Psychology 8th Edition - Gleitman, Gross, Reisberg - Mental Representations
Document Details
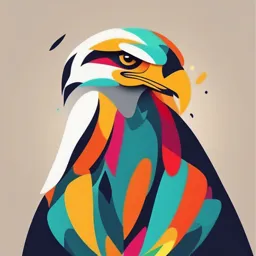
Uploaded by MomentousSaxhorn
Tags
Summary
This is a chapter from a psychology textbook discussing mental representations. It explores different types of mental representations, including images and symbols. The chapter also covers decision making and other cognitive processes and mental operations.
Full Transcript
Mental Representations 342 Decision Making: Choosing Among Options 358 Judgment: Drawing Conclusions from Problem Solving: Finding a Path Toward a Goal 365 Experience 348 Some Final Thoughts: Better Thinking 375 Reasoning: Drawin...
Mental Representations 342 Decision Making: Choosing Among Options 358 Judgment: Drawing Conclusions from Problem Solving: Finding a Path Toward a Goal 365 Experience 348 Some Final Thoughts: Better Thinking 375 Reasoning: Drawing Implications from Our Beliefs 354 Summary 376 9 CHAPTER Thinking P hoebe Ellsworth was facing a difficult decision. As a young professor at Yale University, she had received a tempting job offer from the University of Michigan. Should she stay or should she go? Following the advice of many experts in decision making, she took two large sheets of paper—one for each university—and listed their positives and negatives, assigned numbers to each item according to how important it was to her, and then added up those numbers. But, with the numbers neatly summed, Ellsworth discov- ered she wasn’t content with the result. “It’s not coming out right!” she exclaimed to fellow psychologist Robert Zajonc. In the end, she went with her gut and made her decision based primarily on her feelings about the choice and not on her calculations. The decision has worked out well for her, and, three decades later, she’s a distinguished professor of law and psy- chology at Michigan—where she studies, among many things, how emotions sway people’s decisions about such crucial matters as murder trials. Meanwhile, Zajonc continued to research the interplay between feeling and thinking, and ended up arguing that cases like Ellsworth’s are relatively common, so that, ironically, people rarely use only their minds to “make up their minds.” Zajonc’s claim suggests that people are less “rational” than we believe we are—even when we’re trying to be thoughtful and careful, and even when we’re thinking about highly consequential issues. And, in fact, many other lines of evidence—and many other psychologists—raise their own questions about human rationality. For example, 341 studies suggest that we tend to pay special attention to information that confirms our hunches and hopes and ignore (or overrule) evidence that might challenge our beliefs. We flip-flop in our preferences—even when making important decisions—influenced by trivial changes in how our options are described. We rely on reasoning “shortcuts,” even when drawing life-altering conclusions, apparently making our conclusions using strategies that are efficient but prone to error. And we’re easily persuaded by “man who” stories—“What do you mean cigarettes cause lung cancer? I know a man who smokes two packs a day, and he’s perfectly healthy”—even though, with a moment’s reflection, we see the illogic in this. What should we make of these findings? Is it possible that humans—even smart, well-educated humans, doing their best to think carefully and well—are, in truth, often irrational? And if so, what does this imply about us? Are our heads filled with false beliefs—about ourselves, our friends, and our world? Will we make decisions that fail to bring us happiness? We’ll tackle all these questions in this chapter, asking how people think, and also how well. We’ll focus first on the content of thought and the ways that different types of ideas are represented in the mind. We’ll then turn to the processes of thought, with our main focus on what psychologists call directed thinking—the mental activities we use to achieve goals. Specifically, we’ll look at the processes used in interpreting information, judging the truth of an assertion, solving problems, and weighing the costs and benefits of a decision. MENTAL REPRESENTATIONS Common sense tells us that we’re able to think about objects or events not currently in view. Thus, you can decide whether you want the chocolate ice cream or the strawberry before either is delivered. Likewise, you can draw conclusions about George by remem- bering how he acted at the party, even though the party was two weeks ago and a hun- dred miles away. In these (and many other cases), our thoughts must involve mental mental representations Contents in representations—contents in the mind that stand for some object or event or state of the mind that stand for some object, affairs, allowing us to think about those objects or events even in their absence. event, or state of affairs. Mental representations can also stand for objects or events that exist only in our minds—including fantasy objects, like unicorns or Hogwarts School, or impossible objects, like the precise value of pi. And even when we’re thinking about objects in plain view, mental representations still have a role to play. If you’re thinking about this page, for example, you might represent it for yourself in many ways: “a page from the Thinking chapter,” or “a piece of paper,” or “something colored white,” and so on. These different ideas all refer to the same physical object—but it’s the ideas (the men- tal representations), not the physical object, that matter for thought. (Imagine, for example, that you were hunting for something to start a fire with; for that, it might be helpful to think of this page as a piece of paper rather than a carrier of information.) Mental representations of all sorts provide the content for our thoughts. Said differ- ently, what we call “thinking” is just the set of operations we apply to our mental rep- resentations—analyzing them, contemplating them, and comparing them in order to draw conclusions, solve problems, and more. However, the nature of the operations applied to our mental operations varies—in part because mental representations come in different forms, and each form requires its own type of operations. 342 chapter 9 PTHINKING O Distinguishing Images and Symbols Some of our mental representations are analogical—they capture some of the actual analogical representation An idea characteristics of (and so are analogous to) what they represent. Analogical representa- that shares some of the actual charac- tions usually take the form of mental images. In contrast, other representations are teristics of the object it represents. symbolic and don’t in any way resemble the item they stand for. mental images Mental representations To illustrate the difference between images and symbols, consider a drawing of a cat that resemble the objects they represent (Figure 9.1). The picture consists of marks on paper, but the actual cat is flesh and by directly reflecting the perceptual blood. Clearly, therefore, the picture is not equivalent to a cat; it’s merely a representa- qualities of the thing represented. tion of one. Even so, the picture has many similarities to the creature it represents, so symbolic representation A mental that, in general, the picture looks in some ways like a cat: The cat’s eyes are side by side representation that stands for some con- in reality, and they’re side by side in the picture; the cat’s ears and tail are at opposite tent without sharing any characteristics ends of the creature, and they’re at opposite ends in the picture. It’s properties like these with the thing it represents. that make the picture a type of analogical representation. In contrast, consider the word cat. Unlike a picture, the word in no way resembles the cat. The letter c doesn’t represent the left-hand edge of the cat, nor does the overall shape of the word in any way indicate the shape of this feline. The (A) Analogical representation (B) Symbolic representation word, therefore, is an entirely abstract representation, and the rela- tion between the three letters c-a-t and the animal they represent is essentially arbitrary. For some thoughts, mental images seem crucial. (Try thinking about a particular shade of blue, or try to recall whether a horse’s ears are rounded at the top or slightly pointed. The odds are good that these thoughts will call mental images to mind.) For other thoughts, you probably need a symbolic representation. (Think about the causes of cat global warming;this thought may call images to mind—perhaps smoke pouring out of a car’s exhaust pipes—but it’s likely that your thought specifies relationships and issues not captured in the images at all.) For still other thoughts, it’s largely up to you how to represent the thought, and the form of representation is often consequential. If you form a mental 9.1 Analogical vs. symbolic representa- image of a cat, for example, you may be reminded of other animals that look like the tions (A) Analogical representations have cat—and so you may find yourself thinking about lions or tigers. If you think about cats certain things in common with the thing without