LA 276 Remote Sensing PDF 2024
Document Details
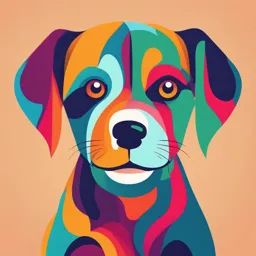
Uploaded by EnviableRetinalite7104
University of Mines and Technology
2024
MANTEY, Saviour
Tags
Summary
This document is a set of lecture notes on remote sensing and drone technology, from the University of Mines and Technology. The summary provides a table of contents and introduction to the module. It has details about remote sensing, drone technology, and image analysis.
Full Transcript
LA 276 Principles of Remote Sensing and Drone Technology Compiled by: MANTEY, Saviour Department of Geomatic Engineering June 2024 TABLE OF CONTENTS COURSE OBJECTIVES/FOCUS..........................
LA 276 Principles of Remote Sensing and Drone Technology Compiled by: MANTEY, Saviour Department of Geomatic Engineering June 2024 TABLE OF CONTENTS COURSE OBJECTIVES/FOCUS........................................................................................... 5 COURSE SCHEDULE........................................................................................................... 6 ASSIGNMENTS..................................................................................................................... 7 ATTENDANCE POLICY........................................................................................................ 7 CLASS CONDUCT................................................................................................................ 8 ASSESSMENT OF LECTURER............................................................................................. 8 ASSESSMENT OF STUDENTS............................................................................................. 9 GRADING POLICIES............................................................................................................ 9 ADDITIONAL COMMENTS.................................................................................................. 9 1. INTRODUCTION TO REMOTE SENSING................................................................... 10 1.1 DEFINITION.................................................................................................................................. 10 1.2 ELEMENTS OF REMOTE SENSING.................................................................................... 10 1.3 ELECTROMAGNETIC RADIATION...................................................................................... 11 1.4 THE ELECTROMAGNETIC SPECTRUM............................................................................ 12 1.5 INTERACTIONS WITH THE ATMOSPHERE.................................................................... 16 1.5.1 Scattering................................................................................................................................ 16 1.5.2 Absorption.............................................................................................................................. 17 1.6 RADIATION - TARGET INTERACTIONS........................................................................... 18 1.7 PASSIVE VERSUS ACTIVE SENSING................................................................................. 19 2. SATELLITES AND SENSORS...................................................................................... 21 2.1 INTRODUCTION......................................................................................................................... 21 2.2 SATELLITE CHARACTERISTICS: ORBITS AND SWATHS........................................ 21 2.3 SPATIAL RESOLUTION AND PIXEL SIZE........................................................................ 23 2.4 SPECTRAL RESOLUTION....................................................................................................... 24 2.5 RADIOMETRIC RESOLUTION............................................................................................... 25 2.6 TEMPORAL RESOLUTION..................................................................................................... 26 2.7 MULTISPECTRAL SCANNING.............................................................................................. 27 2.8 THERMAL IMAGING................................................................................................................. 29 2.9 GEOMETRIC DISTORTION IN IMAGERY......................................................................... 30 3. IMAGE ANALYSIS....................................................................................................... 33 2|Page 3.1 INTRODUCTION TO DIGITAL IMAGE PROCESSING................................................. 33 3.2 PREPROCESSING...................................................................................................................... 34 3.3 IMAGE ENHANCEMENT......................................................................................................... 38 3.4 IMAGE TRANSFORMATIONS................................................................................................ 40 3.5 IMAGE CLASSIFICATION AND ANALYSIS...................................................................... 42 3.6 DATA INTEGRATION AND ANALYSIS.............................................................................. 44 REFERENCES AND RECOMMENDED READING............................................................. 47 APPENDICES...................................................................................................................... 48 Appendix 1: Landsat Spectral Bands............................................................................................. 48 Appendix 2: Step-by-step Guide to Processing Landsat data to detect Landcover Changes: A Case studies of the Accra and Tema Metropolis Techniques.......................... 49 Appendix 3: ESUNλ constant........................................................................................................... 65 Appendix 4a: Non-Leap Year (DOY)............................................................................................ 66 Appendix 4b: Leap Year (DOY)...................................................................................................... 67 Appendix 5: Earth–Sun distance (d) in astronomical units for Day of the Year (DOY). 68 3|Page LIST OF FIGURES FIGURE 5.1ELEMENTS OF REMOTE SENSING (A-G)....................................................................................................10 FIGURE 1.2 ELECTRICAL AND MAGNETIC FIELDS OF ELECTROMAGNETIC RADIATION...................................................11 FIGURE 1.3 ELECTROMAGNETIC SPECTRUM..................................................................................................................12 FIGURE 1.4 DETAILED ELECTROMAGNETIC SPECTRUM ILLUSTRATING WAVELENGTH AND FREQUENCY.......................13 FIGURE 1.5 UV PORTION OF THE ELECTROMAGNETIC SPECTRUM................................................................................13 FIGURE 1.6 PRISM..........................................................................................................................................................14 FIGURE 1.7 IR PORTION OF THE ELECTROMAGNETIC SPECTRUM..................................................................................15 FIGURE 1.8 MICROWAVE PORTION OF THE ELECTROMAGNETIC SPECTRUM..................................................................16 FIGURE 1.9 ENERGY SOURCES AND ATMOSPHERIC TRANSMITTANCE............................................................................18 FIGURE 1.10 RADIATION - TARGET INTERACTIONS........................................................................................................19 FIGURE 2.1 INSTANTANEOUS FIELD OF VIEW.................................................................................................................23 FIGURE 2.2 TARGET (LEAF) INTERACTION WITH VISIBLE AND INFRARED WAVELENGTHS..............................................24 FIGURE 2.3 ACROSS –TRACK SCANNING.......................................................................................................................27 FIGURE 2.4 ALONG-TRACK SCANNING..........................................................................................................................28 FIGURE 2.5 TARGET (LEAF) INTERACTION WITH VISIBLE AND INFRARED WAVELENGTHS.............................................29 FIGURE 2.6 GEOMETRIC DISTORTIONS IN ACROSS-TRACK SCANNING SYSTEMS............................................................31 FIGURE 3.1 DROPPED LINES..........................................................................................................................................35 FIGURE 3.2 GEOMETRIC REGISTRATION PROCESS.........................................................................................................36 FIGURE 3.3 RESAMPLING...............................................................................................................................................37 FIGURE 3.4 BILINEAR INTERPOLATION...........................................................................................................................37 FIGURE 3.5 CUBIC CONVOLUTION.................................................................................................................................38 FIGURE 3.6 SPATIAL FILTERING.....................................................................................................................................39 FIGURE 3.7 IMAGE SUBTRACTION..................................................................................................................................41 FIGURE 3.8 IMAGE CLASSIFICATION...............................................................................................................................42 4|Page COURSE OBJECTIVES/FOCUS The main objective of this course is to introduce students to the basic concepts and techniques of Remote Sensing. At the completion of this course, students should; 1. Define Remote Sensing 2. Know the elements of Remote Sensing 3. Understand the Electromagnetic Spectrum 4. Know the Electromagnetic Radiation 5. Understand the interaction of Remote Sensing radiation with the atmosphere a) Scattering b) Absorption 6. Understand radiation target interaction 7. Differentiate between Active and Passive Sensors 8. Understand the satellites and sensors for Remote Sensing 9. Know the characteristics 10. Be familiar with the various types of sensor resolution which include a) Spatial resolution and Pixel Size b) Spectral resolution c) Radiometric resolution d) Temporal resolution 11. Understand the various scanning platforms in Remote Sensing 12. Understand thermal imaging 13. Be familiar with image distortions 14. Be introduced to Digital Image Processing 15. Be familiar with image pre-processing techniques 16. Understand image classification and analysis 17. Integrate and analyse Remote Sensing Data 18. Be familiar with Drone Technology and its applications 5|Page COURSE SCHEDULE Week Activity Week 1 Understand course objectives, course outline, grading policy, etc, Introduction to Remote Sensing Week 2 Introduction to Remote Sensing (Continued) Week 3 The Electromagnetic Spectrum and Electromagnetic Radiation Week 4 Interactions with the Atmosphere Active and Passive Sensors Week 5 Quiz 1 Satellites and Sensors Week 6 Satellites and Sensors (Continued) Week 7 Introduction to Digital Image Processing Week 8 Quiz 2 Image Preprocessing Week 9 Image Classification and analysis Week 10 Data integration and analysis Week 11 Class Revision Assessment of Course Delivery Week 12-15 Revision in preparation for Examinations/ End of Semester Exams * Note that from time-to-time assignments will be given!! 