Basic Statistics, Reliability, Validity, and Utility Lecture Notes PDF
Document Details
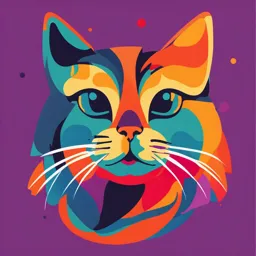
Uploaded by UnrivaledConnemara8735
National University - Manila
Prof. Reyxielle F. Tomas
Tags
Summary
These lecture notes provide an overview of basic concepts in statistics, including descriptive and inferential statistics, scales of measurement, and frequency distributions. The notes also cover various statistical concepts, such as percentiles, variability, standard deviation, and z-scores.
Full Transcript
BASIC STATISTICS FOR TESTING STATISTICS REFRESHER Prof. Reyxielle F. Tomas CEAS- Psychology Department National University- Manila Why we need statistics? Why we need statistics? Statistical methods serve two important purposes in the quest for scientific understanding...
BASIC STATISTICS FOR TESTING STATISTICS REFRESHER Prof. Reyxielle F. Tomas CEAS- Psychology Department National University- Manila Why we need statistics? Why we need statistics? Statistical methods serve two important purposes in the quest for scientific understanding. 1. Statistics are used for purposes of description. 2. We can use statistics to make inferences, which are logical deductions about events that cannot be observed directly. Why we need statistics? Descriptive Statistics are methods used to provide a concise description of a collection of quantitative information. Inferential Statistics are methods used to make inferences from observations of a sample to population. Samples and Populations Population: the entire group of individuals to which a law of nature applies. Sample: a relatively small subset of population that is intended to represent the population. Scales of Measurement Measurement: application of rules for assigning numbers to objects. The rules are the specific procedures used to transform qualities of attributes into numbers. Properties of Scales Three important properties make scales of measurement different from one another: Magnitude Equal Interval Absolute Zero Properties of Scales Magnitude The property of “moreness”. A scale has the property of magnitude if we can say that a particular instance of the attribute represents more, less, or equal amounts of the given quantity than does another instance. Properties of Scales Equal Intervals A scale has the property of equal intervals if the difference between two points at any place on the scale has the same meaning as the difference between two other points that differ by the same number of scale units. Properties of Scales Absolute 0 It is obtained when nothing of the property being measured exists. For many psychological qualities, it is extremely difficulty, if not impossible, to define an absolute 0 point. Types of Scales Nominal scales involve classification or categorization based on one or more distinguishing characteristics. (ex: DSM, yes or no scale, sex, etc.) Ordinal scales, rank ordering characteristics. (ex: board exam topnotchers, likert scale, level of agreement) Types of Scales When a scale has the properties of magnitude and equal intervals but not absolute 0, we refer to it as an interval scale. A scale that has all the three properties is called a ratio scale. Scales of Measurement and Their Properties Frequency Distributions A distribution of scores summarizes the scores for a group of individuals. The frequency distribution displays scores on a variable or a measure to reflect how frequently each value was obtained. With frequency distribution, one defines all the possible scores and determines how many people obtained each of those scores. Frequency Distributions Frequency The frequency Distributions distribution displays scores on a variable or a measure to reflect how frequently each value was obtained. With a frequency distribution, one defines all the possible scores and determines how many people obtained each of those scores. Frequency Distributions Frequency Distributions SKEWNESS Positively skewed- frequent scores are clustered at the lower end and the tail points toward higher or more positive scores. Negatively skewed- frequent scores are clustered at the higher end and the tail points toward lower or more negative scores. Frequency Distributions TEST IS DIFFICULT TEST IS EASY Frequency Distributions KURTOSIS- degree to which scores cluster at the ends of distribution (known as the tails). Leptokurtic- pointy positive kurtosis Mesokurtic- normal distribution Platykurtic- flat negative kurtosis Percentiles The specific scores or points within a distribution. Percentiles divide the total frequency for a set of observations into hundredths. It indicates the particular score, below which a defined percentage of scores falls. PERCENTILE RANKS replace simple ranks to adjust the number of scores in a group. Percentiles What percent of the scores fall below a particular score? To calculate: Percentiles Percentiles are specific scores or points within a distribution. Percentiles divide the total frequency for a set of observations into hundredths. The percentile and percentile rank are similar. The percentile rank gives the percentage of cases below the percentile. What percent of the scores fall below a particular score? Percentiles Example 01: A psychotherapist has rated all 20 of her patients in terms of their progress in