a mental image, this may call a different set of ideas to mind—perhaps thoughts they represent. Thus, the left side of the about other types of pet. In this way, the type of representation can shape the flow of drawing shows the left side of the cat; the right side of the drawing shows the cat’s your thoughts—and thus can influence your judgments, your decisions, and more. right side. (B) There’s no such correspon- dence for a symbolic representation: The letter c doesn’t indicate the left side of Mental Images the cat! People often refer to their mental images as “mental pictures” and comment that they inspect these “pictures” with the “mind’s eye.” In fact, references to a mind’s eye have been part of our language at least since the days of Shakespeare, who used the phrase in Act 1 of Hamlet. But, of course, there is no (literal) mind’s eye—no tiny eye somewhere inside the brain. Likewise, mental pictures cannot be actual pictures: With no eye deep inside the brain, who or what would inspect such pictures? Why, then, do people describe their images as mental pictures? This usage presum- ably reflects the fact that images resemble pictures in some ways, but that simply invites the next question: What is this resemblance? A key part of the answer involves spatial layout. In a classic study, research participants were first shown the map of a fictitious island containing various objects: a hut, a well, a tree, and so on (Kosslyn, Ball, & Reisser, 1978; Figure 9.2). After memorizing this map, the participants were asked to PMental RepresentationsO 343 9.2 SCIENTIFIC METHOD: Do mental images represent spatial relationships the way pictures do? Method Results 1. Participants were shown a The time needed for the speck to “travel” map of a fictitious island between two points on the mental image was containing various landmarks. proportional to the distance between those points on the map. 2. After memorizing this map the 2.1 participants were asked to form a mental image of the island. 1.9 Response time (sec) 3. Participants were timed while 1.7 they imagined a black speck zipping from one landmark on 1.5 the island to another. When the speck “reached” the target, the 1.3 participant pressed a button, 1.1 stopping a clock. 0 2 4 6 8 10 12 14 16 18 Distance (centimeters) CONCLUSION: Mental images accurately represent the spatial relationships inside a scene. SOURCE STUDY: Kosslyn, Ball, & Reisser, 1978 form a mental image of the island. The experimenters then named two objects on the map (e.g., the hut and the tree), and participants had to imagine a black speck zipping from the first location to the second; when the speck “reached” the target, the partici- pant pressed a button, stopping a clock. Then the experimenters did the same for another pair of objects—say the tree and the well—and so on for all the various pairs of objects on the island. The results showed that the time needed for the speck to “travel” across the image was directly proportional to the distance between the two points on the original map. Thus, participants needed little time to scan from the pond to the tree; scanning from the pond to the hut (roughly four times the distance) took roughly four times as long; scanning from the hut to the patch of grass took even longer. Apparently, then, the image accurately depicted the map’s geometric arrangement: Points close together on the map were somehow close to each other in the image; points farther apart on the map were more distant in the image. In this way, the image is unmistakably picture-like, even if it’s not literally a picture. Related evidence indicates enormous overlap between the brain areas crucial for cre- ating and examining mental images and the brain areas crucial for visual perception. Specifically, neuroimaging studies show that many of the same brain structures (prima- rily in the occipital lobe) are active during both visual perception and visual imagery (Figure 9.3). In fact, the parallels between these two activities are quite precise: When people imagine movement patterns, high levels of activation are observed in brain areas that are sensitive to motion in ordinary perception. Likewise, for very detailed images, the brain areas that are especially activated tend to be those crucial for perceiving fine detail in a stimulus (Behrmann, 2000; Thompson & Kosslyn, 2000). 344 chapter 9 PTHINKING O (A) Brain activity while viewing simple pictures (B) Brain activity while thinking of mental pictures 9.3 Brain activity during mental imagery These fMRI images show different “slices” through the living brain, revealing levels of activity in different brain sites. More active regions are shown in yellow, orange, and red. (A) The first row shows brain activity while a person is making judg- ments about simple pictures. (B) The second row shows brain activity while the person is mak- ing the same sorts of judgments about “mental pictures,” visualized before the “mind’s eye.” Further evidence comes from studies using transcranial magnetic stimulation (TMS; see Chapter 3). Using this technique, researchers have produced temporary disruptions in the visual cortex of healthy volunteers—and, as expected, this causes problems in seeing. What’s important here is that this procedure also causes parallel problems in visual imagery—consistent with the idea that this brain region is crucial both for the processing of visual inputs and for the creation and inspection of images (Kosslyn, Pascual-Leone, Felician, Camposano, Keenan et al., 1999). All of these results powerfully confirm that visual images are indeed picture-like; and they lend credence to the often-heard report that people can “think in pictures.” Be aware, though, that visual images are picture-like, but not pictures. In one study, partic- ipants were shown the drawing in Figure 9.4 and asked to memorize it (Chambers & Reisberg, 1985; also Reisberg & Heuer, 2005). The figure was then removed, and partic- ipants were asked to form a mental image of this now absent figure and to describe their image. Some participants reported that they could vividly see a duck facing to the left; others reported seeing a rabbit facing to the right. The participants were then told there was another way to perceive the figure and asked if they could reinterpret the 9.4 Images are not pictures The duck/ image, just as they had reinterpreted a series of practice figures a few moments earlier. rabbit figure, first used in 1900 by Joseph Given this task, not one of the participants was able to reinterpret the form. Even with Jastrow. The picture of this form is easily hints and considerable coaxing, none were able to find a duck in a “rabbit image” or a reinterpreted; the corresponding mental rabbit in a “duck image.” The participants were then given a piece of paper and asked to image, however, is not. draw the figure they had just been imagining; every participant was now able to come up with the perceptual alternative. These findings make it clear that a visual image is different from a picture. The pic- ture of the duck/rabbit is easily reinterpreted; the corresponding image is not. This is because the image is already organized and interpreted to some extent (e.g., facing “to the left” or “to the right”), and this interpretation shapes what the imaged form seems to resemble and what the imaged form will call to mind. PMental RepresentationsO 345 proposition A statement relating a Propositions subject and a claim about that subject. As we’ve seen, mental images—and analogical representations in general—are essen- node In network-based models of men- tial for representing some types of information. Other information, in contrast, tal representation, a “meeting place” for requires a symbolic representation. This type of mental representation is more flexible the various connections associated with because symbols can represent any content we choose, thanks to the fact that it’s a particular topic. entirely up to us what each symbol stands for. Thus, we can use the word mole to stand for an animal that digs in the ground, or we could use the word (as Spanish speakers associative links In network-based do) to refer to a type of sauce used in cooking. Likewise, we can use the word cat to refer models of mental representation, con- nections between the symbols to your pet, Snowflake; but, if we wished, we could instead use the Romanian word (or nodes) in the network. pisică as the symbol representing your pet, or we could use the arbitrary designation X2$. (Of course, for communicating with others, it’s important that we use the same spreading activation The process terms they do. This is not an issue, however, when we’re representing thoughts in our through which activity in one node in a own minds.) network flows outward to other nodes through associative links. Crucially, symbols can also be combined with each other to represent more complex contents—such as “San Diego is in California,” or “cigarette smoking is bad for your health.” There is debate about the exact nature of these combinations, but many schol- ars propose that symbols can be assembled into propositions—statements that relate a subject (the item about which the statement is being made) and a predicate (what’s being asserted about the subject). For example, “Solomon loves to blow glass,” “Jacob lived in Poland,” and “Squirrels eat burritos” are all propositions (although the first two are true, and the last is false). But just the word Susan or the phrase “is squeamish” aren’t propositions—the first is a subject without a predicate; the second is a predicate without a subject. (For more on how propositions are structured and the role they play in our thoughts, see J. Anderson, 1993, 1996.) It’s easy to express propositions as sentences, but this is just a convenience; many 9.5 Associative connections Many inves- other formats are possible. In the mind, propositions are probably expressed via net- tigators propose that our knowledge is rep- work structures, related to the network models we discussed for perception in Chapter 5. resented through a network of associated Individual symbols serve as nodes within the network—meeting places for various ideas, so that the idea of “Abe Lincoln” is links—so if we were to draw a picture of the network, the nodes would look like knots linked to “Civil War” and “President.” in a fisherman’s net, and this is the origin of the term node (derived from the Latin nodus, meaning “knot”). The individual nodes are connected to each other by associative links (Figure 9.5). Thus, in this system there might be a node representing Abe Lincoln and another node repre- senting President, and the link between them represents part of our U.S. knowledge about Lincoln—namely, that he was a president. Other Confederacy penny links have labels on them, as shown in Figure 9.6; these labels allow us to specify other relationships among nodes, and in this Civil way we can use the network to express any proposition at all (after War J. Anderson, 1993, 1996). Slavery The various nodes representing a proposition are activated when- ever a person is thinking about that proposition. This activation Abe Gettysburg then spreads to neighboring nodes, through the associative links, Lincoln Address much as electric current spreads through a network of wires. However, this spread of activation will be weaker (and will occur President more slowly) between nodes that are only weakly associated. The Pennsylvania spreading activation will also dissipate as it spreads outward, so Barack that little or no activation will reach the nodes more distant from the Obama activation’s source. In fact, we can follow the spread of activation directly. In a classic study, participants were presented with two strings of letters, like 346 chapter 9 PTHINKING O 9.6 Propositions One proposal is that CHEW your understanding of dogs—what they are, what they’re likely to do—is repre- sented by an interconnected network of propositions. In this figure, each proposi- Relation tion is represented by a white circle, which serves as the meeting place for the ele- Agent Object DOGS BONES ments included in the proposition. Thus, this bit of memory network contains the propositions “dogs chew bones,” “dogs Agent chase cats,” and so on. A complete repre- Agent Subject sentation about your knowledge of dogs would include many other propositions as Relation Relation CHASE Part of well. Object Relation Object Object CATS EAT MEAT NARDE–DOCTOR, or GARDEN–DOCTOR, or NURSE–DOCTOR (Meyer & Schvaneveldt, 1971). The participants’ job was to press a “yes” button if both sequences were real words (as in the second and third examples here),and a “no” button if either was not a word (the first example). Our interest here is only in the two pairs that required a yes response. (In these tasks, the no items serve only as catch trials, ensuring that partici- pants really are doing the task as they were instructed.) Let’s consider a trial in which participants see a related pair, like NURSE– DOCTOR. In choosing a response, they first need to confirm that, yes, NURSE is a real word in English. To do this, they presumably need to locate the word NURSE in their mental dictionary; once they find it, they can be sure that these letters do form a legitimate word. What this means, though, is that they will have searched for, and activated, the node in memory that represents this word—and this, we have hypothe- sized, will trigger a spread of activation outward from the node, bringing activation to other, nearby nodes. These nearby nodes will surely include the node for DOCTOR, since there’s a strong association between “nurse” and “doctor.” Therefore, once the node for NURSE is activated, some activation should also spread to the node for DOCTOR. Once they’ve dealt with NURSE, the participants can turn their attention to the sec- ond word in the pair. To make a decision about DOCTOR (is this string a word or not?), the participants must locate the node for this word in memory. If they find the relevant node, then they know that this string, too, is a word and can hit the “yes” button. But of course the process of activating the node for DOCTOR has already begun, thanks to the activation this node just received from the node for NURSE. This should accelerate the process of bringing the DOCTOR node to threshold (since it’s already partway there), and so it will take less time to activate. Hence, we expect quicker responses to DOCTOR in this context, compared to a context in which it was preceded by some unrelated word and therefore not primed. This prediction is correct. Participants’ lexi- cal decision responses are faster by almost 100 milliseconds if the stimulus words are related, so that the first word can prime the second in the way we just described. We’ve described this sequence of events within a relatively uninteresting task— participants merely deciding whether letter strings are words in English or not. But the PMental RepresentationsO 347 same dynamic—with one node priming other, nearby nodes—plays a role in, and can shape, the flow of our thoughts. For example, we mentioned in Chapter 6 that the sequence of ideas in a dream is shaped by which nodes are primed. Likewise, in problem solving, we sometimes have to hunt through memory, looking for ideas about how to tackle the problem we’re confronting. In this process, we’re plainly guided by the pattern of which nodes are activated (and so more available) and which nodes aren’t. This pat- tern of activation in turn depends on how the nodes are connected to each other—and so the arrangement of our knowledge within long-term memory can have a powerful impact on whether we’ll locate a problem’s solution. JUD G MENT: DRAWING CONCLUSIONS FROM EXPERIENCE So far we’ve been discussing the content of thought, with an emphasis on how thoughts are represented in the mind. Just as important, though, are the processes of thought—what psychologists call directed thinking—the ways people draw conclu- directed thinking Thinking aimed at a sions or make decisions. What’s more, these two broad topics—the contents of thought particular goal. and the processes—are linked in important ways. As we’ve discussed, representing ideas with images will highlight visual appearance in our thoughts and thus may call to judgment The process of extrapolating from evidence to draw conclusions. mind objects with similar appearance. Likewise, representing ideas as propositions will cause activation to spread to other, associated, nodes; and this too can guide our heuristics A strategy for making judg- thoughts in one direction rather than another. ments quickly, at the price of occasional mistakes. But, of course, the flow of our thoughts also depends on what we’re trying to accom- plish in our thinking.So it will be useful to divide our discussion of thought processes into four sections, each corresponding to a type of goal in our thinking: We will, therefore, con- sider judgment, reasoning, decision making, and problem solving. Let’s begin with judgment. The term judgment refers to the various steps we use when trying to reach beyond the evidence we’ve encountered so far, and to draw conclusions from that evidence. Judgment, 9.7 Daniel Kahneman and Amos Tversky by its nature, involves some degree of extrapolation because we’re going beyond the evi- Much of what we know about judgment dence; and as such, this always involves some risk that the extrapolation will be mistaken. and decision making comes from pioneer- If, for example, we know that Jane has enjoyed many trips to the beach, we might draw the ing work by Daniel Kahneman (A) and conclusion that she will always enjoy such trips. But there’s no guarantee here, and it’s Amos Tversky (B); their work led to surely possible that her view of the beach might change. Likewise, if you have, in the past, Kahneman receiving the Nobel Prize in preferred spending time with quiet people, you might draw a conclusion about how much 2002. you’d enjoy an evening with Sid, who’s quite loud. But here, too, there’s no guarantee—and perhaps you’ll have a great time with Sid. Even with these risks, we routinely rely on judgment to reach beyond the evidence we’ve gathered so far—and so we do make fore- casts about the next beach trip, whether the evening with Sid would be fun, and more. But how do we proceed in making these judgments? Research suggests that we often rely on a small set of shortcuts called judgment heuristics. The word heuristics, borrowed from computer sci- ence, refers to a strategy that’s relatively efficient but occasionally leads to error. Heuristics, in other words, offer a trade-off between efficiency and accuracy, helping us to make judgments more quickly—but at the price of occasional mistakes. Let’s start our discussion with two of these shortcuts—the avail- ability and representativeness heuristics, first described by Amos Tversky (A) (B) and Daniel Kahneman (Figure 9.7); their research in this domain is 348 chapter 9 PTHINKING O part of the scholarship that led to Kahneman’s winning the Nobel Prize in 2002.