Psychology 243 Notes - Understanding Quantitative & Qualitative Research
Document Details
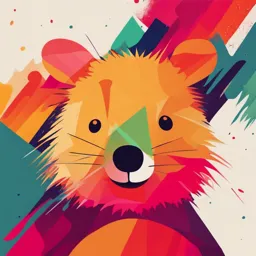
Uploaded by PunctualHummingbird
Stellenbosch University
Tags
Summary
These notes cover the basics of research design in psychology, exploring experimental, correlational, and qualitative designs, questionnaire design, and ethics in psychological research. They are focused on understanding quantitative and qualitative research methods within a psychology course.
Full Transcript
Psychology 243 Understanding Quantitative & Qualitative Research in Psychology CHAPTER 1: THE BASICS OF RESEARCH DESIGN...................................................................................................... 6 THE RESEARCH PROCESS.................................................
Psychology 243 Understanding Quantitative & Qualitative Research in Psychology CHAPTER 1: THE BASICS OF RESEARCH DESIGN...................................................................................................... 6 THE RESEARCH PROCESS..................................................................................................................................................6 MEASURING VARIABLES IN PSYCHOLOGICAL RESEARCH..........................................................................................................6 RESEARCH QUESTIONS & DESIGNS.....................................................................................................................................7 Experimental Design.............................................................................................................................................7 Correlational Design..............................................................................................................................................8 Qualitative Design.................................................................................................................................................8 DEVELOPING HYPOTHESES IN QUANTITATIVE RESEARCH.........................................................................................................9 VALIDITY & RELIABILITY IN RESEARCH...............................................................................................................................11 Construct Validity................................................................................................................................................11 Internal Validity...................................................................................................................................................11 External Validity..................................................................................................................................................11 Ecological Validity...............................................................................................................................................11 Inter-rater Reliability...........................................................................................................................................11 Test-retest Reliability...........................................................................................................................................11 Internal Consistency............................................................................................................................................12 Sampling, Validity, and Reliability.......................................................................................................................12 ETHICS IN RESEARCH.....................................................................................................................................................12 Informed Consent & Debriefing...........................................................................................................................12 Deception............................................................................................................................................................12 Protecting Participants from Harm.....................................................................................................................12 Right to withdraw...............................................................................................................................................12 Anonymity and Confidentiality............................................................................................................................13 CONDUCTING ETHICAL RESEARCH....................................................................................................................................13 PSYCHOLOGICAL REPORTS: STRUCTURE............................................................................................................................14 CHAPTER 2: QUESTIONNAIRE DESIGN.................................................................................................................. 15 DEVELOPING A QUESTIONNAIRE......................................................................................................................................15 WRITING THE QUESTIONS..............................................................................................................................................15 Open vs. Closed Questions..................................................................................................................................15 How To Write Good Questions............................................................................................................................16 CREATING THE RESPONSES.............................................................................................................................................16 Responses to Open-ended Questions..................................................................................................................17 Different Types of Closed Questions....................................................................................................................17 DESIGNING A GOOD QUESTIONNAIRE...............................................................................................................................20 QUESTIONNAIRES & ETHICS...........................................................................................................................................20 ANALYSING QUESTIONNAIRE DATA...................................................................................................................................21 Analysis of Qualitative Data from a Questionnaire.............................................................................................21 Creating Scores from a Questionnaire................................................................................................................21 Determining Scales Within a Questionnaire.......................................................................................................22 CHAPTER 5: EXPERIMENTAL DESIGN.................................................................................................................... 23 THE BASICS OF EXPERIMENTAL DESIGN.............................................................................................................................23 Independent & Dependent Variables..................................................................................................................23 Independent and Repeated Designs....................................................................................................................23 Longitudinal and Cross-Sectional Designs...........................................................................................................23 1|Page Pure-Experimental vs Quasi-Experimental Approach..........................................................................................24 THE SIMPLEST EXPERIMENTAL DESIGN: COMPARING TWO GROUPS/CONDITIONS.....................................................................24 Understanding Variance in Experimental Designs..............................................................................................24 COMPARING THREE OR MORE CONDITIONS.......................................................................................................................25 Familywise Error..................................................................................................................................................25 FACTORIAL (ANOVA) DESIGNS.......................................................................................................................................26 What Is a Main Effect & What Is an Interaction?................................................................................................27 Understanding the Different Ways Interactions May Look.................................................................................28 WHAT DOES A FACTORIAL ANOVA CAKE LOOK LIKE?.........................................................................................................29 DEVELOPING HYPOTHESES FOR EXPERIMENTS....................................................................................................................29 How Many Hypotheses Do You Need?................................................................................................................30 DEALING WITH ASSUMPTIONS WHEN ANALYSING DATA COLLECTED IN EXPERIMENTS................................................................30 Data Should Be (Roughly) Normally Distributed.................................................................................................30 Homogeneity of Variance....................................................................................................................................31 What Do I Do if I Have Violated the Parametric Assumptions?..........................................................................31 CONFOUNDING VARIABLES IN EXPERIMENTAL DESIGNS........................................................................................................31 Designing an Experiment to Deal with Confounding Variables...........................................................................32 Analysis Strategies..............................................................................................................................................33 VALIDITY & RELIABILITY IN EXPERIMENTS..........................................................................................................................33 Internal Validity...................................................................................................................................................33 Test-retest Reliability in Repeated Measures Design...........................................................................................34 ETHICS IN EXPERIMENTS................................................................................................................................................34 RUNNING AN EXPERIMENTAL STUDY................................................................................................................................35 CHAPTER 14: CORRELATIONAL DESIGN................................................................................................................ 