therapy, using a 7-point scale. The results are shown in the following table: Percentiles Example 01: A psychotherapist has rated all 20 of her patients in terms of their progress in therapy, using a 7- point scale. The results are shown in the following table: 1. Percentile rank of a patient who improved slightly. Of a patient who became slightly worse. Percentiles Example 02: A cognitive psychologist is training volunteers to use efficient strategies for memorizing lists of words. The number of words correctly recalled by the participants are as follows: 25, 23, 26, 24, 19, 25, 24, 28, 26, 21, 24, 24, 29, 23, 19, 24, 23, 24, 25, 23, 24, 25, 26, 28, 25. Percentiles Example 02: A cognitive psychologist is training volunteers to use efficient strategies for memorizing lists of words. The number of words correctly recalled by the participants are as follows: 25, 23, 26, 24, 19, 25, 24, 28, 26, 21, 24, 24, 29, 23, 19, 24, 23, 24, 25, 23, 24, 25, 26, 28, 25. Percentile rank of a participant who scored 25. Of a participant who scored 27. Measures of Central Tendency Mean- the average of a data set/ arithmetic average score in a distribution. Median- the middle of the set of numbers. Mode- the most common number in a data set. Standard Deviation It is an approximation of the average deviation around the mean. A measure of how spread out numbers are. Is the square root of the average squared deviation around the mean. Standard Deviation Although the standard deviation is not an average deviation, it gives a useful approximation of how much a typical score is above or below the average score. Because of their mathematical properties, the variance and the standard deviation have many advantages. For example, knowing the standard deviation of a normally distributed batch of data allows us to make precise statements about the distribution. Standard Deviation In calculating the standard deviation, it is often easier to use the raw score equivalent formula, which is: Variance Variance is defined as the average of the squared differences from the mean. In other words, the variance is the average squared deviation around the mean. To get it back into the units that will make sense to us, we need to take the square root of the variance. The square root of the variance is the standard deviation (𝜎) Z score It transforms data into standardized units that are easier to interpret. A z-score is the difference between a score and the mean, divided by the standard deviation. T score (McCall’s T) It refers to a standardized score system that can be obtained from a simple linear transformation of Z scores. Mean= 50 Standard Deviation= 10 T score (McCall’s T) In effect, McCall generated a system that is exactly the same as standard scores (Z scores), except that the mean in McCall’s system is 50 rather than 0 and the standard deviation is 10 rather than 1. Indeed, a Z score can be transformed to a T score T score (McCall’s T) When to use? The general rule of thumb for when to use a t-score is when your sample size meets the following two requirements: The sample size is below 30 The population standard deviation is unknown (estimated from your sample data) In other words, you MUST know the standard deviation of the POPULATION and your sample size MUST be above 30 in order for you to be able to use the z-score. Otherwise, use the t-score. Standard Scores Z scores (mean= 0 , Sd= 1) T scores (mean=50, Sd= 10) Stanine scores (mean= 5, Sd=2) Sten scores (mean=5.5, Sd=2) IQ scores (mean=100, Sd= 15) CEE (mean=500, Sd=100) Variability Range: High score- Low score Quartile: division of frequency, normal distribution divided into 4 parts Interquartile: Q3-Q1 Semi-interquartile: Interquartile divided by 2 Application 1. The area from -+1 SD around the mean occupies approximately %. 2. If there are 30 test takers, what is the approximate number of scores that will fall between -2 and +0.5 SD? 3. If Nikko gets 160 points in a 200 point test, his score is enough to top 27 students out of 36 students. What is the percentage of Nikko’s score? 4. If Nikko gets 160 points in a 200 point test, his score is enough to top 27 students out of 36. What is the percentile rank of Nikko’s score? Statistical Methods Comparative (difference, effect) NO. OF TIMES DV MEASURED NO. OF GROUPS T-test independent means 1 2 T- test dependent means 2 1 Anova one way 1 >2 Anova repeated measures >2 1 Mann Whitney U test 1 2 Wilcoxon Signed Rank test 2 1 Kruskal Wallis test 1 >2 Friedman test >2 1 Anova two way 2 IV, 2 levels MANOVA Many measures of DV Parametric/ Non-Parametric Dependent T-test Wilcoxon Signed Rank Test Independent T-test Mann Whitney U Test Repeated Measures Anova Friedman Test One way/Two way Anova Kruskal Wallis Test Correlation Expresses the degree of correspondence or relationship between two separate scores. 