* As we’ll see, these heuristics are effective but often do lead to errors, and so we’ll turn next to the question of whether—and in what circumstances—people can rise above the shortcuts, and use more accurate judgment strategies. The Availability Heuristic In almost all cases, we want our conclusions to rest not just on one observation, but on patterns of observations. Is last-minute cramming an effective way to prepare for exams? Does your car start more easily if you pump the gas while turning the key? Do you get sick less often if you take vitamin tablets? In each case, you could reach a conclusion based on just one experience (one exam, or one flu season), but that’s a risky strategy because that experience might have been a fluke of some sort, or in some way atypical. Thus, what you really want is a summary of multiple experiences, so that you can draw conclusions only if there’s a consistent pattern in the evidence. Generally, this summary of multiple experiences will require a comparison among frequency estimates—assessments of how often you’ve encountered a particular event or object. How often have you crammed for an exam and done well? How often have you crammed and done poorly? How many people do you know who take vitamins and still get sick? How many stay healthy? In this way, frequency estimates are central for judgment, but there’s an obvious problem here: Most people don’t keep ledgers recording the events of their lives, and so they have no objective record of what happened each time they started their car or how many of their friends take vitamins. What do people do, then, when they need frequency estimates? They rely on a simple strategy: They try to think of spe- cific cases relevant to their judgment—exams that went well after cramming, or frustrating mornings when the car just wouldn’t start. If these examples come easily to mind, people conclude that the circumstance is a common one; if the examples come to mind slowly or only with great effort, people conclude that the circumstance is rare. This strategy is referred to as the availability heuristic, because the judgment uses availability heuristic A strategy for availability (i.e., how easily the cases come to mind) as the basis for assessing frequency judging how frequently something (how common the cases actually are in the world). For many frequency estimates this happens—or how common it is—based on how easily examples of it come to strategy works well, because objects or events that are broadly frequent in the world are mind. likely to be frequent in our personal experience and are therefore well represented in our memories. On this basis, “easily available from memory” is often a good indicator of “frequent in the world.” But there are surely circumstances in which this strategy is misleading. In one study, participants were asked this question: “Considering all the words in the lan- guage, does R occur more frequently in the first position of the word (rose, robot, rocket) or in the third position (care, strive, tarp)?” Over two-thirds of the participants said that R is more common the first position—but actually the reverse is true, by a wide margin. What caused this error? Participants made this judgment by trying to think of words in which R is the first letter, and these came easily to mind. They next tried to think of words in which it’s the third letter, and these came to mind only with some effort. They then interpreted this difference in ease of retrieval (i.e., the difference in availability) as *Amos Tversky died in 1996 and so could not participate in the Nobel Prize, which is never awarded posthumously. PJudgment: Drawing Conclusions from ExperienceO 349 if it reflected a difference in frequency—and so drew the wrong conclusion. As it turns out, the difference in retrieval merely shows that our mental dictionary, roughly like a printed one, is organized according to the starting sound of each word. This arrange- ment makes it easy to search memory using a word’s “starting letter” as the cue; a search based on a word’s third letter is more difficult. In this fashion, the organization of memory creates a bias in what’s easily available; this bias, in turn, leads to an error in frequency judgment (Tversky & Kahneman, 1973). In this task, it seems sensible for people to use a shortcut (the heuristic) rather than some more laborious strategy, such as counting through the pages in a dictionary. The latter strategy would guarantee the right answer but would surely be far more work than the problem is worth. In addition, the error in this case is harmless—nothing hinges on these assessments of spelling patterns. The problem, though, is that people rely on the same shortcut—using availability to assess frequency—in cases that are more con- sequential. For example, many friendships break up because of concerns over fairness: “Why am I always the one who does the dishes?” Or “Why is it that you’re usually the one who starts our fights, but I’m always the one who reaches out afterward?” These questions hinge on frequency estimates—and use of the availability heuristic routinely leads to errors in these estimates (M. Ross & Siccoly, 1979). As a result, this judgment heuristic may leave us with a distorted perception of some social relations—in a way that can undermine some friendships! As a different example, what are the chances that the stock market will go up tomor- row or that a certain psychiatric patient will commit suicide? The stockbrokers and psy- chiatrists who make these judgments regularly base their decisions on an estimate of probabilities. In the past, has the market generally gone up after a performance like today’s? In the past, have patients with these symptoms generally been dangerous to themselves? These estimates, too, are likely to be based on the availability heuristic. Thus, for example, the psychiatrist’s judgment may be poor if he vividly remembers a particular patient who repeatedly threatened suicide but never harmed himself. This easily available recollection may bias the psychiatrist’s frequency judgment, leading to inadequate precautions in the present case. The Representativeness Heuristic When our judgments hinge on frequency estimates, we rely on the availability heuris- tic. Sometimes, though, our judgments hinge on categorization, and then we turn to a different heuristic (Figure 9.8). For example, think about Marie, who you met at lunch yesterday. Is she likely to be a psychology major? If she is, you can rely on your broader knowledge about psych majors to make some forecasts about her—what sorts of con- versations she’s likely to enjoy, what sorts of books she’s likely to read, and so on. If during lunch you asked Marie what her major is, then you’re all set—able to apply your knowledge about the category to this particular individual. But what if you didn’t ask representativeness heuristic A strat- her about her major? You might still try to guess her major, relying on the representative- egy for judging whether an individual, ness heuristic.This is a strategy of assuming that each member of a category is “represen- object, or event belongs in a certain cat- tative” of the category—or, said differently, a strategy of assuming that each category is egory based on how typical of the cate- gory it seems to be. relatively homogeneous, so that every member of the category resembles every other mem- ber. Thus, if Marie resembled other psych majors you know (in her style of conversation, or the things she wanted to talk about), you’re likely to conclude that she is in fact a psych major—so you can use your knowledge about the major to guide your expectations for her. This strategy—like the availability heuristic—serves us well in many settings, because many of the categories we encounter in our lives are homogeneous in important ways. 350 chapter 9 PTHINKING O Purpose: Judging frequency Purpose: Assessing categories How to use it: Use availability as an How to use it: Use resemblance as indicator of frequency an indicator of category membership (A) Availability heuristic Drawback: Sometimes availability (B) Representative heuristic Drawback: Some categories are isn’t correlated with frequency! heterogeneous. 