Evaluating Research Claims - Psychology PDF
Document Details
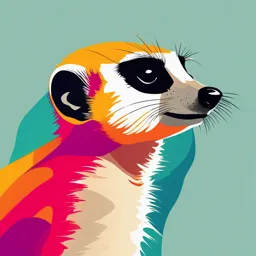
Uploaded by WellRegardedCactus
Tags
Related
- CM4 - Recherche et QoL PDF
- PSYC 2360 The Research Process PDF
- Cambridge International AS and A Level Psychology Coursebook PDF
- Ch.2 PDF - Reading and Evaluating Scientific Research
- Chapter 2: Reading and Evaluating Scientific Research PDF
- Educational Psychology: A Tool for Effective Teaching Lesson 1 PDF
Summary
This document discusses evaluating research claims, focusing on key concepts like population validity and causality within the context of research methods, particularly in psychology. It covers various sampling methods and statistical considerations. The text explores the different elements critical for a sound, properly evaluated, research study.
Full Transcript
EVALUATING RESEARCH CLAIMS? Population: who the claim is about Sample: Who is actually being studied External Validity: is the sample generalizable to the larger population Construct: a concept, phenomenon, or attribute Variable: What is actually being observed Construct Validity: how well are the...
EVALUATING RESEARCH CLAIMS? Population: who the claim is about Sample: Who is actually being studied External Validity: is the sample generalizable to the larger population Construct: a concept, phenomenon, or attribute Variable: What is actually being observed Construct Validity: how well are the construct being operationalized Causality: how are the constructs related? Design: how is causality examined? Internal validity: Can the design be used to establish cause-and-effect? * independent variables causes changes to dependent variables Hypothesis: what outcomes support the claim? Results: what size of an effect can be detected? Statistical Validity: are the conclusions accurate and reasonable given the data * anecdotes are not generalizable. There are too many contextual factors at play to isolate what may have caused what. * even if the trend occurs across a large group of people, without a comparison group one cannot know if the same outcome might have occurred 3 Conditions of Causality - Covariance: as the iv changes, so does dv - Manipulate conditions of the iv allows for clearer comparison of outcomes on the dv - Temporal precedence: changes in the iv occur before changes in the dv - Manipulating the iv before measuring the dv establishes the directionality of the effect - Internal validity: no alternative explanations exist - Randomly assigning participants to conditions is the best was to create unbiased groups for comparison - Holding all factors constant, except for the iv, reduces confounds and helps to isolate cause-and-effect Who is the claim about? - Sample or Population = External Validity What is the claim about? - Construct or Variable = Construct Variable What causes what? - Causality or Design = Internal Validity - Is it a causal claim? - Frequency: how much or how many - Association: covariance or difference - Causality: cause and effect change - Independent variables causes changes to dependent variables - Was this an experiment? - Manipulated IV: Are comparisons based on a manipulated IV? - Experimental Control: Is the IV manipulated before the DV is measured? - Random assignment to condition: Is assignment ti IV conditions unbiased? - ASSOCIATION IS NOT CAUSATION - Establishing an association between two measured variables is not enough to make a claim of causality Possibilities: - A causes B - B causes A - A-B is confounded - Does the data support it? - Hypothesis or Variable = Statistical Validity - SAMPLE SIZE MATTERS - To detect meaningful effects, statistic must be based on a large sample of date, rather than only a few anecdotal data points CHOICES AND TRADE-OFF Low Ex-Low In - Good for initial exploration and theory testing Low Ex-High In - Good for testing theories about causal patterns and exploring new treatments High Ex-Low In - Good for describing population and making real world predictions High Ex-High In - The gold standard of treatment and intervention, but, expensive and rare *External validity: Are the samples and constructs generalizable to the population of interest? *Operationalize: how something is defined, measured, or manipulated Media Article vs Journal Article Media - Secondary source - Written by a journalist - Summary - Not reviewed by scientist Journal - Primary source - Written by the researchers - Detailed report - Peer reviewed by top scientist *Research is probabilistic: research describes general trends, there are always exceptions to the findings - Scientist do not use words like “proof: or “cure” casually External validity - Population Validity - Population of interest > research population > sampling frame > actual sample - Representative sample: a samples in which all the members of a population have an equal chance of being included (unbiased