3 types of Correlation: Perfect positive correlation (+1.00) Perfect negative correlation (-1.00) No correlation (0) Correlation Perfect positive correlation (+1.00) - One variable increases, another variable also increases Perfect negative correlation (-1.00) - One variable increases, another variable decreases No correlation (0) Correlation Interpretation of Scores: 0.00 No Relationship 0.01-0.24 Weak Relationship 0.25-0.49 Low Relationship 0.50-0.74 Strong Relationship 0.75-0.99 Very Strong Relationship 1.00 – Perfect Relationship Correlation Pearson Product Moment Correlation Coefficient – Pearson r is used to measure the test reliability. Applicable for Interval to Interval data Spearman rank- ordinal to ordinal data Statistical Significance If the p-value is less than the significance level (p=0.05), the decision is to ACCEPT the NULL HYPOTHESIS. If you accept the null, or you fail to reject it, this means that no relationship or differences were found. If you reject the null as false, this means that differences or relationships do exists. Probability Levels and Errors TYPE I ERROR (ALPHA)- occurs when you reject the null and it was actually true. We conclude falsely that there were differences when there was none. If the hypothesis is TYPE II ERROR (BETA)- occurs when you accept the true, you accept null and it was in fact false. We conclude that the null, if the there were no differences when in fact there were. hypothesis is false, you reject the null. SIGNIFICANT Ho is FALSE REJECT Ho RESULT Ho INSIGNIFICANT RESULT Ho is TRUE ACCEPT Ho HYPOTHESIS SIGNIFICANT RESULT Ha is FALSE ACCEPT Ha Ha INSIGNIFICANT RESULT Ha is TRUE REJECT Ha NORMS AND A GOOD TEST Norms Refers to the performances by defined groups on particular tests. Standard which results will be compared. It is used to give information about performance relative to what has been observed in a standardization sample. Norms Norm refer to behavior that is usual, average, normal, standard, expected, or typical. Norms, in a psychometric context, are the test performance data of a particular group of testtakers that are design for use as a reference when evaluating or interpreting individual test scores. Normative sample – is the group of people whose performance on particular test is analyzed for reference in evaluating the performance of individual testtakers. Norms Members of the normative sample will all be typical with respect to some characteristic(s) of the people for whom the particular test was design. Norming – refers to process of deriving norms; may be modified to describe a particular type of norm derivation. e.g. Race norming – the basis is the race or ethnic background. Norms Age-related Norms - Certain tests have different normative groups for particular age groups. Ex: SB-IQ test were obtained from various age groups. Norm-referenced Tests - Determine how a test taker compares with other or each person with a norm. Criterion-referenced Tests - Describes the specific types of skills, tasks, or knowledge a test taker can demonstrate. Norming Standardization – the process of administering a test to a representative sample of test takers for the purpose of establishing norms Sample – a portion of the universe of people that represents the whole population Sampling – process of selecting sample Sampling PROBABILITY SAMPLING Simple Random Sampling: everyone has an equal chance of being selected as part of the sample Systematic Sampling: every nth item or person after is picked Stratified Sampling: random selection within predefined groups Cluster Sampling: groups rather than individual units of the target population are selected randomly Sampling NON-PROBABILITY SAMPLING Convenience Sampling: selected based on their availability Quota Sampling: specifying who should be recruited for a survey according to certain groups or criteria Purposive Sampling: chosen consciously based on their knowledge and understanding of the research question Snowball or Referral Sampling: people recruited to be part of a sample are asked to invite those they know to take part RELIABILITY In psychological testing, the word error does not imply that a mistake has been made. error implies that there will always be some inaccuracy in our measurements. In other words, tests that are relatively free of measurement error are reliable, and tests that have so much measurement error are considered unreliable. Reliability can be estimated from the correlation of the observed test score with the true score. Reliability pertains to consistency of test measurement. A test may be reliable in one context and unreliable in another. Basics of Test Score Theory Classical Test Score Theory assumes that each person has a true score that would be obtained if there were no errors in measurement. The difference between the true score and the observed score results from measurement error. Basics of Test Score Theory The difference between the score we obtain and the score we are really interested is the error of measurement: X-T = E Basics of Test Score Theory Standard error of measurement (SEm) estimates how repeated measures of a person on the same instrument tend to be distributed around his “true”score. The true score is always unknown because no measure can be constructed that provides a perfect reflection of the true score. Basics of Test Score Theory Domain Sampling Theory Another central concept in CTT. It assumes that the items that have been selected for any one test are just a sample of items from an infinite domain of potential items. It considers the problems created by using a limited number of items to represent a larger and more complicated construct. Basics of Test Score Theory Domain Sampling Theory As the sample gets larger, it represents the domain more and more accurately. The greater the number of items, the higher the reliability. Basics of Test Score Theory Item Response Theory (IRT) A way to analyze responses to tests or questionnaires with the goal of improving measurement accuracy and reliability. It is used to focus on the range of item difficulty that helps assess an individual’s ability level. Item difficulty= item easiness Sources of Error Variance 1. Test Construction: item sampling or content sampling, terms that refer to a variation among items within a test as well as variation among items between tests. 