Quantitative Research Methods in Political Science Lecture 6 PDF
Document Details
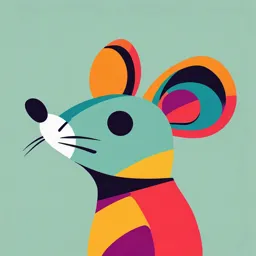
Uploaded by ConscientiousEvergreenForest1127
Toronto Metropolitan University
2024
Michael E. Campbell
Tags
Related
- Quantitative Research Methods in Political Science Lecture 3 PDF
- Quantitative Research Methods In Political Science Lecture 4 PDF
- Quantitative Research Methods In Political Science Lecture 5 (10/03/2024) PDF
- Quantitative Research Methods in Political Science Lecture 3 PDF
- Quantitative Research Methods In Political Science Lecture 8 PDF
- Quantitative Research Methods in Political Science Lecture 12 (12/05/2024) PDF
Summary
Lecture 6 of Quantitative Research Methods in Political Science, focusing on estimation procedures. The lecture introduces point estimates and confidence intervals as two key types of estimation procedures, outlining their differences and applications in inferential statistics. It further details characteristics of estimators, such as unbiasedness and efficiency, and provides examples to illustrate the concept of bias. The lecture also dives into the concept of efficiency.
Full Transcript
Quantitative Research Methods in Political Science Lecture 6: Estimation Procedures Course Instructor: Michael E. Campbell Course Number: PSCI 2702 (A) Date: 10/10/2024 Estimation Procedures...
Quantitative Research Methods in Political Science Lecture 6: Estimation Procedures Course Instructor: Michael E. Campbell Course Number: PSCI 2702 (A) Date: 10/10/2024 Estimation Procedures Are techniques used to estimate population values from sample statistics The primary objective of inferential statistics is infer from sample to population There are two types: 1. Point Estimate: “is a sample statistic that is used to estimate the population value” (Healey, Donoghue, and Prus 2023, 175). 2. Confidence Interval: “consist of a range of values (an interval) instead of a single point” (Healey, Donoghue, and Prus 2023, 175). When we use estimation procedures, we work with estimators… Estimators Are something known about your sample (a statistic that estimates some fact of the population) Examples: 1. A sample mean () can be used to estimate information about the population mean () 2. A sample standard deviation (s) can be an estimator for the population standard deviation () Estimators must be: 1. Unbiased 2. Efficient Bias “An estimator is unbiased if, and only if, the mean of its sampling distribution is equal to the population value of interest” (Healey, Donoghue, and Prus 2023, 175). Symbolically, this is Based on knowledge of sampling distribution, we know this will be the case if n is large enough. If unbiased, the sampling distribution mean / proportion is equal to the population parameter, and we know how many cases fall above and beyond this mean Example: you want to know the household income of a community You collect a random sample of 500 (n=500) The sample mean is $35 000 ( = $35 000) Population mean unknown ( = unknown) Since n = 500, we know the sampling distribution is normal, and its mean is equal to the population Bias Cont’d mean Therefore, if an estimator is unbiased, it is likely to be accurate in terms of estimating the population parameter… However, in less than 1% of cases, the sample will be 3 standard deviations from the mean… Efficiency Refers to the extent that the sampling distribution is clustered around the mean The smaller the standard error or the mean, the more sample means or proportions cluster around the mean… Remember, the standard deviation of a sampling distribution (SE) is the population standard deviation divided by the square root of the sample size (i.e., ) Therefore, the standard error decreases as the sample size increases and efficiency increases as the standard error decreases Efficiency Cont’d Example: You want to know the average income of full-time workers in a community. You collect two random samples with different sizes… Both are unbiased… Sample 1 Sample 2 = $75 000 = $75 000 You know the population standard dev. = = 100 = 1000 $5000 So, let’s calculate the standard error for each sampling distribution () (see right) $500.00 $158.13 Efficiency Cont’d As you can see, the sampling distribution is more clustered around the mean in Figure 6.3 This is because the standard deviation of the sampling (i.e, the SE) is smaller The SE is an inverse function of n More clustering around the mean = higher efficiency Therefore, we can have