Causal Analysis Instrumental Variables PDF
Document Details
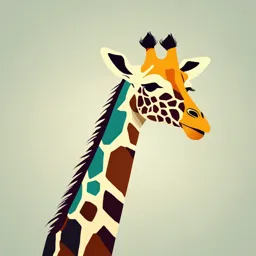
Uploaded by AppreciatedUranium
University of Bern
2024
Michael Gerfin
Tags
Summary
These are lecture notes on Causal Analysis, Instrumental Variables, and are part 1 of the lecture series. The notes cover topics such as instrumental variable (IV) mechanics, and the two-stage least squares (2SLS) method, from the University of Bern in Spring 2024. Notes cover examples such as the effect of military service on wages and fertility on labour supply.
Full Transcript
Causal Analysis Instrumental Variables: Part 1 Michael Gerfin University of Bern Spring 2024 Contents 1. Introduction 2. IV and 2SLS 3. Applications 4. IV Details 2 / 40 Introduction...
Causal Analysis Instrumental Variables: Part 1 Michael Gerfin University of Bern Spring 2024 Contents 1. Introduction 2. IV and 2SLS 3. Applications 4. IV Details 2 / 40 Introduction Introduction IV story in two iterations: first with constant effects, then in a framework with heterogeneous potential outcomes (causal effects are also heterogeneous then) Initial focus on constant effects is to explain the mechanics of IV (how it works and when it fails) But first: Why do IV? The answer is... sometimes the regression we’ve got is not the regression we want bias (selection, omitted variables,...) 3 / 40 Introduction IV and agnostic regression Coming back to previous example : causal link between college and wages written as Yi = α + τ Di + ηi Imagine a vector of controls (“ability”), part of which are observed (Xi ), part are unobserved (Ui ) The regression we want, perhaps causal, can be written Yi = α + τ Di + Xi′ γ + Ui If Di and Ui are correlated then controlling for X is not sufficient for identifying τ the regression we get is not the regression we want The error term Ui is the random part of potential outcomes left after controlling for Xi 4 / 40 Introduction CIA does not identify AT E Here, the causal effect is not identified by assuming CIA (conditioning on X). We cannot close the back-door path D ← U → Y (sometimes called “selection on unobservables”) Remember the identification logic in a DAG: manipulate the treatment (switch the dummy on or off) and observe what happens to Y. The causal effect is identified if nothing else changes on a non-causal path that would confound the change in Y. 5 / 40 IV and 2SLS 1. Introduction 2. IV and 2SLS 3. Applications 4. IV Details 6 / 40 IV and 2SLS IV and OVB Let’s ignore observable elements of ability for the moment Write potential outcome Y0i = α + Ui Assuming a constant effect τ we have Y1,i − Y0,i = τ A variable with the following properties allows to identify τ even if D is correlated with U 1 correlated with the causal variable of interest (Di ) 2 uncorrelated with the omitted variables we need to control for (independence): Cov(U, Z) = 0 3 not part of the causal model of interest (exclusion restriction) A variable with these properties is called an Instrumental Variable (IV) The exclusion restriction in words states that the only reason Y is correlated with Z is through D 7 / 40 IV and 2SLS IV in a DAG Assumption 1 is shown by the arrow from Z to D Assumption 2 is shown by the absence of an arrow between Z and U Assumption 3 is shown by the absence of an arrow between Z and Y 8 / 