9.8 Heuristics In the availability heuristic, we use availability to judge frequency. This may be a problem, though, if examples that are in truth relatively rare are nonetheless available to us. Thus, a stockbroker might be misled by an easily accessible memory of an atypical stock! In the representativeness heuristic, we use resemblance to the category ideal as a basis for judging whether a case is in the category or not. But the problem is that some categories are internally diverse and pose the risk that we may be misled by an atypical case—as in the case of a “man who” story (“I know a man who smoked cigarettes and never developed health problems.”) People don’t vary much in the number of fingers or ears we have. Birds uniformly share the property of having wings and beaks, and hotel rooms share the property of having beds and bathrooms. This uniformity may seem trivial, but it plays an enormously important role: It allows us to extrapolate from our experiences, so that we know what to expect the next time we see a bird or enter a hotel room. Even so, evidence suggests that we overuse the representativeness strategy, extrapo- lating from our experiences even when it’s clear we should not. This pattern is evident, for example, whenever someone offers a “man who” or “woman who” argument: “What do you mean, cigarettes cause cancer? I have an aunt who smokes cigarettes, and she’s perfectly healthy at age 82!” Such arguments are often presented in conversations as well as in more formal settings (political debates, or newspaper editorial pages), pre- sumably relying on the listener’s willingness to generalize from a single case. What’s more, these arguments seem to be persuasive: The listener (to continue the example) seems to assume that the category of all cigarette smokers is uniform, so that any one member of the category (including the speaker’s aunt) can be thought of as representa- tive of the entire group. As a result, the listener draws conclusions about the group based on this single case—even though a moment’s reflection might remind us that the case may be atypical, making the conclusions unjustified. In fact, people are willing to extrapolate from a single case even when they’re explic- itly warned that the case is an unusual one. In one study, participants watched a video- taped interview with a prison guard. Some participants were told in advance that the guard was quite atypical, explicitly chosen for the interview because he held such extreme views. Others weren’t given this warning. Then, at the end of the videotape, participants were asked their own views about the prison system, and their responses showed a clear influence from the interview they had just seen. If the interview had shown a harsh, unsympathetic guard, participants were inclined to believe that, in gen- eral, prison guards are severe and inhumane. If the interview showed a compassionate, PJudgment: Drawing Conclusions from ExperienceO 351 caring guard, participants reported more positive views of the prison system. Remarkably, though, participants who had been told clearly that the guard was atypical were just as willing to draw conclusions from the video as the participants given no warning. Essentially, participants’ reliance on the representativeness heuristic made the warning irrelevant (Hamill, Wilson, & Nisbett, 1980; Kahneman & Tversky, 1972, 1973; Nisbett & Ross, 1980). Dual-Process Theories The two shortcuts we’ve discussed—availability and representativeness—generally work well. Things that are common in the world are likely also to be common in our memory, and so readily available for us; availability in memory is therefore often a good indicator of frequency in the world. Likewise, many of the categories we encounter are relatively homo- geneous; a reliance on representativeness, then, often leads us to the correct conclusions. At the same time, these shortcuts can (and sometimes do) lead to errors. What’s worse, we can easily document the errors in consequential domains—medical profes- sionals drawing conclusions about someone’s health, politicians making judgments about international relations, business leaders making judgments about large sums of money. This demands that we ask: Is the use of the shortcuts inevitable? Are we simply stuck with this risk of error in human judgment? The answer to these questions is plainly no, because people often rise above these shortcuts and rely on other, more laborious—but often more accurate—judgment strategies. For example, how many U.S. presidents have been Jewish? Here, you’re unlikely to draw on the availability heuristic (trying to think of relevant cases and bas- ing your answer on how easily these cases come to mind). Instead you’ll swiftly answer, “zero”—based probably on a bit of reasoning. (Your reasoning might be: “If there had been a Jewish president, this would have been notable and often discussed, and so I’d probably remember it. I don’t remember it. Therefore...”) Likewise, we don’t always use the representativeness heuristic—and so we’re often not persuaded by a man-who story. Imagine, for example, that a friend says, “What do you mean there’s no system for winning the lottery? I know a man who tried out his sys- tem last week, and he won!” Surely you’d respond by saying this guy just got lucky— relying on your knowledge about games of chance to overrule the evidence seemingly provided by this single case. Examples like these (and more formal demonstrations of the same points—e.g., Nisbett et al., 1983) make it clear that sometimes we rely on judgment heuristics and dual-process theory The proposal sometimes we don’t. Apparently, therefore, we need a dual-process theory of judgment— that judgment involves two types of one that describes two different types of thinking. The heuristics are, of course, one type thinking: a fast, efficient, but sometimes of thinking; they allow us to make fast, efficient judgments in a wide range of circum- faulty set of strategies, and a slower, more laborious, but less risky set of stances. The other type of thinking is usually slower and takes more effort—but it’s also strategies. less risky and often avoids the errors encouraged by heuristic use. A number of different terms have been proposed for these two types of thinking—intuition versus reasoning System 1 In dual-process models of judgment, the fast, efficient, but (Kahneman, 2003; Kahneman & Tversky, 1996); association-driven thought versus rule- sometimes faulty type of thinking. driven thought (Sloman, 1996); a peripheral route to conclusions versus a central route (Petty & Cacioppo,1985);intuition versus deliberation (Kuo et al.,2009),and so on.Each System 2 In dual-process models of judgment, the slower, more effortful, of these terms carries its own suggestion about how these two types of thought should be and more accurate type of reasoning. conceptualized, and theorists still disagree about this conceptualization. Therefore, many prefer the more neutral (but less transparent!) terms proposed by Stanovich and West (2000), who use System 1 as the label for the fast, automatic type of thinking and System 2 as the label for the slower, more effortful type (Figure 9.9). 352 chapter 9 PTHINKING O We might hope that people use System 1 for unimportant judg- (A) Brain areas activated by careful deliberation ments and shift to System 2 when the stakes are higher. This would be a desirable state of affairs; but it doesn’t seem to be the case because, as we’ve mentioned, it’s easy to find situations in which people rely on System 1’s shortcuts even when making con- sequential judgments. What does govern the choice between these two types of thinking? The answer has several parts. First, people are—not surprisingly—more likely to rely on the fast and easy strategies of System 1 if they’re tired or pressed for time (e.g., Finucane, Alhakami, Slovic, & Johnson, 2000; D. Gilbert, 1989; Stanovich & West, 1998). Second, they’re much more likely to use System 2’s better quality of thinking if the problem con- (B) Brain areas activated by more intuitive thinking tains certain “triggers.” For example, people are more likely to rely on System 1 if asked to think about probabilities (“If you have this surgery, there’s a.2 chance of side effects”), but they’re more likely to rely on System 2 when thinking about frequencies (“Two out of 10 people who have this surgery expe- rience side effects”). There’s some controversy about why this shift in data format has this effect, but it’s clear that we can improve human judgment simply by presenting the facts in the “right way”—that is, in a format more likely to prompt System 2 thinking (Gigerenzer & Hoffrage, 1995, 1999; C. Lewis & 9.9 Dual-process systems In this study, Keren, 1999; Mellers et al., 2001; Mellers & McGraw, 1999). participants were asked to play either a The use of System 2 also depends on the type of evidence being considered. If, for game that required careful deliberation example, the evidence is easily quantified in some way, this encourages System 2 (System 2), or one that required only rough thinking and makes errors less likely. As one illustration, people tend to be relatively intuitions (System 1). The thought sophisticated in how they think about sporting events. In such cases, each player’s processes involved in these two games performance