Hypothesis Testing, P Values and Statistical Inference MD115 PDF
Document Details
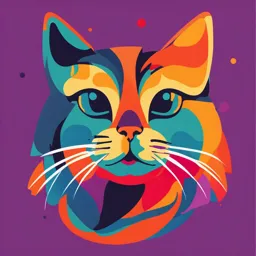
Uploaded by FieryBodhran
European University Cyprus, School of Medicine
2024
Evi Farazi, PhD
Tags
Summary
This document covers hypothesis testing, specifically focusing on statistical inference and p-values for students in MD115 Fall 2024 offered by the European University Cyprus, School of Medicine. The document contains sections explaining concepts like null and alternative hypotheses, significance levels and p-values, and includes examples and exercises.
Full Transcript
Hypothesis Testing, P Values and Statistical Inference MD115 Evi Farazi, PhD Week 4 - Hypothesis Testing Null Hypothesis Statistical analysis is concerned not only with summarising data but also with investigating relationships. An investigat...
Hypothesis Testing, P Values and Statistical Inference MD115 Evi Farazi, PhD Week 4 - Hypothesis Testing Null Hypothesis Statistical analysis is concerned not only with summarising data but also with investigating relationships. An investigator conducting a study usually has a theory in mind; for example, patients with diabetes may have raised blood pressure, or oral contraceptives may cause breast cancer. This theory is known as the study or research hypothesis or research question. However, it is impossible to prove most hypotheses; one can always think of circumstances which have not yet arisen under which a particular hypothesis may or may not hold. The converse of the research hypothesis is the null hypothesis. Example: Distance Walked On an Endurance Shuttle Walking Test (ESWT) Before and After a Rehabilitation Programme in Patients with COPD Chronic obstructive pulmonary disease (COPD) is the name for a collection of lung diseases including chronic bronchitis, emphysema and chronic obstructive airways disease. The damage to the lungs caused by COPD is permanent, but treatment can help slow down the progression of the condition. Treatments include pulmonary rehabilitation – a specialised programme of exercise and education. The Endurance Shuttle Walk Test (ESWT) is a standardised field test for the assessment of endurance capacity in patients with chronic lung disease. The ESWT is performed on a 10 m long course and allows people to walk at a steady pace equivalent to 85% of their maximal oxygen uptake. Patients with COPD recruited into a randomised controlled trial pre‐ and post‐ a six‐week pulmonary‐rehabilitation exercise programme. Patients are instructed to walk as long as possible at the speed that is dictated by an auditory signal. The test is ended when a patient is more than 0.5 m away from the marker before the signal was given on two successive shuttles, or when the patient has indicated they are too exhausted to carry on walking. The test is conducted pre- and post- the six-week pulmonary rehabilitation exercise program. What is the research question? What is the research hypothesis? What is the null hypothesis? What is the research question? Does an exercise programme (rehabilitation) change the distance walked of patients with COPD? What is the research hypothesis? There is a change in distance walked before and after exercise. What is the null hypothesis? There is no change in distance walked before and after exercise. The Main Steps in Hypothesis Testing Step 1: State Your Null Hypothesis (H0) and Alternative Hypothesis (HA) Research hypothesis is that the new treatment will be more effective than the standard or that exposure to the stimulus will change the subject's response or outcome. The null hypothesis is often the negation of the research hypothesis, i.e. no differences. Step 2: Choose a Significance Level, α, for Your Test For consistency we have to specify at the planning stage a value, α, so that once the study is completed and analysed, a P‐value below this would lead to the null hypothesis (which is specified in step 1) being rejected. Thus, if the P‐value obtained from a trial is ≤ α, then one rejects the null hypothesis and concludes that there is a statistically significant difference between treatments. On the other hand, if the P‐value is > α then one does not reject the null hypothesis. Although the value of α is arbitrary, it is often taken as 0.05 or 5%. Small ≤ α Large > α Your results are unlikely when Your results are likely when the null hypothesis is true. the null hypothesis is true. Step 3: Obtain the Probability of Observing Your Results, or Results More Extreme, if the Null Hypothesis is True (P‐value) First calculate a test statistic using your data (this reduces your data down to a single number or value). The general formula for a test statistic is: This test statistic value is then compared to a distribution that we expect if the null hypothesis is true (such as the Normal distribution with mean zero and standard deviation of one, or the t, chi‐squared or F‐distributions) to obtain a P‐value. Step 4: Use Your P‐value to Make a Decision About Whether to Reject, or Not Reject, Your Null Hypothesis We say that our results are statistically significant if the P‐value is less than the significance level α, which is usually set at 5% or 0.05. Example: Distance Walked on a 6MWT Before and After a Rehabilitation Programme The four main steps for hypotheses testing with the distance walked before and after a rehabilitation programme are: Step 1: State Your Null Hypothesis (H0) and Alternative Hypothesis (HA). H0: no difference (or change) in the mean distance walked in patients with COPD before and after exercise (note it is the difference in the population that is of interest – we would expect differences in individual patients), i.e. δPair = 0 m. HA: there is a difference (or change) in the mean distance walked in patients with COPD before and after exercise (could increase or decrease – two‐sided), i.e. δPair ≠ 0 m. Step 2 Choose a Significance Level, α, for Your Test Although the value of α is arbitrary, it is often taken as 0.05 or 5%. Step 3 Obtain the Probability of Observing Your Results, or Results More Extreme, If the Null Hypothesis is True (P‐value) First calculate a