6|Page ASSIGNMENTS All work will be due on the date specified. Late assignments will be assessed a penalty of 5% per day or fraction thereof. All work must be completed to receive a passing grade for this course. No assignments will be accepted after the end of semester exam in which the assignment was given. When you have answers that are less than 1, always begin the number with a zero. For example;.471 shall be written as 0.471. When writing angles, minutes and seconds must always have two units, excluding any decimal portion. If a minute or second contains only single units, i.e., 4 minutes, 7 seconds, the number shall be proceeded by a zero. In this case the angle shall be written as 04’ 07”. Unless otherwise stated, all angles will be presented in degrees, minutes and seconds format. ATTENDANCE POLICY Each student shall attend all lectures and practicals prescribed for this course as a pre-condition for writing this examination. Any student who absents himself/herself for a total of 30 % of the time for this class without proper permission shall be deemed not to have satisfied the attendance requirements for this course and shall not be allowed to take part in the end of semester examinations of this course. I understand that each student may upon occasion need to be away from class due to illness or other important matters. The following policy recognizes these life issues but at the same time reflects the real world need to be present in class in order to learn and share your learning with others in the class. Exceptions to the Attendance Policy (Verification of all exceptions is necessary); 1. A University-sponsored event in which an excused absence from me is obtained. 2. Death of a family member or close relation. 3. Extended hospitalization (this does not apply to a visit to the health centre because of a cold or other illness). 7|Page CLASS CONDUCT It is essential that everyone in this class establish a mutual respect amongst each other in this class. Therefore, there are a few simple rules that you will be asked to adhere to. These rules are; 1. Please make every effort to arrive on time by planning ahead for any contingencies. 2. Do not begin to pack up your books and other items early. This is very distracting. You are expected to participate throughout the entire class period. 3. Turn off all mobile phones and other electronic devices before class. If there are extenuating reasons, please see me. Please note that ½ mark shall be deducted from a student anytime his/her mobile phone rings in class. 4. During the lecture, feel free to ask questions, but refrain from conducting personal conversations. 5. Sleeping, eating and reading newspapers are not allowed in class. While in class the student is expected to pay attention and participate and not finish work for another class during this class periods. ASSESSMENT OF LECTURER Each student at the end of the course will be required to evaluate the course and the lecturer’s performance by answering a questionnaire specifically prepared to obtain the views and opinions of the student about the course and lecturer. Please be honest and candid in this evaluation exercise. 8|Page ASSESSMENT OF STUDENTS The student’s assessment will be in two forms; Continuous Assessment 40% and End of Semester Examination 60%. The Continuous Assessment shall include Quizzes, Class Attendance and Assignments. The results of the cumulated average shall be made known to students at least one week before the start of the Semester Examinations. The End of Semester Examinations shall be marked over a total of 60% out of which 10% shall be reserved for presentation of work (this includes legibility, grammar, spelling, neatness of work and adherence to instructions). GRADING POLICIES Raw Total Score (%) Letter Grade Interpretation 80 – 100 A Excellent 70 – 79.99 B Very Good 60 – 69.99 C Good 50 – 59.99 D Pass Below – 50 F Fail - I Incomplete ADDITIONAL COMMENTS This class represents a commitment of time and energy for both the university and student. If you have problems, please see me as soon as possible. Waiting until the end of the semester may be too late. 9|Page 1. INTRODUCTION TO REMOTE SENSING 1.1 DEFINITION "Remote sensing is the science (and to some extent, art) of acquiring information about the Earth's surface without actually being in contact with it. This is done by sensing and recording reflected or emitted energy and processing, analysing and applying that information." 1.2 ELEMENTS OF REMOTE SENSING Remote sensing process involves an interaction between incident radiation and the targets of interest. This is illustrated by the use of imaging systems where the following seven elements (A-G) are involved (Fig.1.1). Figure 1.1 Elements of Remote Sensing (A-G) (i) Energy Source or Illumination (A) – the first requirement for remote sensing is to have an energy source which illuminates or provides electromagnetic energy to the target of interest. (ii) Radiation and the Atmosphere (B) – as the energy travels from its source to the target, it will come in contact with and interact with the atmosphere it passes through. This interaction may take place a second time as the energy travels from the target to the sensor. (iii) Interaction with the Target (C) - once the energy makes its way to the target through the atmosphere, it interacts with the target depending on the properties of both the target and the radiation. 10 | P a g e (iv) Recording of Energy by the Sensor (D) - after the energy has been scattered by or emitted from the target, we require a sensor (remote - not in contact with the target) to collect and record the electromagnetic radiation. (v) Transmission, Reception and Processing (E) - the energy recorded by the sensor has to be transmitted, often in electronic form, to a receiving and processing station where the data are processed into an image (hardcopy and/or digital). (vi) Interpretation and Analysis (F) - the processed image is interpreted, visually and/or digitally or electronically, to extract information about the target which was illuminated. (vii) Application (G) - the final element of the remote sensing process is achieved when we apply the information we have been able to extract from the imagery about the target in order to better understand it, reveal some new information or assist in solving a particular problem. 1.3 ELECTROMAGNETIC RADIATION As was noted, the first requirement for remote sensing is to have an energy source to illuminate the target (unless the sensed energy is being emitted by the target). This energy is in the form of electromagnetic radiation. All electromagnetic radiation has fundamental properties and behaves in predictable ways according to the basics of wave theory. Electromagnetic radiation consists of an electrical field (E) which varies in magnitude in a direction perpendicular to the direction in which the radiation is travelling and a magnetic field (M) oriented at right angles to the electrical field. Both these fields travel at the speed of light (c). Figure 1.2 Electrical and Magnetic Fields of Electromagnetic Radiation 11 | P a g e Two characteristics of electromagnetic radiation are particularly important for understanding remote sensing. These are the wavelength and frequency. The wavelength is the length of one wave cycle, which can be measured as the distance between successive wave crests. Wavelength is usually represented by the Greek letter lambda (λ). Wavelength is measured in metres (m) or some factor of metres such as nanometres (nm, 10-9 metres), micrometres (μm, 10-6 metres) (μm, 10-6 metres) or centimetres (cm, 10-2 metres). Frequency refers to the number of cycles of a wave passing a fixed point per unit of time. Frequency is normally measured in hertz (Hz), equivalent to one cycle per second and various multiples of hertz. Wavelength and frequency are related by the following formula: 𝑐 = λ𝑣 where; λ = wavelength (m) v = frequency (cycles per second, Hz) c=speed of light (3.8 x 108 m/s) Therefore, the two are inversely related to each other. The shorter the wavelength, the higher the frequency. Understanding the characteristics of electromagnetic radiation in terms of their wavelength and frequency is crucial to understanding the information to be extracted from remote sensing data. 1.4 THE ELECTROMAGNETIC SPECTRUM The electromagnetic spectrum ranges from the shorter wavelengths (including gamma and x- rays) to the longer wavelengths (including microwaves and broadcast radio waves). There are several regions of the electromagnetic spectrum which are useful for remote sensing (Fig. 1.3). Figure 1.3 Electromagnetic Spectrum 12 | P a g e Figure 1.4 Detailed Electromagnetic Spectrum illustrating Wavelength and Frequency For most purposes, the ultraviolet or UV portion of the spectrum has the shortest wavelengths that are practical for remote sensing. This radiation is just beyond the violet portion of the visible wavelengths, hence its name (Fig. 1.4). Some earth surface materials, primarily rocks and minerals emit visible light when illuminated by UV radiation. Figure 1.5 UV Portion of the Electromagnetic Spectrum 13 | P a g e The light which our eyes - our "remote sensors" - can detect is part of the visible spectrum. It is important to recognize how small the visible portion is relative to the rest of the spectrum. There is a lot of radiation around us which is "invisible" to our eyes, but can be detected by other remote sensing instruments and used to our advantage. The visible wavelengths cover a range from approximately 0.4 to 0.7 μm. The longest visible wavelength is red and the shortest is violet. Common wavelengths of what we perceive as particular colours from the visible portion of the spectrum are listed below. It is important to note that this is the only portion of the spectrum we can associate with the concept of colours. Violet: 0.4 - 0.446 μm Green: 0.500 - 0.578 μm Orange: 0.592 - 0.620 μm Blue: 0.446 - 0.500 μm Yellow: 0.578 - 0.592 μm Red: 0.620 - 0.7 μm Blue, green and red are the primary colours or wavelengths of the visible spectrum. They are defined as such because no single primary colour can be created from the other two, but all other colours can be formed by combining blue, green and red in various proportions. Although we see sunlight as a uniform or homogeneous colour, it is actually composed of various wavelengths of radiation in primarily the ultraviolet, visible and infrared portions of the spectrum. The visible portion of this radiation can be shown in its component colours when sunlight is passed through a prism, which bends the light in differing mounts according to wavelength (Fig.1.5). Figure 1.6 Prism The next portion of the spectrum of interest is the infrared (IR) region which covers the wavelength range from approximately 0.7 μm to 100 μm - more than 100 times as wide as the visible portion. 14 | P a g e Figure 1.7 IR Portion of the Electromagnetic Spectrum The infrared region (Fig. 1.6) can be divided into two categories based on their radiation properties - the reflected IR and the emitted or thermal IR. Radiation in the reflected IR region is used for remote sensing purposes in ways very similar to radiation in the visible portion. The reflected IR covers wavelengths from approximately 0.7 μm to 3.0 μm. The thermal IR region is quite different from the visible and reflected IR portions, as this energy is essentially the radiation that is emitted from the Earth's surface in the form of heat. The thermal IR covers wavelengths from approximately 3.0 μm to 100 μm. The portion of the spectrum of more recent interest to remote sensing is the microwave region from about 1 mm to 1 m (Fig. 1.7). This covers the longest wavelengths used for remote sensing. The shorter wavelengths have properties similar to the thermal infrared region while the longer wavelengths approach the wavelengths used for radio broadcasts. 