Remote Sensing Image Pre-processing Lecture 3 PDF
Document Details
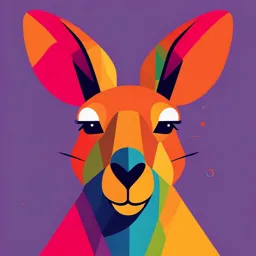
Uploaded by GratifyingMaclaurin
The Hong Kong Polytechnic University
Dr. Zhiwei Li
Tags
Summary
This document is a lecture on remote sensing image pre-processing from the Hong Kong Polytechnic University. The lecture covers various topics such as noise removal, radiometric correction, atmospheric correction, and geometric correction. It also discusses the different types of image distortions.
Full Transcript
LSGI536 Remote Sensing Image Processing Lecture 3 Remote Sensing Image Pre-processing Dr. Zhiwei Li Research Assistant Professor Department of Land Surveying and Geo-Informatics The Hong Kong Polytechnic University Email: [email protected] Outlines 1. Processes of Image Pre-processing 2. Radiom...
LSGI536 Remote Sensing Image Processing Lecture 3 Remote Sensing Image Pre-processing Dr. Zhiwei Li Research Assistant Professor Department of Land Surveying and Geo-Informatics The Hong Kong Polytechnic University Email: [email protected] Outlines 1. Processes of Image Pre-processing 2. Radiometric Pre-processing 3. Geometric Pre-processing 4. Other Pre-processing Processes 2 Section 1 Processes of Image Pre-processing 3 How to collect and exploit Remote Sensing data? Space-borne instruments can only measure the properties of electromagnetic waves emitted, reflected or scattered by the Earth’s surface. Scientists need to understand where these waves originate from, how they interact with the environment, and how they propagate towards the sensor. This is based on understanding the radiation sources and how radiation propagates in the atmosphere so that sensor for measuring radiation can be developed. More precisely, sensors on space platforms are developed that record target scene by receiving photons or EM waves in term of variations in electrical currents or voltages. The electrical variations are immediately converted into digital numbers forming a pixel value in an image. 4 How to collect and exploit Remote Sensing data? The ultimate goal is that users can obtain reliable biogeophysical quantities with respect to the environment such as atmospheric pollution, oceanic currents, crop productivity, etc. However, extract useful and reliable information from remote sensing data is a big challenge. To know the value of the radiation measurements captured by the sensors in space, the next question is How can we derive the bio-geophysical quantities correctly subject to systematic distortion, random error and characteristics of the environment that are responsible for the radiation to reach the sensor? All these are related to pre-processing of remote sensing data. 5 Image pre-processing The goal of pre-processing is to correct distorted (geometric) or degraded (radiometric) image data. to create a more faithful representation of the original image. depict the reality prior to any further enhancement, interpretation, or analysis. 6 Image pre-processing Signal Noise Removal This is mainly related to the senor and its platform and should be removed before applying other corrections. Geometric Correction Due to altitude, orientation and velocity of the platform Earth curvature and rotation. Cloud Screening Radiometric Correction Conversion from DN to radiance, related to sensor sensitivity and calibration. Sun Angle correction apply to images acquired under different solar illumination angles by normalization assuming the sun was at the zenith on each of the sensing. Atmospheric Correction Radiation attenuation due to scattering and absorption May not be necessary for some applications such as classification for single date. But crucial for spectral comparison of image acquired on different dates. 7 After image pre-processing Image enhancement contrast enhancement (make features look more clearly by optimal use of colour) and image filtering (enhance or suppress) specific spatial patterns in an image to improve the appearance of the imagery to assist in visual interpretation, automated feature extraction, and analysis Image classification digitally identify and classify pixels and each objects in the image Data merging/data fusion combine and transform the original bands into “new” images that better display or highlight certain features in the scene, e.g., pan sharpening. 8 Digital Image Processing The process of extracting information from digital images obtained from satellites. Information regarding each pixel is fed into an algorithm and the result of the computation stored for that pixel. Thus, for each image being processed by a particular algorithm there is an input and output image. Order of processing is important. 