Experimental Designs PDF
Document Details
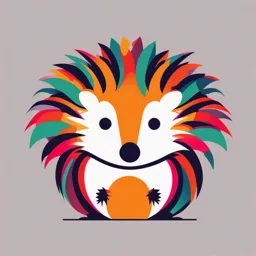
Uploaded by BlissfulCatSEye9737
Dalhousie University
Tags
Summary
This document provides an overview of experimental designs, including within-subject, between-subject, factorial, and mixed designs. It also covers relevant statistical concepts like descriptive and inferential statistics. The document likely serves as lecture notes or study material for a psychology or research methods course.
Full Transcript
Experimental designs Within-subject designs, between subject designs, mixed designs, factorial designs Experimental designs Within Quasi-experimental Between Ex-post facto Factorial Developmental designs (...
Experimental designs Within-subject designs, between subject designs, mixed designs, factorial designs Experimental designs Within Quasi-experimental Between Ex-post facto Factorial Developmental designs (quickly Mixed mentioned) Nested Correlational- experimental (not { covered in this class) This section Statistics: recap Descriptive statistics (important: underlined): describing the data. Measures of central tendency: arithmetic mean (average), harmonic mean, geometric mean, mode, median. Measures of variability: standard deviation (SD), variance, standard error (SE), con dence intervals (CI), least signi cant differences (LSD’s), coef cient of variation (CV), range. Inferential statistics: con rming or not the hypothesis or hypotheses (t-tests, ANOVA's, etc.) fi fi fi fi Terminology Factorial designs: when more than one independent variable is used (independent variable = factor). You have three main types of factorial designs: Between-subjects factorial designs Within-subjects factorial designs Combination of within and between subjects designs: Mixed (between and within) factorial design Nested factorial designs Factorial designs When two or more independent variables are used (bivalent or multivalent experiments), and each variable has 2 or more levels we talk about factorial designs. ANOVA's (or "analysis of variance") inferential statistics are needed for the data analysis of these experiments. Example: Between subjects factorial design Effect of a neurotoxin on a suppressed Factor B: Immunosuppressant drug immune system Placebo (saline) CsA Factor A: Placebo (saline) G1 G2 Neurotoxin Neurotoxin G3 G4 Between / Within designs Between subjects (groups) designs Type 1: Completely randomized designs. Type 2: Matched groups designs (more later). For t-tests: "t-test for independent groups" or unpaired t-test. Within subjects designs Also called: Repeated measures designs or randomized- blocks designs For t-tests: "t-test for dependent groups" or paired t-test or “related t-test” or “correlated t-test”. Mixed designs: within and between components. Also called split-plot designs. Analysis: SPANOVA. Also: Nested designs. Within subject designs Three main types: The same subject is observed under all treatment conditions. The same subject is observed before and after a treatment (pretest-posttest design). Subjects are matched on a subject variable* and then randomly assigned to the treatments. In fact, this is a between subject design requiring a within subject analysis of variance. * = organismic variable or individual differences variable Within-subjects designs: advantages Each level of the independent variable is applied to all subjects. So we can evaluate how each level of the independent variable affects each subject. Each subject is its own control. Excellent for assessing experiments on learning, transfer of training, practice effects. May help increase statistical sensitivity or statistical power (subjects are not divided into groups, all subjects are involved in all conditions). Within-subjects designs: disadvantages Practice effects: If not the focus of study, it becomes a problem. Solution: Appropriate counterbalancing procedures can counteract practice effects. Also: Make the treatment order an independent