main plots into 4 sub-plots. d. Assign the sub-treatment random in sub-plot for all the main plots and blocks. COMPARISON AMONG TREATMENT MEANS I. If the RESULT indicates INSIGNIFICANCE, no significant differences among the affects of the different treatments. II. If the RESULT indicates SIGNIFICANCE or HIGHLY SIGNIFICANCE, the most commonly used procedures in determining the significant difference between any pair of treatment means are: 1. LEAST SIGNIFICANT MEAN DIFFERENCE, LSMD: The LSMD is used only whenever the RESULT of the F-test is SIGNIFICANT (=0.05) or HIGHLY SIGNIFICANT (=0.05) or HIGHLY SIGNIFICANT (=0.01) and only for the comparison of not more than 5 treatments. If the difference between any two treatment means is equal or greater than the value of the LSMD, the difference is considered significant. Page 7 of 10 Formula: a. for equal number of replications: LSMD = t 2MSe n Where: t = tabular t- value at level or significance, using error df. MSe = mean square for error n = no. of replications b. for unequal no. of replications: LSMD = t AMSe ( nA /- Nb) NaNb 2. DUNCAN'S MULTIPLE RANGE TEST, LSMD in which only one value is computed for all the possible pairs of comparison, the DMRT, although additional computations are needed, this overcomes the major defects in the use of LSMD when testing differences among all possible pairs of treatment means regardless of the number of treatments being tested. Formula: DMRTk = rK MSe n Where : rk = tabular range value using the dfe. k = intervening means = 2,3,4,......., t t = no. of treatments MSe = mean square for error n = no. of replications HOW TO DRAW CONCLUSION USING DMRT: To determine the significant difference between any intervening means, compare the difference with the value of DMRT. The difference is considered significant if it is equal or greater than the DMRT, however, if the difference is less than the DMRT, the difference is considered insignificant. Factorial Experiments are used when experimenting on two or more factors or variables simultaneously instead of one at a time. There are essentially two different ways of analyzing factorial experiments - they depend on whether the two factors are INDEPENDENT or whether they INTERACT. INDEPENDENT - When the change in one factor does not influence the relative effects of the other factor (NO INTERACTION). Example: Suppose a tire manufacturer is experimenting with different kinds of treads, and that he finds that one king is especially good for use on dirt roads while another kind is especially good on cemented roads. In this case , we say that there is an INTERACTION between road conditions and the design of the treads. On the other band , if all the reads behaved equally well or equally poor under all kinds of road condition, we say that there is NO INTERACTION (that the two factors are independent). Problems: Sample data set 16.1 16.2 15.9 15.9 15.9 16.0 16.0 16.1 15.8 16.1 16.1 16.0 16.3 16.0 16.0 16.0 Page 8 of 10 FREQUENCY DISTRIBUTION TEST SCORES OF STUDENTS IN AE PRE-BOARD EXAMS SCORES Frequency, f 85-89 2 80-84 1 75-79 4 70-74 9 65-69 13 60-64 26 55-59 19 50-54 12 45-49 8 40-45 3 35-39 2 30-34 1 N = 100 PROBABILITY Probability - the measure or estimation of events that are likely to happen. It is a type of ratio where we compare how many times an outcome can occur compared to all possible outcomes Number of Possible Outcomes = Sample Space, S SAMPLE PROBLEM FOR SINGLE EVENT: Calculate the probability of getting an odd number if a dice is rolled? Solution: Sample space(S) if a dice is rolled = {1, 2, 3, 4, 5, 6} Let "E" be the event of getting an odd number, E = {1, 3, 5} So, P(E) = 3/6 = ½ PRACTICE EXERCISES: What is the probability to get a 6 when you roll a dice? A card is taken from a 52-card deck. What is the probability the card will be an ace? What the probability of getting a red card? What is the probability of getting a clover? A six-sided die was rolled. What is the probability that the number showing is a perfect square? INDEPENDENT EVENTS Two events are independent when the outcome of the first event does not influence the outcome of the second event. P(AuB) = P(A) x P(B) SAMPLE PROBLEMS FOR INDEPENDENT EVENTS: If two coins are tossed, calculate the probability of getting two tails? Solution: Sample space(S), when two coins are tossed = {(H, H), (H, T), (T, H), (T, T) } = 4 Let "E" be the event of getting two tails, E = {(T, T)} = 1 So, the Probability of getting 2 tails P(E) = 1/2 Page 9 of 10 If one has three dice what is the probability of getting three 4s? P(A u B u C) = P(A) x P(B) x P(C) P(A) = 1/6 P(B) = 1/6 P(C) = 1/6 P(A u B u C) = 1/6 x 1/6 x 1/6 = 1/216 DEPENDENT EVENTS Two events are dependent when the outcome of the first event influences the outcome of the second event. The probability of two dependent events is the product of the probability of X and the probability of Y AFTER X occurs. P (X∩Y) = P(X) x P(Y after X) SAMPLE PROBLEM FOR DEPENDENT EVENTS What is the probability for you to choose two red cards in a deck of cards? P (X and Y) = P(X) x P(Y after X) First Draw: P (red) = 26/52 = 1/2 Second Draw: P (another red) = 25/51 P (X and Y) = 1/2 x 25/51 = 25/102 MUTUALLY EXCLUSIVE EVENTS Two events are mutually exclusive when two events cannot happen at the same time. The probability that one of the mutually exclusive events occur is the sum of their individual probabilities. P(X or Y) = P(X) + P(Y) SAMPLE PROBLEM FOR MUTUALLY EXCLUSIVE EVENT: What is the possibility of getting either a red or pink field in the wheel of fortune above? P(red or pink) = P(red) + P(pink) P(red) = 2/8 = ¼ P(pink) = 1/8 P(red or pink) = ¼ + 1/8 = 3/8 INCLUSIVE EVENTS Inclusive events are events that can happen at the same time. To find the probability of an inclusive event we first add the probabilities of the individual events and then subtract the probability of the two events happening at the same time. P(X or Y) = P(X) + P(Y) – P(X and Y) SAMPLE PROBLEM FOR INCLUSIVE EVENTS: What is the probability of drawing a black card or a ten in a deck of cards? P(black or 10) = P(black) + P(10) – P (black and 10) There are 4 tens in a deck of cards P(10) = 4/52 There are 26 black cards P(black) = 26/52 There are 2 black tens P(black and 10) = 2/52 P(black or 10) = 4/52 + 26/52 – 2/52 = 28/52 Page 10 of 10 PERMUTATIONS AND COMBINATIONS Total number of possible outcomes Combinations – the order of the possible outcomes does not matter (e.g. lotto) Permutations (ordered combinations) – the order of the possible outcomes is important (e.g. padlock combination, sweepstakes) The number of permutations of n objects taken r objects at a time P(n,r) The number of combinations of n objects taken r objects at a time C(n,r) SAMPLE PROBLEM ON PERMUTATIONS A code have 4 digits in a specific order, the digits are between 0-9. How many different permutations are there if one digit may only be used once? P(n,r) n = 10 r=4 SAMPLE PROBLEM FOR COMBINATIONS How many combinations are there in a 6/42 lotto? C(n,r) n = 42 r=6 - END -