Marketing Analytics Final Exam Study Guide PDF
Document Details
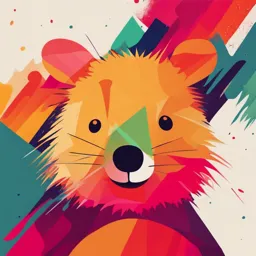
Uploaded by ProficientCoral
Tags
Related
- Dispensa Marketing Chiacchio-Ambrosanio 2 PDF
- Chapter 4: Marketing Plan PDF
- AR 541: Business Management & Application for Architecture 1 Midterm - Lecture Handouts PDF
- HUM 4072 - Unit 2_ Data Analytic Thinking PDF
- Marketing Strategy Summary PDF
- Market Planning, Business Development, and Financial Management PDF
Summary
This study guide provides an overview of various marketing analytics concepts, including heuristics, anchoring and adjustment, scientific approaches, and experimental-based attribution. It examines how to maximize profits and understand decision-making processes in marketing.
Full Transcript
Marketing Analytics Study Guide Part 1 Resource Allocation Heuristic Approach: when you use common sense/ knowledge to make judgements about reallocating Simple to understand and easier to use Often incorrect, because they rely on gut, personal know...
Marketing Analytics Study Guide Part 1 Resource Allocation Heuristic Approach: when you use common sense/ knowledge to make judgements about reallocating Simple to understand and easier to use Often incorrect, because they rely on gut, personal knowledge, lack scientific basis marketing outcomes might include outcomes such as market share, sales, brand loyalty, customer satisfaction, and stock price. Each outcome potentially is influenced by managers’ past decisions about various resource trade-offs Anchoring and Adjustment Period : start with an initial idea (the "anchor") and then make changes to that idea based on new information you get later. Here’s how it works in simple terms: 1. Anchoring: You begin with a starting point or assumption. For example, if you're deciding how much money to spend on marketing, you might start with a rough figure based on past experiences or what you’ve done before. 2. Adjusting: As you get more information (like new sales numbers or customer feedback), you update or change your initial assumption to better reflect the new data. So, you "anchor" your decision on something you already know, and then "adjust" it when new facts or experiences suggest a change is needed. Scientific Approach: How to maximize profits while deciding the level of marketing efforts we need to do so. Essentially figuring out how do sales change depending on marketing efforts Black box?? Experimental-Based Attribution a strategy where a company uses data from controlled experiments to determine how much to spend on different marketing touchpoints (like ads, emails, website visits) in a customer journey ○ Involves an intervention, outcome experimental condition design, and control condition They set up two groups: one group gets the new marketing effort (the "intervention"), and the other group doesn’t get it (the "control"). This helps them compare results and see what actually works. ○ Only change one thing at a time helps them see how each change affects customer behavior Expensive, sometimes impossible Through this approach all factors under the firms control remain constant Shows relationship between marketing resources and past outcomes Shows historical data that contains insightful information about how much marketing resources truly increase outcomes Profit Change = (sales increase x gross margin) - profit change Uphill/Downhill Firms If firm is on the uphill side it needs to increase its investments to reach the sweet spot at the top If it is on the downhill side it needs to decrease its investments Profit Formula Profit= (sales x margin) - total fixed cost Write out steps with pen/paper Text Analysis Structured Data Numbers Row x column format 20 percent of enterprise data Unstructured Data Text, images, audio, video Cannot be organized in row x column format More storage 80 percent of enterprise data Text data ○ Social media, emails, text files, news articles Image Data ○ Instagram Videos ○ Youtube 8 Vs of BIG DATA Volume: can you find the information you’re looking for? Value: can you find it when you most need it? Veracity: are you dealing with information or disinformation? Visualization: Can you make sense at a glance? Does it trigger a decision? Variety: Is a picture worth a thousand words in 70 languages? Is your info balanced? Velocity: Information gains momentum and crises and opportunities evolve in real time. How is the outlook today? Viscosity: Does it stick with you? Does it call to action? Virality: Does it convey a message that can be passed into a presentation or instagrammed? Features of Textual Data Unstructured Noisy Redundant Context sensitive content Multiple interpretations possible Processing difficulty Many possible features Natural Process Learning Area of computer science and AI that deals with human and computer language interaction NLP is a type of Ai that allows computers to understand and process human language NLP is used for tasks such as machine translation, spam filtering, and sentiment analysis NLP is used by ○ Google translate: to translate from one language to another ○ Gmail uses NLP to filter spam emails ○ Twitter uses NLP to identify and remove abusive tweets ○ Amazon uses NLP to understand customer reviews ○ Apple uses NLP to power its Siri virtual assistant NLP, text mining, and large language models (LLMS) are all related fields that deal with the analysis of text data NLP is a broader field that includes all aspects of how computers understand and process human language Text mining is a more specific field that focuses on extracting patterns and insights from text data LLMS are trained on large amounts of text data. This allows them to generate text, translate languages, write different kinds of creative content, and answer your questions in an informative way NLP continued POS tagging: identifies parts of speech Parsing: like POS but goes a step further by braking it down into a diagram like a tree Sentence breaking: breaks blocks of texts into sentences Terminology extraction: identifying and extracting the most used and important words in the text Key Steps in Processing Textual Data Preprocess data ○ Syntactic: Grammar ○ Semantic: Meaning Generate features ○ Bag of Words: counts how many times each word is used in a sentence The cat sat on the mat The 2, cat: 1, sat: 1, on: 1 Select features like simple counts and statistics Text mining ○ Classify, cluster, associate, analyze Key Applications Categorizes documents using supervised learning Cluster documents using unsupervised learning Summarize documents Visualizes documents (word clouds, graphs) Predict sentiment or next word Sentiment Analysis Sentiment analysis is the process of figuring out the feelings or opinions expressed in a piece of text. In simple terms, it helps a computer understand whether the mood of the text is positive, negative, or neutral. Computer rating how possy or neggy a sentence is Determine the author’s attitude towards a topic Uses Improves customer services and increase brand reputation Gain better and meaningful insights Aid in innovation Stay in-line with ongoing trends Predict stock performance Analyze high volumes at affordable costs Sentiment Analysis in Marketing STP Improve customer service Brand monitoring Market research Track campaign performance Broader Technique Train computer software to understand text in ways similar to humans Several stages of analysis before providing the finding sentiment Identify key words to highlight the core message of the text Tokenization: breaking sentence into words Lemmatization: converts words into their root form Stop-word removal: filters out words that don’t add meaning Keyword Analysis ○ Analyze extracted keywords and give them a sentiment score ○ Provides a relative perception of the emotion expressed in text for analytical purposes For example, 10 for satisfaction 0 for disappointment 3 Approaches Rules (Lexicon) based Machine Learning Based Hybrid Rules (Lexicon) based ○ Identify, classify, and score keywords based on lexicons ○ Scan for all words in a sentence and sum it up for final sentiment score ○ Issues: new words mean expanding lexicons, different cultures Lexicon (Sentiment Dictionary): 1. A lexicon is a list of words, where each word has an associated sentiment score (e.g., positive, negative, or neutral). 2. When you input a piece of text (like a sentence or a review), the system compares the words in the text with the lexicon. 3. Each word is checked against the lexicon to see if it has a positive or negative sentiment score. 4. After analyzing all the words in the text, the system sums up the scores: Machine Learning Based Neutral networks and deep learning Creates a sentiment analysis model and train it repeatedly on known data so that it can guess the sentiment in unknown data with high accuracy Issues: may need retraining to use in a different context Hybrid Combines ML with rules-based approaches