more confidence in larger samples They will also allow us to make more precise estimations Point Estimates To construct a point estimate 1. Draw EPSEM sample 2. Calculate mean or proportion In our last example, the point estimate was $75 000 This does not mean that the actual mean of the population is $75 000, but we can be confident that our sample mean approaches the population mean due to the theorems that underpin the sampling distribution Furthermore, the larger the sample, the more confidence we can have in our estimator (because it will be more efficient) Interval Estimates / Confidence Intervals More complex than point estimates, but safer bet… Not a single value, but a range of values This range of values is more likely to include the true population parameter Calculated through a series of steps… Alpha () is the significance level It represents the level of risk a researcher is willing to take of being wrong Most common = 0.05 (but varies by research) Step 1 – Select When set at 0.05, you can also say you are using a 95% confidence level Alpha Essentially, if you were to construct an infinite number of confidence intervals with set at 0.05, 95% of them would contain the population value and 5% would not Setting at a low-level means there is only a small chance the population parameter will fall outside the interval Step 2 – Find Corresponding Z Score for Alpha First, divide the probability into the upper and lower tails of the sampling distribution Remember, probabilities are often expressed as proportions (See Lecture 4 + Textbook Chap. 4) If is 0.05, place half probability in lower tail and half in upper tail Now, find the Z score associated with a proportion of 0.0250 in “Area Beyond Z” (see Lecture 4 + Textbook Chap. 4) Step 2 – Find Corresponding Z Score for Alpha Cont’d Corresponding Z score is 1.96 This tells us 95% of all possible sample outcomes will fall within 1.96 Z-score units of the population value You will only have one sample in research – so this tells you that 95% of all such intervals will include the population parameter Step 2 – Find the Corresponding Z Score for Alpha Cont’d Therefore, we can be 95% confident that out interval contains the population parameter Different Levels of Confidence Social scientists use whole-number areas when constructing confidence intervals (most commonly 90%, 95%, and 99%) These values always correspond with standard deviations values of 1.65, 1.96, and 2.58 A point estimate is a single value that doesn’t account for uncertainty Note that 99.9% is rarely used in the Confidence intervals account for sampling variability and uncertainty – and reflect the social sciences greater degree of confidence that you have in your estimate Step 3 – Construct Confidence Interval The final step is to actually construct the confidence interval How you do this will change, depending on the information you have Interval When you know the standard deviation for the population, you construct confidence interval Estimation using the following formula: Procedures c.i. = for Sample In this equation: Means = the sample mean ( known) = the Z score determined by the alpha level = the standard deviation of the sampling distribution (i.e., the standard error of the mean) Example for Constructing Confidence Interval ( known) You want to know the average IQ of a c.i. = community c.i. = You collect sample of 200 individuals (n c.i. = = 200) c.i. = c.i. = Sample mean () is 105 Population standard deviation () is 15 Therefore… 105 - 2.08 = 102.92 You set alpha () at 0.05 (5% chance of 105 + 2.08 = 107.08 being wrong) “We can be 95% confident that the Z score is 1.96 average IQ score for the community we are studying will fall somewhere A Note on Sample Size When n is 100 or larger, Central Limit Theorem applies Central Limit Theorem: “If repeated samples of size n are drawn from any population with mean and standard deviation , then, as n becomes large, the sampling distribution of sample means will approach normality, with mean and standard deviation ” (Healey, Donoghue, and Prus 202, 159). (See Lecture 5 + Textbook Chap. 5) In other words, this theorem tells us that if something (i.e., a trait) is not normally distributed in the population, we can still construct a normal curve if we increase the size of our samples (i.e., if we increase the size of n) When we do not know the standard deviation of Interval the population, you construct confidence interval using the following formula: Estimation Procedures c.i. = for Sample In this equation: Means = the sample mean ( unknown) t = the t score as determined by the alpha level and n – 1 degrees of freedom = the estimated standard error of the mean when is unknown Notice the use of t as opposed to Z Student’s t Distribution We use the t distribution in two situations: 1. When we use the sample standard deviation df Example 1 : you have five scores (1, 2, 3, 4, 5) 2. When the sample size is smaller than 100 The mean for the group is 3 Therefore, the distribution has 5-1 (or 4 df) The t Distribution acts as a function of the If the four scores are 1, 2, 3, and 4, the fifth must be 5 degrees of freedom (df) df Example 2: You have five roommates with whom you “Degrees of freedom are the number of values split the bills in a distribution that are free to vary” (Healey, Bills per month = $500 Donoghue, and Prus 2023, 183). If you’re roommates pay $435 per month, you don’t need to know how much each of them pays to know you owe $65 In other words, when you have a specific Any combination of values can be assigned to them value for the mean, n – 1 scores are free If this sum equals $435, the amount you owe will not to vary because one score is fixed change Student’s t Distribution Cont’d As you can see, with smaller samples, the t distribution is smaller and flatter As n increases, the t distribution begins to resemble the Z distribution (converges when n = 120) When sample size increases, the sample standard deviation becomes a more reliable estimator of the population standard deviation Student’s t Distributio n Cont’d Using the t Distribution Let’s say you have a sample size of 30 (n = 30), with an alpha of 0.05 ( = 0.05) First, compute df (in this case 30-1 = 29) you have 29 degrees of freedom Using “Significance for Two Tailed Tests” go to column 0.05 Go down rows until you find df 29 This gives you a t score of 2.045 A Note on t and Z Converge nce Constructing Confidence Interval ( unknown and n = 30) c.i. = You want to know the average IQ for a community c.i. = You take a sample (n = 30) c.i. = c.i. = = 30 and s = 15 c.i. = Therefore, we can estimate that the = 0.05 (corresponding t equals 2.045) population IQ has a mean that is in the range of 99.31 and 110.69 There is only a 5% chance that we are wrong Constructing Confidence Interval ( unknown and n = 30) Cont’d The issue here is that n = 30… Therefore, Central Limit Theorem does not apply This means we need to assume that the IQ scores are normally distributed in the population Therefore, to summarize, there are two instances in which the t distribution is used instead of the Z distribution: 1. When you have a small sample size, but you can assume the population has a normal distribution 2. When the population standard deviation is unknown (which will be most cases) Graphing a Confidenc e Interval Indicates the lower and upper levels of the confidence We can say interval (LCL we are 95% Graphing and UCL) confident that out of an a Dot in the infinite center is sample number of Confidenc mean samples of the same e Interval size, that the population mean will fall somewhere between 99.31 and 110.69 Confidence Interval Summary If Sample and Use formula Statistic is a Population standard Mean deviation is known c.i. = and n 100 or population is normally distributed Population standard Mean deviation is unknown c.i. = and n 100 or population is Controlling Width of Confidence Intervals Two things affect width of confidence intervals: 1. The level at which you set alpha (i.e., your accepted probability of being wrong) 2. The sample size Alpha is most commonly set at 0.10 (90%), 0.05 (95%), 0.01 (99%) The higher the confidence level, the wider the width of the interval Conversely, the larger the sample size, the narrower the confidence interval Interval Width and Alpha 90% () 95% () 99% () c.i. = c.i. = c.i. = c.i. = c.i. = c.i. = c.i. = c.i. = c.i. = c.i. = 115 000 811.72 c.i. = 115 000 964.22 c.i. = 115 000 1269.23 Interval Width and Alpha Cont’d 90% confidence Interval () 𝑿 c.i. = 115 000 811.72 LC UC L L $115 000 $114 188.28 $115 811.72 95% confidence Interval () 𝑿 c.i. = 115 000 964.22 LC UC L L $115 000 $114 035.78 $115 964.22 99% confidence Interval () 𝑿 c.i. = 115 000 1269.23 LC UC L L $115 000 $113 730.77 $116 269.23 Confidenc e Width and Sample Size A Note on Sample Size and Margins of Error Sampling Approximate Minimum Sample Size Error (%) ±1 10 000 ±2 2 500 ±3 1 111 ±4 625 ±5 400 ±10 100