40 IV and 2SLS IV mechanics Rewrite the independence assumption as follows Cov(U, Z) = 0 Cov(Y − τ D, Z) = 0 Cov(Y, Z) − τ Cov(D, Z) = 0 Cov(Y, Z) τ= Cov(D, Z) Cov(Y, Z)/V ar(Z) = Cov(D, Z)/V ar(Z) “RF” (regression of Y on Z) = “1st”(regression of D on Z) The IV estimator is the sample analog of this expression 9 / 40 IV and 2SLS IV in a DAG There is causal chain from Z through D to Y What’s going on here? In the IV framework, not D is manipulated, but Z. When we change Z we record the changes in D (first stage, π1 ) and Y (reduced form, π1 × τ ). The ratio of these changes is the causal effect. There is no open back-door path Z → D ← U → Y because D is a collider on this path and not controlled for. 10 / 40 IV and 2SLS The Wald estimator Without covariates, the causal constant-effects model is Yi = α + τ Di + Ui where Ui and Di may be correlated. When Zi is a dummy variable that equals 1 with probability p, we can show that Cov(Yi , Zi ) = {E[Yi |Zi = 1] − E[Yi |Zi = 0]}p(1 − p) with an analogous formula for Cov(Di , Zi ). It follows that Cov(Yi , Zi ) E[Yi |Zi = 1] − E[Yi |Zi = 0] = Cov(Di , Zi ) E[Di |Zi = 1] − E[Di |Zi = 0] 11 / 40 IV and 2SLS Two-stage least squares (2SLS) In practice, we do IV by doing 2SLS, which allows us to add exogenous covariates and to use multiple instruments In our example, a causal model, without covariates, is Yi = α + τ Di + Ui Where does two-stage least squares come from? Write 1st as the sum of fitted values plus first-stage residuals: Di = π̂0 + π̂1 Zi + ξˆ1i = D̂i + ξˆi Substitute first-stage fitted values for Di , D̂i , in causal model: Yi = α + τ D̂i + [Ui + τ ξi ] and use OLS to estimate the “second stage” 12 / 40 IV and 2SLS Two Stage Least Squares (2SLS) 1. Stage Di = π0 + π1 Zi + ξi D̂i = π̂0 + π̂1 Zi π̂1 = Cov(D, Z)/V ar(Z) 2. Stage Y = α + τ D̂i + [Ui + τ ξi ] = α + τ D̂i + ei = α + τ (π̂0 + π̂1 Zi ) + ei = (α + τ π̂0 ) + τ (π̂1 Zi ) + ei Cov(Y, π̂1 Z) π̂1 · Cov(Y, Z) τ= = V ar(π̂1 Z) π̂12 · V ar(Z) 1 Cov(Y, Z) V ar(Z) Cov(Y, Z) = = π̂1 V ar(Z) Cov(D, Z) V ar(Z) Cov(Y, Z) = Cov(D, Z) 13 / 40 IV and 2SLS Two-stage least squares (2SLS) Intuitively, what does 2SLS do? It decomposes D into two parts: 1 the part that is uncorrelated with U , D̂, which is a function of Z and X, both assumed to be uncorrelated with U 2 the part that is correlated with U , ξˆ1i For identification in the second stage, only the uncorrelated part D̂ is used In other words, 2SLS removes the correlation between the potential outcomes and the treatment The precise connection between IV and potential outcomes will be discussed in the second part of the IV section 14 / 40 IV and 2SLS Two-stage least squares (2SLS) Where does a good instrument come from? Ideally, it is as good as randomly assigned Random assignment, e.g. lotteries, with partial compliance Random events like date of birth, combined with legislation referring to age Gender of children If the instrument is non-random we need a further assumption: conditional on X potential outcomes are independent of Z 15 / 40 IV and 2SLS 2SLS with random instrument and partial compliance Despite random assignment some agents choose not to get treated. This decision is a function of observables X and unobservables, shown by the dashed arrow. In this case we do not need to adjust for X because the back-door path D ← X → Y is closed anyway (D is collider on path Z → D ← X → Y ). The same argument holds for Z→D↔Y A reason to adjust for X is to reduce residual variance in Y , making estimates more precise 16 / 40 IV and 2SLS 2SLS with non-random instrument Here the instrument is a function of X. In this case we need to adjust for X because the back-door path Z ← X → Y is open. Adjusting for X allows to identify the casual effect. 