vs biased) - Sampling Methods: - Probability: random method - Generalisation goal: when the goal of research is to generalise research findings to describe a specific population of interest - Simple random - List of everyone on population > random process to select your sample - List of possible phone numbers > random process to select numbers - Stratified - recruit is based on specific demographic characteristics - Proportional: identify strata and then randomly sample from within each strata to ensure proportional representation - Oversampling: identify strata and then randomly sample from within each strata to equal proportional - Common to combine methods - Cluster - Identify natural clusters > randomly select clusters > sample within each cluster - Multistage usually - Combined - Limits - Nonresponse bias: even when participants are chosen based on a random method, there may be bias in who chooses to actually participate in the research - Nonprobability: nonrandom method - Theory-testing goal: when the goal of the research is to test a theoretical relationship irrespective of a specific identified population - Convenience sample: the researcher samples those who are conveniently available or who volunteer to participate - Purposive sample: recruit volunteers the meet specific criterion - Quota sample: researchers recruit volunteers from different subsets of the population until specific quotas are filled - Limits - Biased sampling: people who are conveniently available may not be representative of the population - Self-selection bias: people who volunteer to be in a study may not be representative of the population of interest * Sampling Errors is always possible * Margins of error for percentage estimates Sample size affects statistical validity Sample method affects external validity - Ecological Validity - Experimental Realism - Key Within Psychology Describing Data vs Making Inferences Descriptive stats: help organise and summarise the data that come from sample - Frequency distribution describes how often each score occurs (histogram) - Central tendency describes what is typical (mean, median, mode) - Variance describes the amount of spread across the scores Inferential stats: use data from samples to test theories and make inferences about population 1. Data from samples can only provide a rough point estimate of the true population parameter 2. The margin of error must be taken into consideration when making statistical inferences 3. Even when accounting for the margin of error, there is always a risk of making an error Null Hypothesis Significance Testing: a set of decision rule that can help a researcher determine if an observed effect is extreme enough to reject a null hypothesis - Starts with the assumption that there is no effect (aka null effect) - If observed effect =/ null, reject null hypothesis, “statistically significant”, explore alternative hypothesis - If observed effect = null, cannot reject null hypothesis, “not statistically significant”, hypothesis not supported - Not reliable at all when sample size is low - tells nothing about the size of the effect - An effect is a specific outcome that you are testing - Group Comparisons: an effect that compares the data from different groups - Bar graphs - Correlation Effects: an effect that examines the correlation between variables - Scatterplots - Correlation coefficient ‘r’ - -1 to +1 Effect size: considers the strength of the relationship or effect between two or more variable - Cohen's D - Point Estimate: the estimate of the effect calculated from the data - Margin of Error: the likely range of error around the point estimate - Confidence interval: a range of values likely to obtain the “true” effect P Value: the probability that an effect as extreme as what has been observed would occur if the null hypothesis were true 2 Types of Error - Type 1 Error (False Positive): concludes that there is an effect when there is none - Testing controls for type 1 errors - Type 2 Error (False Negative): concludes there isn’t effect when there is one - Statistical Power: the probability a study will detect an effect if that effect actually exist - Influence by effect size and sample size - Larger effects are easier to detect than smaller ones - Larger sample sizes make it easier to detect an effect - Power Analysis: the size of a sample that would be needed to statistically detect an effect at a given significance level and desired level of power *small sample sizes increase the risks of both type 1 and type 2 errors The replication crisis: arose when key scientific findings could not be independently replicated by other research teams Replication: when an effect is independently replicated across multiple studies, one can feel more confident that it is a “true” effect - A meta analysis averages the effects size for each study to calculate an overall effect size