36 ANALYSING DATA FROM CORRELATIONAL STUDIES...............................................................................................................36 What Variables Can Be Used in a Correlational Study?......................................................................................37 Correlation Does Not Imply Causation................................................................................................................38 Confounding & Control Variables in Correlational Designs.................................................................................38 Regression Analyses............................................................................................................................................38 How is Regression Analysis like a Cake?..............................................................................................................38 What Kinds of Variables Can We Use as Predictor Variables?............................................................................39 Must the Outcome Variable Always Be Continuous?..........................................................................................40 How Can We Deal with Confounding Variables in Regression Analyses?............................................................41 EFFECTS SIZES IN CORRELATION & REGRESSION ANALYSES....................................................................................................42 How Can We Use Correlational Analyses to Understand Large Datasets?.........................................................42 DEALING WITH ASSUMPTIONS IN A CORRELATIONAL DESIGN.................................................................................................43 VALIDITY & RELIABILITY IN CORRELATIONAL DESIGNS..........................................................................................................43 ETHICS IN CORRELATIONAL RESEARCH..............................................................................................................................45 CHAPTER 21: QUALITATIVE DESIGN...................................................................................................................... 46 QUALITATIVE RESEARCH QUESTIONS................................................................................................................................46 WORDING RESEARCH QUESTIONS....................................................................................................................................47 QUALITATIVE APPROACHES.............................................................................................................................................47 Approaches to Reasoning....................................................................................................................................47 Approaches to Epistemology (Knowledge)..........................................................................................................48 Approaches to Ontology (Perceiving Reality)......................................................................................................48 Approaches to the Researcher’s Influence..........................................................................................................49 Putting it All Together.........................................................................................................................................50 REASONS TO USE A QUALITATIVE DESIGN..........................................................................................................................50 To Generate Theory.............................................................................................................................................50 To Gain Deeper Insight........................................................................................................................................51 Ethnography........................................................................................................................................................51 2|Page To Understand How Meanings are Constructed..................................................................................................51 CHOOSING A QUALITATIVE ANALYSIS TECHNIQUES..............................................................................................................51 DATA IN QUALITATIVE DESIGNS.......................................................................................................................................53 Types of Qualitative Data....................................................................................................................................53 Common Data Collection Methods.....................................................................................................................53 Sampling.............................................................................................................................................................55 SCIENTIFIC RIGOR IN QUALITATIVE DESIGN........................................................................................................................55 ETHICAL IN QUALITATIVE RESEARCH.................................................................................................................................58 CHAPTER 22: DESIGNING INTERVIEWS & FOCUS GROUPS.................................................................................... 61 WHEN MIGHT INTERVIEWS AND FOCUS GROUPS BE USED?.................................................................................................61 Primary Data Collection......................................................................................................................................61 Hypothesis or Research Generation....................................................................................................................62 Measure Development........................................................................................................................................62 Understanding Findings......................................................................................................................................62 CHOOSING BETWEEN INTERVIEWS OR FOCUS GROUPS.........................................................................................................62 Level of Interview Structure................................................................................................................................62 Depth vs Breath & Other Pros and Cons.............................................................................................................63 Participants Behaviour as an Additional Source of Data.....................................................................................63 SAMPLING..................................................................................................................................................................64 Considering your Participant Group....................................................................................................................64 Choosing your Participants.................................................................................................................................64 Sampling for Focus Groups..................................................................................................................................64 DESIGNING INTERVIEW & FOCUS GROUP SCHEDULES..........................................................................................................64 Planning and Reviewing......................................................................................................................................65 Types of Questions..............................................................................................................................................66 Question Wording...............................................................................................................................................67 RUNNING INTERVIEWS AND FOCUS GROUPS......................................................................................................................68 Practicalities........................................................................................................................................................69 Starting & Ending Interviews and Focus Groups.................................................................................................70 Interpersonal Dynamics......................................................................................................................................71 HANDLING INTERVIEW AND FOCUS GROUP DATA................................................................................................................72 Transcribing.........................................................................................................................................................72 Quotations..........................................................................................................................................................73 Data Analysis & Storage......................................................................................................................................73 ETHICAL CONSIDERATIONS.............................................................................................................................................73 REFLEXIVITY................................................................................................................................................................74 CHAPTER 29: MIXED METHODS RESEARCH.......................................................................................................... 