2. Test Administration: may influence the test taker’s attention or motivation. 3. Test Scoring and Interpretation: individual administered test still require scoring by trained personnel. RELIABILITY ESTIMATE MODELS Test Re-Test Reliability 1 group, 1 test, 2 administration It is used to evaluate the error associated with administering a test at two different times. It is appropriate when evaluating the reliability of a test that purports to measure something that s relatively stable over time, ex: personality trait. COEFFICIENT OF STABILITY Test Re-Test Reliability Carryover Effect This effect occurs when the first testing session influences the scores from the old session. (short span of interval) The shorter the interval, the more at risk for carryover effect. Practice effect: the test-retest correlation usually overestimate the true reliability. (test-taker’s concern) Test Re-Test Reliability TEST-RETEST PROCEDURE Sample population = (Test A) + (Test A) Administer the psychological test Get the test result Wait for the interval (time gap) Re-administer the psychological test Get the test result Correlate the results What are the Disadvantages of Test-Retest? Possible better performance Checking / knowing the answers Practice effect Test Re-Test Reliability When the interval between testing is greater than six months, the estimate of test-retest reliability is often referred to as the coefficient of stability. The higher the interval, the higher the reliability. Test Re-Test Reliability Guidelines for interpreting the coefficient of stability: 0.9 and greater: excellent reliability Between 0.9 and 0.8: good reliability Between 0.8 and 0.7: acceptable reliability Between 0.7 and 0.6: questionable reliability Between 0.6 and 0.5: poor reliability Less than 0.5: unacceptable reliability Parallel-Forms & Alternate- Forms Reliability 1 group, 2 test, 1 administration Parallel forms reliability (Alternate form) – compares two equivalent forms of test that measure the same attribute. The two forms use different items; however the rules used to select items of a particular difficulty level are the same. Parallel-Forms & Alternate- Forms Reliability Two forms are administered to the same group of people on the same day. Pearson’s R product- moment correlation is used to estimate the reliability. Alternate forms are designed to be equivalent with respect to variables such as content and level of difficulty. Coefficient of equivalence- measures the same attributes. Parallel-Forms & Alternate- Forms Reliability PROCEDURE: Administer the first test Administer the Alternate form test Score both tests Correlate What are the disadvantages of using the Alternate Form Method? Hard to develop or construct Time consuming Split Half Reliability 1 group, 2 test, 2 administration It is obtained by correlating two pairs of scores obtained from equivalent halves of a single test administered once. A test is given and divided into halves that are scored separately. The results of one half of the test are then compared with the results of the other. Split Half Reliability 1 group, 2 test, 2 administration If the test is long, the best method is to divide the items randomly into two halves. To shorten the items, odd-even system or top bottom method can be used. Top bottom method: 1st half (25 items), 2nd half (25 items) Odd even system: 1st half (odd), 2nd half (even) Split Half Reliability To adjust the half-test reliability, use the Spearman Brown Formula. It allows a test developer or user to estimate internal consistency reliability from correlation of two halves of a test. Spearman-Brown formula is: r = 2r / 1+ r where, (r) is the estimated correlation between the two halves of the test (if each had had the total number of items) and r is the correlation between the two halves of the test. Kuder-Richardson Formula (KR20) KR20 is (Kuder & Richardson, 1937) developed this formula to measure the reliability estimate of Split-half. The formula is applicable to items that are DICHOTOMOUS, scored 0 or 1(usually right or wrong). It is used when the items varies the level of difficulty. KR21: used when the items have same level of difficulty. Kuder-Richardson Formula (KR21) KR21: used when the items have same level of difficulty. Instead, the KR21 uses an approximation of the sum of the pq products—the mean test score. The KR21 procedure rests on several important assumptions. The most important is that all the items are of equal difficulty, or that the average difficulty level is 50%. Difficulty is defined as the percentage of test takers who pass the test. Coefficient Alpha Cronbach’s Alpha (Cronbach, 1951) sometimes called coefficient alpha ( a ) is the most common measure