Big Data in Health PDF
Document Details
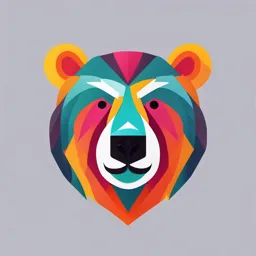
Uploaded by StableEpilogue
King's College London
2024
Prof Vasa Ćurčin
Tags
Summary
This presentation provides an overview of big data in health, including its characteristics, challenges in its application, and the concept of a Learning Health System. The presentation explores various aspects of big data in healthcare, such as data collection, challenges, and the benefits of using big data to improve healthcare.
Full Transcript
Faculty of Life Science and Medicine 26th January 2024 Prof Vasa Ćurčin Department of Population Health Sciences Block Title: Health Informatics and Evidence-Based Practice (HIEBP) Big Data technologies in Health Study Design and Summarising Data Learning Objectives Understand the concept of...
Faculty of Life Science and Medicine 26th January 2024 Prof Vasa Ćurčin Department of Population Health Sciences Block Title: Health Informatics and Evidence-Based Practice (HIEBP) Big Data technologies in Health Study Design and Summarising Data Learning Objectives Understand the concept of Big Data Inspect challenges of research with health data Types of bias in Big Health Data Appreciate the importance of reproducibility in health research Understand the concept of Learning Health Systems 26th January 2024 Professor Vasa Ćurčin Topic title: Big Data 2 Deluge of data 3 26th January 2024 Professor Vasa Ćurčin Topic title: Big Data Deluge of data Mobile sensors Social media Video surveillance Medical imaging Gene sequencing… 4 26th January 2024 Professor Vasa Ćurčin Topic title: Big Data Deluge of data New routes of exploitation: Credit card companies identify fraudulent behaviour Mobile phone companies prevent churn Companies such as Facebook and LinkedIn treat data as their primary product. Valuations based on data they control. 5 26th January 2024 Professor Vasa Ćurčin Topic title: Big Data Characteristics of ”Big Data” Huge volume of data Billions of rows, millions of columns Complexity of data types and structures Relational, unstructured Speed of new data creation and growth 3(4) V-s of Big Data: Volume Velocity Variety (Veracity) Big Data is data whose scale, distribution, diversity, and/or timeliness require the use of new technical architectures and analytics to enable insights that unlock new sources of business value McKinsey & Co.; Big data: The Next Frontier for Innovation, Competition, and Productivity 26th January 2024 Professor Vasa Ćurčin Topic title: Big Data 4 V-s variant 26th January 2024 Professor Vasa Ćurčin Topic title: Big Data Business Intelligence vs Big Data Analytics 26th January 2024 Professor Vasa Ćurčin Topic title: Big Data Structure 26th January 2024 Professor Vasa Ćurčin Topic title: Big Data Software Tools Preparation / Extract Transform Load: Python - Pandas Hadoop Data Wrangler / Trifacta Machine learning: model planning and building: Python, TensorFlow, PyTorch R SAS/ACCESS SQL Analysis SPSS Matlab STATA 26th January 2024 Professor Vasa Ćurčin Topic title: Big Data Data in health 26th January 2024 Professor Vasa Ćurčin Topic title: Big Data 11 Healthcare data architecture Source systems Data Integration Enterprise Data Data Marts Research EHR Real-time EDW Load Finance Accidents & Emergency Clean oad L Quality Finance Periodic Standardise Improvement Reformat Clinical Trial Lo ad Supply Management Chains 1-time feed Claims data ODS Data Social Security Extracts 26th January 2024 Professor Vasa Ćurčin Topic title: Big Data Elements of a healthcare architecture Enterprise Data Warehouse (EDW) Central repository of all relevant data Non-dimensional models, not changing over time Data extracted through programmatic access Data Marts Application oriented, ETL-ed from Enterprise Data Warehouses Operational Data Store (ODS) Trimmed down Enterprise Data Warehouses Immediate, real-time access to operational data 26th January 2024 Professor Vasa Ćurčin Topic title: Big Data Modelling the knowledge How do we represent the medical knowledge in data, so that it is: Standardised Portable Computable Text means nothing Not searchable Not interoperable Not computable Computers need codes – i.e human input to define a concept more clearly at input. 