75 COMBINING QUANTITATIVE & QUALITATIVE APPROACHES....................................................................................................75 REASONS FOR USING MIXED METHODS............................................................................................................................76 Fuller Understanding...........................................................................................................................................76 Theory Development and Testing........................................................................................................................76 Intervention Evaluation.......................................................................................................................................76 Sampling and Generalisation..............................................................................................................................77 PHILOSOPHICAL CONSIDERATIONS....................................................................................................................................77 Handling Philosophical Differences.....................................................................................................................77 DESIGNING MIXED METHODS RESEARCH..........................................................................................................................78 Timing of the Two Elements................................................................................................................................79 Emphasis Upon the Approaches..........................................................................................................................79 Practical Considerations......................................................................................................................................80 Ethical Considerations.........................................................................................................................................81 Integration and Integrity of the Two Approaches...............................................................................................81 3|Page Presenting Mixed Methods Research..................................................................................................................82 THINKING CRITICALLY ABOUT MIXED METHODS RESEARCH...................................................................................................83 Questions to Ask..................................................................................................................................................83 PLURALISM: COMBINING MULTIPLE QUALITATIVE APPROACHES.............................................................................................84 4|Page Outcomes ✓ Understand how knowledge is acquired with a specific emphasis on the scientific method ✓ Understand the role of theory in scientific research ✓ Formulate a research problem ✓ Understand the difference between descriptive and intervention studies ✓ Understand the difference between quantitative and qualitative research ✓ Understand the various approaches to qualitative research methods ✓ Understand qualitative data collection methods and analysis Textbook V. Bourne, A. I. James, & K. Wilson-Smith (2021). Understanding Quantitative and Qualitative Research in Psychology: A Practical Guide to Methods, Statistics and Analysis. Oxford University Press. 5|Page Chapter 1: The Basics of Research Design Understanding Quantitative & Qualitative Research in Psychology The Research Process Write Analyse Design a Run the your Find & read Develop a Apply for the data study to study and study up background research ethical and see test the collect as a research question approval what you question the data research found report Research area that you are interested in Hypothesis: predicted findings based on research that you have reviewed Replication Crisis: repetition of major psychological research findings which are difficult/impossible to reproduce Three largest problematic practices: o Small sample size (having low power) o HARKing (Hypothesizing After the Results are Known) – developing hypotheses after having analysed data o P-hacking – Analysing data repeatedly, in different ways (such as including/excluding different variables and participants) File Drawer Problem: when studies with strong methodologies which do not replicate previous findings, are not published Pre-registration: when you submit a research proposal to the journal with a clear review of the previous literature, hypotheses developed, and the methods and analysis strategy fully planned out (solution to three practices mentioned above) Measuring Variables in Psychological Research Variable: something that you can measure or manipulate, which will provide data for you to analyse Operationalize: clearly define what you intend to measure Qualitative data will mainly be words whereas quantitative data will be numeric Four Types of Quantitative Data: 1) Nominal Data (categorical, extensive, discrete) a. Frequency of belonging to a “category” with no particular order b. Simply put, it’s like holding up signs and asking participants to stand under the sign that best describes them c. Example: left, right or mixed handedness OR political party choice 2) Ordinal Data (continuous, +++ hierarchical) 6|Page a. Exist on a continuum where there is a clear order to data, but the distance between points may vary b. Example: Place in a race, 1st, 2nd, 3rd place etc... i. There is a clear order (1st to 3rd). However, 1st and 2nd place could have a larger distance between them than 2nd and 3rd. 3) Interval Data (continuous, equal intervals) a. Order to data points, fixed distance between points and negative values b. Example: Temperature, 1° is always the same and negative temperatures are possible i. The difference between 10°C & 20°C is the same amount of difference between 30°C and 40°C 4) Ratio data (continuous, absolute zero) a. Order to data points, fixed distance between points no negative values b. Example: Height, cm is same at all heights, there are no negative heights c. Examples: age, intelligence personality traits, etc. Research Questions & Designs Experimental designs Correlational designs Qualitative designs Experimental Design Where you measure quantitative variables and focus on looking at differences between separate groups of participants/conditions Aim: to manipulate one variable, and then measure another variable that you think might differ according to that manipulation 7|Page Independent variable (what YOU will be comparing) – always a nominal variable Dependent variable (what you will be measuring that you think will vary according to the independent variable) – always continuous variables Correlational Design Based on qualitative data and looks for relationships between variables Example: you might explore the relationship between the amount of chocolate eaten per week and how intelligent a person is POSITIVE relationship VS NO relationship VS NEGATIVE relationship Qualitative Design Analysis of text-based data to identify frequently occurring themes or categories within a dataset Can be collected through questionnaires, interviews, focus groups Example: focus groups with participants who claim to be addicted to chocolate where data might show that there are two different triggers 8|Page Developing Hypotheses in Quantitative Research When conducting a quantitative psychological research study, the way that you frame hypotheses will differ depending on whether you are conducting an experimental or correlational study In an experimental design you would want to frame the hypothesis around the difference that you may or may not find o I might have a null hypothesis that there will be no difference in happiness between participants who like chocolate or not. o Whereas a one-tailed hypothesis would clearly state which group has higher happiness scores. For example, that it is predicted that participants who like chocolate will be happier than those who do not. o A two-tailed hypothesis would predict that the two groups would differ in happiness, but it would not specify which group has higher happiness scores In a correlational design, the hypothesis should focus on the relationship between the two variables o The null hypothesis would predict no relationship without stating whether it was expected to be a positive or negative relationship o A one-tailed hypothesis would include the direction within the prediction (e.g., predicting a positive relationship between cholate consumption and intelligence) o A two-tailed hypothesis would state that there will be a relationship but will not state the specifics of the relationship 9|Page 10 | P a g e Validity & Reliability in Research Validity: you are measuring what you want to measure Reliability: you are measuring it consistently It does what it’s supposed to do consistently Reliability is necessary but not sufficient You can’t have validity without reliability Construct Validity Ensuring that you are measuring what you think you are measuring (content, validity, convergent validity, divergent validity) Internal Validity About how you design your study to ensure that there are no sources of bias that might influence your findings Example: only recruiting participants in the coffee shop located in the Mathematics Department, might bias a sample in the other direction, which would bias the findings and threaten the internal validity External Validity Being able to use your data, which is based on a small sample, and extrapolating it to the wider population