15 | P a g e Figure 1.8 Microwave Portion of the Electromagnetic Spectrum 1.5 INTERACTIONS WITH THE ATMOSPHERE Before radiation used for remote sensing reaches the Earth's surface it has to travel through some distance of the Earth's atmosphere. Particles and gases in the atmosphere can affect the incoming light and radiation. These effects are caused by the mechanisms of scattering and absorption. 1.5.1 Scattering Scattering occurs when particles or large gas molecules present in the atmosphere interact with and cause the electromagnetic radiation to be redirected from its original path. How much scattering takes place depends on several factors including the wavelength of the radiation, the abundance of particles or gases and the distance the radiation travels through the atmosphere. There are three (3) types of scattering which take place. Rayleigh scattering occurs when particles are very small compared to the wavelength of the radiation. These could be particles such as small specks of dust or nitrogen and oxygen molecules. Rayleigh scattering causes shorter wavelengths of energy to be scattered much more than longer wavelengths. Rayleigh scattering is the dominant scattering mechanism in 16 | P a g e the upper atmosphere. The fact that the sky appears "blue" during the day is because of this phenomenon. As sunlight passes through the atmosphere, the shorter wavelengths (i.e. blue) of the visible spectrum are scattered more than the other (longer) visible wavelengths. At sunrise and sunset the light has to travel farther through the atmosphere than at midday and the scattering of the shorter wavelengths is more complete; this leaves a greater proportion of the longer wavelengths to penetrate the atmosphere. Mie scattering occurs when the particles are just about the same size as the wavelength of the radiation. Dust, pollen, smoke and water vapour are common causes of Mie scattering which tends to affect longer wavelengths than those affected by Rayleigh scattering. Mie scattering occurs mostly in the lower portions of the atmosphere where larger particles are more abundant and dominates when cloud conditions are overcast. The final scattering mechanism of importance is called non-selective scattering. This occurs when the particles are much larger than the wavelength of the radiation. Water droplets and large dust particles can cause this type of scattering. Non-selective scattering gets its name from the fact that all wavelengths are scattered about equally. This type of scattering causes fog and clouds to appear white to our eyes because blue, green and red light are all scattered in approximately equal quantities (blue+green+red light = white light). 1.5.2 Absorption Absorption is the other main mechanism at work when electromagnetic radiation interacts with the atmosphere. In contrast to scattering, this phenomenon causes molecules in the atmosphere to absorb energy at various wavelengths. Ozone, carbon dioxide and water vapour are the three main atmospheric constituents which absorb radiation. Ozone absorbs the harmful (to most living things) ultraviolet radiation from the sun. Without this protective layer in the atmosphere our skin would burn when exposed to sunlight. You may have heard carbon dioxide referred to as a greenhouse gas. This is because it tends to absorb radiation strongly in the far infrared portion of the spectrum - that area associated with thermal heating - which serves to trap this heat inside the atmosphere. Water vapour in the atmosphere absorbs much of the incoming long wave infrared and shortwave microwave radiation (between 22 μm and 1m). The presence of water vapour in the lower atmosphere 17 | P a g e varies greatly from location to location and at different times of the year. For example, the air mass above a desert would have very little water vapour to absorb energy, while the tropics would have high concentrations of water vapour (i.e. high humidity). Figure 1.9 Energy sources and Atmospheric Transmittance Because these gases absorb electromagnetic energy in very specific regions of the spectrum, they influence where (in the spectrum) we can "look" for remote sensing purposes. Those areas of the spectrum which are not severely influenced by atmospheric absorption and thus, are useful to remote sensors, are called atmospheric windows. By comparing the characteristics of the two most common energy/radiation sources (the sun and the earth) with the atmospheric windows available to us, we can define those wavelengths that we can use most effectively for remote sensing. The visible portion of the spectrum, to which our eyes are most sensitive, corresponds to both an atmospheric window and the peak energy level of the sun. Note also that heat energy emitted by the Earth corresponds to a window around 10 μm in the thermal IR portion of the spectrum, while the large window at wavelengths beyond 1 mm is associated with the microwave region. 1.6 RADIATION - TARGET INTERACTIONS Radiation that is not absorbed or scattered in the atmosphere can reach and interact with the Earth's surface. There are three (3) forms of interaction that can take place when energy strikes or is incident (I) upon the surface. These are: absorption (A); transmission (T); and reflection (R) (Fig. 1.9). The total incident energy will interact with the surface in one or more of these 18 | P a g e three ways. The proportions of each will depend on the wavelength of the energy and the material and condition of the feature. Figure 1.10 Radiation - Target Interactions Absorption (A) occurs when radiation (energy) is absorbed into the target while transmission (T) occurs when radiation passes through a target. Reflection (R) occurs when radiation "bounces" off the target and is redirected. In remote sensing, we are most interested in measuring the radiation reflected from targets. We refer to two types of reflection, which represent the two extreme ends of the way in which energy is reflected from a target: secular reflection and diffuse reflection. When a surface is smooth we get secular or mirror-like reflection where all (or almost all) of the energy is directed away from the surface in a single direction. Diffuse reflection occurs when the surface is rough and the energy is reflected almost uniformly in all directions. Most earth surface features live somewhere between perfectly secular or perfectly diffuse reflectors. Whether a particular target reflects secularly or diffusely or somewhere in between, depends on the surface roughness of the feature in comparison to the wavelength of the incoming radiation. If the wavelengths are much smaller than the surface variations or the particle sizes that make up the surface, diffuse reflection will dominate. For example, fine grained sand would appear fairly smooth to long wavelength microwaves but would appear quite rough to the visible wavelengths. 1.7 PASSIVE VERSUS ACTIVE SENSING The sun provides a very convenient source of energy for remote sensing. The sun's energy is either reflected, as it is for visible wavelengths or absorbed and then reemitted, as it is for thermal infrared wavelengths. Remote sensing systems which measure energy that is naturally available are called passive sensors. Passive sensors can only be used to detect energy when the naturally occurring energy is available. For all reflected energy, this can only take place during the time when the sun is illuminating the Earth. There is no reflected energy available 19 | P a g e from the sun at night. Energy that is naturally emitted (such as thermal infrared) can be detected day or night, as long as the amount of energy is large enough to be recorded. Active sensors, on the other hand, provide their own energy source for illumination. The sensor emits radiation which is directed toward the target to be investigated. The radiation reflected from that target is detected and measured by the sensor. Advantages for active sensors include the ability to obtain measurements anytime, regardless of the time of day or season. Active sensors can be used for examining wavelengths that are not sufficiently provided by the sun, such as microwaves or to better control the way a target is illuminated. However, active systems require the generation of a fairly large amount of energy to adequately illuminate targets. An example of an active sensor is synthetic aperture radar (SAR). 20 | P a g e 2. SATELLITES AND SENSORS 2.1 INTRODUCTION In order for a sensor to collect and record energy reflected or emitted from a target or surface, it must reside on a stable platform removed from the target or surface being observed. Platforms for remote sensors may be situated on the ground, on an aircraft or balloon (or some other platform within the Earth's atmosphere) or on a spacecraft or satellite outside of the Earth's atmosphere. Ground-based sensors are often used to record detailed information about the surface which is compared with information collected from aircraft or satellite sensors. In some cases, this can be used to better characterize the target which is being imaged by these other sensors, making it possible to better understand the information in the imagery. Sensors may be placed on a ladder, scaffolding, tall building, crane, etc. Aerial platforms are primarily stable wing aircraft, although helicopters are occasionally used. Aircraft are often used to collect very detailed images and facilitate the collection of data over virtually any portion of the Earth's surface at any time. In space, remote sensing is sometimes conducted from the space shuttle or, more commonly, from satellites. Satellites are objects which revolve around another object - in this case, the Earth. For example, the moon is a natural satellite, whereas man-made satellites include those platforms launched for remote sensing, communication and telemetry (location and navigation) purposes. Because of their orbits, satellites permit repetitive coverage of the Earth's surface on a continuing basis. Cost is often a significant factor in choosing among the various platform options. 