10 What are tiers? Landsat Collections — What are Tiers? (3 mins) https://www.youtube.com/watch?v=NruC3z4peBc What are the differences between the three tiers? 11 What are tiers? – Real Time All the data are immediately provided after acquisition in less than 12 hours (4-6 hours typically) – nearly in real time. Be useful when monitoring events and natural disasters. Transition from RT to Tier 1 or Tier 2 is 14-26 days delay. 12 What are tiers? – Tier 1 RMSE smaller than or equal to 12 meters Landsat scenes with the highest available data quality are placed into Tier 1 13 What are tiers? – Tier 2 Adhere to the same radiometric standard as Tier 1 scenes Do not meet the Tier 1 geometry specification RMSE higher than 12 meters caused by significant cloud cover and/or insufficient ground control points 14 Section 2 Radiometric Pre-processing 15 Radiometric pre-processing Noise removal Radiometric correction Atmospheric correction Topographic correction 16 Noise removal Noise It is unwanted disturbance in image data that is due to limitations in the sensing, signal digitation, or data recording process. The effects of noise range from a degradation to total masking of the true radiometric information content of the digital image. It may either be systematic (banding of multispectral images) to dropped lines or parts of lines, or random speckle. Critical to the subsequent processing and classification of an image. Produce an image that is as close to the original radiometry of the scene as possible. 17 Example of systematic noise removal Line drop A number of adjacent pixels along a line (or an entire line) may contain spurious DNs. Solution? 18 Example of systematic noise removal Line drop A number of adjacent pixels along a line (or an entire line) may contain spurious DNs. Solution Can be solved by replacing the defective DNs with the average of the values of the pixels occurring in the lines just above and below. Alternatively The DNs from the preceding line can simply be inserted in the defective pixels. 19 Example of random noise removal Bad pixels (bit errors): Detector does not record spectral data for an individual pixel. Such noise is often referred to as being “spikey” in character, and it causes images to have a “salt and pepper” or “snowy” appearance. Solution? 20 Example of random noise removal Bad pixels (bit errors): Detector does not record spectral data for an individual pixel. Such noise is often referred to as being “spikey” in character, and it causes images to have a “salt and pepper” or “snowy” appearance. Solution: Noise can be identified by comparing each pixel in an image with its neighbours. Normally change much more abruptly than true image values. 21 Example of random noise removal The noisy pixel value can then be replaced by the average of its neighbouring values. Moving neighbourhoods or windows of 3 х 3 or 5 x 5 pixels are typically used in such procedures. 22 Stripe noise Sixteen-line frequency noise in a Landsat TM band 2 – Sumatra coastline De-striping is using low pass filter/noise removal filter 23 Radiometric correction Need to calibrate data radiometrically due to: (i) System effects Different sensors and bands convert differently to byte scale Systems have noise on pixel values Systematic differences in the digital numbers, e.g. striping Convert the DN value to Radiance, then to the Top of Atmosphere Reflectance (TOA) (ii) Sun angle and atmospheric effects Scene illumination (time of day, season) Atmospheric scattering and absorption 24 Radiometric correction: DN-to-Radiance conversion Two formulas to convert DNs to radiance Depends on the scene calibration data available in the header file(s) 1. Gain & Bias Method Use Gain and Bias (or Offset) values from the header file 2. Spectral Radiance Scaling method Use the LMin and LMax spectral radiance scaling factors High gain mode is used when the surface brightness is low and low gain mode is used when surface brightness is high. 25 Gain change Anomaly occurs on Landsat-7 A gain change occurs in a scene. Generally this change occurs on the beginning or the end of the scene. Usually, it is splitting the image band into two areas with different brightness. The upper one is brighter than the lower one in the case of the gain switching from high to low gain and vice versa. https://earth.esa.int/c/document_library/get_file?folderId=25717&name=DLFE-522.pdf 26 Landsat 7 ETM+ DN to Radiance LMAXs will ensure accurate conversion to radiance units. Source: Landsat 7 Data Users Handbook 27 Radiometric correction: DN-to-Radiance conversion for Landsat 7 This is spectral specific. A form of radiometric correction is the conversion of the digital numbers to absolute radiance values This is to convert any DN in a particular band to absolute units of spectral radiance in that band if LMAX and LMIN are known from the sensor calibration. 