17 / 40 Applications 1. Introduction 2. IV and 2SLS 3. Applications 4. IV Details 18 / 40 Applications Overview of applications Angrist (1990), “Lifetime Earnings and the Vietnam Era Draft Lottery: Evidence from Social Security Administrative Records”, AER (in part 1) Angrist and Krueger (1991), “Does Compulsory Schooling Attendance Affect Schooling and Earnings?”QJE (in part 2) Angrist and Evans (1998), “Children and Their Parents’ Labor Supply: Evidence from Exogenous Variation in Family Size”, AER (in part 2) Abadie, Angrist, and Imbens (2002) “Instrumental Variables Estimates of the Effect of Subsidized Training on the Quantiles of Trainee Earnings,” Econometrica (simplified example using the data in part 1 and 2) Abadie (2003), “Semiparametric Instrumental Variable Estimation of Treatment Response Models” Journal of Econometrics (simplied example using the data in part 1) 19 / 40 Applications Effect of military service on earnings (Angrist 1990) How were men who served in the Vietnam-era affected by their service? Let Di indicate veterans Key variables Zi = randomly assigned draft-eligibility in the 1970-72 draft lotteries Di = a dummy indicating Vietnam-era veterans The causal effect of Vietnam-era military service is the difference in average earnings by draft-eligibility status (the draft-eligibility reduced form) divided by the difference in the probability of service (the draft-eligibility first stage) 20 / 40 Applications Military service and earnings (Angrist 1990) Table 4.1.3 IV Estimates of the Effects of Military Service on the Earnings of White Men born in 1950 Earnings Veteran Status Wald Estimate of Earnings Mean Eligibility Mean Eligibility Veteran year Effect Effect Effect (1) (2) (3) (4) (5) 1981 16,461 -435.8.267.159 -2,741 (210.5) (.040) (1,324) 1971 3,338 -325.9 -2050 (46.6) (293) 1969 2,299 -2.0 (34.5) Note: Adapted from Table 5 in Angrist and Krueger (1999) and author tabulations. Standard errors are shown in parentheses. Earnings data are from Social Security administrative records. Figures are in nominal dollars. Veteran status data are from the Survey of Program Participation. There are about 13,500 individuals in the sample. MIT 14.387 SPRING 2010 21 / 40 Applications Fertility and labor supply (Angrist & Evans 1998) Effect of children on labor supply of mothers The decision to have children is endogenous and probably correlated with preferences regarding work Angrist & Evans (1998) analyze the effect of having a third child on mothers’ labor supply D=1 if mother has three children, D = 0 if she has 2 children As possible instruments z they Z = 1 if the second birth was a twin birth Z = 1 if the first two children have the same sex In both cases A&E argue that the instruments are as good as randomly assigned 22 / 40 Applications Fertility and labor supply (Angrist & Evans 1998) 23 / 40 Applications Randomized trial with