10) Fuzzy regression discontinuity designs .pdf
Document Details
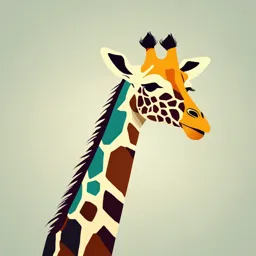
Uploaded by AppreciatedUranium
University of Bern
Tags
Full Transcript
Causal Analysis Fuzzy regression discontinuity design Blaise Melly Blaise Melly Fuyyz RDD 1 / 13 Fuzzy regression discontinuity Often the participation decision is not completely determined by X , even in...
Causal Analysis Fuzzy regression discontinuity design Blaise Melly Blaise Melly Fuyyz RDD 1 / 13 Fuzzy regression discontinuity Often the participation decision is not completely determined by X , even in a rule-based selection process. Case workers and/or individuals may have some discretion about program participation. Fuzzy RD exploits discontinuities in the probability of treatment conditional on a covariate. Di is no longer deterministically related to crossing a threshold but there is a jump in the probability of treatment at x0 ( g0 (Xi ) if Xi < x0 Pr (Di = 1|Xi ) = g1 (Xi ) if Xi ≥ x0 where g0 (Xi ) and g1 (Xi ) can be any smooth functions as long as they differ (and the more, the better) at x0. Sharp RD is a special case of fuzzy RD. Blaise Melly Fuyyz RDD 2 / 13 Nonparametric identification We still need that E [Y0 |X ] and E [Y1 |X ] are continuous in X. The discontinuity becomes an instrumental variable for treatment status locally at Xi = x0. Exclusion restriction: continuity of E [Yd |X ] First stage relevance: discontinuity in Pr (D = 1|X ) Local binary instrument → local ”Wald” estimator lim E [Y |X = x ] − lim E [Y |X = x ] x ↓x0 x ↑x0 E [Y1 − Y0 |X = x0 ] =. lim E [D |X = x ] − lim E [D |X = x ] x ↓x0 x ↑x0 (Here we assume homogeneous treatment effect at x0. See next slide.) Weak instrument = small jumps. Blaise Melly Fuyyz RDD 3 / 13 LATE As Hahn, Todd, and van der Klaauw (2001) point out, one needs the same assumptions as in the standard IV framework. If we want to allow for heterogeneous treatment effects (at the discontinuity), we need to exclude local defiers. As with other binary IVs one then estimates LATE: the average treatment effect of the compliers. In RD the compliers are those whose treatment status changes as we move the value of X from just the left of x0 to just to the right of x0. Doubly local population: compliers at x0. Blaise Melly Fuyyz RDD 4 / 13 Local linear regression As for the sharp design, we can use two estimation methods: local linear regression global polynomial regression. We can use 4 different local linear regression to estimate the 4 elements in lim E [Y |X = x ] − lim E [Y |X = x ] x ↓x0 x ↑x0 lim E [D |X = x ] − lim E [D |X = x ] x ↓x0 x ↑x0 The estimated treatment effects is m̂Y+ − m̂Y− + − m̂D − m̂D Blaise Melly Fuyyz RDD 5 / 13 Local 2SLS In the simplest case: uniform (rectangular) kernel + − + − same bandwidth for m̂Y , m̂Y , m̂D and m̂D The estimator is numerically equivalent to the 2SLS estimator using only observations close to x0 with Ti ≡ 1 (Xi ≥ x0 ) as an instrument for Di while controlling for Xi and Ti · Xi. Standard standard errors can be used. Blaise Melly Fuyyz RDD 6 / 13 Polynomial approximation Using a p-order polynomial, we can write the first-stage relationship p p Di = γ0 + πTi + ∑ γp Xij + ∑ γxp Ti Xij + ui j =1 j =1 Second stage p p Yi = µ + ρDi + ∑ βp Xij + ∑ βxp Ti Xij + vi j =1 j =1 2SLS estimator using polynomial in Xi interacted with Ti as control variables. Blaise Melly Fuyyz RDD 7 / 13 An application of fuzzy RD on class sizes Angrist and Lavy (1999) use a fuzzy RD design to analyze the effect of class size on test scores. They extend RD in two ways compared to the discussion above: The causal variable of interest (class size) takes on many values. → the first stage exploits discontinuities in average class size instead of probabilities of a single treatment. They use multiple discontinuities. Angrist and Lavy exploit an old Talmudic rule that classes should be split if they have more than 40 students in Israel. A school with 40 students has only one class. → class size 40. A school with 41 students has two classes. → class sizes 21 and 20. Predicted class size from a strict application of Maimonides rule is: es msc = e s −1 int 40 +1 where int [a] is the integer part of number a and es is enrollment. Blaise Melly Fuyyz RDD 8 / 13 Maimonides rule and actual class size Blaise Melly Fuyyz RDD 9 / 13 Econometric specification They want to estimate the relationship between average achievement and class size. Yisc = α0 + ρnsc + ηisc where nsc is the class size. Estimating this relationship with OLS may lead to biased results because class size is likely to be correlated with the error term. The 2 main reasons for this are: 1 Parents from higher socioeconomic backgrounds may put their children in schools with smaller classes. 2 Because principals may put weaker students in smaller classes. Blaise Melly Fuyyz RDD 10 / 13 Fuzzy RD design Angrist & Lavy therefore use the Maimonides rule in a fuzzy RD design. The variables relate to the previous description as follows: nsc plays the role of Di es plays the role of Xi msc plays the role of Ti In addition, they also control for the percentage of disadvantage students in class. Blaise Melly Fuyyz RDD 11 / 13 2SLS results Blaise Melly Fuyyz RDD 12 / 13 RDD in Stata It is relatively easy to estimate RDD in Stata with standard built-in commands. See example do-file. See also the impressive set of commands written by Matias Cattaneo and co-authors available here. Some other users have written additional commands: ssc install rd ssc install cmogram McCrary If you want to read more about the recent developments in the RDD literature: Melly and Lalive Blaise Melly Fuyyz RDD 13 / 13