28 Landsat 7 ETM+ DN to Radiance =127 29 Landsat 7 ETM+ DN to Radiance 30 Radiometric correction: Sun angle effects due to seasonal change Radiance to Reflectance In order to normalize the sun angle effect, radiance at sensor’s aperture can be divided by cosine of sun angle from zenith for particular time/location. This is correction to images acquired under different solar illumination angles by normalization, assuming the sun was at the zenith on each of the sensing. 𝐿𝜆 𝐿𝜆⊥ = cos𝜃 𝑠 We can use Top of Atmosphere (TOA) Reflectance, which is a unitless measurement that provides the ratio of radiation reflected to the incident solar radiation on a given surface. It can be computed from satellite measured spectral radiance using the mean solar spectral irradiance and the solar zenith angle. Sun Elevation Angle 𝜋 × 𝐿 × 𝑑2 𝜌𝜆 = 𝐸𝑆𝑈𝑁 ×𝜆 cos𝜃 𝜆 𝑠 where: ρλ = Unitless planetary reflectance (TOA Reflectance) Lλ= spectral radiance received at sensor’s aperture, converted from DN d = Earth-Sun distance in astronomical units (one such a d equals 1.496e+8 km) ESUNλ = mean solar exo-atmospheric irradiances θs = solar zenith angle Effects of Seasonal Changes on Solar Elevation Angle 31 Radiometric correction: Sun angle effects due to seasonal change 32 Radiometric correction: Band 6 Conversion to Temperature Thermal Infrared (TIR) Band Conversion to Temperature 33 Atmospheric Correction The effects of the atmosphere include Radiation attenuation before reaching the ground due to absorption and scattering Increasing the amount of energy reaching the sensor by scattering the radiation (diffuse radiation) Decrease in thermal radiation due to water vapour absorption Atmospheric correction can be handled by 1) Dark object subtraction/Dark pixel method 2) Empirical methods 3) Radiation transfer models/methods Reflected radiance (EI/π) = Incident radiation over pi with the assumption that ground surface is Lambertian surface (radiance is constant across all incident directions in the hemisphere). Reflectance (ρ): Surface reflectance Transmittance (T): Transmission of atmosphere Path Radiance (Lp), which is attributed by atmospheric disturbance, which is spectral specific 34 Atmospheric Correction: Dark object subtraction Assumes that the darkest objects in the image should have a DN of zero and therefore, any response is accounted from path radiance (Lp) with the assumption that it affects the entire scene uniformly. For example: Reflectance of deep clear water is essentially zero in the near-infrared region. Any signal observed over this area represents the path radiance. This value can be subtracted from all pixels in that band. 35 Atmospheric Correction: Dark object subtraction Find the minimum pixel value from each band (using histograms) (minimum vs lowest value that has significant number of counts, may examine dark objects from the entire image not subset of it) Subtract that value from all of the pixels in the band Must be done separately for each band However, this is not always a correct assumption! Because it depends uniformity of the atmosphere over a scene. For example, haze is viewing-angle dependent, as such for extreme viewing angle, it is necessary to normalize the radiance value to nadir position rather than applying dark object subtraction. Overall brightness increases, this reduces image contrast and may due to haze. 70.5°forward 60.0°forward 45.6°forward 0°Nadir 36 Atmospheric Correction: Empirical method Absolute atmospheric correction may also be performed Using empirical line calibration (ELC), which forces the remote sensing image data to match in situ spectral reflectance measurements. Empirical line calibration is based on the equation: Reflectance (field spectrum) = gain x radiance (image) + offset Multi-spectral Ground Calibration Targets (source: link1, link2) 37 Atmospheric Correction: Radiative Transfer Models (RTMs) RTMs simulate the radiative transfer interactions of light scattering and absorption through the atmosphere. These models are typically used for the atmospheric correction of airborne/satellite data and allow for retrieving atmospheric composition. Require parameters of atmospheric condition at the time of image acquisition such as visibility, pressure and so on, can be obtained from local meteorological station. Available numerical models such as LOTRAN, MODTRAN, ATERM, ATCOR and 6S. 38 Atmospheric Correction: Bottom Of Atmosphere Reflectance (BOA) Upon Atmospheric correction on TOA reflectance is applied, Bottom