of internal consistency (reliability). Cronbach’s Alpha is most commonly used when you have multiple Likert questions in a survey/questionnaire that form a scale and you wish to determine if the scale is reliable. Items are not scored as 0 or 1.Applicable for personality and attitude scales. Coefficient Alpha Coefficient alpha is the most general method of finding estimates of reliability through internal consistency. The only difference is that ∑pq has been replaced by ∑S2i. This new term, S2i , is for the variance of the individual items (i). The summation sign informs us that we are to sum the individual item variances. S2 is for the variance of the total test score. Internal Consistency All of the measures of internal consistency evaluate the extent to which the different items on a test measure the same ability or trait. They will all give low estimates of reliability if the test is designed to measure several traits. Using the domain sampling model, we define a domain that represents a single trait or characteristic, and each item is an individual sample of this general characteristic. When the items do not measure the same characteristic, the test will not be internally consistent. Method No. of Forms No. of Sessions Sources of Error Variance Test - Retest 1 2 Changes over time Alternative – Forms 2 1 Item sampling (Immediate) Alternative – Forms 2 2 Item sampling (Delayed) changes over time Split - Half 1 1 Item sampling Nature of split Coefficent Alpha & 1 1 Item sampling Kuder – Richardson Test Heterogeneity Inter - Scorer 1 1 Scorer Differences How reliable is reliability? In research setting, it has been suggested that a reliability estimates in the range of.70 and.80 are good enough for most purposes in basic research (Kaplan & Saccuzzo, 2005) In clinical setting, high reliability is extremely important. When tests are used to make important decisions about someone’s future, a reliability of.90 to.95 might be good enough If the test is unreliable, information obtained with it is of little or no value. Which type of Reliability is Appropriate? Tests designed to be administered to individuals more than once, it would be reasonable to expect that the test demonstrate reliability across time – test retest reliability; Split-half methods work well for instruments, which have items carefully ordered according to difficulty level; Inter-scorer reliability is appropriate for any test, which involves subjectivity of scoring. Reliability of Tests A PURE SPEED TEST is one in which individual difference depend entirely on speed of performance; such a test is constructed from items of uniformity low difficulty, all of which are well within the ability level of the person for whom the test is design. A PURE POWER TEST, on the other hand, has a time limit long enough to permit everyone to attempt all items. The difficulty of the items is steeply graded, and the test includes some items too difficult for anyone to solve, so that no one can get a perfect score. What to do with low reliability? 1. Increase the number of items. 2. Factor and item analysis. 3. Spearman Brown Formula What to do with low reliability? Factor and item analysis. Factor Analysis – designed to identify factors or specific variables that are typically attributes, characteristics, or dimensions on which people may differ ▪ Employed as data reduction method ▪ Identify the factor or factors in common between test scores on subscales within a particular test What to do with low reliability? Factor and item analysis. ▪ Explanatory FA – estimating or extracting factors; deciding how many factors must be retained ▪ Confirmatory FA – researchers test the degree to which a hypothetical model fits the actual data ▪ Factor Loading – conveys info about the extent to which the factor determine the test score or scores VALIDITY Validity, as applied to a test, is a judgment or estimate of how well a test measures what it purports to measure in a particular context. Validity indicated what the test aims or purports to measure. It answer the question, “Does the test measure what it is supposed to measure? Three Categories of Validity: 1. Content Validity 2. Criterion-related Validity - Predictive Validity - Concurrent Validity 3. Construct Validity - Convergent Validity - Discriminant Validity Face Validity Face validity is not really a validity at all because it does not offer evidence to support conclusions drawn from test score. Face validity relates more to what a test appears to measure to the person being tested than to what the test actually measures. Face validity only establishes the presentation, physical appearance of the psychological test (Is the test presentable to the test takers?) Content Validity It explores the appropriateness of test items of a psychological test. It means that the test covers the content what is supposed to cover. This describes a judgment of how adequately a test samples behavior representative of what the test was designed to sample. Test blueprint for the “structure” of the test evaluation. A plan regarding the types of information to be covered by the items. Criterion-related Validity Criterion: a standard on which a judgment or decision may be based. The standard against which a test or a test score is evaluated. Criterion validity