Is it representative enough? Ecological Validity Specific form of external validity where the aim is to be able to extrapolate from the lab to the real world (close to real-life as possible) Inter-rater Reliability Only when you have multiple people coding or scoring data where you want to ensure that they are all doing it in exactly the same way Test-retest Reliability If we use our measure with the same person at two different time points, they should get a very similar score o Nothing major should have happened between these two times 11 | P a g e Internal Consistency If we have a measure with multiple data points that all contribute to measuring a single thing, we would expect them all to give similar scores E.g., a person gets a high score on 7 extraversion tests but a low score on another, then we would assume the 8th test does not only measure for extraversion Sampling, Validity, and Reliability Sample should reflect the wider population otherwise the findings are not truly valid and might lack reliability Can use volunteer sampling or random selection, etc. Ethics in Research Informed Consent & Debriefing Participants should be fully aware of their obligation before consenting to take part – consent form o How long will it take? o Where will it take place? o What will they be asked to do? o Are there any risks? After participating, the participant should be fully debriefed regarding the aims of the study, why it was designed in this particular way, and the expected findings Deception Passive deception: not fully informing participants about study details in case it influences their responses Active deception: purposefully misleading participants Protecting Participants from Harm Study should be designed to minimize any potential physical or psychological harm to the participants as well as stating potential harm to participant Right to withdraw Studies should be designed so that participants can withdraw from the study at any point, without any penalty Should be made clear in consent form 12 | P a g e Anonymity and Confidentiality Data must be treated and stored so that participants cannot be identified to ensure anonymity, or if they can be identified, data must be stored securely to ensure confidentiality Conducting Ethical Research The following three documents need to be submitted for review, along with a summary of the rationale for your study and an outline of the methods to be used Participant Information Sheet Title of the project Aims of the project and the reasons why the research is necessary Summary of what participants will be asked to do Statement about anonymity and confidentiality Statement about participation being voluntary and the right to withdraw Information about any possible risked, or that there are none Statement that ethical approval has been granted, and by whom Contact details of the researchers Consent Form Title of the project Statements of confirmation, each of which has a ‘Yes/No’ response o Confirmation that the study and its aims have been explained o Confirmation that they have been able to ask questions about the study o Confirmation that they are aware they can withdraw from the study at any time o Confirmation that they agree to participate in the study Participant’s name, signature, and date Debriefing Sheet Title of the project Aims of the project o This can be more detailed than in the ‘Participant Information Sheet’ Expected findings from the study An explanation of any potential risk, and how to reduce possible lasting effects Contact details of the researchers Say ‘Thank you’ to thy participant 13 | P a g e Psychological Reports: Structure After designing your study, collecting, and analyzing your data, you need to write up your findings in a research report. Basic Outline: Title - About 10-15 words (specific) Abstract - Overall summary of your study (150-250 words) that includes overview of rationale for the study, key methodological points, main findings, brief mention of implications of research Introduction - What do we know? Why is your study needed? Methods - What did you do in your study? Includes participants, materials, procedure, and design & analysis Results - What did you find? Discussion – Summary of results, interpretation, critique, and future directions References - What previous research did you cite? Appendices - Materials to aid understanding of methods/results APA Style for Statistics APA Style for Referencing 14 | P a g e Chapter 2: Questionnaire Design Developing a Questionnaire Consider your research question and what you want to measure Why do you need a questionnaire? What are you measuring? Review existing research and measures Is there an existing measure that I can use? How will yours be novel/new? Gather information to inform questionnaire development (preliminary data) For example, review literature and terminology, interviews Develop the items for your questionnaire Main items of interest Demographics, etc. E.g., Were any of Pilot your questionnaire the questions Ask a few participants to complete it, and gain feedback difficult to answer? Revise your questionnaire Revise (or remove) problematic items, possibly add new items When you are satisfied with your questionnaire, you are ready to use it on a large sample for your main study Writing the Questions It is good to be as consistent as possible throughout the process of developing a questionnaire as participants will respond better if you do so Plan out the way that you want to elicit responses from participants & think about how to structure the questionnaire Open vs. Closed Questions Open-ended questions: allow participant to provide an unconstrained written response (likely qualitative) Closed questions: participant is given a predetermined set of responses from which they need to select the most appropriate response (likely quantitative) 15 | P a g e o Will you be asking participants questions OR asking them to respond to items? Mixed methodology approach – using open-ended and closed questions o Think carefully about how you structure and order the different kinds of questions Advantages & Disadvantages: Open-ended Questions Closed Questions Participant can give an Participant cannot provide an irrelevant unconstrained response answer Participant may give an irrelevant The appropriate response may not be response included Can collect a detailed qualitative Quantitative data can be easier to dataset analyse/interpret Analysis can be complex and time- Data quality is only as good as the quality of consuming the measure How To Write Good Questions Tips Badly Worded Question Well Worded Question Be specific with your Do you regularly participate Do you participate in wording in creative hobbies? creative hobbies more than once a week? Avoid double negatives Do you disagree that Do you value creativity in creativity is not important in everyday life? everyday life? Avoid including two issues Do you take part in creative Do you take part in creative hobbies to relax and to hobbies to relax? socialise? Do you take part in creative hobbies to socialise? Encouraging a detailed Do you like creative Do you like creative response hobbies? hobbies? Please give a detailed answer. Can be answered with a ‘Yes/No’ Creating the Responses Think about the way that participants respond and the options that you make available to them 16 | P a g e Responses to Open-ended Questions Think about the layout of your questionnaire, the space you provide, the detail you want in the answer, etc. The space you leave, indicates approximately the length required of the response Different Types of Closed Questions Three main styles of responding that you can use: 1) Categorical a. Simply ticking a box b. Generates categorical/nominal data c. Ensure that all participants are able to tick one of your responses (E.g., including ‘Other’) 2) Rank-ordered responses a. Ranking options b. Be very clear 3) Likert-scale responses a. Various types b. Consistency – pick one scale c. Need to ensure that your scale has an equal number of ‘positive’ and ‘negative’ responses Five-point Likert Scale 1 2 3 4 5 Very poor Poor Neutral Good Very good Strongly disapprove Disapprove Neither approve nor approve Strongly approve disapprove Strongly disagree Disagree Neither agree nor Agree Strongly agree disagree Far too little Too little About right Too much Far too much Far worse Worse About the same Better Far better Never Seldom Sometimes Often Regularly Never Rarely Some of the time Most of the time Always Far less likely Less likely About the same More likely Far more likely Not interested Slightly interested Interested Very interested Extremely interested 17 | P a g e Likert Scales: 3, 5, or 7 point E.g., When you participate in a creative activity, how often do you feel that your mood is improved? Ways of Scoring a Five Point Likert Scale After you have received your Likert-scale responses, you need to think about how you will turn the responses into quantitative data that you can statistically analyse Example for 5-point scale: neither agree nor strongly disagree disagree agree strongly agree disagree -2 -1 0 +1 +2 0 1 2 3 4 1 2 3 4 5 The different coding systems can have a big impact on the descriptive statistics, such as the mean, minimum, and maximum scores If you are using, or adapting, an established questionnaire, then you should use the same coding so that you can compare your findings with other published research using the same scale Are Likert Scales Parametric or Non-Parametric? Parametric: based on assumptions about the distribution of population from which the sample was taken o The preferable way of analysing data o Need to make certain assumptions o Better to use if your data are calculated from a larger number of Likert scales Non-parametric: not based on assumptions, that is, the data can be collected from a sample that does not follow a specific distribution o Better to use if your data are taken from a single Likert-scale item as the numbers collected are relatively limited in range Acquiescence Bias & Negative/Reverse Scoring Acquiescence bias: the tendency to agree with things 18 | P a g e o Easily resolved by using negative/reversed-scored items Negative/reversed-scored items: having some items where strongly agree is good & having some items where strongly agree is bad o