Of Atmosphere (BOA) Reflectance / Surface Reflectance (SR) can be obtained. Look from Space vs See what is on the surface. Sentinel-2 TOA Level-1C image data (left) and associated Level-2A BOA image data (right) [link] 39 Atmospheric Correction Atmospheric Correction in QGIS https://youtu.be/myBn8u9MbjM ~ 6 mins 40 Topographic correction Cosine Correction for Terrain Slope cos o L H = LT cos i where: LH = radiance observed for a horizontal surface (i.e., slope-aspect corrected remote sensor data). LT = radiance observed over sloped terrain (i.e., the raw remote sensor data) 0 = sun’s zenith angle i = sun’s incidence angle in relation to the normal on a pixel 41 Section 3 Geometric Pre-processing 42 Geometric correction Remove geometric distortion so that individual picture elements (pixels) are in their proper planimetric (x, y) map locations. Internal and external geometric error Systematic (predictable) Non-systematic (random) 43 Geometric correction Various geometric distortions: Systematic distortions (predictable) Panoramic distortion Skew distortion due to earth rotation during sweep of IFOV Earth curvature – orbit variation due to ellipsoid After applying geometric correction, you will obtain a geometrically accurate image, registered to a ground coordinate system – georeferenced. 44 Geometric correction Various geometric distortions: Non-systematic (random) Variations in altitude, attitude, and velocity of the sensor platform Variable speed of scanning mirror Atmospheric refraction Relief displacement 45 Reasons to apply geometric correction Using coordinate system Perform accurate distance and area measurements Allow co-registration of images for change detection Mosaics of many image sets Overlay image with GIS data 46 Systematic distortions Panoramic Distortion (or Tangential Scale Distortion) The ground area imaged is proportional to the tangent of the scan angle rather than to the angle itself. Because data are sampled at regular intervals, this produces along-scan distortion. Change in scale at edge of scan (tangential sale distortion) 47 Systematic distortions Panoramic Distortion (or Tangential Scale Distortion) 49 Systematic distortions 50 Systematic distortions Skew Distortion Earth rotates as the sensor scans the terrain. This results in a shift of the ground swath being scanned, meaning each sweep covers an area slightly to the west of the previous weep, causing along-scan distortion. Deskewing involves offsetting each scan line successively to west. Skewed parallelogram appearance of images 51 External distortions Caused by attitude of the sensor or the shape of the object. 52 Terrain relief displacement 53 Geometric distortion due to change in altitude and platform attitude a) Altitude increase: smaller-scale imagery. Decrease: larger-scale imagery. b) An aircraft flies in the x-direction. Roll: Directional stability but the wings move up or down, i.e. they rotate about the x-axis angle (omega: w). Pitch: Wings are stable but the nose or tail moves up or down, i.e., they rotate about they-axis angle (phi: f). Yaw: Wings remain parallel but the fuselage is forced by wind to be oriented some angle to the left or right of the intended line of flight, i.e., it rotates about the z-axis angle (kappa: k). Suffers: combination of changes in altitude and rotation (roll, pitch, and 54 yaw). Correction for geometric distortions Most systematic distortions can be corrected at ground station using mathematical modelling. Most random distortions can be corrected by Ground Control Points (GCPs) identified in the image and register the image to the ground coordinate system (geo-referencing). For image data, geometric correction comprises two parts: geocoding and resampling. 56 Ground Control Points (GCP) Image coordinates specified in i rows and j columns, and Map coordinates (e.g., x, y measured in degrees of latitude and longitude, or meters in a Universal Transverse Mercator (UTM) projection). A location on the surface of the Earth (e.g., a road intersection) that can be identified on the imagery and located accurately on a map. 57 Types of Geometric Correction Two common methods: image-to-map rectification, and image-to-image registration 58 Image to Map Rectification Image-to-map rectification is the process by which the geometry of an image is made planimetric. 59 Image to Image Registration Instead of map, previously rectified image can be used for the rectification. Unrectified image can also be used. 60 Example: transformation equation (affine transformation) Image to Map: X=f1(X’,Y’), Y=f2(X’,Y’) X = aX’ + bY’ + c Y = dX’ + eY’ + f X’, Y’ : image; X, Y : map a, b, c, d, e, f are transformation parameters. To find the 6 parameters, at least 3 control points are required. Inverted to find new map locations for image pixels: x=g1(X’,Y’), y=g2(X’,Y’) The statistical technique of least squares regression is generally used to determine the coefficients for the coordinate transformation equations. 