Data: Types and Collection in Market Research (PDF)
Document Details
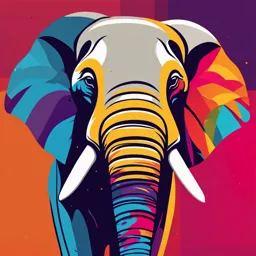
Uploaded by HolyMercury3977
Emlyon Business School
2014
M. Sarstedt and E. Mooi
Tags
Related
- MARKET RESEARCH & B2B B2C 5 PDF
- Tema 4: Investigacion Comercial Y Informacion en Marketing PDF
- Errors and Issues in Secondary Data Used in Marketing Research PDF
- Case Study: Developing a Market Research Plan for EcoSip PDF
- Tema 9. Proceso de Estudio Cuantitativo UAO PDF
- Marketing Communications Research PDF
Summary
This document introduces concepts of data types and collection in market research, including primary and secondary data, quantitative and qualitative data, variables, cases, constructs, and measurement scales. It emphasizes the importance of data for market research and discusses strategies for data collection.
Full Transcript
Data 3 Learning Objectives After reading this chapter you should understand: – How to explain what kind of data you use. – The differences between primary and secondary data. – The differences bet...
Data 3 Learning Objectives After reading this chapter you should understand: – How to explain what kind of data you use. – The differences between primary and secondary data. – The differences between quantitative and qualitative data. – What the unit of analysis is. – When observations are independent and when they are dependent. – The difference between dependent and independent variables. – Different measurement scales and equidistance. – Validity and reliability from a conceptual viewpoint. – How to set up different sampling designs. – How to determine acceptable sample sizes. Keywords Case Construct Data Equidistance Item Measurement scaling Observa- tion Operationalization Primary and secondary data Qualitative and quanti- tative data Reliability Sample sizes Sampling Scale development Validity Variable 3.1 Introduction Data lie at the heart of conducting market research. By data we mean a collection of facts that can be used as a basis for analysis, reasoning, or discussions. Think, for example, of the answers people give to surveys, existing company records, or observations of shoppers’ behaviors. In practice, “good” data are very important because they form the basis for useful market research. In this chapter, we will discuss some of the different types of data. This will help you describe what data you use and why. Subsequently, we discuss strategies to collect data in Chap. 4. M. Sarstedt and E. Mooi, A Concise Guide to Market Research, 25 Springer Texts in Business and Economics, DOI 10.1007/978-3-642-53965-7_3, # Springer-Verlag Berlin Heidelberg 2014 [email protected] 26 3 Data 3.2 Types of Data Before we start discussing data, it is a good idea to introduce some terminology. In the next sections, we will discuss the following four concepts: – Variables, – Constants, – Cases, and – Constructs. A variable is an attribute whose value can change. For example, the price of a product is an attribute of that product and typically varies over time. If the price does not change, it is a constant. Although marketers often talk about variables, they also use the word item, which usually refers to a survey question put to a respondent. A case (or observation) consists of all the observed variables that belong to an object such as a customer, a company or a country. The relationship between variables and cases is that within one case we usually find multiple variables. Table 3.1 includes six variables; type of car bought, age and gender, as well as brand_1, brand_2, and brand_3 which capture statements related to brand trust. In the lower rows, you can see four observations. Table 3.1 Quantitative data Variable Type of car name bought Age Gender Brand_1 Brand_2 Brand_3 This brand’s This brand This brand has a Name of Age in product claims delivers what name that you can Description car bought years Gender are believable it promises trust Customer 1 BMW 328i 29 1 6 5 7 Customer 2 Mercedes 45 0 6 6 6 C180K Customer 3 VW Passat 35 0 7 5 5 2.0 TFSI Customer 4 BMW 61 1 5 4 5 525ix Coding for gender: 0=male, 1=female Coding for brand_1, brand_2, and brand_3: 1=fully disagree, 7=fully agree Another important term that is frequently used in market research is construct, which refers to a variable that is not directly observable (i.e., a latent variable). More precisely, a construct is a latent concept that researchers can define in conceptual terms but cannot measure directly (i.e., the respondent cannot articulate a single response that will totally and perfectly provide a measure of that concept). For example, constructs such as satisfaction, loyalty, or brand trust cannot be measured directly. However, we can measure indicators or manifestations of what we have agreed to call satisfaction, loyalty, or brand trust using several variables (or items). This requires combining these items to form a so called multi-item scale which can be used to measure a construct. Through multiple items, which all imperfectly capture a construct, we can create a measure, which better captures a [email protected] 3.2 Types of Data 27 construct. On the contrary, type of car bought from Table 3.1 is not a construct as this trait is directly observable. For example, we can directly see if a car is a BMW 328i or a Mercedes C180K. Similar to creating constructs, we can create an index of sets of variables. For example, we can create an index of information search activities, which is the sum of the information that customers require from dealers, promotional materials, the Internet, and other sources. This measure of information search activities is also referred to as a composite measure but, unlike a construct, the