Aim to have an equal split between the two and place it in random order Below, number 1 is positively marked (5 is good) and number 2 is negatively marked (5 is bad) neither strongly strongly with coding disagree agree nor agree disagree agree disagree 1. I find it easy to complete 1 2 3 4 5 creative projects 2. I struggle to think in a 5 4 3 2 1 creative way 3. I enjoy working with 1 2 3 4 5 creative people 4. I am too logical to be 5 4 3 2 1 creative Another method – use tick boxes instead of scores neither strongly strongly without coding disagree agree nor agree disagree agree disagree I find it easy to complete ❑ ❑ ❑ ❑ ❑ creative projects I struggle to think in a ❑ ❑ ❑ ❑ ❑ creative way I enjoy working with ❑ ❑ ❑ ❑ ❑ creative people I am too logical to be ❑ ❑ ❑ ❑ ❑ creative 19 | P a g e Designing a Good Questionnaire Think about your question order: Aim to put important questions early in the questionnaire to ensure you get the vital data from participants Try to ensure that the first few questions are easy and non-threatening, avoid awkward, sensitive, or embarrassing questions If you use both positively and negatively marked items, aim to have an equal number of each, and place them in a random order Remember to include any relevant background or demographic questions that will be needed for your study Think about the layout and formatting: Want to make it as simple and easy as possible for your participants to complete (keep it professional) Give instructions for completing the questionnaire Pick a clear font, and ensure that the layout makes it clear which response options belong to which questions Ensure the pages are not too crowded or too spaced out If there are multiple pages, check that the page turn doesn’t impact on completion and give the instruction to “turn page” Finish by thanking participants and let them know what to do next (e.g., ask the researcher) Proofread Questionnaires & Ethics 20 | P a g e Analysing Questionnaire Data Analysis of Qualitative Data from a Questionnaire Content analysis: converts open data into quantitative data by systematically coding it into categories E.g., ‘What helps you to be creative?’ – You could code the content of each participant’s answer for whether it mentioned the following factors: social support, creative materials, time, motivation. For each category you could set up a variable in SPSS where you code the participants’ answers as yes, it did include category 1 or no it did not include category 1 This process would turn the open data into frequency data, allowing you to report the number and percentage of participants for each category Thematic analysis: looks for repeated patterns of meaning within open data, identifying qualitative themes rather than converting the text into numerical data E.g., you might identify a pattern of ‘preparation’ with various subthemes You might then identify a central theme or a set of core themes and subthemes within these Creating Scores from a Questionnaire Imagine you have designed a creativity questionnaire that contains 30 items - 10 items related to verbal aspects of creativity (e.g., writing), 10 items related to spatial aspects of creativity and 10 items related to physical creativity. Three ways to extract scores: Look at entire questionnaire which will give an Most common overall measure of creativity Look at each scale separately which will give you three measures of creativity – verbal, spatial, physical Look at each item separately which will give you 30 pieces of data to analyse 2 ways to combine the item scores to create summary variables: Sum: add the item scores Mean: calculate the average 21 | P a g e Determining Scales Within a Questionnaire Factor analysis: A statistical method often used to analyse questionnaire data Process – look for groups of items where the responses are highly correlated with each other, and then grouping them together to form a factor Useful when designing a questionnaire that includes multiple scales to confirm that your questionnaire contains multiple and independent scales 22 | P a g e Chapter 5: Experimental Design The Basics of Experimental Design Key idea behind experimental design – want to manipulate something & look for differences between groups/conditions Independent & Dependent Variables Independent variable must be a nominal/categorical variable Dependent variable must be a continuous variable (ordinal, ratio, interval) E.g., Testing whether mindfulness treatment will help with chocolate addiction You need random selection & random assignment o Random Selection: everyone in population has equal chance to be selected o Random Assignment/Randomisation: equal chance of being assigned to one of the other groups Independent and Repeated Designs When thinking about your independent variable you need to decide whether you will use an independent measures design or a repeated measures design Independent (also called between/unrelated/unpaired) measures design: different participants are recruited into each of the conditions o E.g., chocolate addiction study – half of the participants in the control condition and the other half in mindfulness treatment condition o Would allow one to see how much participants crave chocolate if they have had no treatment at all and to compare this to the chocolate cravings of a different set of participants who have had the mindfulness treatment Repeated measures design: just have one group of participants, and they repeatedly take part in the study under different conditions o E.g., chocolate addiction study – test participants before they take part in the treatment and then after treatment Longitudinal and Cross-Sectional Designs Developmental or lifespan research 23 | P a g e Longitudinal design: a repeated measures design, but the phases of testing are often very widely spaced over months/years o Ideal for developmental research Cross-sectional design: independent measures design, comparing separate groups of participants Pure-Experimental vs Quasi-Experimental Approach You should be able to randomly allocate participants to conditions in most experiments (e.g., allocating random people to the mindfulness and control group) However, it’s not always possible o E.g., wanting to compare participants who are addicted to chocolate with participants who are not addicted to chocolate You cannot randomly allocate participants as they are either addicted or not addicted which you cannot determine for them The Simplest Experimental Design: Comparing Two Groups/Conditions Understanding Variance in Experimental Designs There will always be variability in data (e.g., levels of cravings for chocolate) o What explains this variability? Experimental variance: How much of the variability have we created through our experimental manipulation? Variance: a measure that tells us how much the numbers in a group are spread out from the average o If they're all close to the average, the variance is low o If they're far from the average, the variance is high Important to ask – how much of this variability have we caused (by giving half of our participants treatment for their addiction)? By looking at the experimental variance, one can see that the two groups do differ overall (the group who had the mindfulness treatment crave chocolate less) However, there is also variability within each of these two groups – some participants crave chocolate more than others which is called random variance (e.g., some people may have an addictive personality while others do not) Between groups variance: experimental/model variability that can be explained by the IV manipulation Within groups variance: random/residual variability that cannot be explained by the IV manipulation 24 | P a g e Variance as cake o Each statistical test will quantify the amount of experimental variance in the dataset (or the size of the experimental slice of cake) and the amount of random variance in the dataset (or the size of the random slice) o Simply - every statistical test calculates how much of the differences we see in the data are due to the things we're testing and how much are just random differences Lab One Lab Two The amount of random variance is much less in lab one (all participants had similar levels of cravings for chocolate) The mean difference between the control and mindfulness groups was identical in lab one and two Comparing Three or More Conditions Familywise Error Imagine doing three tests on the chocolate addiction participants: control, mindfulness, cold turkey With each test of statistical significance, you have a 5% chance of making a Type I error o Type I error: like a false alarm where the test wrongly indicates something is happening when it's actually due to random variation o We accept that there is a 5% chance that we will find a significant difference in our sample that does not really exist in the population 25 | P a g e Thus, when doing three tests for the chocolate addiction, you are 3 times more likely to find a significant difference (familywise error) Solution: run one overarching analysis that considers the amount of experimental and random variance within a dataset, looking across all of the conditions at the same time (Analysis of Variance/ANOVA) Different types of ANOVA are used to analyse the data collected from different experimental designs (e.g., depending on whether your design is independent or repeated measures) Chocolate addiction study – ANOVA would calculate the amount of experimental and random variance in the dataset The significance of the analysis would then be determined by whether the variance was best explained by the experimental variability (the difference between the three conditions) or the random variance (the variability in scores within each condition) Main effect: tells you whether, overall, there is a difference across all the conditions within that independent variable Once you know that you have a significant main effect, you need to run further analyses, called contrast, to see where these differences come from Factorial (ANOVA) Designs Factorial Designs: manipulating more than one independent variable at a time In another experiment an independent measures (independent variable) where participants were shown either happy or angry faces and then the dependent variable measured their mood We might want to