refers to the ability to draw accurate inferences from test scores to a related behavioral criterion of interest. This refers to the extent to which a measure is related to an outcome. Criterion-related Validity CONCURRENT VALIDITY - statements of concurrent validity indicate the extent to which test scores may be used to estimate an individual’s present standing on a criterion. PREDICTIVE VALIDITY - how well a certain measure can predict future behavior. Criterion-related Validity PREDICTIVE VALIDITY how well a certain measure can predict future behavior. Measures of the relationship between test scores and a criterion measure obtained at a future time Base Rate – the extent to which a particular trait, behavior, characteristic, or attribute exist in the population Hit Rate – defined as the proportion of people a test accurately identifies possessing a particular trait, behavior, etc. Criterion-related Validity PREDICTIVE VALIDITY how well a certain measure can predict future behavior. Miss Rate – fails to identify having that particular characteristic False Positive –miss; the test predicted that they do possess a particular trait but actually not False Negative – miss; the test predicted they do not possess a particular trait but actually do Construct Validity A judgment about appropriateness of inferences drawn from test scores regarding individual standings on a variable called construct. It arrived at by executing a comprehensive analysis of: a. How scores on the test relate to other test scores and measures, and b. How scores on the test can be understood within some theoretical framework for understanding the construct that the test was designed to measure. Construct Validity CONVERGENT VALIDITY - when a measure correlates well with other tests believed to measure the same construct. - ex: correlate assessment scores for a math ability test with scores obtained from other math ability tests. DISCRIMINANT VALIDITY - a construct measure diverges from other measures that should be measuring different things. - ex: correlate assessment scores for a math ability test with scores obtained from a verbal ability test. Practicality of a Test A test must be usable Selection of the test should also be based on: -Effort -Affordability -Time frame Test requires simple directions Easy administration and scoring UTILITY usefulness or practical value of testing to improve efficiency can tell us something about the practical value of the information derived from scores on the test UTILITY One of the most basic elements in utility analysis is financial cost of the selection device Cost – disadvantages, losses, or expenses both economic and noneconomic terms Benefit – profits, gains or advantages The cost of test administration can be well worth it if the results is certain noneconomic benefits UTILITY Utility Analysis – family of techniques that entail a cost-benefit analysis designed to yield information relevant to a decision about the usefulness and/or practical value of a tool of assessment. How is Utility Analysis Conducted? Expectancy Data Expectancy table – provide an indication that a testtaker will score within some interval of scores on a criterion measure – passing, acceptable, failing. 1. Taylor-Russel Tables – provide an estimate of the extent to which inclusion of a particular test in the selection system will improve selection How is Utility Analysis Conducted? One limitation of Taylor-Russel Tables is that the relationship between the predictor (test) and criterion must be linear 2. Naylor-Shine Tables – entails obtaining the difference between the means of the selected and unselected groups to derive an index of what the test is adding to already established procedures. How is Utility Analysis Conducted? High performing applicants may have been offered in other companies as well The more complex the job, the more people differ on how well or poorly they do that job Cut Score – reference point derived as a result of a judgement and used to divide a set of data into two or more classifications. How is Utility Analysis Conducted? Relative Cut Score – reference point based on norm-related considerations (norm-referenced); e.g, NMAT ▪ Fixed Cut Scores – set with reference to a judgement concerning minimum level of proficiency required; e.g., Board Exams ▪ Multiple Cut Scores – refers to the use of two or more cut scores with reference to one predictor for the purpose of categorization Methods for Setting Cut Scores Angoff Method – setting fixed cut scores ▪ low interrater reliability Known Groups Method – collection of data on the predictor of interest from group known to possess and not possess a trait of interest ▪ The determination of where to set cutoff score is inherently affected by the composition of contrasting groups Methods for Setting Cut Scores IRT-Based Methods – cut scores are typically set based on testtaker’s performance across all the items on the test Method of Predictive Yield – took into account the number of positions to be filled, projections regarding the likelihood of offer acceptance, and the distribution of applicant scores Discriminant Analysis –relationship between identified variables and two naturally occurring groups End of Presentation Lesson 2: Basic Statistics, Reliability, Validity, Utility