is easily assessed via the game’s score or a race’s outcome, and each contest involved clearly different patterns of brain is immediately understood as a “sample” that may or may not be a good indicator of a activation. player’s (or team’s) overall quality. In contrast, people are less sophisticated in how they think about a job candidate’s performance in an interview. Here it’s less obvious how to evaluate the candidate’s performance: How should we measure the candidate’s friendli- ness, or her motivation? People also seem not to realize that the 10 minutes of interview can be thought of as just a “sample” of evidence, and that other impressions might come from other samples (e.g., reinterviewing the person on a different day or seeing the person in a different setting; after J. Holland, Holyoak, Nisbett, & Thagard, 1986; Kunda & Nisbett, 1986). Finally, some forms of education make System 2 thinking more likely. For example, training in the elementary principles of statistics seems to make students more alert to the problems of drawing a conclusion from a small sample and also more alert to the possibility of bias within a sample. This is, of course, a powerful argument for educa- tional programs that will ensure some basic numeracy—that is, competence in think- ing about numbers. But it’s not just courses in mathematics that are useful, because the benefits of training can also be derived from courses—such as those in psychology— that provide numerous examples of how sample size and sample bias affect any attempt to draw conclusions from evidence (Fong & Nisbett, 1991; Gigerenzer, Gaissmaier, Kurz-Milcke, Schwartz, & Woloshin, 2007; Lehman, Lempert, & Nisbett, 1988; Lehman & Nisbett, 1990; also see Perkins & Grotzer, 1997). In short, then, our theorizing about judgment will need several parts. We rely on System 1 shortcuts, and these often serve us well—but can lead to error. We also can rise PJudgment: Drawing Conclusions from ExperienceO 353 above the shortcuts and use System 2 thinking instead, and multiple factors govern whether (and when) this happens. Even so, the overall pattern of evidence points toward a relatively optimistic view—that our judgment is often accurate, and that it’s possible to make it more so. REASONING: DRAWING IMPLICATIONS FROM OUR BELIEFS The processes involved in judgment are crucial for us because they allow us to draw new information from our prior experiences.No less important are the processes of reasoning, in which we start with certain beliefs and try to draw out the implications of these beliefs: “If I believe X, what other claims follow from this?” The processes in place here resemble the processes that philosophers call deduction—when someone seeks to derive new asser- tions from assertions already in place. Why is reasoning (or deduction) so important? One reason is that this process allows you to use your knowledge in new ways. For example, you might know that engineers need to be comfortable with math, and you might know that Debby is an engineer. With a trivial bit of reasoning, you now know something about Debby— namely, that she’s comfortable with math. Likewise, you might know that if it’s raining, then today’s picnic will be canceled. If you also know that it’s now raining, some quick reasoning tells you that the picnic is off. These are, of course, simple examples; even so, without the capacity for reasoning, these examples would be incomprehensible for you—making it clear just how impor- tant reasoning is. In addition, reasoning serves another function: It provides a means of testing your beliefs. Let’s say, as an illustration, that you suspect that Alex likes you, but you’re not sure. To check on your suspicion, you might try the following deduction: If he does like you, then he’ll enthusiastically say yes when you ask him out. This pro- vides an obvious way to confirm (or disconfirm) your suspicion. In several ways, then, the skill of reasoning is quite important. We need to ask, there- fore, how well humans do in reasoning. Do we reach sensible, justified conclusions? The answers parallel our comments about judgment: Examples of high-quality reason- ing are easy to find, and so are examples of reasoning errors. We’ll therefore need to explain both of these observations. Confirmation Bias One line of research on reasoning concerns a pattern known as confirmation bias. reasoning The process of figuring out This term applies to several different phenomena but, in general, describes a tendency the implications of particular beliefs. to take evidence that’s consistent with our beliefs more seriously than evidence confirmation bias The tendency to inconsistent with our beliefs. Thus, when they’re trying to test a belief, people often take evidence that’s consistent with your tend to seek out information that would confirm the belief rather than information that beliefs more seriously than evidence might challenge the belief. Likewise, if we give people evidence that’s consistent with inconsistent with your beliefs. their beliefs, they tend to take this evidence at face value and count it as persuasive— and so they strengthen their commitment to their beliefs. But if we give people evidence syllogism A logic problem containing two premises and a conclusion; the syllo- that’s contrary to their beliefs, they often greet it with skepticism, look for flaws, or gism is valid if the conclusion follows ignore it altogether (Figure 9.10). logically from the premises. This pattern is evident in many procedures. In one classic study, participants were presented with a balanced package of evidence concerned with whether capital punish- 354 chapter 9 PTHINKING O ment acts as a deterrent to crime. Half of the evidence favored the partici- pant’s view, and half challenged that view (C. Lord, Ross, & Lepper, 1979). We might hope that this balanced presentation would remind people that there’s evidence on both sides of this issue, and thus reason to take the opposing viewpoint seriously. This reminder in turn should pull people away from extreme positions and toward a more moderate stance. Thanks to confirmation bias, however, the actual outcome was different. The partici- pants found the evidence consistent with their view to be persuasive and the opposing evidence to be flimsy. Of course, this disparity in the evidence was created by the participants’ (biased) interpretation of the facts, and partici- pants with a different starting position perceived the opposite disparity! Even so, participants were impressed by what they perceived as the uneven quality of the evidence, and this led them to shift to views even more extreme than those they’d had at the start. 9.10 Confirmation bias In the Salem Notice the circularity here. Because of their initial bias, participants perceived an witch trials, the investigators believed the asymmetry in the evidence—the evidence offered on one side seemed persuasive; the evidence that fit with their accusations and evidence on the other side seemed weak. The participants then used that asymmetry, discounted (or reinterpreted) the evidence created by their bias, to reinforce and strengthen that same bias. that challenged the accusation. Here we Confirmation bias can also be documented outside the laboratory. Many compul- see Daniel Day-Lewis as John Proctor in a sive gamblers, for example, believe they have a “winning strategy” that will bring them 1996 film version of The Crucible, Arthur great wealth. Their empty wallets provide powerful evidence against this belief, but Miller’s play about the witch trials. they stick with it anyway. How is this possible? In this case, confirmation bias takes the form of influencing how the gamblers think about their past wagers. Of course, they focus on their wins, using those instances to bolster the belief that they have a surefire strategy. What about their past losses? They also consider these, but usually not as losses. Instead, they regard their failed bets as “near wins” (“The team I bet on would have won if not for the ref’s bad call!”) or as chance events (“It was just bad luck that I got a deuce of clubs instead of an ace.”). In this way, confirming evidence is taken at face value, but disconfirming evidence is reinterpreted, leaving the gamblers’ erroneous beliefs intact (Gilovich, 1991; for other examples of confirmation bias, see Schulz-Hardt, Frey, Lüthgens, & Moscovici, 2000; Tweney, Doherty, & Mynatt, 1981; Wason, 1960, 1968). Faulty Logic When people fall prey to confirmation bias, their thinking seems illogical: “If I know All artwork is made of wood. how to pick winners, then I should win my bets. In fact, though, I lose my bets. All wooden things can be turned into clocks. Therefore, I know how to pick winners.” But could this be? Are people really this illog- Therefore all artwork can be turned into clocks. ical in their reasoning? One way to find out is by asking people to solve simple prob- lems in logic—for example, problems involving syllogisms. All artwork is valuable. A syllogism contains two premises and a conclusion, and the question is whether the All valuable things should be cherished. conclusion follows logically from the