add an additional independent variable like the participant’s mental health which could be sub-divided into no mental health diagnosis, previous diagnosis of anxiety, or previous diagnosis of depression We would then have one independent variable (called IV1) which would be the emotional expression of the face they saw, which would be an independent measures IV with two conditions There would also be a second IV, called IV2, which would be their mental health history (an independent measures IV with three conditions) The dependent variable would then be the mood measure For this example – 2*3 independent measures factorial design o It is 2 because the first IV has 2 conditions o Its 3 because IV2 has 3 conditions o It also tells us that two IVs were manipulated as there are only two numbers 26 | P a g e o If both IVs were repeated measures, then it would be a repeated factorial design o If one IV were independent and the other repeated, we would have a mixed factorial design Summarised below What Is a Main Effect & What Is an Interaction? When you analyse a factorial experimental design, you will be using a factorial ANOVA A two-way ANOVA will contain three separate findings, each of which you will need to understand and interpret: o The main effect of IV1 which will tell you whether the conditions of IV1 differ significantly, when ignoring any possible effects of IV2 ▪ E.g., chocolate – looking at differences between the three treatment types, ignoring IV2, so disregarding whether the data were collected immediately after treatment or six months later o The main effect of IV2, which would be looking at the chocolate cravings immediately after treatment and again six months later, ignoring the type of treatment people had 27 | P a g e o Is there an interaction between IV1 and IV2 ▪ E.g., we might find that the change in chocolate cravings from immediately completing treatment through six months later is different across the three treatment types Understanding the Different Ways Interactions May Look 2*2 interaction o Three possible findings of the chocolate study with either control condition or mindfulness condition o 2*2 interaction (IV1 – control vs mindfulness * IV2 – immediately after six months vs six months later) 3*2 interaction o 3*2 interaction (IV1 – control vs mindfulness vs cold turkey * IV2 – immediately after vs six months later) 28 | P a g e o Parallel lines – little significant interaction (change in chocolate cravings is similar across all three treatment types) o Not parallel – the difference in one IV varies according to the other IV (the change in chocolate cravings is different across the three different groups) What Does a Factorial ANOVA Cake Look Like? The analysis of data would be based on understanding where the variance in your dataset comes from – experimental differences between conditions or the random variance between conditions Factorial ANOVA as a variance cake but with more slices Four slices of variance that we need to understand: o One for each main effect o One for the interaction o One for the residual variance within the dataset that we cannot explain Two examples of how a factorial ANOVA cake might look: Developing Hypotheses for Experiments When developing hypotheses there are quite a few things to consider – such as: 29 | P a g e o Two-tailed (where you predict a difference but with no directional prediction – best for inconsistent findings or when you’re using a different manipulation that has not been considered before) o One-tailed (where you very specifically predict which condition will have significantly higher scores – best when previous research consistently shows higher scores for a condition) How Many Hypotheses Do You Need? Depends on the type of experimental design you use Dealing with Assumptions When Analysing Data Collected in Experiments Four assumptions that must be satisfied to use a parametric analysis: o The data collected must be independent o In experimental design the DV should be at the interval or ratio level o Data must be roughly normally distributed o There should be homogeneity if variance across groups Data Should Be (Roughly) Normally Distributed If you have an independent measures design, you will look at the distribution for each condition separately, and if any condition appears to be not normally distributed, then a non-parametric analysis is best If you have a repeated measures design, you should look at whether the difference scores are normally distributed 30 | P a g e Homogeneity of Variance Mostly, it’s only an issue in independent measures designs You want each group within your design to have similar variability, so you want homogenous variances The variances are small in the first example and large in the second, but homogeneity of variance isn’t concerned with how much variability there is, rather the amount of variance to be similar in each condition The example on the right – the variances are quite different between the groups o Difficult to make analyses and conclusions What Do I Do if I Have Violated the Parametric Assumptions? Confounding Variables in Experimental Designs Confounding variables may explain some of the variability in our dataset, but they are not ones that we are necessarily directly interested in Two different ways to deal with confounding variables: o Design your experiment to minimize the effects of confounding variables 31 | P a g e o Analyse your data to take measured confounds, called co-variables/control variables once measured, into account Designing an Experiment to Deal with Confounding Variables Randomizing participants o If allocations are random, any potentially confounding variables should be randomly spread across the conditions Selecting participants and setting recruitment/exclusion criteria o Only do when a potential confound is unlikely to affect a large proportion of your sample o E.g., having diabetes might be a confounding in the chocolate addiction study thus you might create exclusion criteria for your study o Need to include exclusion criteria in methods and when advertising to participants Matching participants o E.g., you might think that having other addictions might be a confounding variable in your design so it could be problematic if, just by random chance, most of the people with multiple addictions end up in the control group o How would you know whether participants in the control group crave chocolate more than those in the treatment groups, is due to the treatments? Could be due to multiple addictions. o If 6 people report having other addictions, two would go to each group to ensure it is balanced o Once the confound has been considered, aim to randomly allocate participants to groups Counterbalancing o If your design has a repeated measures component, some aspect of your measurement of the DV will have to be repeated for each participant o E.g., chocolate study – participants will have to complete the chocolate craving questionnaire twice: before and after the treatment o Have two comparable versions of the same questionnaire to avoid people responding in a particular o Counterbalancing and randomization ▪ At the first point, half of the participants would complete the questionnaire version 1 and the other half would complete questionnaire version 2 & at the second time vice versa ▪ Need to randomly allocate participants to each of the version orders 32 | P a g e Analysis Strategies Another approach to reduce the potential impact of any confounding variables is to deal with confounds by measuring them, and then controlling them within a particular kind of analysis If the confound explains lots of the variability in our cake, then we no longer know whether our division of the variance into the experimental variance between conditions and the random variance within the dataset, is correct Validity & Reliability in Experiments Validity: you are confident that you are measuring/manipulating what you intend to Reliability: you are doing this in a consistent way Internal Validity Being confident that you have designed and run your experiment in such a way that no other variables may explain your findings E.g., if we know that the severity of the addiction is a confounding variable, then how can we design our study to ensure that any effects of addition severity are minimized 33 | P a g e Experimenter and participant biases can also threaten the internal validity of an experiment o Experimenter – treat all participants in exactly the same way o Participants – behaviour in a research setting Run your experiment as a double-blind study: both the researcher and the participant are blind to the condition that they have been allocated to If this is not possible, run it as a single bond study Test-retest Reliability in Repeated Measures Design If you are collecting data more than one time point, ensure that any measure you use has test-retest reliability – if you collect data twice using the same measure, the scores should be roughly same, if nothing major has changed in between the two times Ethics in Experiments Informed Consent in Experiments Participants should be fully aware of what they are signing up to do, what they will have to do, how long they will be doing it for, whether they must return at a later date, whether there are any risks to them, etc. Let participants know the general aims of the study and avoid specific predictions Deception in Experiments Passive deception: simply not telling participants which condition they have been allocated to (chocolate example) Active deception: telling participants something quite different to what they will actually be asked to do o E.g., you wanted to test a medicinal intervention, and you snuck this into the water that half of the participants were given during their treatment 34 | P a g e How to reduce negative impacts of active deception: o Getting ethical approval o Having an independent group of experts agree that it is necessary o Safeguards like pre-screening o Debriefing: clearly explaining the active deception and the reason it was necessary o Gaining consent after debriefing to publish the results Protecting Participants from Harm Consider how you can protect participants from various risks Ethical approval Safeguards Be clear about potential risks Consent Running an Experimental Study 35 | P