test statistic using your data (reduce your data down to a single value). The general formula for a test statistic is: n = 161 patients with COPD, the sample paired mean difference in distance walked is 251.6 m, with a standard deviation, s, of 351.1 m and a standard error of: The corresponding test statistic is: The test statistic, z, is compared to a distribution that we expect if the null hypothesis is true (such as the Normal distribution with mean zero and standard deviation unity) to obtain a P‐value. Using the corresponding Table with Z = 9.10, a P‐value 0.05 Result is Not Statistically significant Decide That there is insufficient evidence to reject the null hypothesis We cannot say the null hypothesis is true, only that there is not enough evidence to reject it. If one rejects the null hypothesis when it is in fact true, then one makes what is known as a Type I error. The significance level α is the probability of making a Type I error and is set before the test is carried out. The P‐value is the result observed after the study is completed and is based on the observed result. The term statistically significant is spread throughout the published medical literature. It is a common mistake to state that it is the probability that the null hypothesis is true as the null hypothesis is either true or it is false. The null hypothesis is not, therefore, ‘true’ or ‘false’ with a certain probability. P‐value can be thought of as a measure of the strength of the belief in the null hypothesis. Statistical significance does not necessarily mean the result is clinically significant or important. Power of a Study The power is defined as one minus the probability of a Type II error, thus the power equals 1 – β. The power is the probability of obtaining a ‘statistically significant’ P‐value when the null hypothesis is truly false. Test Statistically Difference Exists Difference Does Significant HA True not Exist H0 True Yes Power (1-b) Type I error (a) No Type II error (b) One-Sided vs Two-Sided Test The P‐value is the probability of obtaining a result at least as extreme as the observed result when the null hypothesis is true, and such extreme results can occur by chance equally often in either direction (i.e. calculate a two‐sided P‐value). In the vast majority of cases this is the correct procedure. In rare cases it is reasonable to consider that a real difference can occur in only one direction, so that an observed difference in the opposite direction must be due to chance. Here, the alternative hypothesis is restricted to an effect in one direction only (i.e. calculate a one‐sided P‐value by considering only one tail of the distribution of the test statistic). For a test statistic with a Normal distribution, the usual two‐sided 5% cut‐off point is 1.96, whereas the corresponding one‐sided 5% cut‐off value is 1.64. Confidence Interval All that we know from a hypothesis test is, for example, that there is a difference in the distance walked of COPD patients before and after exercise. It does not tell us what the difference is or how large the difference is. To answer this, we need to supplement the hypothesis test with an estimate and a CI, which will give us a range of values in which we are confident the true population mean difference will lie. P value and Clinical Importance The P‐value does not relate to the clinical importance of a finding, as it depends to a large extent on the size of the study. Thus, a large study may find small, unimportant, differences that are highly significant and a small study may fail to find important differences. P value and Clinical Importance - Example A study into the effects of alcohol on health (GBD 2016, Alcohol Collaborators 2018) showed a statistically significant risk of drinking slightly more than the UK guidelines of 14 units per week. This does not indicate a clinically significant risk. About 914 people in 100 000 would die in a year if they did not drink, and this is raised to 918 in 100 000 for those that drank one alcoholic drink per day. Thus about 4 people in 100 000 would die in a year as a result of drinking one alcoholic drink per day. This is a very low risk, and other risks (such as driving a car) are much more hazardous. Relationship Between Confidence Intervals and Statistical Significance If the 95% CI does not include zero (or, more generally the value specified in the null hypothesis) then a hypothesis test will return a statistically significant result. If the 95% CI does include zero, then the hypothesis test will return a non‐significant result. The CI shows the magnitude of the difference and the uncertainty or lack of precision in the estimate of interest. Thus, the CI conveys more useful information than a P‐value. For example, whether a clinician will use a new treatment that reduces blood pressure or not will depend on the amount of that reduction and how consistent the effect is across patients. So, the presentation of both the P‐value and the CI is desirable. Confidence Interval and P value Supplementing the hypothesis test with a CI will indicate the magnitude of the result and this will aid the investigators to decide whether the difference is of interest clinically. The CI gives an estimate of the precision with which a statistic estimates a population value, which is useful information for the reader. This does not mean that one should not carry out statistical tests and quote P‐values, rather that these results should supplement an estimate of an effect and a CI. Many medical journals now require papers to contain CIs where appropriate and not just P‐values. Large Sample Tests for Two Independent Means or Proportions Null hypothesis of no difference between groups Large Sample Z‐Test for Comparison of Two Independent Means Can calculate Z value from Z test using sample estimates Z = d/SEPooled(d) Use Z value to find corresponding p value from table Calculate Confidence Intervals using SE and Z value Review Exercises for Week 4 1. Which (if any) of the following statements about P‐values is CORRECT? A. The P‐value from a hypothesis test is the probability of obtaining your results, or more extreme results. B. The P‐value from a hypothesis test is the probability of obtaining your results, or more extreme results, if the null hypothesis is true. C. If the P‐value is small then your results are unlikely when the null hypothesis is true. D. If the P‐value is large your results are likely when the null hypothesis is true. E. The P‐value ranges from 0 to 1. 