premises; if it does follow, we say that the conclu- Therefore all artwork should be cherished. sion is valid. Figure 9.11 offers several examples; and let’s be clear that in these (or any) syllogisms, the validity of the conclusion depends only on the premises. It doesn’t mat- All A’s are not B’s. ter if the conclusion is plausible or not, in light of other things you know about the All A’s are G’s. world. It also doesn’t matter if the premises happen to be true or not. All that matters Therefore some G’s are not B’s. is the relationship between the premises and the conclusion—and, in particular, whether the conclusion must be true if the premises are true. 9.11 Categorical syllogisms All of the Syllogisms seem straightforward, and so it’s disheartening that people make an syllogisms shown here are valid—that is, enormous number of errors in evaluating them. To be sure, some syllogisms are easier if the two premises are true, then the than others—and so participants are more accurate, for example, if a syllogism is set in conclusion must be true. PReasoning: Drawing Implications from Our BeliefsO 355 concrete terms rather than abstract symbols. Still, across all the syllogisms, mistakes are frequent, and error rates are sometimes as high as 70 or 80% (Gilhooly, 1988). What produces this high error rate? Despite careful instructions and considerable coaching, many participants seem not to understand what syllogistic reasoning requires. Specifically, they seem not to get the fact that they’re supposed to focus only on the relationship between the premises and conclusion. They focus instead on whether the conclusion seems plausible on its own—and if it is, they judge the syllo- gism to be valid (Klauer, Musch, & Naumer, 2000). Thus, they’re more likely to endorse the conclusion “Therefore all artwork should be cherished” in Figure 9.11 than they are to endorse the conclusion “Therefore all artwork can be turned into clocks.” Both of these conclusions are warranted by their premises, but the first is plausible and so more likely to be accepted as valid. In some ways, this reliance on plausibility is a sensible strategy. Participants are doing their best to assess the syllogisms’ conclusions based on all they know (cf. Evans & Feeney, 2004). At the same time, this strategy implies a profound misunderstanding of the rules of logic (Figure 9.12). With this strategy, people are willing to endorse a bad argument if it happens to lead to conclusions they already believe are true, and they’re 9.12 Deductive reasoning? willing to reject a good argument if it leads to conclusions they already believe are false. Triggers for Good Reasoning We are moving toward an unflattering portrait of human reasoning. In logic, one starts with the premises and asks whether a conclusion follows from these premises. In studies of confirmation bias or syllogistic reasoning, however, people seem to do the opposite: They start with the conclusion, and use that as a basis for evaluating the argument. Thus, they accept syllogisms as valid if the conclusion seems believable on its own, and they count evi- dence as persuasive if it leads to a view they held in the first place. Which card(s) must be turned over to check this rule? We also know, however, that humans are capable of high-quality “If a card has a vowel on one side, reasoning. After all, we do seem able to manage the pragmatic and it must have an even number on the other side.” social demands of our world, and we’re able to make good use of our knowledge. None of this would be possible if we were utterly inept in reasoning; reasoning errors, if they occurred all the time, would trip us up in many ways and lead to a succession of beliefs completely out of line with reality. In addition, impressive skill in reasoning is visible in some for- mal settings. Humans do, after all, sometimes lay out carefully argued positions on political matters and academic questions. 9.13 The selection task The correct Scientists trace through the implications of their theories as they develop new cancer- answer, offered by very few participants, is fighting drugs. And for that matter, mathematicians and logicians rely on deduction as to turn over the A and the 7. If the A (a a way of proving their theorems. vowel) has an odd number on the reverse How should we think about this mixed pattern? Why is our reasoning sometimes side, this would break the rule. If the 7 has a accurate and sometimes filled with errors? Important insights into these questions come vowel on the reverse side, this too would from studies of the selection task. In the standard version of this task, participants are break the rule. No matter what’s on the shown four cards, like those in Figure 9.13. They’re told that these cards may or may not other side of the 6, a vowel or consonant, this would be consistent with the rule. follow a simple rule: “If there is a vowel on one side of the card, there must be an even (After all, the rule didn’t say that only vow- number on the other side.” Their task is to figure out which cards to turn over to deter- els have even numbers on the reverse side.) mine whether the cards do, in fact, follow this rule; they can turn over however many Likewise, no matter what’s on the reverse cards they think are necessary—just one, perhaps; or two, three, or all four. side of the J, this would be consistent with In this task, roughly half the participants make the mistake of turning over the “A” the rule. and the “6” cards. Another 33% make the mistake of turning over just the “A” card. 356 chapter 9 PTHINKING O Only 4% of the participants correctly select the “A” and the “7” Which card(s) must be turned over to check this rule? cards; said differently, fully 96% of the participants get this prob- “If the person is drinking beer, then the person must be over 19 years of age” lem wrong (Wason, 1966, 1968). Performance is much better, though, in other versions of the selection task. In one study, participants were shown the four cards pictured in Figure 9.14 and were told that each card identified the age Drinking Drinking 16 years 22 years of a customer at a bar and what that customer was drinking. Their a beer a Coke of age of age task was to evaluate this rule: “If a person is drinking beer, then the person must be over 19 years of age.” This problem is logically identical to the original selection task, but performance was vastly better—three-fourths of the participants correctly chose the cards 9.14 Variant of the selection task This “drinking a beer” and “16 years old” (Griggs & Cox, 1982). task is formally identical to the standard The contrast between this task and the standard version of the selection task makes selection task, but turns out to be much it clear that the content of the problem matters—and so how well we reason depends on easier. what we are reasoning about. But why is this? One proposal comes from an evolutionary perspective on psychology and begins with the suggestion that our ancient ancestors didn’t have to reason about abstract matters like As and 7s, or vowels and even num- bers. Instead, our ancestors had to worry about issues involving social interactions, including issues of betrayal and cheating: “I asked you to gather firewood; have you done it, or have you betrayed me?” “None of our clan is supposed to eat more than one share of meat; is that guy perhaps cheating and eating too much?” Leda Cosmides and John Tooby have argued that if our ancestors needed to reason about these issues, then individuals who were particularly skilled in this reasoning would have had a survival advantage; and so, little by little, they would have become more numerous within the population, while those without the skill would have died off. In the end, only those skillful at social reasoning would have been left—and we, as their descendants, inherited their skills. This is why, according to Cosmides and Tooby, we perform badly with problems like the “classic” selection task (for which we’re evolutionarily unprepared) but perform well with the drinking-beer problem, since it involves a specific content—cheating—for which we are well prepared (Cosmides, 1989; Cosmides & Tooby, 1992, 2005; Cummins & Allen, 1998; Gigerenzer & Hug, 1992). A different approach emphasizes learning across the life span of the individual, rather than learning across the history of our species. Specifically, Patricia Cheng and Keith Holyoak have argued that, in our everyday lives, we often need to reason about down-to-earth issues that can be cast as “if-then” relationships. One example involves permission, in which we must act according to this rule: “If I want to do X, then I better get permission.” Other examples include obligation and cause-effect rela- tionships: “If I buy him lunch, then he’ll probably lend me his iPod.” Because of this experience, we’ve developed reasoning strategies that apply specifically to these prag- matic issues. In the laboratory, therefore, we’ll reason well if an experimenter gives us a task that triggers one of these strategies—but not otherwise. Thus, for example, the drinking-beer problem involves permission, so it calls up our