a g e Chapter 14: Correlational Design The most important distinction between experimental and correlational designs is the way that variables are treated o Experimental design: we manipulate particular variables (IV) and then see whether this had an effect on another variable (DV) o Correlational study: we do not manipulate anything, we simply measure existing variables (e.g., measuring a person’s age, their intelligence, their personality, or attitudes to look for relationships between these variables) The intricacies of designing correlational studies can vary depending on the questions you want to ask of the data you collect, and the method of analysis that you will employ Analysing Data from Correlational Studies Key design issues in selecting variables in correlational designs: Look at the linear relationship between two continuous variables o This would tell us how the scores on one variable may systematically change as the scores in another variable change o Can analyse this relationship using a Pearson’s correlation 3 different kinds of relationships you can find when running a correlation analysis: o Positive correlation – when the scores on one variable increase as scores on the other variable increase o Negative correlation – as scores on one variable increase, scores on the other variable decrease o No relationship See below for examples 36 | P a g e When a correlation is calculated, you calculate an r statistic o r = correlation value o These values run from -1 (showing a perfect negative correlation) through to +1 (showing a perfect positive correlation) What Variables Can Be Used in a Correlational Study? When designing a simple study that looks at the correlation between two variables, remember that only continuous variables can be correlated (diagram above) Thus, both variables must be measured in such a way that they provide a wide range of values (from smaller to higher numbers) Easiest way to think about this is to fit the variables that you want to measure into this sentence – As the scores on one variable increase, the scores on the other variable increase/decrease. o E.g., As happiness increase, the amount of chocolate eaten will increase. o E.g., As happiness increase, subject choice will ??? (doesn’t work) Attempting to Correlate Categorical Variables: 37 | P a g e o Would be better to use ANOVA to look at whether happiness differs significantly between students studying different subjects Correlation Does Not Imply Causation You might find a significant correlation between two continuous variables, but this does not mean that higher or lower scores on one variable cause higher or lower scores on the other variable E.g., Happiness and chocolate may be correlated but you cannot assume any kind of casual relationship between them Confounding & Control Variables in Correlational Designs When interpreting the findings from a simple correlational analysis, always bear in mind that few things in life can be explained in such a simple way where only two variables are relevant E.g., even if we found a significant positive correlation between happiness and chocolate consumption, there will be lots of potentially confounding variables that might influence that simple relationship like stress levels Partial correlation: when you know potential confounds before running the study and therefore you measure them, and use them as control variables Regression Analyses Designing correlational studies with more than two variables Allows you to include a larger number of variables in your analyses, and you can use these to create a predictive model where you can use a range of different predictor valuables to predict a single outcome variable E.g., we could measure a range of different variables, including chocolate consumption, stress levels, and the amount of exercise someone does as three predictors of how happy someone is How is Regression Analysis like a Cake? Statistical analyses are comparable to slicing up a cake Whichever type of statistics we decide to run, they are always doing the same basic thing – measuring the variability in a dataset and deciding whether the variability represents something meaningful or something random Our variance cake represents all of the variance in the dataset, and the purpose of our analysis is to determine how much of this variance can be explained by the relationship between variables and how much is the leftover residual/random variance Correlational design: 38 | P a g e o The model variance describes the slope of the line of best fit (the straight line that characteristics the strength of a correlation that you see in most scatterplots) o The stronger the relationship is, the better the variance in the dataset is described by the model The unexplained/random/residual variance then comes from how far the raw data points sit from the line of best fit Left: the correlation is quite strong and the raw data points sit quite close to the line Right: has a similarly steep line of best fit but in this example, there is far more error in the dataset which you can see by how far the data points are from the line Although the slopes of the two correlations above are similar, the different amounts of unexplained variance in the dataset mean that the dataset shown on the left has a larger r value, at.90, than the dataset on the right, which has a smaller r value of.74 r values are easy to convert into a meaningful number that will tell you about the amount of variance that is explained by the model, and the amount of leftover, unexplained random variance If you square the r value, giving you the R2, you can find out the proportion of the variance in the dataset that is explained by the model o It is probably easiest to understand this as a percentage, so multiply the R2 by 100 to find out the percentage of the variance in the dataset that is explained by the model (see diagram above) What Kinds of Variables Can We Use as Predictor Variables? Simple correlational analysis – continuous variables 39 | P a g e Regression model has more flexibility in the types of variables that you can include: o Continuous categorical variables o Binary categorical variables – defines which of only two groups that a participant belongs to (e.g., if someone is innocent or guilty of a crime) If your categorical variable only has two groups, it is possible to include them as predictors in a multiple regression analysis (unlike in a simple correlation analysis) However, if you try to use a categorical variable with 3+ categories, you can manipulate your findings Why is it okay to include binary categorical predictors in a multiple regression analysis? o When you have a binary predictor, it should always be coded so that one of the groups is coded as 0 and the other as 1 o E.g., if we code history as 0 and psychology as 1, the analysis will tell us how much happiness changes as a result of one one-point increase in the binary variable o Binary variables as predictors doesn’t change the analysis if we code the two groups the other way around, it just changes the magnitude’s direction Why is it okay to include a binary variable in a regression analysis, but not in a correlation analysis? o In a multiple regression, you are using multiple variables to predict an outcome variable (helpful to include more kinds of predictors that are continuous) o In a correlation you are just analysing two variables Must the Outcome Variable Always Be Continuous? Depends on the type of regression analysis that you will be running In a typical multiple regression, the analyses are based on there being a linear relationship between the predictor and outcome variables 40 | P a g e o Single straight line to describe the relationship o Can have a number of predictor variables that can either be continuous or binary categorical variables, but the outcome must always be a continuous variable Logistic regression: o Predicting an outcome variable that is a binary categorical variable ▪ So, we would be trying to predict which of two categories participants belong to o E.g., we may want to predict whether a participant would prefer to eat white chocolate or milk chocolate. We could then have a number of different predictors, both continuous and categorical. So, we might use happiness, chocolate consumption, and whether the participants have a criminal conviction or not, in an attempt to predict their choice of chocolate. How Can We Deal with Confounding Variables in Regression Analyses? Can include a control variable in a correlation analysis by running a partial correlation, and you can do something similar with a multiple regression called hierarchical regression analysis Hierarchical regression – the analysis calculates the total amount of variance that exists in the dataset o This variance is then, in terms of a cake, divided into three slices: model variance, unexplained variance, control variance o Example: First, the regression determines how much of the variability can be explained by the control variable(s) – example above: o 23% of the variance in the dataset is explained by the control variable o The regression divides up the remaining variance (77%) into that which can be explained by the model (predictor variable(s)) and how much of the variance remains unexplained 41 | P a g e Effects Sizes in Correlation & Regression Analyses In simple correlation analyses, the r value can be treated as an effect size in itself and the conventions for its interpretation as small, medium, or large can be seen below For regression analyses there are a range of different effect sizes, but the most often used is the R2 statistic This tells you about the amount of variance that is explained in the dataset o Instead of saying you have a medium effect size, you would talk about the amount of variance explained Remember: R2 x 100 is the model variance % and the remainder % is the random variance How Can We Use Correlational Analyses to Understand Large Datasets? Factor analysis: used when you have a large dataset that contains many variables, and you want to reduce the number of variables (items, questions, etc.) down to a smaller number of variables that will summarise the entire dataset Best known for analysing data collected in large questionnaires Manifest variables: the original measured variables (e.g., items