Quantitative Research Methods In Political Science Lecture 4 PDF
Document Details
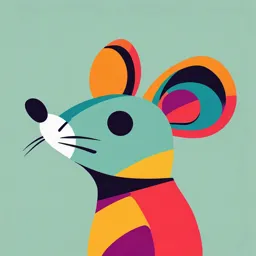
Uploaded by ConscientiousEvergreenForest1127
Toronto Metropolitan University
2024
Michael E. Campbell
Tags
Related
- Research Methodology in Political Science PDF
- Lecture 1 - Introduction to Quantitative Methods 09-04-2024 PDF
- Quantitative Research Methods in Political Science Lecture 2 PDF
- Quantitative Research Methods in Political Science Lecture 3 PDF
- Quantitative Research Methods In Political Science Lecture 5 (10/03/2024) PDF
- Quantitative Research Methods in Political Science Lecture 6 PDF
Summary
This document is a lecture on quantitative research methods in political science, focusing on the normal curve and Z-scores. The lecture covers topics like causality, variables, and the use of systematic processes in the social sciences, with examples for adults and children's IQ scores.
Full Transcript
Quantitative Research Methods in Political Science Lecture 4: The Normal Curve and Z Scores Course Instructor: Michael E. Campbell Course Number: PSCI 2702 (A) Date: 09/26/2024 Qu...
Quantitative Research Methods in Political Science Lecture 4: The Normal Curve and Z Scores Course Instructor: Michael E. Campbell Course Number: PSCI 2702 (A) Date: 09/26/2024 Quick Recap Lecture 1 Lecture 2 The role of statistics in the social Causality (or causal relationships) sciences The use of systematic processes Independent and dependent variables Difference between facts and values Conceptualization and operationalization The characteristics of variables Instruments and instrumentation Discrete vs. continuous Variables Systematic and random measurement Levels of measurement error (reliability and validity) Lecture 3 Descriptive and univariate statistics: Proportions, percentages, rates, ratios Measures of central tendency: Quick Mode, median, mean Recap Measures of dispersion: IQV, range, interquartile range, variance, standard Cont’d deviation Frequency distribution tables Graphs and charts: Pie, bar, histograms All of what we’ve looked at, in one way or another, serves as the foundation for the Normal Curve The Normal Curve Is a theoretical model used in statistics Can be used to precisely describe empirical distributions The Normal Curve: “a special kind of perfectly smooth frequency polygon that is unimodal (i.e., it has a single mode or peak) and symmetrical (unskewed) so that its mean, median, and mode are all exactly the same value” Healey, Donoghue, and Prus 2023, 126. The Normal Curve Cont’d The Normal Curve resembles the unskewed distribution from last lecture (Chapter 3) Is bell shaped (gaussian curve) and the tails extend into infinity Does not exist in nature – no distribution we observe in empirical reality will ever match this exactly Several variables (or data distributions) come close enough that we can assume data are The Normal Curve Cont’d Think of normal curve as a tool: 1. To make descriptive statements about empirical distributions 2. To be used in inferential statistics to generalize from sample to population Distances along the horizontal axis will always encompass same proportion of total area under the curve when measured in standard deviations The distances between any point and the mean cuts off the same proportion of the total area when measured in standard deviations The Normal Curve Example These data represent the IQ scores for children Hypothetical Distribution of IQ Scores and adults Among Children and Adults Children Adults In each case, the distributions are symmetrical (unskewed) = 100 = 100 Each has a sample (n) of 1000 = 20 = 10 The mean () is the same for each = 1000 = 1000 The standard deviations (s): 1. For children s = 20 2. For adults s = 10 The Normal Curve Example Cont’d Larger spread of data for children’s IQ scores because std. dev. is larger Two scales appear here: 1. IQ Units 2. Standard deviations from the mean Technically no difference between these scales Since the mean is 100: 1. One standard deviation above the mean for children is 120 2. One standard deviation below the mean for children is 80 Why? Because the standard deviation for The Normal Curve Example Cont’d The same logic applies for adult IQ scores Since the standard deviation is 10 for adults: 1. One standard deviation below the mean = 90 2. One standard deviation above the mean = 110 Area Under the Normal Curve Between Lies +/- 1 Std. Dev. 68.26% of area +/- 2 Std. Dev. 95.44% of area +/- 3 Std. Dev. 99.72% of area When measured in standard deviations, the distances along the horizontal axis on any normal curve will always encompass the same proportion of area under the curve Area Under the Normal Curve Cont’d 68.26 % Area Under the Normal Curve Cont’d Between Lies +/- 1.65 Std. Dev. 90% of area +/- 1.96 Std. Dev. 95% of area +/- 2.58 Std. Dev. 99% of area Social scientists tend to use whole number area values (90%, 95%, 99%) Useful for building confidence intervals (see textbook chapter 6) Can also be expressed in # of cases… For example, if you have 1000 cases 1. 