2.2 SATELLITE CHARACTERISTICS: ORBITS AND SWATHS Although ground-based and aircraft platforms may be used, satellites provide a great deal of the remote sensing imagery commonly used today. Satellites have several unique characteristics which make them particularly useful for remote sensing of the Earth's surface. The path followed by a satellite is referred to as its orbit. Satellite orbits are matched to the capability and objective of the sensor(s) they carry. Orbit selection can vary in terms of altitude (their height above the Earth's surface) and their orientation and rotation relative to the Earth. Satellites at very high altitudes, which view the same portion of the Earth's surface at all times have geostationary orbits. These geostationary satellites, at altitudes of approximately 36,000 km, revolve at speeds which match the rotation of the Earth so they seem stationary, relative 21 | P a g e to the Earth's surface. This allows the satellites to observe and collect information continuously over specific areas. Weather and communications satellites commonly have these types of orbits. Due to their high altitude, some geostationary weather satellites can monitor weather and cloud patterns covering an entire hemisphere of the Earth. Many remote sensing platforms are designed to follow an orbit (basically north-south) which, in conjunction with the Earth's rotation (west-east), allows them to cover most of the Earth's surface over a certain period of time. These are near polar orbits, so named for the inclination of the orbit relative to a line running between the North and South poles. Many of these satellite orbits are also sun-synchronous such that they cover each area of the world at a constant local time of day called local sun time. At any given latitude, the position of the sun in the sky as the satellite passes overhead will be the same within the same season. This ensures consistent illumination conditions when acquiring images in a specific season over successive years or over a particular area over a series of days. This is an important factor for monitoring changes between images or for mosaic king adjacent images together, as they do not have to be corrected for different illumination conditions. Most of the remote sensing satellite platforms today are in near-polar orbits, which means that the satellite travels northwards on one side of the Earth and then toward the southern pole on the second half of its orbit. These are called ascending and descending passes, respectively. If the orbit is also sun synchronous, the ascending pass is most likely on the shadowed side of the Earth while the descending pass is on the sunlit side. Sensors recording reflected solar energy only image the surface on a descending pass, when solar illumination is available. Active sensors which provide their own illumination or passive sensors that record emitted (e.g. thermal) radiation can also image the surface on ascending passes. As a satellite revolves around the Earth, the sensor "sees" a certain portion of the Earth's surface. The area imaged on the surface, is referred to as the swath. Imaging swaths for space borne sensors generally vary between tens and hundreds of kilometres wide. As the satellite orbits the Earth from pole to pole, its east-west position wouldn't change if the Earth didn't rotate. However, as seen from the Earth, it seems that the satellite is shifting westward because the Earth is rotating (from west to east) beneath it. This apparent movement allows the satellite swath to cover a new area with each consecutive pass. The satellite's orbit and the rotation of the Earth work together to allow complete coverage of the Earth's surface, after it has completed one complete cycle of orbits. 22 | P a g e If we start with any randomly selected pass in a satellite's orbit, an orbit cycle will be completed when the satellite retraces its path, passing over the same point on the Earth's surface directly below the satellite (called the nadir point) for a second time. The exact length of time of the orbital cycle will vary with each satellite. The interval of time required for the satellite to complete its orbit cycle is not the same as the "revisit period". Using steerable sensors, a satellite-borne instrument can view an area (off-nadir) before and after the orbit passes over a target, thus making the 'revisit' time less than the orbit cycle time. The revisit period is an important consideration for a number of monitoring applications, especially when frequent imaging is required (for example, to monitor the spread of an oil spill or the extent of flooding). In near-polar orbits, areas at high latitudes will be imaged more frequently than the equatorial zone due to the increasing overlap in adjacent swaths as the orbit paths come closer together near the poles. 2.3 SPATIAL RESOLUTION AND PIXEL SIZE The detail discernible in an image is dependent on the spatial resolution of the sensor and refers to the size of the smallest possible feature that can be detected. Spatial resolution of passive sensors depends primarily on their Instantaneous Field of View (IFOV). Figure 2.1 Instantaneous Field of View The IFOV is the angular cone of visibility of the sensor (A) and determines the area on the Earth's surface which is "seen" from a given altitude at one particular moment in time (B) (Fig. 2.1). The size of the area viewed is determined by multiplying the IFOV by the distance from the ground to the sensor (H). This area on the ground is called the resolution cell and determines a sensor's maximum spatial resolution. For a homogeneous feature to be detected, its size generally has to be equal to or larger than the resolution cell. If the feature is smaller than this, it may not be detectable as the average brightness of all features in that resolution 23 | P a g e cell will be recorded. However, smaller features may sometimes be detectable if their reflectance dominates within a particular resolution cell allowing sub-pixel or resolution cell detection. Most remote sensing images are composed of a matrix of picture elements or pixels, which are the smallest units of an image. Image pixels are normally square and represent a certain area on an image. It is important to distinguish between pixel size and spatial resolution - they are not interchangeable. If a sensor has a spatial resolution of 20 m and an image from that sensor is displayed at full resolution, each pixel represents an area of 20m x 20m on the ground. In this case the pixel size and resolution are the same. However, it is possible to display an image with a pixel size different than the resolution. Many posters of satellite images of the Earth have their pixels averaged to represent larger areas, although the original spatial resolution of the sensor that collected the imagery remains the same. 2.4 SPECTRAL RESOLUTION Different classes of features and details in an image can often be distinguished by comparing their responses over distinct wavelength ranges. Broad classes, such as water and vegetation, can usually be separated using very broad wavelength ranges - the visible and near infrared. Other more specific classes, such as different rock types, may not be easily distinguishable using either of these broad wavelength ranges and would require comparison at much finer wavelength ranges to separate them. Thus, we would require a sensor with higher spectral resolution. Spectral resolution describes the ability of a sensor to define fine wavelength intervals. The finer the spectral resolution, the narrower the wavelength ranges for a particular channel or band. Figure 2.2 Target (leaf) Interaction with Visible and Infrared Wavelengths 24 | P a g e Black and white film records wavelengths extending over much or all of the visible portion of the electromagnetic spectrum. Its spectral resolution is fairly coarse, as the various wavelengths of the visible spectrum are not individually distinguished and the overall reflectance in the entire visible portion is recorded. Colour film is also sensitive to the reflected energy over the visible portion of the spectrum, but has higher spectral resolution, as it is individually sensitive to the reflected energy at the blue, green and red wavelengths of the spectrum. Thus, it can represent features of various colours based on their reflectance in each of these distinct wavelength ranges. Many remote sensing systems record energy over several separate wavelength ranges at various spectral resolutions. These are referred to as multi-spectral sensors and will be described in some detail in following sections. Advanced multi-spectral sensors called hyperspectral sensors, detect hundreds of very narrow spectral bands throughout the visible, near-infrared and mid-infrared portions of the electromagnetic spectrum. Their very high spectral resolution facilitates fine discrimination between different targets based on their spectral response in each of the narrow bands. 2.5 RADIOMETRIC RESOLUTION While the arrangement of pixels describes the spatial structure of an image, the radiometric characteristics describe the actual information content in an image. Every time an image is acquired on film or by a sensor, its sensitivity to the magnitude of the electromagnetic energy determines the radiometric resolution. The radiometric resolution of an imaging system describes its ability to discriminate very slight differences in energy. The finer the radiometric resolution of a sensor, the more sensitive it is to detecting small differences in reflected or emitted energy. Imagery data are represented by positive digital numbers which vary from 0 to (one less than) a selected power of 2. This range corresponds to the number of bits used for coding numbers in binary format. Each bit records an exponent of power 2 (e.g. 1 bit=2 1=2). The maximum number of brightness levels available depends on the number of bits used in representing the energy recorded. Thus, if a sensor used 8 bits to record the data, there would be 28=256 digital values available, ranging from 0 to 255. However, if only 4 bits were used, then only 24=16 values ranging from 0 to 15 would be available. Thus, the radiometric resolution would be much less. Image data are generally displayed in a range of grey tones, with black representing a digital number of 0 and white representing the maximum value (for example, 25 | P a g e 255 in 8-bit data). By comparing a 2-bit image with an 8-bit image, we can see that there is a large difference in the level of detail discernible depending on their radiometric resolutions. 2.6 TEMPORAL RESOLUTION In addition to spatial, spectral and radiometric resolution, the concept of temporal resolution is also important to consider in a remote sensing system. As already, discussed, revisit period refers to the length of time it takes for a satellite to complete one entire orbit cycle. The revisit period of a satellite sensor is usually several days. Therefore, the absolute temporal resolution of a remote sensing system to image the exact same area at the same viewing angle a second time is equal to this period. However, because of some degree of overlap in the imaging swaths of adjacent orbits for most satellites and the increase in this overlap with increasing latitude, some areas of the Earth tend to be re-imaged more frequently. Also, some satellite systems are able to point their sensors to image the same area between different satellite passes separated by periods from one to five days. Thus, the actual temporal resolution of a sensor depends on a variety of factors, including the satellite/sensor capabilities, the swath overlap and latitude. The ability to collect imagery of the same area of the Earth's surface at different periods of time is one of the most important elements for applying remote sensing data. Spectral characteristics of features may change over time and these changes can be detected by collecting and comparing multi-temporal imagery. For example, during the growing season, most species of vegetation are in a continual state of change and our ability to monitor those subtle changes using remote sensing is dependent on when and how frequently we collect imagery. By imaging on a continuing basis at different times we are able to monitor the changes that take place on the Earth's surface, whether they are naturally occurring (such as changes in natural vegetation cover or flooding) or induced by humans (such as urban development or deforestation). The time factor in imaging is important when: persistent clouds offer limited clear views of the Earth's surface (often in the tropics) short-lived phenomena (floods, oil slicks, etc.) need to be imaged multi-temporal comparisons are required (e.g. the spread of a forest disease from one year to the