Week 6 Lecture 1 Statistical Inference PDF
Document Details
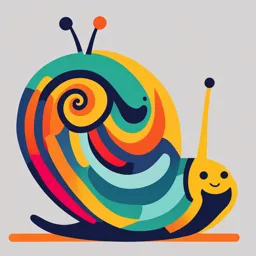
Uploaded by HeartwarmingSun
Nipissing University
Tags
Summary
This lecture covers inferential statistics, population vs. sample data, the standard error of the mean (SEM), and its application in hypothesis testing within the context of physical activity statistics. It includes a thought experiment and example questions on calculating and interpreting SEM.
Full Transcript
Week 6 Lecture 1: Statistical Inference PHED 3306: Statistics in Physical Activity Outline 1. Define inferential statistics 2. Review: Population vs Sample data 3. Standard Error of the Mean (SEM) 4. SEM and Z scores 5. A thought experiment Inferential statistics It is not practical to...
Week 6 Lecture 1: Statistical Inference PHED 3306: Statistics in Physical Activity Outline 1. Define inferential statistics 2. Review: Population vs Sample data 3. Standard Error of the Mean (SEM) 4. SEM and Z scores 5. A thought experiment Inferential statistics It is not practical to know a population mean We must infer the mean and error of a population We want to make conclusions about our data but there is a chance that we are wrong How much of a chance of being wrong is acceptable? Samples and Populations Population: Set of all individuals of interest in a particular study Sample: Set of individuals selected from a population Usually intended to represent population of interest in a study Samples and Populations Population (All individuals of interest) Results from the sample are Sample is selected generalized to the from the population population Sample (Individuals randomly selected to participate in study) Statistics and Parameters Parameter Statistic Value that describes a Value that describes a sample population Derived from measurements of Derived from measurements of individuals in all individuals in the sample the population The problem – samples are an estimate How do we use sample data? 1. We need to estimate the usual response – How do we do this? 2. We need to know how much error is in our estimation – How do we do this? Our best estimate: Mean +/- Standard Deviation How do we estimate error in a sample? Solution #1: Standard Deviation correction population sample NOTE When calculating the standard σ= ∑ (X − µ ) 2 SD = ∑ (X − X) 2 deviation of a sample, a correction N N −1 factor must be applied to the equation so that the estimate of the population is not biased by a small sample. Which one is larger? How do we estimate error in a sample? The solution #2: Standard Error of the Mean (SEM) μ Standard Error of the Mean (SEM) = amount of error that may exist when a random sample mean is Χ used to predict a population mean. µ = Χ ± SEM SD SEM = N Practice question: We want to estimate the population mean for the sit-and-reach tests for high school females. We obtain results from a sample of 64 subjects, with a mean of 35 cm and a standard deviation of 10 cm. 1. What is the standard error of the mean (SEM)? 2. What does it mean to have a large SEM? 3. What does it mean to have a small SEM? 4. What two ways can we reduce the SEM? Just like with SD, we can use SEM to create Z scores Z scores are valuable because: Z = X -X 1. Allow us to compare to the normal curve SEM 2. Allow us to make predictions 3. Allow us to test hypotheses 4. Allow us to understand the risk of being wrong The z scores of the normal distribution SEM -4SEM -3SEM -2SEM -1SEM 0 +1SEM +2SEM +3SEM +4SEM To refresh your memory: If you had a z score of 0.26 on a test, how many of your classmates scored higher than you? Recall: Standard Normal Distribution and Z scores =34.13 + 34.13 We need to find critical z scores What is the z score equal to the 2.5 percentile? What is the z score equal to the 97.5 percentile? -1.96 1.96 2.5% 95% 2.5% This gives us the Z scores we need to achieve for a p value of 0.05 to accept/reject the null hypothesis We want to run a thought experiment To do this we must: 1. Form a hypothesis 2. Collect data in a valid and reliable fashion 3. Find out if our hypothesis is correct 4. Understand how likely we are to be wrong in our conclusion Our question: Do biomechanists (B) have a different BMI than exercise physiologists (EP)? Forming a Statistical Hypothesis Create two mutually exclusive and exhaustive mathematical statements about the outcome of the analysis Mutually exclusive (only one of the two can be true) Exhaustive (no other option can exist) Statistical hypotheses: H0—the null hypothesis (e.g., there is no different between 2 groups) H1—the alternate hypothesis (e.g., there is a difference between 2 groups) Our hypothesis: Biomechanists (B) have a different BMI than Exercise Physiologists (EP) Ho = XB = XEP : BMIs is the same Ha = XB ≠XEP : BMIs are different Another easy way to do this… Ho = XB – XEP = 0 : Difference in the BMIs is 0 Ha = XB - XEP ≠0 : Difference in BMIs is not 0 Experiment: We collect BMI data from 50 Biomechanists and 50 Exercise Physiologists, all chosen at random These are 2 sample datasets from a 2 populations, so they each have: A mean A standard deviation A standard error of the mean We can calculate the mean difference in BMI between the groups (e.g., calculate XB – XEP) Hypothesis testing After calculating the mean difference estimate the probability (p) that you could have gotten a mean difference this big or bigger if H0 is true. This will give you an idea of the confidence in your conclusion Statistical Significance This decision of significance is based on the probability that you might be wrong Degree of risk you are willing to take that you will reject a null hypothesis when it is actually true. Significance Level: risk associated with not being 100% positive that what occurred in experiment is a result of what you did or what is being tested. Statistical Significance p <.05 is by far the most commonly used level of confidence. 5 % chance that you reject the null hypothesis when it is actually true You are 95% confident in your conclusion How is this level set? When should you be more stringent? When should you be less stringent? Type I and Type II Error Type I Error (FALSE POSITIVE) The probability of rejecting a null hypothesis when it is true Alpha level (α) Conventional levels are set between.01 and.05 Usually represented in a report as p <.05 Caused by: Measurement error If.01 is “better”, why Lack of random sample not set p @.0001? Alpha too liberal (e.g.,.10) Investigator bias Improper use of 1 tailed test Type II Error (FALSE NEGATIVE) Probability of accepting a null hypothesis when it is false. Beta level (β) β-level is often set at.2 (20%) More difficult to control than Type I Caused by: Measurement error Low power (N too small) Alpha too conservative (.01) Treatment effect not properly applied Draw a conclusion State the result (difference in BMI), your conclusion, and the the degree of confidence you have in this conclusion (p value) Indicates how generalizable the conclusion is to the larger population Summary Step 1: Select representative samples. Step 2: Collect the relevant data. Step 3: Reach a conclusion as to whether or not the difference between the scores is the result of chance. Step 4: Reach a conclusion that applies to the whole population based on the finding within the samples. Summary 1. Define inferential statistics 2. Review: Population vs Sample data 3. Standard Error of the Mean (SEM) 4. SEM and Z scores 5. A thought experiment Week 6 Lecture 1: Statistical Inference PHED 3306: Statistics in Physical Activity