26th January 2024 Professor Vasa Ćurčin Topic title: Big Data Informatics Classification – A systematic representation of terms and concepts and the relationship between them. The apple is the fruit of the APPLE TREE, which is part of the ROSE family. Nomenclature (vocabulary) – An agreed system of assigned names. Type 2 diabetes is a life-long disease marked by high levels of sugar in the blood. It occurs when the body does not respond correctly to insulin, a hormone released by the pancreas. Type 2 diabetes is the most common form of diabetes. Terminology – A set of words or expressions together with definitions used within a certain field Codes – numeric or alphanumeric abbreviations ICD-10 - E11 Read v3 – CT10F Umls - C0375115 ICPC – T31 SNOMED CT 16403005 26th January 2024 Professor Vasa Ćurčin Topic title: Big Data Challenge of bias in RWD Collected data used for multiple purposes Patient information may not be complete, accurate, or current. Clinicians and insurers have to be aware of this Greater attention needs to be paid to the context in which data is recorded in the EHR system. Addressing information gaps in Randomised Control Trials Tracking provenance of data being produced Reimbursement bias Why record a Body Mass Index (BMI) in a thin person? Software bias System initiated – UK eHRs don’t allow negative values and Data errors 1% ‘resurrection’ rate in one UK longitudinal study Myocardial infarction in code ‘NOT’ in text…. Different pick lists for terminologies and the use of non-standard representations e.g. BP! 26th January 2024 Professor Vasa Ćurčin Topic title: Big Data Possible sources of bias 1 Health care system bias a Reimbursement system, pay for performance (why record BMI of a thin person?) b Role of clinician in the health care system; gatekeeping/non-gatekeeping c Professional guidelines for recording (UK’s Quality Outcomes Framework) d Ease of access by patients to their records e Data sharing between health care providers 2 Practice workload 3 Variations between EHR system functionalities and lay-out 4 Coding systems and thesauruses 5 Knowledge and education regarding the use of electronic health record systems 6 Data extraction tools 7 Data processing – re-databasing 8 Research dataset preparation 9 Research methodologies 26th January 2024 Professor Vasa Ćurčin Topic title: Big Data Anonymisation techniques Quantitative removing or aggregating variables reducing the precision or detailed textual meaning of a variable In relational data, where connections between variables in related datasets can disclose identities For geo-referenced data, where identifying spatial references also have a geographical value. Qualitative identifiers should not be crudely removed or aggregated, as this can distort the data or even make them unusable Pseudonyms, replacement terms or vaguer descriptors should be used. The objective: reasonable level of anonymisation whilst maintaining maximum content. 26th January 2024 Professor Vasa Ćurčin Topic title: Big Data Obstacles in Big Data collection Restrictive policies on data access Lack of standard policy on patient data privacy/confidentiality No international standardisation on data collection routes Licenses for access to data can be expensive 26th January 2024 Professor Vasa Ćurčin Topic title: Big Data Data governance Each research data set has associated with it its own set of information governance regulations, which vary depending on: the type of data, presence of consent, relevant data controller, the parameters of the data collection Some data sources differentiate between confidential (patient-identifiable) data and sensitive data Sensitive: ethnicity, geographical information (sometimes including general practice location), political and religious views, and criminal records. Exact definition of these two classes of data is variable, even for the data sources with the same controller. 26th January 2024 Professor Vasa Ćurčin Topic title: Big Data Research data governance in UK NHS data collected for clinical or administrative purposes can be used without consent for clinical audit and service evaluation, but not always for research. However, most uses of this data are for observational research, often indistinguishable from service evaluation. Clinical trials require ethical approval by the National Research Ethics Committee 26th January 2024 Professor Vasa Ćurčin Topic title: Big Data Legislation in UK Data Protection Act 1998 Provisions for secure processing of identifiable data for medical research No definitions of “secure” and “medical research” Led to consent-or-anonymise approach According to Information Commisioner's Office (ICO) anonymisation code Health and Social Care Act 2002 Section 251 of the NHS Act of 2006 provisions for allowing linkage of patient-identifiable data Applications made to Health Research Authority (HRA) NHS Information Centre for Health and Social Care (NHSIC) Application assistance Trusted third party for data linkage 26th January 2024 Professor Vasa Ćurčin Topic title: Big Data Caldicott 2 review Distinction was made between: data for publication which is anonymised and from which determining individual identities is unlikely, personal confidential data, which should only be disclosed with consent or under statute de-identified data for limited disclosure or access which could potentially be re-identified, particularly with access to other data sets. To support the use of the latter data category, report recommended: contracts to prevent re-identification, assured data stewardship agreements and restriction of any linkage to accredited safe havens. 