Instrumental Variables - Part 2 PDF
Document Details
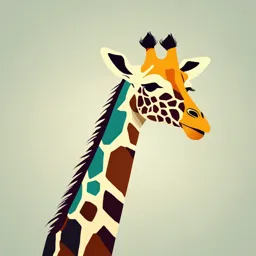
Uploaded by AppreciatedUranium
University of Bern
Blaise Melly
Tags
Related
- Applied Econometrics Endogeneity & Instrumental Variables Lecture 3 PDF
- Causal Analysis Instrumental Variables Part 1 PDF
- Capítulo II Y III: El Instrumento de Medición PDF
- Instrumental Variables & 2SLS PDF
- Causal Analysis Instrumental Variables PDF
- Research Methods: Applied Empirical Economics Lecture 3 PDF
Summary
This document discusses instrumental variables (IV) with heterogeneous potential outcomes. It explores different aspects of IV analysis and provides examples from economic studies.
Full Transcript
Causal Analysis Instrumental Variables - Part 2: IV with heterogeneous potential outcomes Blaise Melly University of Bern Blaise Melly (Universi...
Causal Analysis Instrumental Variables - Part 2: IV with heterogeneous potential outcomes Blaise Melly University of Bern Blaise Melly (University of Bern) IV with heterogeneous potential outcomes 1 / 25 Today’s class Instrumental variables: Why we were cheating: homogeneous treatment effect. What we get if we do not cheat: local average treatment effect (LATE). Is LATE an economically interesting quantity? Perhaps. To avoid misunderstandings: It is NOT about a new estimator. It is about the interpretation of the instrumental variable estimator under a different set of assumptions. Blaise Melly (University of Bern) IV with heterogeneous potential outcomes 2 / 25 Angrist and Evans (1998): fertility and labor supply Sibling sex mix of the first two children as an instrument for the decision to have a third child. Based on two assumptions: Parental preference for mixed sibling-sex composition Sex mix is virtually randomly assigned =⇒ the variable: having two kids with the same sex is a good instrument to measure the impact of the number of kids on labor market participation Blaise Melly (University of Bern) IV with heterogeneous potential outcomes 3 / 25 Angrist and Evans (1998): IV estimates Blaise Melly (University of Bern) IV with heterogeneous potential outcomes 4 / 25 Angrist and Evans (1998): first stage Blaise Melly (University of Bern) IV with heterogeneous potential outcomes 5 / 25 Angrist and Krueger (1991): education and wages The idea exploits the variation induced by compulsory schooling laws in the US: most states require students to enter school in the calendar year they turn 6. In addition, these laws require students to remain in school at least until their 16th birthday. For instance, a student born in January starts school at 6 (and 8 months), and at her 16th birthday she will have 9 years of completed schooling. A student born in December starts school at 5 (and 8 months), and when she turns 16 she will have 10 years of schooling. Then, depending on the date of birth students will have attended school during a different number of years when they reach the legal dropout age. Blaise Melly (University of Bern) IV with heterogeneous potential outcomes 6 / 25 Angrist and Krueger (1991): first stage Blaise Melly (University of Bern) IV with heterogeneous potential outcomes 7 / 25 Angrist and Krueger (1991): reduced form Blaise Melly (University of Bern) IV with heterogeneous potential outcomes 8 / 25 Angrist and Krueger (1991): IV estimates Blaise Melly (University of Bern) IV with heterogeneous potential outcomes 9 / 25 Homogeneity How can we identify effects for the whole population when only a small subpopulation reacts to the instrument? Because we assume that the treatment effect is homogeneous: Ys,i − Ys −1,i = ρ for all i and s. In other words, we impose linearity as well as homogeneity. These are clearly very restrictive assumptions. To focus on one thing at a time we consider a binary (zero-one) treatment variable and analyze IV in a heterogeneous-effects model. Issue of internal versus external validity: internal: instrument allows to identify effect on well-defined subpopulation within overall population (those whose decisions are affected by the instrument) external: predictive power for other populations Blaise Melly (University of Bern) IV with heterogeneous potential outcomes 10 / 25 Potential outcome set up We consider only the case with a binary treatment and binary instrument. Some generalizations exist. We observe Zi. Let Di (z ) be the potential values of the treatment when the instrument is set exogenously to z. We observe only one of them: Di = Di (1) · Zi + Di (0) · (1 − Zi ) Let Yi (d, z ) be the potential outcomes for unit i, when D is exogenously set to d and Z to z. There are 4 potential outcomes and we observe only one: Yi = Yi (1, 1) · Di (1) · Zi + Yi (1, 0) · Di (0) · (1 − Zi ) +Yi (0, 1) · (1 − Di (1)) · Zi + Yi (0, 0) · (1 − Di (1)) · (1 − Zi ) Blaise Melly (University of Bern) IV with heterogeneous potential outcomes 11 / 25 First assumption: independence Instrument is as good as randomly assigned, i.e. it is independent of the vector of potential outcomes and potential treatments: {Yi (0, 0) , Yi (0, 1) , Yi (1, 0) , Yi (1, 1) , Di (0) , Di (1)} ⊥⊥ Zi Implication 1: the first stage capture the causal effect of Z on D: E [Di |Zi = 1] − E [Di |Zi = 0] = E [Di (1) − Di (0)] Implication 2: the reduced form effect of Z on Y is the causal effect of Z on Y: E [Yi |Zi = 1] − E [Yi |Zi = 0] = E [Yi (Di (1) , 1) − Yi (Di (0) , 0)]. In the context of clinical trial, this is called the intention-to-treat effect. Blaise Melly (University of Bern) IV with heterogeneous potential outcomes 12 / 25 Second assumption: exclusion restriction Yi (d, z ) is only a function of d. Formally, Yi (d, 0) = Yi (d, 1) = Yi (d ) for d = 0, 1 Under the exclusion restriction we can define potential outcomes indexed only against treatment status using the same notation as before. Blaise Melly (University of Bern) IV with heterogeneous potential outcomes 13 / 25 Third assumption: relevance, first stage The average causal effect of the instrument on the treatment is not zero. Formally, E [Di (1) − Di (0)] ̸= 0 In the following we assume without lack of generality that E [Di (1) − Di (0)] > 0 Blaise Melly (University of Bern) IV with heterogeneous potential outcomes 14 / 25 Fourth assumption: monotonicity Introduced by Angrist and Imbens (1994): Di (1) ≥ Di (0) for ∀i Instrument may have no effect on some people, but all those who are affected are affected in the same way. Blaise Melly (University of Bern) IV with heterogeneous potential outcomes 15 / 25 Compliance types For binary instrument and treatment, we can define four types of individuals: always-takers: Di (1) = Di (0) = 1 never-takers: Di (1) = Di (0) = 0 compliers: Di (1) = 1, Di (0) = 0 defiers: Di (1) = 0, Di (0) = 1 The monotonicity assumption implies that there are no defiers. The population proportions of these types are identified. Blaise Melly (University of Bern) IV with heterogeneous potential outcomes 16 / 25 Random coefficients There is an equivalent representation as a random coefficients model In a traditional IV model we assumed Yi = α 0 + ρ i Di + η i Di = 1 (π0 + π1i Zi + ζ i ≥ 0) Assumptions independence (ηi , ζ i ) ⊥⊥ Zi monotonicity π1i ≥ 0 for all i relevance π1i > 0 for some i Blaise Melly (University of Bern) IV with heterogeneous potential outcomes 17 / 25 Local average treatment effects Under the four assumptions (theorem 4.4.1 in Angrist and Pischke) E (Yi |Zi = 1) − E (Yi |Zi = 0) = E ( Di | Zi = 1 ) − E ( Di | Zi = 0 ) E (Yi (1) − Yi (0) |Di (1) > Di (0)) Same estimator, different interpretation. What does the IV estimator identifies if the monotonicity assumption is violated (i.e. there are defiers)? Do we need the monotonicity assumption if the average treatment effect for the defiers is the same as the average treatment effect for the compliers? Blaise Melly (University of Bern) IV with heterogeneous potential outcomes 18 / 25 One-sided perfect compliance Example: Job Training Partnership Act Non-compliance: 40% of the persons randomly assigned to the treatment decide not to take the treatment. One-sided perfect compliance: none of the control units can take the treatment. It is impossible to be treated when the instrument is 0. The decision to be effectively treated is probably non-random, so regressing Y on D will produce biased results. What is the interpretation of the regression of Y on Z , the indicator of random assignment to training? it is the reduced form of 2SLS with Z as instrument it is the causal effect of the intention to treat (ITT-effect) Blaise Melly (University of Bern) IV with heterogeneous potential outcomes 19 / 25 One-sided perfect compliance (cont.) Can we do better? Yes, the IV estimator identifies the LATE. In this particular case: LATE =ATET. Blaise Melly (University of Bern) IV with heterogeneous potential outcomes 20 / 25 Empirical results Blaise Melly (University of Bern) IV with heterogeneous potential outcomes 21 / 25 Describing compliers We don’t know whether an individual is a complier or not. But we know the proportion of compliers in the population. And we can identify the distribution of the covariates for compliers. Blaise Melly (University of Bern) IV with heterogeneous potential outcomes 22 / 25 Example Blaise Melly (University of Bern) IV with heterogeneous potential outcomes 23 / 25 LATE with multiple instruments The LATE is always closely connected to the underlying instrument, since whether someone is a complier likely depends on what the instrument is. Different instruments will therefore identify different LATEs. ”Overidentification tests” are no longer tests of the validity of the model. Using 2SLS with several instruments simultaneously produces a linear combination of the instrument-specific LATEs. Whether or not that is interesting clearly depends on the context. Blaise Melly (University of Bern) IV with heterogeneous potential outcomes 24 / 25 Covariates We may want to include covariates in the estimation 1 Even if the instrument is valid unconditionally including covariates may reduce the s.e. 2 Sometimes the instrument is valid only conditionally on the covariates. In such a case, the LATE is still identified but requires a different estimator. 2SLS identifies a weighted-average of covariate-specific LATEs. The weights are proportional to the average conditional variance of the population first-stage fitted value. Blaise Melly (University of Bern) IV with heterogeneous potential outcomes 25 / 25