683 cases +/- 1 std. dev. from mean Z scores are the way scores are expressed after they have been standardized to the theoretical normal curve Z Scores (Standard This means that if we want to find “the percentage of the total area (or number of cases) above, below, or between scores in an empirical distribution, we must first express the original Scores) scores in units of the standard deviation or convert them to Z scores, which are also called standard scores” (Healey, Donoghue, and Prus 2023, 129). Original units can be anything (weight, time, IQ scores, etc.) Z scores will always have the same values for mean and std. dev. Mean will always be 0 and standard deviation will always be 1 Computing Z Scores When we convert raw scores (e.g., in our case the IQ of an adult of child) into Z scores, we are changing the original units of measurement into Z scores This standardizes the normal curve to a distribution that has a mean of 0 and a standard deviation of 1 The formula for Z scores is: In this equation: is an individual score is the sample mean is the sample standard deviation Computing Z Scores Cont’d Let’s say you have 5 scores: 10, 20, 30, 40, 50 First, calculate the mean (see lecture 3 + textbook chapter 3): Computing Z Scores Cont’d Then calculate standard deviation (see lecture 3 + textbook chapter 3): Remember, to calculate this, you need to know the deviations (the distance of each score from the mean) Computing Z Scores Cont’d Take the sum of squared deviations and input them into the std. dev. formula… Therefore, the standard deviation is: Computing Z Scores Cont’d With this information, you can now compute the Z scores: Raw Scores: 10, 20, 30, 40, 50 s = 14.14 The five Z scores are: 1. -1.414 2. -0.707 3. 0.000 4. 0.707 5. 1.414 Positive and Negative Z Scores A positive Z score will fall to the right of the mean A negative Z score will fall to the left of the mean For instance, a positive Z score of +1.00 indicates that the score will fall exactly +1.00 standard deviation to the right of the mean Remember, when you convert the In our case, a positive Z score of 1.414 original scores to Z scores the nomal indicates that a score of 50 will fall 1.414 distribution takes on a mean of 0.00 and standard deviations to the right of the mean a standard deviation of 1.00 (see above) The Standard Normal Curve Table A Standard Normal Curve Table (or Z table) tells you the areas related to any Z score(s) Can be found in Appendix A of textbook (p.501 to 504 in 5th ed.) For our purposes right now, we can used abridged version… The Standard Normal Curve Table Cont’d There are three columns: 1. Column (a) shows Z score 2. Column (b) shows area between mean and Z 3. Column (c) shows area beyond the Z If we look at a Z score of 1.00 in this table, we see the area between Z and the mean is 0.3413 – why? When you calculate Z scores, you are standardizing a data point by expressing how many standard deviations it is away from the mean… 68.26% of all cases fall within 1.00 standard deviations from the mean… Therefore, the distance between a Z score of 1.00 and the mean is half of this – i.e., 0.3413 Answer 0.341 0.34 3 13 0.6826 Positive Z Score Example Let’s go back to the IQ of children Children’s IQ Scores = 100 Question: if a child has an IQ score of 130, how much of the = 20 area under the normal curve lies between the mean and this score? = 1000 Positive Z Score Example Cont’d First thing, convert raw score into Z score: Positive Z Score Example Cont’d We see that the area covered in column (b) of the standard normal curve table is 0.4332 Therefore, we can say a proportion of 0.4332 (or 43.32%) of the total area under the curve lies between this score and the mean.” Negative Z Score Example The same logic applies if the Z score is negative For instance, let’s say a child had an IQ of 93 Since the mean is 100, this score will fall to the left of the mean and will yield a negative Z score Negative Z Score Example Cont’d With a Z score of -0.35, the area between Z and the mean is 0.1368 We know the Z score is negative, so we move to the left of the mean Knowing this, we can say “a proportion of 0.1368 (or 13.68%) of the total area under the curve lies between this score and the mean” Note, the Z table will always have positive proportions. However, if the Z score is negative, it simply means you Finding the Total Area Below a Score Let’s say we want to find the total area below the scores of two child subjects in the IQ sample distribution we’ve been following Therefore… Their scores are 117 and 73 (therefore = 117 and = 73) = -1.35 First, calculate the Z scores for each … Finding the Total Area Below a Score Let’s start with the area below a positive score ( It is positive, so it will fall to the right of the mean The Z table tells us that that there is a proportion of area that is equal to 0.3023 (or 30.23%) between the Z score of +0.85 and the mean Keep in mind Normal Curve is symmetrical (mean median and mode are all the same) We know that 50.00% of all cases fall below the median and 50.00% above the median Finding the Area Below a Score Cont’d Therefore, we