partial compliance Analyze the effect of training provided under the Job Training Partnership Act (JTPA), a large publicly-funded training program Individuals in the randomly assigned JTPA treatment group were offered training, while those in the control group were excluded for a period of 18 months Only 60 percent of the treatment group actually received training, but the randomized treatment assignment provides an instrument for treatment status Use data analyzed by Abadie, Angrist and Imbens (2002) y is 30-months earnings, d is participation in training, z is randomly assigned treatment eligibility 24 / 40 Applications Descriptive Statistics I 25 / 40 Applications JTPA in a DAG 26 / 40 Applications Descriptive Statistics II 27 / 40 Applications Wald estimator z=0 z=1 d=0 d=1 d¯.01.62 ȳ 18’494 19’520 17’485 21’4550 (a) ȳ|z = 1 − ȳ|z = 0 = 19′ 520 − 18′ 404 = 1′ 116 (= π1 × τ ) ¯ = 1 − d|z (b) d|z ¯ = 0 =.62 −.01 =.61 (= π1 ) τ = 1′ 116/.61 = 1′ 830 (c) ȳ|x = 1 − ȳ|x = 0 = 21′ 485 − 17′ 485 = 3′ 970 (biased) 28 / 40 Applications JTPA analysis: OLS. reg y d , robust Linear regression Number of obs = 5,102 F(1, 5100) = 51.15 Prob > F = 0.0000 R-squared = 0.0100 Root MSE = 19444 Robust y Coef. Std. Err. t P>|t| [95% Conf. Interval] d 3970.212 555.1287 7.15 0.000 2881.921 5058.502 _cons 17485.29 351.3664 49.76 0.000 16796.46 18174.12. reg y d hsorged hispanic black married wkless13 age2225 age2629 age3035 age3644 age4554 /// > class_tr ojt_jsa f2sms, robust Linear regression Number of obs = 5,102 F(14, 5087) = 38.35 Prob > F = 0.0000 R-squared = 0.0909 Root MSE = 18657 Robust y Coef. Std. Err. t P>|t| [95% Conf. Interval] d 3753.648 536.3313 7.00 0.000 2702.208 4805.089 hsorged 4015.431 570.7427 7.04 0.000 2896.529 5134.332 hispanic 250.9286 883.456 0.28 0.776 -1481.025 1982.883 black -2354.207 625.851 -3.76 0.000 -3581.144 -1127.269 married 6545.679 628.5225 10.41 0.000 5313.505 7777.854 wkless13 -6581.672 565.9342 -11.63 0.000 -7691.146 -5472.197 age2225 5945.867 1419.189 4.19 0.000 3163.645 8728.088 age2629 7205.01 1456.585 4.95 0.000 4349.477 10060.54 age3035 5736.843 1432.993 4.00 0.000 2927.561 8546.126 age3644 4603.918 1442.486 3.19 0.001 1776.025 7431.81 age4554 2125.385 1544.613 1.38 0.169 -902.7205 5153.491 class_tr -1644.469 752.8068 -2.18 0.029 -3120.294 -168.6433 ojt_jsa 454.4841 621.3878 0.73 0.465 -763.7035 1672.672 f2sms 2101.602 550.6325 3.82 0.000 1022.125 3181.079 _cons 9810.577 1541.213 6.37 0.000 6789.137 12832.02 29 / 40 Applications JTPA analysis: 2SLS without covariates. ivregress 2sls y (d=z), first robust First-stage regressions Number of obs = 5,102 F( 1, 5100) = 4947.68 Prob > F = 0.0000 R-squared = 0.3418 Adj R-squared = 0.3417 Root MSE = 0.4003 Robust d Coef. Std. Err. t P>|t| [95% Conf. Interval] z.6116735.008696 70.34 0.000.5946256.6287213 _cons.0111568.0025457 4.38 0.000.0061661.0161475 Instrumental variables (2SLS) regression Number of obs = 5,102 Wald chi2(1) = 3.87 Prob > chi2 = 0.0492 R-squared = 0.0071 Root MSE = 19469 Robust y Coef. Std. Err. z P>|z| [95% Conf. Interval] d 1825.459 927.9389 1.97 0.049 6.732185 3644.186 _cons 18383.21 462.9362 39.71 0.000 17475.87 19290.55 Instrumented: d Instruments: z 30 / 40 Applications JTPA analysis: 2SLS with covariates. ivregress 2sls y (d=z) hsorged hispanic black married wkless13 age2225 age2629 