61 Performance evaluation The performance of the transformation often evaluated by the computation of the root-mean-square error (RMS error) for each of the ground control points. Finally the computation of RMSE of the total model, which measures the difference between an estimator/computed value and observed value/control value. The smaller the error the better the model. 62 RMS Error: Example where: xorig and yorig are the original row and column coordinates of the GCP in the image x’ and y’ are the computed or estimated coordinates in the original image when we utilize the six coefficients. the closer these paired values are to one another, the more accurate the algorithm. 63 RMS Error: Example 64 Intensity Interpolation (Resampling) An empty output matrix is created with the desired coordinate. Interpolation needs to transfer brightness value from an x’, y’ location in the original (distorted) to the rectified output image. The practice is commonly referred to as resampling. Three resampling methods are commonly used: Nearest neighbor Bilinear interpolation Cubic convolution 66 Resampling – Nearest-neighbour Substitutes in DN value of the closest pixel. Transfers original pixel brightness values without averaging them. Keeps extremes. Advantages: Keep original values and no averaging. Since original data are retained, this method is recommended before classification. Easy to compute and therefore fastest to use. Disadvantages: Produces a “stair-stepped” effect meaning features may be offset spatially. Data values may be lost, while other values may be duplicated. The brightness value closest to the x’, y’ coordinate is assigned to the output x, y coordinate. 67 Resampling – Bilinear interpolation Advantages: More spatially accurate than nearest neighbour. Stair-step effect reduced, the image looks smooth and less blocky. Still fast to compute. Disadvantages: Alters original data and reduces contrast by averaging neighbouring values together. Computationally more expensive than the nearest neighbour. Distance weighted average of the DN’s of the four closest pixels where Zk are the surrounding four data point values, and D2k are the distances squared from the point in question (x’, y’) to the these data points. 68 Resampling – Cubic convolution Determines the value from the weighted average of the 16 closest pixels to the specified input coordinates and assigns that value to the output coordinates. Advantages: More spatially accurate than nearest neighbor; Stair-step effect reduced, image looks smooth and sharpening as well Disadvantages: Alters original data and reduces contrast by averaging neighbouring values together; Computation takes a longer time when compared with the other methods 69 Nearest neighbour vs Bilinear vs Cubic convolution 70 Section 4 Other Pre-processing Processes 71 Cloud screening Cloud affects information retrieval from image Thick and bright clouds block all optical bands reflected from the Earth’s surface. Optically thin clouds affects retrieval of real reflectance from ground. Clouds over bright surfaces creates confusion for identifying snow, ice, and bright sand. It is difficult to separate between clouds and heavy aerosol loading due to similarities between the spectral reflectance of large aerosol particles (e.g., dust) and clouds. 72 Cloud screening Detection of cloud is spectral dependence, e.g. cirrus band 9 in Landsat 8 Many different algorithms have been developed. OLI bands 4,3,2 composite cirrus band 9 “Landsat 8’s Band 9 (1.360-1.390 µm) provides detection of high-altitude cloud contamination that may not be visible in other spectral bands.” [link] 73 Cloud screening Screening clouds and cloud shadows in optical satellite image False color composite of the four representative Landsat MSS images in the Puerto Rico site and their cloud and shadow masks by ATSA (gray: clear pixels; black: shadows; white: clouds) (Zhu et al., 2018) [link] 74 Cloud removal Remove Clouds in Landsat-8 image using ArcGIS https://youtu.be/vx6VYLm48DQ ~ 5 mins 75 Questions What are the basic processes in pre-processing of image data? List and explain the geometric distortion. What is radiometric correction? List and explain image interpolation methods. Explain the reasons for atmospheric correction by the radiance/energy equation. List and explain methods for atmospheric correction. What is RMSE? 76 End of Lecture 3 79