26th January 2024 Professor Vasa Ćurčin Topic title: Big Data Open Data initiatives UK Government committed to promoting use of data for research Clinical Practice Research Datalink (CPRD) established jointly by the NIHR and the Medicines and Healthcare products Regulatory Agency (MHRA) provides anonymised linked data from general practice, HES, registries, and other sources. Applications approved by external committee – ISAC – and for specified study only 26th January 2024 Professor Vasa Ćurčin Topic title: Big Data Challenges of Research Data Management Insufficient incentive for researchers to publish datasets Academic funders and institutions to add dataset citation indices to research excellence assessment, with clear mechanisms for referencing (e.g. DOI-s) Academia, government, publishers Governance models outdated and too restrictive, with little or no audit of adherence More devolved approval process for dataset usage needed, with proactive approach by the Health Research Authority, that is taking over from National Information Governance Board. Government, NHS Lack of awareness of data available to researchers within institutions Introduce metadata registries where users can find details on available data sets and their governance and provenance information. Academia, industry 26th January 2024 Professor Vasa Ćurčin Topic title: Big Data Challenges of Research Data Management Little or no provenance captured during data analysis Increase usage of provenance-aware software tools and middleware in standard research practice, and incorporate it into publication requirements. Academia, industry, publishers Poor data management and lack of coherent analytical software strategy Better health informatics training and permanent data manager and software architect positions in health research groups Academia, industry. 26th January 2024 Professor Vasa Ćurčin Topic title: Big Data Reproducibility challenge Research community is struggling to ensure transparency and correctness of published research Reasons complex and interleaving (positive bias, intractable analysis, deluge of journals) Bayer Healthcare team published work showing that only 25% of the academic studies they examined could be replicated Prinz et al. Nat. Rev. Drug Discov. 10, 712, 2011 Of 53 oncology studies from 2001-2011, each highlighting big new apparent advances in the field, only 11% (6) could be robustly replicated. Begley & Ellis Nature 483, 531–533, 2012 26th January 2024 Professor Vasa Ćurčin Topic title: Big Data Reproducibility gap Out of 18 microarray papers, results from 10 could not be reproduced 1. Ioannidis et al., 2009. Repeatability of published microarray gene expression analyses. Nature Genetics 41: 14 2. Science publishing: The trouble with retractions http://www.nature.com/news/2011/111005/full/478026a.html 3. Bjorn Brembs: Open Access and the looming crisis in science https://theconversation.com/open-access-and-the-looming-crisis-in-science-14950 26th January 2024 Professor Vasa Ćurčin Topic title: Big Data Threats to reproducible science 29 26th January 2024 Professor Vasa Ćurčin Topic title: Big Data Reporting Experimental results What the... We didn't do any of are reproducible - on this! Has my supervisor edited it Thursdays #overlyhonestmethods without telling me? Oh, great. Now I'll look stupid #overlyhonestmethods Heat shock of E.coli was performed at 42-43 degree C for exactly 45-120 seconds. #overlyhonestmethods 26th January 2024 Professor Vasa Ćurčin Topic title: Big Data Reporting standards Traceability and accountability of research data are essential in clinical research Standards incude: GxP (including Good Clinical Data Management Practice and Good Clinical Practice) CONSORT for trial reporting CDISC ADAM – documents each derived variable STROBE for reporting observational studies RECORD, evolution of STROBE REporting of studies Conducted using Observational Routinely-collected Data 26th January 2024 Professor Vasa Ćurčin Topic title: Big Data Learning Health System “... one in which progress in science, informatics, and care culture align to generate new knowledge as an