age3035 age3644 age4554 /// > class_tr ojt_jsa f2sms, robust Instrumental variables (2SLS) regression Number of obs = 5,102 Wald chi2(14) = 482.79 Prob > chi2 = 0.0000 R-squared = 0.0880 Root MSE = 18659 Robust y Coef. Std. Err. z P>|z| [95% Conf. Interval] d 1592.937 893.2956 1.78 0.075 -157.8901 3343.764 hsorged 4075.108 572.4642 7.12 0.000 2953.099 5197.118 hispanic 335.1946 886.2038 0.38 0.705 -1401.733 2072.122 black -2349.052 624.4687 -3.76 0.000 -3572.988 -1125.116 married 6647.189 626.4701 10.61 0.000 5419.33 7875.048 wkless13 -6574.585 566.407 -11.61 0.000 -7684.722 -5464.448 age2225 5941.196 1417.888 4.19 0.000 3162.186 8720.206 age2629 7113.773 1454.796 4.89 0.000 4262.425 9965.121 age3035 5685.077 1431.701 3.97 0.000 2878.995 8491.159 age3644 4510.579 1441.645 3.13 0.002 1685.007 7336.151 age4554 2004.085 1543.366 1.30 0.194 -1020.858 5029.027 class_tr -1351.742 754.8366 -1.79 0.073 -2831.195 127.7104 ojt_jsa 425.173 621.6454 0.68 0.494 -793.2296 1643.576 f2sms 2101.86 551.0114 3.81 0.000 1021.897 3181.822 _cons 10641.25 1567.037 6.79 0.000 7569.913 13712.58 Instrumented: d Instruments: hsorged hispanic black married wkless13 age2225 age2629 age3035 age3644 age4554 class_tr ojt_jsa f2sms z 31 / 40 Applications Effect of 401(k) plans on savings 401(k) plans are pension savings plans in which employers match the workers contribution with given percentage Data used are called 401KSUBS. describe nettfa p401k e401k inc age agesq marr fsize storage display value variable name type format label variable label nettfa float %9.0g net total fin. assets, $1000 p401k byte %9.0g =1 if participate in 401(k) e401k byte %9.0g =1 if eligble for 401(k) inc float %9.0g annual income, $1000s age byte %9.0g age^2 agesq int %9.0g age^2 marr byte %9.0g =1 if married fsize byte %9.0g family size 32 / 40 Applications Descriptive Statistics. sum nettfa e401k inc age agesq marr fsize if p401k Variable Obs Mean Std. Dev. Min Max nettfa 2562 38.47296 79.27108 -283.356 1536.798 e401k 2562 1 0 1 1 inc 2562 49.81514 26.81424 10.14 192.99 age 2562 41.51327 9.651726 25 64 agesq 2562 1816.471 838.3487 625 4096 marr 2562.6955504.4602638 0 1 fsize 2562 2.920375 1.468098 1 13. sum nettfa e401k inc age agesq marr fsize if p401k==0 Variable Obs Mean Std. Dev. Min Max nettfa 6713 11.66722 55.28923 -502.302 1462.115 e401k 6713.160137.3667604 0 1 inc 6713 35.22425 21.64917 10.008 199.041 age 6713 40.91494 10.53225 25 64 agesq 6713 1784.944 916.4837 625 4096 marr 6713.6030091.4893105 0 1 fsize 6713 2.871592 1.547197 1 13 33 / 40 Applications OLS. reg nettfa p401k nettfa Coef. Std. Err. t P>|t| [95% Conf. Interval] p401k 26.80574 1.459166 18.37 0.000 23.94546 29.66603 _cons 11.66722.7668973 15.21 0.000 10.16393 13.17051. reg nettfa p401k inc age agesq marr fsize nettfa Coef. Std. Err. t P>|t| [95% Conf. Interval] p401k 13.52705 1.394046 9.70 0.000 10.79441 16.25968 inc.9769312.0282551 34.58 0.000.921545 1.032317 age -2.311125.5017084 -4.61 0.000 -3.294583 -1.327666 agesq.0386992.005774 6.70 0.000.0273809.0500175 marr -8.369471 1.639216 -5.11 0.000 -11.58269 -5.156248 fsize -.7856499.4970725 -1.58 0.114 -1.760021.1887215 _cons 10.04212 10.12265 0.99 0.321 -9.800509 29.88474 34 / 40 Applications 2SLS. ivregress 2sls nettfa (p401k=e401k) Instrumental variables (2SLS) regression Number of obs = 9275 nettfa