well-practiced skills in thinking about permission. The same logic problem cast in terms of vowels and even numbers has no obvious connection to everyday reasoning, so it calls up no strategy and leads to poor performance (Cheng & Holyoak, 1986; Cheng, Holyoak, Nisbett, & Oliver, 1985; for a different perspective on the selection task, see Ahn & Graham, 1999). The available evidence doesn’t favor one of these accounts over the other, mostly because the two proposals have a great deal in common. Both proposals, one cast in the light of evolution, one in the light of everyday experience, emphasize that the content of a problem influences our reasoning—and so, as we said earlier, how we reason depends PReasoning: Drawing Implications from Our BeliefsO 357 on the mental representations we’re reasoning about. Likewise, both proposals empha- size pragmatic considerations—the need to reason well about cheaters and betrayal, in one view, or the need to reason about permission or obligation, in the other. Above all, both proposals emphasize the uneven quality of human reasoning. If we encounter a problem of the “right sort,” our reasoning is usually accurate. (We note, though, that even with the drinking-beer problem, some people do make errors!) If, however, we encounter a problem that doesn’t trigger one of our specialized reasoning strategies, then performance is—as we’ve seen—often poor. Judgment and Reasoning: An Overview There are both parallels and contrasts between judgment and reasoning. In both domains, we find uneven performance—sometimes people are capable of wonderfully high-quality thinking, and sometimes they make outrageous errors in their judging or reasoning. We also find,in both judgment and reasoning,that various factors or cues within a problem can trigger better quality thinking (System 2)—so that the way someone thinks depends heav- ily on what they’re thinking about.Thus,when thinking about a Sunday football game,peo- ple are alert to the role of chance and wary of drawing conclusions from a single game. The same people, in thinking about a job interview, might not realize the sample of information is small and so might overinterpret the evidence. Likewise, people’s performance in the selection task is fine if the problem contains cues suggesting a possibility of cheating or a need for permission; the same people perform miserably without these cues. Judgment and reasoning differ, though, in how they proceed in the absence of these triggers. In making judgments, we often rely on System 1 thinking—a set of strategies that generally lead us to sensible conclusions and that are quick and efficient. But there’s no obvious parallel to these strategies in many reasoning tasks—for example, when we’re trying to evaluate an if-then sentence (like the one in the selection task). This situation is reflected in the fact that people don’t make occasional errors with logic problems—instead, we’ve mentioned error rates of 80 and 90%! It’s fortunate, therefore, that our daily experience unfolds in a context in which the triggers we need, leading us into better quality reasoning, are often in place. One last parallel between judgment and reasoning is important and quite encouraging: In both domains, training helps. We’ve mentioned that courses in statistics, and training in the interpretation of data, seem to improve people’s judgment—perhaps by making them more sensitive to the need to gather an ade- quate sample of evidence and by making them more cautious about samples that may be biased. Education also improves people’s ability to reason well—and, again, the education seems to help by making people more alert to cues that might trigger decent reasoning—cues that help people to think about issues (for example) of per- mission or obligation (Lehman & Nisbett, 1990). Thus, we can offer the optimistic conclusion that, with the appropriate training, people can learn to think more care- fully and accurately than they ordinarily do. DECISION M AKING: CHO OSING AMONG OPTIONS Judgment and reasoning allow us to expand our knowledge in important ways—when, for example, we draw some new conclusion from our experiences, or when we deduce a novel claim from our other beliefs. A third type of thinking, in contrast, is more closely tied to our actions. This is the thinking involved in decision making. 358 chapter 9 PTHINKING O We make decisions all the time—some trivial (which brand of toilet paper should you buy?) and some deeply important (should you get that surgery, or not?). Some deci- sions get made over and over (should you go back to that Mexican restaurant one more time?) and some are made just once (should you get a job when you finish school, or seek out some further education?). Researchers have proposed, however, that all of these decisions get made in same way, with the same sort of processes, and so we obvi- ously need to take a close look at what those processes involve. Framing Effects Two factors are obviously crucial for any decision, and these factors are central to utility theory, a conception of decision making endorsed by many economists. According to this theory, you should, first, always consider the possible outcomes of a decision and choose the most desirable one. Would you rather have $10 or $100? Would you rather work for 2 weeks to earn a paycheck or work for 1 week to earn the same pay- check? In each case, it seems obvious that you should choose the option with the great- est benefit ($100) or the lowest cost (working for just 1 week). Second, you should consider the risks. Would you rather buy a lottery ticket with 1 chance in 100 of winning, or a lottery ticket—offered at the same price and with the same prize—with just 1 chance in 1,000 of winning? If one of your friends liked a movie and another didn’t, would you want to go see it? If five of your friends had seen the movie and all liked it, would you want to see it then? In these cases, you should (and probably would) choose the options that give the greatest likelihood of achieving the things you value (increasing your odds of winning the lottery or seeing a movie you’ll enjoy). It’s no surprise, therefore, that our decisions are influenced by both of these factors—the attractiveness of the outcome and the likelihood of achieving that framing The way a decision is phrased outcome. But our decisions are also influenced by something else that seems trivial and or the way options are described. irrelevant—namely, how a question is phrased or how our options are described. In Seemingly peripheral aspects of the many cases, these changes in the framing of a decision can reverse our decisions, turn- framing can influence decisions by ing a strong preference in one direction into an equally strong preference in the oppo- changing the point of reference. site direction. Take, for example, the following problem: Imagine that the United States is preparing for the outbreak of an unusual disease, which is expected to kill 600 people. Two alternative programs to combat the disease have been proposed. Assume that the exact scientific estimate of the consequences of the two programs is as follows: If Program A is adopted, 400 people will die. If Program B is adopted, there’s a one-third probability that nobody will die and a two-thirds probability that 600 people will die. Which of the two programs would you favor? With these alternatives, a clear majority of participants (78%) opted for Program B—presumably in the hope that, in this way, they could avoid any deaths. But now consider what happens when participants are given exactly the same problem but with the options framed differently. In this case, participants were again told that if no action is taken, the disease will kill 600 people. They were then asked to choose between the following options: If Program A is adopted, 200 of these people will be saved. If Program B is adopted, there’s a one-third probability that 600 people will be saved and a two-thirds probability that no people will be saved. PDecision Making: Choosing Among OptionsO 359 1. Assume yourself richer by $300 than you are today. Given this formulation, a clear majority of participants (72%) opted for You have to choose between: Program A. To them, the certainty of saving 200 people was clearly preferable A. A sure gain of $100, and to a one-third probability of saving everybody (Tversky & Kahneman, 1981). B. A 50% chance to gain $200 and a 50% But of course the options here are identical to the options in the first version chance to gain nothing. of this program—400 dead, out of 600, is equivalent to 200 saved out of 600. The only difference between the problems lies in how the alternatives are phrased, but this shift in framing has an enormous impact (Kahneman & 2. Assume yourself richer by $500 than you are today. You have to choose between: Tversky, 1984). Indeed, with one framing, the vote is almost 4 to 1 in favor of A; A. A sure loss of $100, and with the other framing, the vote is almost 3 to 1 in the opposite direction! B. A 50% chance to lose nothing and a 50% It’s important to realize that neither of these framings is “better” than the chance to lose $200. other—since,