next) the changing appearance of a feature over time can be used to distinguish it from near similar features (wheat / maize) 26 | P a g e 2.7 MULTISPECTRAL SCANNING Many electronic (as opposed to photographic) remote sensors acquire data using scanning systems, which employ a sensor with a narrow field of view (i.e. IFOV) that sweeps over the terrain to build up and produce a two-dimensional image of the surface. Scanning systems can be used on both aircraft and satellite platforms and have essentially the same operating principles. A scanning system used to collect data over a variety of different wavelength ranges is called a multispectral scanner (MSS) and is the most commonly used scanning system. There are two main modes or methods of scanning employed to acquire multispectral image data - across-track scanning and along-track scanning. Figure 2.3 Across –Track Scanning Across-track scanners scan the Earth in a series of lines. The lines are oriented perpendicular to the direction of motion of the sensor platform (i.e. across the swath). Each line is scanned from one side of the sensor to the other, using a rotating mirror (A). As the platform moves forward over the Earth, successive scans build up a two-dimensional image of the Earth´s surface. The incoming reflected or emitted radiation is separated into several spectral components that are detected independently. The UV, visible, near-infrared and thermal radiation are dispersed into their constituent wavelengths. A bank of internal detectors (B), each sensitive to a specific range of wavelengths, detects and measures the energy for each spectral band and then, as an electrical signal, they are converted to digital data and recorded for subsequent computer processing. The IFOV (C) of the sensor and the altitude of the platform determine the ground resolution cell viewed (D) and thus the spatial resolution. The angular field of view (E) is the sweep of the mirror, measured in degrees, used to record a scan line and determines the width of the imaged swath (F). Airborne scanners typically sweep large angles (between 90º and 120º), while satellites, because of their higher altitude need only to sweep fairly small angles (10 - 20º) to cover a broad region. Because the distance from the 27 | P a g e sensor to the target increases towards the edges of the swath, the ground resolution cells also become larger and introduce geometric distortions to the images. Also, the length of time the IFOV "sees" a ground resolution cell as the rotating mirror scans (called the dwell time), is generally quite short and influences the design of the spatial, spectral and radiometric resolution of the sensor. Figure 2.4 Along-Track Scanning Along-track scanners also use the forward motion of the platform to record successive scan lines and build up a two-dimensional image, perpendicular to the flight direction. However, instead of a scanning mirror, they use a linear array of detectors (A) located at the focal plane of the image (B) formed by lens systems (C), which are "pushed" along in the flight track direction (i.e. along track). These systems are also referred to as pushbroom scanners, as the motion of the detector array is analogous to the bristles of a broom being pushed along a floor. Each individual detector measures the energy for a single ground resolution cell (D) and thus the size and IFOV of the detectors determines the spatial resolution of the system. A separate linear array is required to measure each spectral band or channel. For each scan line, the energy detected by each detector of each linear array is sampled electronically and digitally recorded. Along-track scanners with linear arrays have several advantages over across-track mirror scanners. The array of detectors combined with the pushbroom motion allows each detector to "see" and measure the energy from each ground resolution cell for a longer period of time (dwell time). This allows more energy to be detected and improves the radiometric resolution. The increased dwell time also facilitates smaller IFOVs and narrower bandwidths for each detector. Thus, finer spatial and spectral resolution can be achieved without impacting radiometric resolution. Because detectors are usually solid-state microelectronic devices, they are generally smaller, lighter, require less power and are more reliable and last longer because they have no moving parts. On the other hand, cross-calibrating thousands of detectors to achieve uniform sensitivity across the array are necessary and complicated. 28 | P a g e Regardless of whether the scanning system used is either of these two types, it has several advantages over photographic systems. The spectral range of photographic systems is restricted to the visible and near-infrared regions while MSS systems can extend this range into the thermal infrared. They are also capable of much higher spectral resolution than photographic systems. Multi-band or multispectral photographic systems use separate lens systems to acquire each spectral band. This may cause problems in ensuring that the different bands are comparable both spatially and radiometrically and with registration of the multiple images. MSS systems acquire all spectral bands simultaneously through the same optical system to alleviate these problems. Photographic systems record the energy detected by means of a photochemical process which is difficult to measure and to make consistent. Because MSS data are recorded electronically, it is easier to determine the specific amount of energy measured and they can record over a greater range of values in a digital format. Photographic systems require a continuous supply of film and processing on the ground after the photos have been taken. The digital recording in MSS systems facilitates transmission of data to receiving stations on the ground and immediate processing of data in a computer environment. 2.8 THERMAL IMAGING Many multispectral (MSS) systems sense radiation in the thermal infrared as well as the visible and reflected infrared portions of the spectrum. However, remote sensing of energy emitted from the Earth's surface in the thermal infrared (3 μm to 15 μm) is different than the sensing of reflected energy. Thermal sensors use photo detectors sensitive to the direct contact of photons on their surface, to detect emitted thermal radiation. The detectors are cooled to temperatures close to absolute zero in order to limit their own thermal emissions. Thermal sensors essentially measure the surface temperature and thermal properties of targets. Figure 2.5 Target (Leaf) Interaction with Visible and Infrared Wavelengths 29 | P a g e Thermal imagers are typically across-track scanners (like those described in the previous section) that detect emitted radiation in only the thermal portion of the spectrum. Thermal sensors employ one or more internal temperature references for comparison with the detected radiation, so they can be related to absolute radiant temperature. The data are generally recorded on film and/or magnetic tape and the temperature resolution of current sensors can reach 0.1 °C. For analysis, an image of relative radiant temperatures (a thermogram) is depicted in grey levels, with warmer temperatures shown in light tones and cooler temperatures in dark tones. Imagery which portrays relative temperature differences in their relative spatial locations are sufficient for most applications. Absolute temperature measurements may be calculated but require accurate calibration and measurement of the temperature references and detailed knowledge of the thermal properties of the target, geometric distortions and radiometric effects. Because of the relatively long wavelength of thermal radiation (compared to visible radiation), atmospheric scattering is minimal. However, absorption by atmospheric gases normally restricts thermal sensing to two specific regions - 3 to 5 μm and 8 to 14 μm. Because energy decreases as the wavelength increases, thermal sensors generally have large IFOVs to ensure that enough energy reaches the detector in order to make a reliable measurement. Therefore, the spatial resolution of thermal sensors is usually fairly coarse, relative to the spatial resolution possible in the visible and reflected infrared. Thermal imagery can be acquired during the day or night (because the radiation is emitted not reflected) and is used for a variety of applications such as military reconnaissance, disaster management (forest fire mapping) and heat loss monitoring. 2.9 GEOMETRIC DISTORTION IN IMAGERY Any remote sensing image, regardless of whether it is acquired by a multispectral scanner on board a satellite, a photographic system in an aircraft or any other platform/sensor combination, will have various geometric distortions. This problem is inherent in remote sensing, as we attempt to accurately represent the three-dimensional surface of the Earth as a two-dimensional image. All remote sensing images are subject to some form of geometric distortions, depending on the manner in which the data are acquired. These errors may be due to a variety of factors, including one or more of the following, to name only a few: 1. the perspective of the sensor optics, 2. the motion of the scanning system, 30 | P a g e 3. the motion and (in)stability of the platform, 4. the platform altitude, attitude and velocity, 5. the terrain relief and the curvature and rotation of the Earth. Framing systems, such as cameras used for aerial photography, provide an instantaneous "snapshot" view of the Earth from directly overhead. The primary geometric distortion in vertical aerial photograph is due to relief displacement. Objects directly below the centre of the camera lens (i.e. at the nadir) will have only their tops visible, while all other objects will appear to lean away from the centre of the photo such that their tops and sides are visible. If the objects are tall or are far away from the centre of the photo, the distortion and positional error will be larger. The geometry of along-track scanner imagery is similar to that of an aerial photograph for each scan line as each detector essentially takes a "snapshot" of each ground resolution cell. Geometric variations between lines are caused by random variations in platform altitude and attitude along the direction of flight. Images from across-track scanning systems exhibit two main types of geometric distortion. Figure 2.6 Geometric Distortions in Across-track scanning systems They too exhibit relief displacement (A), similar to aerial photographs, but in only one direction parallel to the direction of scan. There is no displacement directly below the sensor, at nadir. As the sensor scans across the swath, the top and side of objects are imaged and appear to lean away from the nadir point in each scan line. Again, the displacement increases, moving towards the edges of the swath. Another distortion (B) occurs due to the rotation of the scanning optics. As the sensor scans across each line, the distance from the sensor to the ground increases further away from the centre of the swath. Although the scanning mirror rotates at a constant speed, the IFOV of the sensor moves faster (relative to the ground) and scans a larger area as it moves closer to the edges. This effect results in the compression of image features at points away from the nadir and is called tangential scale distortion. 