variable. Differential carryover effects: Lingering effects of one or more treatment conditions. Often an issue in drug studies. Solution: Recovery periods (intervals). Violation of statistical assumptions: Covered in inferential statistics course. Solution: Use a more strict signi cance level (e.g.,.025 instead of.05). fi Practice and carryover effects Practice/learning: increase in performance via practice. Sometimes considered a carryover effect. Not necessarily a problem in some experiments. Carryover effects: Fatigue: Decreased performance with time. Contrast: Treatments are compared by subjects. Habituation or sensitization. Adaptation*: e.g., tolerance in drug studies. * Note: habituation is often considered under conscious control, adaptation is not. Types of factorial designs The study example: Sleep study investigating the effect of synthetic melatonin (hormone) and phototherapy ("bright- light therapy"). Design examples are 2 factor designs: 2x3 Note: Designs are the same in number of data points, but vary in number of subjects. Factor a: phototherapy Factor b: melatonin a1: no phototherapy b1: no melatonin a2: phototherapy b2: 1 mg melatonin b3: 3 mg melatonin Type 1: Within subject design (Repeated measures design) a1 a1 a1 a2 a2 a2 b1 b2 b3 b1 b2 b3 s1 1 2 3 4 5 6 s2 7 8 9 10 11 12 s3 13 14 15 16 17 18 Type 2: Between subject design (Completely randomized design) a1 a1 a1 a2 a2 a2 b1 b2 b3 b1 b2 b3 s1 1 s4 4 s7 7 s10 10 s13 13 s16 16 s2 2 s5 5 s8 8 s11 11 s14 14 s17 17 s3 3 s6 6 s9 9 s12 12 s15 15 s18 18 Type 3: Mixed design (2x3) option 1 (factor a between, factor b within) a1 a1 a1 a2 a2 a2 b1 b2 b3 b1 b2 b3 s1 1 2 3 s4 10 11 12 s2 4 5 6 s5 13 14 15 s3 7 8 9 s6 16 17 18 Type 3: Mixed design (2x3) option 2 (factor a within, factor b between) b1 b1 b2 b2 b3 b3 a1 a2 a1 a2 a1 a2 s1 1 2 s4 7 8 s7 13 14 s2 3 4 s5 9 10 s8 15 16 s3 5 6 s6 11 12 s9 17 18 Nested designs In nested designs, the levels of a factor A found under different levels of a factor B are NOT THE SAME. a1 a1 a1 a2 a2 a2 b1 b2 b3 b4 b5 b6 s1 1 2 3 s4 10 11 12 s2 4 5 6 s5 13 14 15 s3 7 8 9 s6 16 17 18 Examples of nested designs Spatial: Multiple samples of a single tissue type within a rat Estuaries are unique to each river Temporal Sub-samples in time can only be sampled at one time and not another Summary Design De nition Observation Requires the least number of subjects. Within All subjects go through all the For equal number of subjects, higher subject conditions (6/6). power than “between”. Speci c subjects go through Requires the most number of subjects. Between only one treatment condition For equal number of subjects, lower subject (1/6). power than “within”. Any given subject receives only 2 (option 2) or 3 (option 1) of Requires a moderate amount of Mixed the 6 treatment conditions (2/6 subjects. (split- or 3/6) depending on which For a x number of subjects, moderate plot) factor is within and which power compared to the previous two. factor is between. More economical but some Nested See previous slide. interactions cannot be evaluated. fi fi fi Interactions Interaction between variables Interactions between variables/factors = interconnectivity Variables (factors): x, y, z Main effects: x, y, z Interactions: xy, xz, yz, xyz You have an interaction when the effect of two or more variables is not simply additive (see next slide) Interactions make the interpretation of experimental data more challenging: A signi cant interaction will often mask the signi cance of main effects fi fi Main effects and interactions Phototherapy (PG) No phototherapy (NPG) Main effects 10 8 Combined effects: 6 4 Additive 2 0 Interactive 0 1 3 10 Design: 8 6 P Group NP Group 4 0 mg 1 mg 3 mg 0 mg 1 mg 3 mg 2 Counterbalanced Counterbalanced 0 0 1 3 Interaction between variables Interactions between variables: There is an interaction between two variables if the effect of one independent variable changes with different values of the second independent variable. The