add 50.00% to 30.23% This gives us a total of 80.23% Therefore, a child who scored 117 on their IQ test scored higher than 80.23% of the sample Finding the Area Below a Negative Score Now, let’s use the negative Z score ( = -1.35) It is negative, so it will fall to the left of the mean Because the score is negative and we want to know the area below it, we use “the Area Beyond Z” in the Standard Normal Curve Table This Z score corresponds with a total area of 0.0885, expressed as 8.85% Therefore, since we are looking at the area beyond Z, we can say that 8.85% of children had an IQ lower than 73 Finding the Area Below a Negative Score Cont’d Finding a Z Score Above a Positive Score To find the area above a positive score, it is essentially the same process Let’s say the child’s IQ score was 108 First, find Z: Since Z is positive, look at “Area Beyond Z” in Z table In this instance, proportion of area is 0.3446 (or 34.46%) Finding a Z Score Above a Positive Score Cont’d Since you’re looking at the area beyond Z, it is as simple as stating that 34.46% of children in the same have an IQ score above 108. Finding the Area Above/Below Z Summarized Sometimes, we only know the percentile of a score A percentile is similar to a quartile (See Lecture 3 + Textbook Chapter 3) Percentile: “identifies the point below which a Finding specific percentage of cases fall” (Healey, Donoghue, and Prus 2023, 135). Raw Scores For example, if 25% of cases fall below a quartile, 30% of cases will fall below the 30th percentile With our knowledge of the Normal Curve, we can determine the original (or raw) score when we know a percentile Finding Raw Score Example Let’s use the example of adult IQ scores ( = 100, s = 10, n = 1000) We want to find an adult whose IQ is at the 98.5th percentile (98.5% of all cases had a lower IQ) First, subtract 50% from 98.5%, which gives us 48.5% Then, divide this number by 100 (to turn percentage into proportion) This gives us a proportion of 0.4850 Finding Raw Score Example Cont’d Look in column (b) “Area Between the Mean and Z” in Z Table 0.4850 is associated with a Z score of 2.17 Input into Z score equation This becomes: = (2.17)(10) + 100 = 121.70 Finding Raw Score Example Cont’d Therefore, the raw score is 121.70 Or “the raw score of an adult whose IQ is at he 98.5th percentile is 121.70” IQ = 121.70 Finding the Area Between Two Scores on Opposite Sides of the Mean If you have two scores on opposite sides of the mean, find the area between them by adding the areas between each score and the mean Using the Children’s IQ sample, we want to find the area between the scores of 93 and 112 Solve for Z: Finding the Area Between Two Scores on Opposite Sides of the Mean Cont’d The area between the mean and is 0.1368 (or 13.68%) The area between the mean and is 0.2257 (or 22.57%) Therefore, 13.68% + 22.57% = 36.25% This means that 36.25% of the total area under the curve falls between IQ scores of 93 and 112 Or, about 363 cases fall between the IQ scores of 93 and 112 Finding the Area Between Scores on Same Side of Mean Let’s say we have IQ scores for children of 113 and 121 Solve for Z: Finding the Area Between Scores on Same Side of Mean Cont’d Find the area between each score and the mean by looking at column (b) “Area Between Mean and Z” (area between Z of +0.65 and mean is 0.2422 (or 24.22%)) (area between Z of +1.05 and mean is 0.3531 (or 35.31%)) Subtract from (35.31% -24.22% = 11.09%) Finding the Area Between Scores on Same Side of Mean Cont’d Therefore, 11.09% of the total area under the curve lies between these two IQ scores Or, we might say “about 111 cases out of the 1000 cases falls between these two IQ scores” Note: the same technique is used if you have two negative Z scores Using the Normal Curve to Estimate Probabilities So why does any of this matter? Beyond the techniques we just learned, “the theoretical normal curve may also be thought of as a distribution of probabilities” (Healey, Donoghue, and Prus 2023, 140). Probabilities: “the likelihood that some event…will occur” (Healey, Donoghue, and Prus 2023, 140). We can use the normal curve to estimate the probability that a randomly selected case from a normally distributed interval-ratio level variable has a score that falls in a certain range Probabilities The formula for probabilities is: Depending on what you want to find the probability for, the definition of “events” will change You want to know the probability of selecting a king of hearts from a deck of cards on your first draw The number of events that constitute a success =1 The number of possible events = 52 Probabilitie s Example The probability of selecting the card is 1 in 52 But probabilities are expressed in proportions (thus why we use “p” for both proportions and probabilities) “Over the long run, the events we define as successes will bear a certain proportional relationship to the total number of events” (Healey, Donoghue and Prus 2023, 141) Probabilitie Therefore, over an infinite number of draws, the s Example proportion of successful draws would be 0.0192 Or, if we did 10 000 