31 | P a g e All images are susceptible to geometric distortions caused by variations in platform stability including changes in their speed, altitude and attitude (angular orientation with respect to the ground) during data acquisition. These effects are most pronounced when using aircraft platforms and are alleviated to a large degree with the use of satellite platforms, as their orbits are relatively stable, particularly in relation to their distance from the Earth. However, the eastward rotation of the Earth, during a satellite orbit causes the sweep of scanning systems to cover an area slightly to the west of each previous scan. The resultant imagery is thus skewed across the image. This is known as skew distortion and is common in imagery obtained from satellite multispectral scanners. The sources of geometric distortion and positional error vary with each specific situation, but are inherent in remote sensing imagery. In most instances, we may be able to remove or at least reduce these errors but they must be taken into account in each instance before attempting to make measurements or extract further information. 32 | P a g e 3. IMAGE ANALYSIS 3.1 INTRODUCTION TO DIGITAL IMAGE PROCESSING In today's world of advanced technology where most remote sensing data are recorded in digital format, virtually all image interpretation and analysis involves some element of digital processing. Digital image processing may involve numerous procedures including formatting and correcting of the data, digital image enhancement to facilitate better visual interpretation or even automated classification of targets and features entirely by computer. In order to process remote sensing imagery digitally, the data must be recorded and available in a digital form suitable for storage on a computer tape or disk. Obviously, the other requirement for digital image processing is a computer system, sometimes referred to as an image analysis system, with the appropriate hardware and software to process the data. Several commercially available software systems have been developed specifically for remote sensing image processing and analysis. Examples include Erdas Imagine and ENVI. Most of the common image processing functions available in image analysis systems can be categorized into the following four categories: 1. Pre-processing 2. Image Enhancement 3. Image Transformation 4. Image Classification and Analysis Pre-processingfunctions involve those operations that are normally required prior to the main data analysis and extraction of information and are generally grouped as radiometric or geometric corrections. Radiometric corrections include correcting the data for sensor irregularities and unwanted sensor or atmospheric noise and converting the data so they accurately represent the reflected or emitted radiation measured by the sensor. Geometric corrections include correcting for geometric distortions due to sensor-earth geometry variations and conversion of the data to real world coordinates (e.g. latitude and longitude) on the Earth's surface. The objective of the second group of image processing functions grouped under the term of image enhancement, is solely to improve the appearance of the imagery to assist in visual interpretation and analysis. Examples of enhancement functions include contrast stretching to 33 | P a g e increase the tonal distinction between various features in a scene and spatial filtering to enhance (or suppress) specific spatial patterns in an image. Image transformations are operations similar in concept to those for image enhancement. However, unlike image enhancement operations which are normally applied only to a single channel of data at a time, image transformations usually involve combined processing of data from multiple spectral bands. Arithmetic operations (i.e. subtraction, addition, multiplication, division) are performed to combine and transform the original bands into "new" images which better display or highlight certain features in the scene. Examples of these operations includes spectral or band ratioing and a procedure called principal components analysis (PCA) which is used to more efficiently represent the information in multichannel imagery. Image classification and analysis operations are used to digitally identify and classify pixels in the data. Classification is usually performed on multi-channel data sets and this process assigns each pixel in an image to a particular class or theme based on statistical characteristics of the pixel brightness values. There are a variety of approaches taken to perform digital classification. The two generic approaches are supervised and unsupervised classification. 3.2 PREPROCESSING Pre-processing operations, sometimes referred to as image restoration and rectification, are intended to correct for sensor- and platform-specific radiometric and geometric distortions of data. Radiometric corrections may be necessary due to variations in scene illumination and viewing geometry, atmospheric conditions and sensor noise and response. Each of these will vary depending on the specific sensor and platform used to acquire the data and the conditions during data acquisition. Also, it may be desirable to convert and/or calibrate the data to known (absolute) radiation or reflectance units to facilitate comparison between data. Variations in illumination and viewing geometry between images (for optical sensors) can be corrected by modelling the geometric relationship and distance between the areas of the Earth's surface imaged, the sun and the sensor. This is often required so as to be able to more readily compare images collected by different sensors at different dates or times or to mosaic multiple images from a single sensor while maintaining uniform illumination conditions from scene to scene. As discussed in Chapter 1, scattering of radiation occurs as it passes through and interacts with the atmosphere. This scattering may reduce or attenuate, some of the energy illuminating the 34 | P a g e surface. In addition, the atmosphere will further attenuate the signal propagating from the target to the sensor. Various methods of atmospheric correction can be applied ranging from detailed modelling of the atmospheric conditions during data acquisition, to simple calculations based solely on the image data. An example of the latter method is to examine the observed brightness values (digital numbers), in an area of shadow or for a very dark object (such as a large clear lake - A) and determine the minimum value (B). The correction is applied by subtracting the minimum observed value, determined for each specific band, from all pixel values in each respective band. Since scattering is wavelength dependent (Chapter 1), the minimum values will vary from band to band. This method is based on the assumption that the reflectance from these features, if the atmosphere is clear, should be very small, if not zero. If we observe values much greater than zero, then they are considered to have resulted from atmospheric scattering. Noise in an image may be due to irregularities or errors that occur in the sensor response and/or data recording and transmission. Common forms of noise include systematic striping or banding and dropped lines. Figure 3.1 Dropped Lines Both of these effects should be corrected before further enhancement or classification is performed. Striping was common in early Landsat MSS data due to variations and drift in the response over time of the six MSS detectors. The "drift" was different for each of the six detectors, causing the same brightness to be represented differently by each detector. The overall appearance was thus a 'striped' effect. The corrective process made a relative correction among the six sensors to bring their apparent values in line with each other. Dropped lines occur when there are systems errors which result in missing or defective data along a scan line. Dropped lines are normally 'corrected' by replacing the line with the pixel values in the line above or below or with the average of the two. For many quantitative applications of remote sensing data, it is necessary to convert the digital numbers to measurements in units which represent the actual reflectance or emittance from the surface. This is done based on detailed knowledge of the sensor response and the way in which the analog signal (i.e. the reflected or emitted radiation) is converted to a digital number, 35 | P a g e called analog-to-digital (A-to-D) conversion. By solving this relationship in the reverse direction, the absolute radiance can be calculated for each pixel, so that comparisons can be accurately made over time and between different sensors. All remote sensing imagery are inherently subject to geometric distortions. These distortions may be due to several factors, including: the perspective of the sensor optics; the motion of the scanning system; the motion of the platform; the platform altitude, attitude and velocity; the terrain relief; and, the curvature and rotation of the Earth. Geometric corrections are intended to compensate for these distortions so that the geometric representation of the imagery will be as close as possible to the real world. Many of these variations are systematic or predictable in nature and can be accounted for by accurate modelling of the sensor and platform motion and the geometric relationship of the platform with the Earth. Other unsystematic or random, errors cannot be modelled and corrected in this way. Therefore, geometric registration of the imagery to a known ground coordinate system must be performed. The geometric registration process involves identifying the image coordinates (i.e. row, column) of several clearly discernible points, called ground control points (or GCPs), in the distorted image (A - A1 to A4) and matching them to their true positions in ground coordinates (e.g. latitude, longitude). The true ground coordinates are typically measured from a map (B - B1 to B4), either in paper or digital format. This is image-to-map registration. Figure 3.2 Geometric Registration Process Once several well-distributed GCP pairs have been identified, the coordinate information is processed by the computer to determine the proper transformation equations to apply to the original (row and column) image coordinates to map them into their new ground coordinates. Geometric registration may also be performed by registering one (or more) images to another image, instead of to geographic coordinates. This is called image-to-image registration and is often done prior to performing various image transformation procedures. 36 | P a g e Figure 3.3 Resampling In order to actually geometrically correct the original distorted image, a procedure called resampling is used to determine the digital values to place in the new pixel locations of the corrected output image. The resampling process calculates the new pixel values from the original digital pixel values in the uncorrected image. There are three common methods for resampling: nearest neighbour, bilinear interpolation and cubic convolution. Nearest neighbour resampling uses the digital value from the pixel in the original image which is nearest to the new pixel location in the corrected image. This is the simplest method and does not alter the original values, but may result in some pixel values being duplicated while others are lost. This method also tends to result in a disjointed or blocky image appearance. Bilinear interpolation resampling takes a weighted average of four pixels in the original image nearest to the new pixel location. The averaging process alters the original pixel values and creates entirely new digital values in the output image. This may be undesirable if further processing and analysis, such as classification based on spectral response, is to be done. If this is the case, resampling may best be done after the classification process. Figure 3.4 Bilinear Interpolation Cubic convolution resampling goes even further to calculate a distance weighted average of a block of sixteen pixels from the original image which surround the new output pixel location. 