Coef. Std. Err. z P>|z| [95% Conf. Interval] p401k 26.77116 1.896862 14.11 0.000 23.05338 30.48894 _cons 11.67677.8367317 13.96 0.000 10.03681 13.31674 Instrumented: p401k Instruments: e401k. ivregress 2sls nettfa (p401k=e401k) inc age agesq marr fsize Instrumental variables (2SLS) regression Number of obs = 9275 nettfa Coef. Std. Err. z P>|z| [95% Conf. Interval] p401k 9.418828 1.85786 5.07 0.000 5.777489 13.06017 inc.99719.0288992 34.51 0.000.9405485 1.053831 age -2.238551.5022227 -4.46 0.000 -3.222889 -1.254213 agesq.0378519.0057801 6.55 0.000.0265232.0491806 marr -8.355871 1.639369 -5.10 0.000 -11.56898 -5.142766 fsize -.8189625.4972173 -1.65 0.100 -1.793491.1555656 _cons 9.007582 10.12829 0.89 0.374 -10.84351 28.85867 Instrumented: p401k Instruments: inc age agesq marr fsize e401k. 35 / 40 Applications First stage. reg p401k e401k inc age agesq marr fsize Source SS df MS Number of obs = 9275 F( 6, 9268) = 2281.60 Model 1105.7232 6 184.287199 Prob > F = 0.0000 Residual 748.584728 9268.080770903 R-squared = 0.5963 Adj R-squared = 0.5960 Total 1854.30792 9274.19994694 Root MSE =.2842 p401k Coef. Std. Err. t P>|t| [95% Conf. Interval] e401k.6883064.0062974 109.30 0.000.6759621.7006507 inc.001334.0001389 9.60 0.000.0010616.0016063 age -.0048197.0024765 -1.95 0.052 -.0096741.0000348 agesq.0000532.0000285 1.87 0.062 -2.68e-06.0001091 marr -.0004663.0080732 -0.06 0.954 -.0162916.0153589 fsize.0000588.0024486 0.02 0.981 -.004741.0048586 _cons.0566798.0499041 1.14 0.256 -.0411432.1545027 36 / 40 Applications Instrument. reg e401k inc age agesq marr fsize Source SS df MS Number of obs = 9275 F( 5, 9269) = 158.48 Model 174.111763 5 34.8223526 Prob > F = 0.0000 Residual 2036.71368 9269.219733918 R-squared = 0.0788 Adj R-squared = 0.0783 Total 2210.82544 9274.238389632 Root MSE =.46876 e401k Coef. Std. Err. t P>|t| [95% Conf. Interval] inc.0052263.0002226 23.48 0.000.0047899.0056627 age.0326673.0040706 8.03 0.000.0246881.0406466 agesq -.0003769.0000468 -8.05 0.000 -.0004687 -.0002851 marr.005487.0133157 0.41 0.680 -.0206146.0315886 fsize -.0118661.0040368 -2.94 0.003 -.0197791 -.0039531 _cons -.4482027.0821791 -5.45 0.000 -.6092918 -.2871137 Obviously, instrument is not as good as randomly assigned. We may argue that it is as good as randomly assigned conditional on X (CIA assumption with respect to instrument, not treatment) 37 / 40 IV Details 1. Introduction 2. IV and 2SLS 3. Applications 4. IV Details 38 / 40 IV Details 2SLS Mistakes: Manual 2SLS Manual 2SLS estimate the first stage yourself and generate fitted values of endogenous variable plug the fitted values into the second stage equation and run OLS OLS standard errors from the manual second stage will not be correct the fact that the fitted values are estimated is not taken into account There are other risks as well 39 / 40 IV Details IV bias As stated (but not proven) IV is biased Intuitively, the bias is a consequence of the fact that the first stage is estimated Just-identified 2SLS (e.g. the Wald estimator) is approximately unbiased The just-identified sampling distribution has no moments, but just-identified 2SLS is approximately centered where it should be unless the instruments are really weak The reduced form is unbiased: if you can’t see the relationship you’re after in the reduced form, it ain’t there 40 / 40