draws, we could say “for every 10 000 draws, about 192 will be he king of hearts, and the remaining 9808 or so selections will be other cards” (Healey, Donoghue, and Prus 2023, 141). Using the Normal Curve to Estimate Probabilities Cont’d Probabilities range form 0.00 (no possibility of event happening) to 1.00 (a certainty that the event will occur) The higher the value of the probability, the more likely the event is to happen For example, 0.0192 is close to zero and therefore unlikely (or improbable) “It is unlikely that you will draw the king of hearts on your first try” Using the Normal Curve to Estimate Probabilities Cont’d If you can specify the number of successes and the number of events, you can always determine the probability For example, what is the probability of rolling 4 on a die? A die has six sides, and you have 1 possibility of success… Probability Distributions Listing the probability of each event gives you a Probability Distribution He probability distribution of rolling a single die is: Discrete Probability Distribution Event 1 1 1 1 1 1 Probabi 0.1667 0.1667 0.1667 0.1667 0.1667 0.1667 lity The sum of probabilities will always equal 1.00 with rounding error What is the probability of rolling a 1 or a 3 on your first try? 0.1667*2 = 0.3334 Variables can be (see Lecture 1 + Textbook Discrete Chapter 1): and 1. discrete 2. continuous Continuous Discrete variables are whole numbers (nominal Probability and ordinal variables will always be discrete) Distribution Continuous variables may or may not have s decimals Interval-Ratio variables may or may not be continuous It is important to distinguish between these, as the way to compute probabilities is different for each Discrete and Continuous Probability Distributions Cont’d Discrete probability distribution describes probability of occurrence of each event of a discrete variable Continuous probability distribution, like the normal curve, describes the probability of an area under the curve This happens because of the infinite nature of continuous variables… They require that probabilities be calculated for a range of values under the normal curve (and not for a specific value) Probabilities for Continuous Variables “By combining our understanding of probability (as the ratio of the number of successes to the number of possible events) with our knowledge of the normal curve, we can conveniently estimate the likelihood of selecting a case within a certain range for any continuous variable with a normal distribution” (Healey, Donoghue, Prus 2023, 142). Probabilities for Continuous Variables Example We want to know the probability of randomly selecting a subject from the distribution of children’s IQ scores between 95 and 100 (which is the mean) The probabilities are given to us in the Standard Normal Curve Table First, convert 95 into a Z score: Probabilitie Using the standard normal curve table, we see that the area between the score and the mean s for is 0.0987 Continuous This is the probability Variables In other words, the probability of randomly Example selecting a case that will have a score between 95 and 100 is 0.0987 (or 0.10 if we round off) Cont’d Therefore, the probability is one in ten, meaning we have a 1 in 10 chance of randomly selecting a case whose IQ falls between 95 and 100 Think of the areas under the normal curve as probabilities and not just proportions Probabilities for Continuous Variables Example #2 What is the probability of selecting a child whose IQ is less than 123? The score is above the mean, so we must use our knowledge of the median Solve for Z: Probabilities for Continuous Variables Example #2 We look at the “Area Between Mean and Z” – which is 0.3749 Add 0.5000 to this… We get a value of 0.8749 (rounded to 0.88) Therefore, the probability of randomly selecting a child with an IQ of less than 123 is 0.88… Or, for every 100 children selected from this group over an infinite number of trials, 88 would have IQ scores of less than 123 and 12 would not Remember, you are stating “over an infinite number of trials,” because the probability is expressed in terms of what happens over the long Probabilities at a Glance When you have a normal distribution, the probability that you will select a case close to the mean is very high The further away from the mean, the lower the probability of selecting it This is because the majority of cases cluster around the mean The probability of randomly selecting a case that within 1 standard deviation from the mean is 0.6826 Probabilities at a Glance Cont’d The probability of selecting a case that falls beyond three standard deviations from the mean is very small See “Area Beyond Z” for Z score of 3.00 (area under the curve equals 0.0013) Multiply by area in both tails = 0.0026 Therefore, the probability of randomly selecting a case that falls 3 standard deviations (or selecting a case with a very high score or a very low score) will only occur 26 times About 68 cases out of 100 cases selected over out of 10 000 trials the long run will have a score that falls between -1 and +1 standard deviations