37 | P a g e As with bilinear interpolation, this method results in completely new pixel values. However, these two methods both produce images which have a much sharper appearance and avoid the blocky appearance of the nearest neighbour method. Figure 3.5 Cubic Convolution 3.3 IMAGE ENHANCEMENT Enhancements are used to make it easier for visual interpretation and understanding of imagery. The advantage of digital imagery is that it allows us to manipulate the digital pixel values in an image. Although radiometric corrections for illumination, atmospheric influences and sensor characteristics may be done prior to distribution of data to the user, the image may still not be optimized for visual interpretation. Remote sensing devices, particularly those operated from satellite platforms, must be designed to cope with levels of target/background energy which are typical of all conditions likely to be encountered in routine use. With large variations in spectral response from a diverse range of targets (e.g. forest, deserts, water, etc.) no generic radiometric correction could optimally account for and display the optimum brightness range and contrast for all targets. Thus, for each application and each image, a custom adjustment of the range and distribution of brightness values is usually necessary. In raw imagery, the useful data often populates only a small portion of the available range of digital values (commonly 8 bits or 256 levels). Contrast enhancement involves changing the original values so that more of the available range is used, thereby increasing the contrast between targets and their backgrounds. The key to understanding contrast enhancements is to understand the concept of an image histogram. A histogram is a graphical representation of the brightness values that comprise an image. The brightness values (i.e. 0-255) are displayed along the x-axis of the graph. The frequency of occurrence of each of these values in the image is shown on the y-axis. By manipulating the range of digital values in an image, graphically represented by its histogram, we can apply various enhancements to the data. There are many different 38 | P a g e techniques and methods of enhancing contrast and detail in an image; we will cover only a few common ones here. The simplest type of enhancement is a linear contrast stretch. This involves identifying lower and upper bounds from the histogram (usually the minimum and maximum brightness values in the image) and applying a transformation to stretch this range to fill the full range. In our example, the minimum value (occupied by actual data) in the histogram is 84 and the maximum value is 153. These 70 levels occupy less than one-third of the full 256 levels available. A linear stretch uniformly expands this small range to cover the full range of values from 0 to 255. This enhances the contrast in the image with light toned areas appearing lighter and dark areas appearing darker, making visual interpretation much easier. This graphic illustrates the increase in contrast in an image before (left) and after (right) a linear contrast stretch. A uniform distribution of the input range of values across the full range may not always be an appropriate enhancement, particularly if the input range is not uniformly distributed. In this case, a histogram-equalized stretch may be better. This stretch assigns more display values (range) to the frequently occurring portions of the histogram. In this way, the detail in these areas will be better enhanced relative to those areas of the original histogram where values occur less frequently. In other cases, it may be desirable to enhance the contrast in only a specific portion of the histogram. For example, suppose we have an image of the mouth of a river and the water portions of the image occupy the digital values from 40 to 76 out of the entire image histogram. If we wished to enhance the detail in the water, perhaps to see variations in sediment load, we could stretch only that small portion of the histogram represented by the water (40 to 76) to the full grey level range (0 to 255). All pixels below or above these values would be assigned to 0 and 255, respectively and the detail in these areas would be lost. However, the detail in the water would be greatly enhanced. Spatial filtering encompasses another set of digital processing functions which are used to enhance the appearance of an image. Spatial filters are designed to highlight or suppress specific features in an image based on their spatial frequency. Figure 3.6 Spatial Filtering 39 | P a g e Spatial frequency refers to the frequency of the variations in tone that appear in an image. "Rough" textured areas of an image, where the changes in tone are abrupt over a small area, have high spatial frequencies, while "smooth" areas with little variation in tone over several pixels, have low spatial frequencies. A common filtering procedure involves moving a 'window' of a few pixels in dimension (e.g. 3x3, 5x5, etc.) over each pixel in the image, applying a mathematical calculation using the pixel values under that window and replacing the central pixel with the new value. The window is moved along in both the row and column dimensions one pixel at a time and the calculation is repeated until the entire image has been filtered and a "new" image has been generated. By varying the calculation performed and the weightings of the individual pixels in the filter window, filters can be designed to enhance or suppress different types of features. A low-pass filter is designed to emphasize larger, homogeneous areas of similar tone and reduce the smaller detail in an image. Thus, low-pass filters generally serve to smooth the appearance of an image. Average and median filters, often used for radar imagery, are examples of low-pass filters. High-pass filters do the opposite and serve to sharpen the appearance of fine detail in an image. One implementation of a high-pass filter first applies a low-pass filter to an image and then subtracts the result from the original, leaving behind only the high spatial frequency information. Directional or edge detection filters are designed to highlight linear features, such as roads or field boundaries. These filters can also be designed to enhance features which are oriented in specific directions. These filters are useful in applications such as geology, for the detection of linear geologic structures. 3.4 IMAGE TRANSFORMATIONS Image transformations typically involve the manipulation of multiple bands of data, whether from a single multispectral image or from two or more images of the same area acquired at different times (i.e. multi-temporal image data). Either way, image transformations generate "new" images from two or more sources which highlight particular features or properties of interest, better than the original input images. Basic image transformations apply simple arithmetic operations to the image data. Image subtraction is often used to identify changes that have occurred between images collected on different dates. 40 | P a g e Figure 3.7 Image Subtraction Typically, two images which have been geometrically registered (see section 4.4), are used with the pixel (brightness) values in one image (1) being subtracted from the pixel values in the other (2). Scaling the resultant image (3) by adding a constant (127 in this case) to the output values will result in a suitable 'difference' image. In such an image, areas where there has been little or no change (A) between the original images, will have resultant brightness values around 127 (mid-grey tones), while those areas where significant change has occurred (B) will have values higher or lower than 127 – brighter or darker depending on the 'direction' of change in reflectance between the two images. This type of image transform can be useful for mapping changes in urban development around cities and for identifying areas where deforestation is occurring, as in this example. Image division or spectral ratioing is one of the most common transforms applied to image data. Image ratioing serves to highlight subtle variations in the spectral responses of various surface covers. By ratioing the data from two different spectral bands, the resultant image enhances variations in the slopes of the spectral reflectance curves between the two different spectral ranges that may otherwise be masked by the pixel brightness variations in each of the bands. The following example illustrates the concept of spectral ratioing. Healthy vegetation reflects strongly in the near-infrared portion of the spectrum while absorbing strongly in the visible red. Other surface types, such as soil and water, show near equal reflectances in both the near-infrared and red portions. Thus, a ratio image of Landsat MSS Band 7 (Near-Infrared - 0.8 to 1.1 mm) divided by Band 5 (Red - 0.6 to 0.7 mm) would result in ratios much greater than 1.0 for vegetation and ratios around 1.0 for soil and water. Thus the discrimination of vegetation from other surface cover types is significantly enhanced. Also, we may be better able to identify areas of unhealthy or stressed vegetation, which show low near-infrared reflectance, as the ratios would be lower than for healthy green vegetation. Another benefit of spectral ratioing is that, because we are looking at relative values (i.e. ratios) instead of absolute brightness values, variations in scene illumination as a result of topographic effects are reduced. Thus, although the absolute reflectances for forest covered slopes may 41 | P a g e vary depending on their orientation relative to the sun's illumination, the ratio of their reflectances between the two bands should always be very similar. More complex ratios involving the sums of and differences between spectral bands for various sensors, have been developed for monitoring vegetation conditions. One widely used image transform is the Normalized Difference Vegetation Index (NDVI) which has been used to monitor vegetation conditions on continental and global scales using the Advanced Very High Resolution Radiometer (AVHRR) sensor onboard the NOAA series of satellites. Different bands of multispectral data are often highly correlated and thus contain similar information. For example, Landsat MSS Bands 4 and 5 (green and red, respectively) typically have similar visual appearances since reflectances for the same surface cover types are almost equal. Image transformation techniques based on complex processing of the statistical characteristics of multi-band data sets can be used to reduce this data redundancy and correlation between bands. One such transform is called principal components analysis. The objective of this transformation is to reduce the dimensionality (i.e. the number of bands) in the data and compress as much of the information in the original bands into fewer bands. The "new" bands that result from this statistical procedure are called components. This process attempts to maximize (statistically) the amount of information (or variance) from the original data into the least number of new components. As an example of the use of principal components analysis, a seven band Thematic Mapper (TM) data set may be transformed such that the first three principal components contain over 90 percent of the information in the original seven bands. Interpretation and analysis of these three bands of data, combining them either visually or digitally, is simpler and more efficient than trying to use all of the original seven bands. Principal components analysis and other complex transforms, can be used either as an enhancement technique to improve visual interpretation or to reduce the number of bands to be used as input to digital classification procedures, discussed in the next section. 