ongoing, natural by-product of the care experience, and seamlessly refine and deliver best practices for continuous improvement in health and health care.” (Institute of Medicine) We can’t afford to waste data! 26th January 2024 Professor Vasa Ćurčin Topic title: Big Data Context for the Learning Health System Persistent issues with clinical research Hard to identify subjects Complex, costly CRFs with duplicate data entry Funding not cost-effective Integrated approach needed between clinical trials and observational studies Secondary problem: Diagnostic error 60% of litigation claims against GPs (UK/EU/US) Failure of Decision Support Systems for Diagnosis System increasingly data-driven! Fundamentally a cross-disciplinary challenge 26th January 2024 Professor Vasa Ćurčin Topic title: Big Data Learning Health System Defining functions of a LHS are to: 1.routinely and securely aggregate data from disparate sources 2.convert the data to knowledge 3.disseminate that knowledge, in actionable forms, to everyone who can benefit from it. c/o C. Friedman 26th January 2024 Professor Vasa Ćurčin Topic title: Big Data LHS Endorsements 26th January 2024 Professor Vasa Ćurčin Topic title: Big Data Journal from Wiley www.lhsjournal.com Theory, complex issues, conceptual syntheses, education models Research reports, experience reports, technical reports, briefs and commentaries. 26th January 2024 Professor Vasa Ćurčin Topic title: Big Data Checklist View: Properties of an LHS Every consenting patient’s characteristics and experience are available to learn from Best practice knowledge is immediately available to support decisions Improvement is continuous through ongoing study An infrastructure enables this to happen routinely and with economy of scale All of this is part of the culture 26th January 2024 Professor Vasa Ćurčin Topic title: Big Data Macro view: An Ultra-Large Scale System Trusted Decentralised Reciprocal All-Inclusive Pharma Patient Groups Insurers Tech Industry Governance Engagement Data Aggregation Universities Analysis Dissemination Healthcare Delivery Government/Public 38 Networks Health Research 38 26th January 2024 Professor Vasa Ćurčin Topic title: Big Data Institutes How Learning Happens: Virtuous Cycles Interpret Results Tailored Messages Analyze to Decision-Makers Data A Problem of Interest Take Assemble Action Experience Data Formation of Learning Community 26th January 2024 Professor Vasa Ćurčin Topic title: Big Data Example: Precision Medicine Interpret Results: Are the results credible? What advice should be given? Analyze Data: What predicts Tailored Messages: better health Tailoring For this patient, the best therapy is… status? Intervention to the Individual Take Action: Assemble Data: Patient Administer Patient genotypes, recommended or clinical history, other therapy environment and 26th January 2024 health status Professor Vasa Ćurčin Topic title: Big Data Community Formation Summary Big Data has become all-pervasive in our daily lives In health, it offers multiple opportunities for improving treatments, outcomes and health systems Important to understand the biases present in the data Science has to be conducted in a responsible and reproducible manner Ideal of a Learning Health System Examples of questions to be asked: Explain the concept of Big Data, its characteristics and give some examples How does research with Big Data differ from classical research approaches What are some of the biases you may encounter in Big Health Data Why is reproducibility particularly relevant in health research What are Learning Health Systems, and give an example of a system you are familiar with that could be transformed into an LHS 41 26th January 2024 Professor Vasa Ćurčin Topic title: Big Data Reference List DOMO Annual Reports, https://www.domo.com/data-never-sleeps Verheij RA, Curcin V, Delaney BC, McGilchrist MM. Possible Sources of Bias in Primary Care Electronic Health Record Data Use and Reuse. J Med Internet Res. 2018 May 29;20(5):e185. doi: 10.2196/jmir.9134. PMID: 29844010; PMCID: PMC5997930. Munafò, M., Nosek, B., Bishop, D. et al. A manifesto for reproducible science. Nat Hum Behav 1, 0021 (2017). https://doi.org/10.1038/s41562-016-0021 Friedman, CP, Allee, NJ, Delaney, BC, Flynn, AJ, Silverstein, JC, Sullivan, K, Young, KA. The science of Learning Health Systems: Foundations for a new journal. Learn Health Sys. 2017; 1:e10020. doi: 10.1002/lrh2.10020 26th January 2024 Professor Vasa Ćurčin Topic title: Big Data 42 Thank you Contact details/for more information: Prof. Vasa Ćurčin [email protected] www.kcl.ac.uk/people/vasa-curcin © 2020 King’s College London. All rights reserved