on questionnaire) Latent variables: manifest variables are reduced to latent variables Example: 42 | P a g e Dealing with Assumptions in a Correlational Design Assumptions across each of the different analyses: o All continuous variables should be (roughly) normally distributed o The relationships between variables are linear (straight line) Linear & Non-linear Relationships The relationship between the two variables differs across the range of scores E.g., variable 1 is age & variable 2 is the number of people within a person’s social network Problem – assuming a linear relationship exists thus forcing a linear description on the dataset If you analyse the distribution of your data and find that they are not normally distributed, then some transformation can be used to make them normal: o Calculate the square root of the variable o To compute logarithmic (log) transformation Validity & Reliability in Correlational Designs 43 | P a g e Test-retest Reliability Would you get the same (or similar) scores if a participant were to complete a measure twice, at different time points? o Good test-retest reliability = same/similar score The more highly correlated the two measures are, the more similar the scores are, and the better the test-retest reliability of the measure Corelations range from -1 to +1, with an r value of +1 showing perfect positive correlation (possible for variance to occur) Internal Consistency All of the items within a scale (or factor) are providing similar scores If all the items are truly measuring the same thing, then when a participant completes the measure, they should get very similar scores across all the items in a scale/factor (should be highly correlated) Cronbach’s Alpha: o The statistic that tells you about internal consistency o Based on making split-half comparisons where the items within a scale are randomly split into two halves, and then the correlation between these two halves is calculated o If they have similar scores, the correlation should be high o Computes all possible split-half companions and then calculates an overall summary score o Example: o Runs from 0 to 1 (Higher values indicating higher levels of internal consistency) 44 | P a g e Ethics in Correlational Research 45 | P a g e Chapter 21: Qualitative Design Qualitative Research Questions Enables you to explore research questions, rather than investigate hypotheses Find out how people perceive and make sense of the world and their experiences within it, as well as construct meanings and accomplish actions with their use of language There are many ways to collect qualitative data, but what they generally have in common is the attempt to find out how people express themselves on the research topic Open data: the data are not limited by measurement scales or driven by experimental manipulations (the possibilities are left open) o E.g., How are you feeling? o Language such as ‘How…?’, ‘What…?’ and ‘In what ways…?’ rather than wording related to making predictions, such as ‘it is predicted that…’ or ‘it is expected that…’ Most common methods of qualitative data collection: o Interviews o Focus groups o Questionnaires Qualitative analysis techniques: o Content analysis o Thematic analysis o Discourse analysis o Interpretative phenomenological analysis (IPA) o Grounded theory o *Conversation analysis o *Narrative analysis Qualitative analysis involves coding the data o Coding: clearly describing categories or themes identified within the data The technique used is linked to the type of research question Qualitative approaches tend to explore research questions rather than hypotheses o Research questions indicate what your research is seeking to investigate but do not make predictions about the outcomes o Explore rather than investigate Inclusion criteria: the key features of the target population that the investigators will use to answer their research question 46 | P a g e Wording Research Questions Worded in an open way to indicate that no particular outcome is being expected Sometimes a qualitative paper will state a research aim rather than a question o Makes it clear what the focus of the study is without assuming the findings Contrastingly, quantitative research has hypotheses and predictions where the wording defines variables in a measurable way and often specifies the expected nature of the relationship between them o Avoid saying things like ‘X will increase or decrease’ or ‘X will lead to Y’ or that ‘X and Y will be correlated’ Examples of potential wording for qualitative research questions and quantitative hypotheses on the same research topics: Qualitative Research Question Quantitative Hypothesis How do older adults in care homes experience It is hypothesized that short-term memory memory loss? loss will increase at a significantly faster rate in older adults who are transferred to care homes compared with those remaining at home. What factors are evident in teenage We predict that the following factors will be reoffenders’ online discussions about their correlated with increased reoffending rates in crimes and rehabilitation? teenagers: i) amount of time spent with peers who have also committed crimes ii) level of financial insecurity Our study aims to identify in what ways It is predicted that passing a grammar school children talk about their experiences of entry exam will lead to higher scores on a grammar school entry exams. wellbeing measure in children. Qualitative Approaches Qualitative Quantitative Explore research questions Investigates research question & hypotheses Inductive reasoning Deductive reasoning Interpretivism Positivism Constructionism Objectivism Approaches to Reasoning Researchers may use both approaches to investigate a research topic Deductive reasoning/top-down reasoning: o Based on information about past findings, qualitative researchers form a theory from which specific hypotheses are developed and tested 47 | P a g e o Starting from the wide picture (the theory) and working down from this to the specifics (hypothesis testing) o Quantitative studies emphasize deductive reasoning Inductive reasoning/bottom-up reasoning: o Instead of generating and examining data through hypotheses testing, qualitative researchers start by collecting and examining the data o Starting with the specifics (the data) and working up from this to generate ideas about the bigger picture (theories and concepts) o Qualitative studies emphasize inductive reasoning Approaches to Epistemology (Knowledge) In experimental and correlational designs, we aim to design studies which often control for factors beyond our variables which might influence the results o Underlying idea – it is possible to narrow in on just what you are interested Different epistemological in and gain objective knowledge about that through your research positions In qualitative designs we aim to design rigorous studies which enable us to fully understand the phenomenon of interest and wider factors which influence it o Underlying idea – it is not possible to gain useful knowledge about something without seeing it in context Epistemology: the theory of knowledge, covering the nature of knowledge and how it can be used Positivism: o A philosophy of science which suggests that true knowledge can only be gained through objective scientific methods o This approach is linked to deductive, quantitative studies Interpretivism: o A philosophical approach which suggests that it is only possible to understand something in the context of how people perceive and make sense of it (how they interpret it) o This approach is linked to inductive, qualitative studies Approaches to Ontology (Perceiving Reality) ‘If a tree falls in the forest and no-one is there to hear it, does it make a sound?’ o Do things in the world exist independent of human perception? Ontological o If a human is in the forest, the vibrations in the air from the falling tree will Questions lead to the person perceiving a sound through the effect on their ears o If no human is there, the vibrations will not affect any ears and so arguably there is no sound Ontology: philosophical study of being, covering the nature of reality and our existence 48 | P a g e Objectivism: o An approach to the perception of reality which suggests that phenomena exist outside of social actors o Quantitative research generally takes an objectivist approach Constructionism: o An approach to the perception of reality which suggests that phenomena are created by the actions and perceptions of social actors, and are a continuous process o Qualitative research generally takes a constructionist approach Social constructionism: knowledge is seen as constructed by people Constructivism: approach suggesting that reality is subjectively constructed by individuals and linked to individuals’ cognitive processes Work that’s described as ‘experiential’ or ‘realist’ suggests that is it possible to access people’s internal states and experiences Work that’s described as containing a relativist position suggests that reality is constructed through language Approaches to the Researcher’s Influence Quantitative researchers aim to prevent any researcher influence upon the research process o This is linked to positivist and objectivist approaches – objective knowledge of a phenomenon can be gained, and is separate from researchers’ interpretation Phenomenology: o A philosophy that focuses on understanding and making sense of experiences o Phenomnological psychology: ▪ Emphasis upon the diversity of human experience and suggests that it is not possible to suspend our own biases when studying a phenomenon o Interpretative phenomenology: ▪ Suggests that it is impossible to separate out the researcher’s interpretation from the description of a phenomenon, and that their assumptions and knowledge can be used to gain a fuller understanding ▪ Aim: to provide interpretative accounts of how participants make sense of their experiences o Descriptive phenomenology: 49 | P a g e ▪ Places importance on the researcher bracketing out their own prior biases and knowledge, to seek the purest possible understanding of a phenomenon ▪ Aim: to reach a detailed description of participants’ lived experience Reflexivity: o Qualitative researchers focus on being able to examine their own thoughts, feelings, motivations, and