sleep study with melatonin and phototherapy treatments is a good example of a factorial design with two independent variables (factors) potentially interacting. Example: Melatonin and phototherapy to treat DSPS (delayed sleep phase syndrome) The treatments The participants of this study are suffering from delayed sleep phase syndrome (DSPS) and tend to naturally go to sleep late (e.g., 3 a.m.) and wake-up late as well (e.g., noon). It is not insomnia (they usually sleep the average 8 hours/night). The (full spectrum) light treatment is given in the morning to help them wake-up "early" (e.g., 7 a.m.) by suppressing melatonin levels The melatonin treatment is given at night, half an hour before the ideal bed time (e.g., 11 p.m.). Examples: general information The ctitious examples given below will now assume that a completely randomized factorial design was chosen; this means: Different participants are in each treatment condition. Each group of participants is independent of every other group. Summary: completely randomized 2x3 factorial design. fi Questions Main effects? Main effect of melatonin? Main effect of phototherapy? Interaction? Fictitious data 1a: No interaction In this example, we notice that in the Phototherapy (PG) chosen dependent variable, participants No phototherapy (NPG) have higher scores of sleep quality in 10 the phototherapy group (PG) than the no 8 Depth of sleep scale phototherapy group (NPG). 6 Dosages of melatonin seem to also increase the quality of sleep. 3 mg of 4 melatonin (M3), better than 1 mg (M1), 2 better than 0 mg (M0). The lines being perfectly parallel, we 0 0 1 3 can conclude that there is no Dosage of melatonin interaction. Fictitious data 1b: No interaction In this example, we notice that in the Phototherapy (PG) chosen dependent variable, No phototherapy (NPG) 10 participants have higher scores of sleep quality in the phototherapy group (PG) 8 Depth of sleep scale than the no phototherapy group (NPG). 6 Dosages of melatonin don’t seem to 4 increase the quality of sleep in either of the two phototherapy conditions. 2 The lines being perfectly parallel, we 0 can conclude that there is no 0 1 3 interaction. Dosage of melatonin Fictitious data 1c: No interaction In this example, we notice that in the Phototherapy (PG) chosen dependent variable, participants No phototherapy (NPG) have the same scores of sleep quality in 10 both the phototherapy group (PG) and the no phototherapy group (NPG). 8 Depth of sleep scale Dosages of melatonin seem to increase 6 the quality of sleep. 3 mg of melatonin 4 (M3), better than 1 mg (M1), better than 0 mg (M0). 2 The lines being perfectly parallel, we 0 can conclude that there is no 0 1 3 interaction. Dosage of melatonin Fictitious data 2: Mild interaction In this example, we notice the same Phototherapy (PG) trend for the phototherapy treatment as No phototherapy (NPG) in the previous example. 10 Dosages of melatonin seem to also 8 Depth of sleep scale increase the quality of sleep, although, 6 in this case, for participants with no phototherapy, there is no difference 4 between M0 and M1. 2 The lines not being perfectly parallel suggest a mild interaction. What is 0 0 1 3 uncertain in this case, is if the Dosage of melatonin interaction will be signi cant or not. fi Fictitious data 3: Strong interaction In this example, the phototherapy treatment Phototherapy (PG) No phototherapy (NPG) seems to help tremendously participants 10 getting 1 mg of melatonin at bed time. The language of the previous paragraph 8 Depth of sleep scale clearly identi es the impact of one factor 6 over the other other factor. In this case, the (statistical) signi cance of the interaction is 4 dif cult to doubt. 