3.5 IMAGE CLASSIFICATION AND ANALYSIS Figure 3.8 Image Classification 42 | P a g e A human analyst attempting to classify features in an image uses the elements of visual interpretation to identify homogeneous groups of pixels which represent various features or land cover classes of interest. Digital image classification uses the spectral information represented by the digital numbers in one or more spectral bands and attempts to classify each individual pixel based on this spectral information. This type of classification is termed spectral pattern recognition. In either case, the objective is to assign all pixels in the image to particular classes or themes (e.g. water, coniferous forest, deciduous forest, corn, wheat, etc.). The resulting classified image is comprised of a mosaic of pixels, each of which belong to a particular theme and is essentially a thematic "map" of the original image. When talking about classes, we need to distinguish between information classes and spectral classes. Information classes are those categories of interest that the analyst is actually trying to identify in the imagery, such as different kinds of crops, different forest types or tree species, different geologic units or rock types, etc. Spectral classes are groups of pixels that are uniform (or near-similar) with respect to their brightness values in the different spectral channels of the data. The objective is to match the spectral classes in the data to the information classes of interest. Rarely is there a simple one-to-one match between these two types of classes. Rather, unique spectral classes may appear which do not necessarily correspond to any information class of particular use or interest to the analyst. Alternatively, a broad information class (e.g. forest) may contain a number of spectral sub-classes with unique spectral variations. Using the forest example, spectral sub-classes may be due to variations in age, species and density or perhaps as a result of shadowing or variations in scene illumination. It is the analyst's job to decide on the utility of the different spectral classes and their correspondence to useful information classes. Common classification procedures can be broken down into two broad subdivisions based on the method used: supervised classification and unsupervised classification. In a supervised classification, the analyst identifies in the imagery homogeneous representative samples of the different surface cover types (information classes) of interest. These samples are referred to as training areas. The selection of appropriate training areas is based on the analyst's familiarity with the geographical area and their knowledge of the actual surface cover types present in the image. Thus, the analyst is "supervising" the categorization of a set of specific classes. The numerical information in all spectral bands for the pixels comprising these areas are used to "train" the computer to recognize spectrally similar areas for each class. The 43 | P a g e computer uses a special program or algorithm (of which there are several variations), to determine the numerical "signatures" for each training class. Once the computer has determined the signatures for each class, each pixel in the image is compared to these signatures and labelled as the class it most closely "resembles" digitally. Thus, in a supervised classification we are first identifying the information classes which are then used to determine the spectral classes which represent them. Unsupervised classification in essence reverses the supervised classification process. Spectral classes are grouped first, based solely on the numerical information in the data and are then matched by the analyst to information classes (if possible). Programs, called clustering algorithms, are used to determine the natural (statistical) groupings or structures in the data. Usually, the analyst specifies how many groups or clusters are to be looked for in the data. In addition to specifying the desired number of classes, the analyst may also specify parameters related to the separation distance among the clusters and the variation within each cluster. The final result of this iterative clustering process may result in some clusters that the analyst will want to subsequently combine or clusters that should be broken down further - each of these requiring a further application of the clustering algorithm. Thus, unsupervised classification is not completely without human intervention. However, it does not start with a pre-determined set of classes as in a supervised classification. 3.6 DATA INTEGRATION AND ANALYSIS In the early days of analog remote sensing when the only remote sensing data source was aerial photography, the capability for integration of data from different sources was limited. Today, with most data available in digital format from a wide array of sensors, data integration is a common method used for interpretation and analysis. Data integration fundamentally involves the combining or merging of data from multiple sources in an effort to extract better and/or more information. This may include data that are multi-temporal, multi-resolution, multi-sensor or multi-data type in nature. Imagery collected at different times is integrated to identify areas of change. Multi-temporal change detection can be achieved through simple methods such as these or by other more complex approaches such as multiple classification comparisons or classifications using integrated multi-temporal data sets. Multi-resolution data merging is useful for a variety of applications. The merging of data of a higher spatial resolution with data of lower resolution can significantly sharpen the spatial detail in an image and enhance the discrimination of 44 | P a g e features. SPOT data are well suited to this approach as the 10 m panchromatic data can be easily merged with the 20 metre multispectral data. Additionally, the multispectral data serve to retain good spectral resolution while the panchromatic data provide the improved spatial resolution. Data from different sensors may also be merged, bringing in the concept of multi-sensor data fusion. An excellent example of this technique is the combination of multispectral optical data with radar imagery. These two diverse spectral representations of the surface can provide complementary information. The optical data provide detailed spectral information useful for discriminating between surface cover types, while the radar imagery highlights the structural detail in the image. Applications of multi-sensor data integration generally require that the data be geometrically registered, either to each other or to a common geographic coordinate system or map base. This also allows other ancillary (supplementary) data sources to be integrated with the remote sensing data. For example, elevation data in digital form, called Digital Elevation or Digital Terrain Models (DEMs/DTMs), may be combined with remote sensing data for a variety of purposes. DEMs/DTMs may be useful in image classification, as effects due to terrain and slope variability can be corrected, potentially increasing the accuracy of the resultant classification. DEMs/DTMs are also useful for generating three-dimensional perspective views by draping remote sensing imagery over the elevation data, enhancing visualization of the area imaged. Combining data of different types and from different sources, such as we have described above, is the pinnacle of data integration and analysis. In a digital environment where all the data sources are geometrically registered to a common geographic base, the potential for information extraction is extremely wide. This is the concept for analysis within a digital Geographical Information System (GIS) database. Any data source which can be referenced spatially can be used in this type of environment. A DEM/DTM is just one example of this kind of data. Other examples could include digital maps of soil type, land cover classes, forest species, road networks and many others, depending on the application. The results from a classification of a remote sensing data set in map format, could also be used in a GIS as another data source to update existing map data. In essence, by analysing diverse data sets 45 | P a g e together, it is possible to extract better and more accurate information in a synergistic manner than by using a single data. 46 | P a g e REFERENCES AND RECOMMENDED READING Anderson, J.R., E.E. Hardy, J.T. Roach and R.E. Witmer, (2001), A land use and land cover classification system for use with remote sensor data. Geological Survey Professional Paper 964, United States Government Printing Office, Washington, pp: 40. Campbell, J.B. and Wynne, R.H., (2011). Introduction to remote sensing. Guilford Press. Chander, G., B.L. Markham and D.L. Helder, (2009), Summary of current radiometric calibration coefficients for landsat MSS, TM, ETM+ and EO-1 ALI sensors. Remote Sens. Environ., 113: 893-903. Congalton, R. G., & Green, K. (1993). A practical look at the sources of confusion in error matrix generation. Photogrammetric Engineering and Remote Sensing, 59pp. Huth, J., C. Kuenzer, T. Wehrmann, S. Gebhardt, V.Q. Tuan and S. Dech, (2012), Land cover and land use classification with TWOPAC: Towards automated processing for pixel- and object-based image classification. Remote Sens., 4: 2530-2553. Jensen, J.R., (2000), Remote Sensing of the Environment: An Earth Resource Perspective. Prentice-Hall, New Jersey, pp: 181-529. Lillesand, T.M., and Kiefer, R., W., (1994). Remote sensing and image interpretation, John Wiley and Sons, Chichester, 354pp. Mather, P.M., (1987), Computer Processing of Remotely Sensed Images: An Introduction. Wiley and Sons, Chichester, pp. 352. 47 | P a g e APPENDICES Appendix 1: Landsat Spectral Bands 48 | P a g e Appendix 2: Step-by-step Guide to Processing Landsat data to detect Landcover Changes: A Case studies of the Accra and Tema Metropolis Techniques Introduction: Change detection techniques enable us to compare satellite data from different times to assess damage from natural disasters, characterize climatic and seasonal changes to the landscape, and understand the ways in which humans alter the land. In this exercise, you will use Landsat data of Accra and Tema Metropolis to look for changes in vegetation cover over a four-year period. The scenes for this exercise have already been downloaded for you in the data package that comes with this module, along with a shapefile defining the study area. You will be using a Landsat 4 scene from 1991 and a Landsat 7 scene from 2013, so that you can quantify changes over that time period. Objectives: The objective of this practical is to detect land use/land cover changes in the Accra and Tema metropolis within the period 1991 to 2013. 49 | P a g e Methods: The method to adopt for the landcover change of this practical is to detect land use/land cover changes in the Accra Clip Landsat bands 1-5 and 7 to study area Geo-reference of images * Convert Digital Numbers (DNs) to at-sensor spectral radiance (Lλ)