2. Which (if any) of the following statements about the Type I error is CORRECT? A. The Type I error is the probability of rejecting the null hypothesis when it is true. B. The Type I error is the probability of a false positive result. C. The usual cut‐off for the Type I error rate in hypothesis tests is 0.05. D. The Type I error is the probability of rejecting the null hypothesis when it is false. E. We can reduce the risk of a Type I error by changing the level of statistical significance we demand from 1% to 5%. D is incorrect because A is true. E is incorrect because we increase the risk of a Type I error by going from a 1% to 5% level of statistical significance. 3. Which (if any) of the following statements about hypothesis testing is CORRECT? A. With a large sample size you will always calculate a large P‐value from your hypothesis test. B. The P‐value from a hypothesis test is the probability of obtaining your results, or more extreme results, if the null hypothesis were true. C. With a small sample size you will always calculate a small P‐value from your hypothesis test. D. The P‐value from a hypothesis test tells you what the difference is and how large the difference is. E. A statistically significant result means the result is clinically significant/practically important. Farndon et al. (2013) report the results of a randomised controlled trial that investigated the effectiveness of salicylic acid plasters (Corn plasters) compared with usual scalpel debridement for treatment of foot corns. One of the secondary outcome measures was the size of the index corn (in mm) measured at 3, 6, 9 and 12 months post‐randomisation. Table 6.4 shows the outcome data. You may assume a significance level of 0.05 or 5% has been specified for the various hypothesis tests. 4. Table 6.4 reports that the P‐value for the comparison of mean corn size at 12 months between the corn plaster and scalpel groups is P = 0.010. Which, if any of the following statements is CORRECT? A. The result at 12 months is statistically significant. B. The probability of getting this difference or more extreme by chance if there had been no difference in population mean outcomes is 0.010. C. The probability of getting this difference or more extreme is 0.010. D. There is sufficient evidence to reject the null hypothesis. E. The results are unlikely when the null hypothesis is true. 5. Table 6.4 reports that the 95% CI for the comparison of mean corn size at nine months between the corn plaster and scalpel groups is: −1.2 to 0.1 mm. The research literature suggests that a difference or change in corn size of 1 mm or more would be regarded as clinically or practically important. Therefore the 95% CI shows that the difference in mean corn size at nine months between the corn plaster and scalpel groups is: A. Statistically significant and potentially clinically important. B. Statistically significant and not clinically important. C. Statistically significant and clinically important. D. Not statistically significant but potentially clinically important. E. Not statistically significant and not clinically important. 6. Table 6.4 reports that the 95% CI for the comparison of mean corn size at 12 months between the corn plaster and scalpel groups is: −1.7 to −0.2 mm. The research literature suggests that a difference or change in corn size of 1 mm or more would be regarded as clinically or practically important. Therefore the 95% CI shows that the difference in mean corn size at 12 months between the corn plaster and scalpel groups is: A. Statistically significant and potentially clinically important. B. Statistically significant and not clinically important. C. Statistically significant and clinically important. D. Not statistically significant but potentially clinically important. E. Not statistically significant and not clinically important. 7. Table 6.4 reports that the 95% CI for the comparison of mean corn size at three months between the corn plaster and scalpel groups is: −1.5 to −0.5 mm. The research literature suggests that a difference or change in corn size of 0.5 mm or more would be regarded as clinically or practically important. Therefore the 95% CI shows that the difference in mean corn size at three months between the corn plaster and scalpel groups is: A. Statistically significant and potentially clinically important. B. Statistically significant and not clinically important. C. Statistically significant and clinically important. D. Not statistically significant but potentially clinically important. E. Not statistically significant and not clinically important. Summary Research questions need to be turned into a statement for which we can find evidence to disprove – the null hypothesis. The study data are reduced down to a single probability – the probability of observing our result, or one more extreme, if the null hypothesis is true (P‐value). We use this P‐value to decide whether to reject or not reject the null hypothesis. Remember that ‘statistical significance’ does not necessarily mean ‘clinical significance’ or ‘clinical or practical importance’. CIs should always be quoted with a hypothesis test to give the magnitude and precision of the effect size. Additional Reading 1. Laake P and Fagerland MW. Statistical inference. In: Laake et al (2015) Research in medical and biological sciences. p.1-41 Currently only sections 11.1, 11.2, 11.6, 11.7, 11.8 Read carefully and make sure you understand the concepts 2. The ASA Statement on p-Values: Context, Process, and Purpose, The American Statistician, 70:2, 129-133, DOI: 10.1080/00031305.2016.1154108 3. Altman DG, Bland JM. Interaction revisited: the difference between two estimates. BMJ 2005;326:219 4. https://www.stat.berkeley.edu/~stark/SticiGui/Text/zTest.htm