2 Factorial analysis of variance (factorial ANOVA's) will determine the signi cance 0 of each treatment as well as the 0 1 3 signi cance of the interaction. Dosage of melatonin fi fi fi fi fi Fictitious data 4: The problem of interpretation. The main issue with factorial designs, is Phototherapy (PG) that interpretation of the interactions No phototherapy (NPG) may be a challenge. Note that here you 10 are dealing with only two independent 8 variables, with relatively few levels Depth of sleep scale each. 6 This is why many authors will suggest to 4 limit yourself to three or four independent variables or factors. 2 Be parsimonious in your choice of 0 0 1 3 design. The interpretation of the data is more likely to be straightforward. Dosage of melatonin Fictitious data 5: Complex designs invite complex interactions. Phototherapy: 10000 lux The simple addition of levels for each factor Phototherapy: 5000 lux is contributing in this case to chaotic data. No phototherapy: 0 lux Good luck in the interpretation of this data 10 set! 8 Depth of sleep scale When you design an experiment, do not 6 forget that you will have to explain the data: the signi cant differences between 4 each treatment or factor and the signi cant interactions! 2 Now imagine adding factors (such as 0 "mattress rmness" and/or "presence of pink 0 1 3 5 10 noise" in the bedroom, etc. Dosage of melatonin fi fi fi Fictitious data 6: Interactions and main effects Phototherapy (PG) Phototherapy (PG) No phototherapy (NPG) No phototherapy (NPG) 10 10 8 8 Depth of sleep scale Depth of sleep scale 6 6 4 4 2 2 0 0 0 1 3 0 1 3 Dosage of melatonin Dosage of melatonin Main effect of phototherapy Main effect of phototherapy No main effect of melatonin Main effect of melatonin No interaction No interaction Fictitious data 7: Interactions and main effects Phototherapy (PG) Phototherapy (PG) No phototherapy (NPG) No phototherapy (NPG) 10 10 8 8 Depth of sleep scale Depth of sleep scale 6 6 4 4 2 2 0 0 0 1 3 0 1 3 Dosage of melatonin Dosage of melatonin No main effect of phototherapy Main effect of phototherapy Main effect of melatonin Main effect of melatonin No interaction Interaction Fictitious data 8: Types of interactions Phototherapy (PG) Phototherapy (PG) No phototherapy (NPG) No phototherapy (NPG) 10 10 8 8 Depth of sleep scale Depth of sleep scale 6 6 4 4 2 2 0 0 0 1 3 0 1 3 Dosage of melatonin Dosage of melatonin Main effect of phototherapy Main effect of phototherapy Main effect of melatonin Main effect of melatonin Ordinal interaction Disordinal interaction Fictitious data 9: Antagonistic interactions Certain kinds of interactions can Phototherapy (PG) cancel out the main effects. No phototherapy (NPG) The independent variable melatonin is 10 effective, but the statistical analysis fails 8 to reveal statistically signi cant main Depth of sleep scale effects for the melatonin factor. 6 The dotted line above represents the main effect of melatonin. 4 2 10 8 0 0 3 6 Dosage of melatonin 4 2 Signi cant interaction 0 No main effects 0 3 Main effects masked by the interaction fi fi Fictitious data 10: Antagonistic interactions Phototherapy (PG) Similar situation: You have a signi cant No phototherapy (NPG) interaction with equal means, in other words, 10 an interaction without main effects. 8 This example justi es why IT IS justi able to Depth of sleep scale report signi cant main effects even in the 6 presence of an interaction effect. If you nd an interaction, investigate the main effects. 4 The graph could be translated into this one: 2 10 0 8 0 1 3 10 6 Dosage of melatonin 4 2 Signi cant interaction 0 No main effects 0 1 3 10 Disordinal interaction fi fi fi fi fi fi Caution: Factors and levels Limit the number of levels for each factor: Example: 3 x 12 Limit the number of factors: Example: 2 x 3 x 4 x 6 x 4 This limits the number of potential interactions (as they may be hard to explain). Possible main effects and interactions in 2, 3 and 4-way factorial designs ⎡higher-order factorial designs⎤ Two-way Three-way Four-way Main effects A, B A, B, C A, B, C, D Two-way AxB, BxC, CxD, AxB AxB, BxC, AxC interactions AxC, AxD, BxD Three-way AxBxC, BxCxD, - AxBxC interactions AxBxD, AxCxD Four-way - - AxBxCxD interactions