items in an index define the trait to be measured. For example, the Retail Price Index consists of a “shopping” bag of common retail products multiplied by their price. Unlike a construct, each item in a scale perfectly captures a part of the index. The procedure of combining several items is called scale development, operatio- nalization, or, in the case of an index, index construction. These procedures involve a combination of theory and statistical analysis, such as factor analysis (discussed in Chap. 8) aimed at developing an appropriate measure of a construct. For example, in Table 3.1, brand_1, brand_2, and brand_3 are items that belong to a construct called brand trust (as defined by Erdem and Swait 2004). The construct is not an individual item that you see in the list, but it is captured by calculating the average of a number of related items. Thus, for brand trust, the score for customer 1 is (6 þ 5 þ 7)/3 ¼ 6. But how do we decide which and how many items to use when measuring specific constructs? To answer these questions, market researchers make use of scale development procedures which follow an iterative process with several steps and feedback loops. For example, DeVellis (2011) provides a thorough introduction to scale development. Unfortunately, scale development requires much (technical) expertise. Describing each step goes beyond the scope of this book. However, for many scales you do not need to use this procedure, as existing scales can be found in scale handbooks, such as the Handbook of Marketing Scales by Bearden et al. (2011). Furthermore, marketing and management journals frequently publish research articles that introduce new scales, such as for the reputation of non-profit organizations (for example Sarstedt and Schloderer 2010) or refine existing scales (for example Kuppelwieser and Sarstedt 2014). We introduce two distinctions that are often used to discuss constructs in Box 3.1. Box 3.1 Types of constructs Reflective vs. formative constructs: for reflective constructs, there is a causal relationship from the construct to the items, indicating that the items reflect the construct. Our example on brand trust suggests a reflective construct as the items reflect trust. Thus, if a respondent changes his assessment of brand trust (e.g., because of a negative brand experience), this reflects in the answers to the three items. Reflective constructs typically use multiple items (3 or more) to increase measurement stability and accuracy. If we have multiple items, we can use analysis techniques to inform us about the quality of measurement such as factor or reliability analysis (discussed in Chap. 8). (continued) [email protected] 28 3 Data Box 3.1 (continued) Formative constructs consist of a number of items that define a construct. A typical example is socioeconomic status, which is formed by a combination of education, income, occupation, and residence. If any of these measures increases, socioeconomic status would increase (even if the other items did not change). Conversely, if a person’s socioeconomic status increases, this would not go hand in hand with an increase in all four measures. This distinction is important when operationalizing constructs, as it requires different approaches to decide on the type and number of items. Specifically, reliability analyses (discussed in Chap. 8) cannot be used for formative measures. For an overview of this distinction, see Diamantopoulos and Winklhofer (2001) or Diamantopoulos et al. (2008). Multi-item constructs vs. single-item constructs: Rather than using a large number of items to measure constructs, practitioners often use a single item. For example, we may use only “This brand has a name that you can trust” to measure brand trust instead of all three items. While this is a good way to make the questionnaire shorter, it also reduces the quality of your measures. Generally, you should avoid using single items as they have a pronounced negative impact on your findings. Only in very specific situations is the use of single items justifiable from an empirical perspective. See Diamantopoulos et al. (2012) for a discussion. 3.2.1 Primary and Secondary Data Generally, we can distinguish between two types of data: primary and secondary data. While primary data are data that a researcher has collected for a specific purpose, secondary data are collected by another researcher for another purpose. An example of secondary data is the US Consumer Expenditure Survey (http:// www.bls.gov/cex/), which makes data available on what people in the US buy, such as insurances, personal care items, or food. It also includes the prices people pay for these products and services. Since these data have already been collected, they are secondary data. If a researcher sends out a survey with various questions to find an answer to a specific issue, the collected data are primary data. If primary data are re- used to answer another research question, it becomes secondary data. Secondary data can either be internal or external (or a mix of both). Internal secondary data are data that an organization or individual already has collected, but wants to use for (other) research purposes. For example, we can use sales data to investigate the success of new products, or we can use the warranty claims people make to investigate why certain products are defective. External secondary data are data that other companies, organizations, or individuals have available, sometimes at a cost. [email protected] 3.2 Types of Data 29 Table 3.2 The advantages and disadvantages of secondary and primary data Secondary data Primary data Advantages – Tends to be cheaper – Are recent – Sample sizes tend to be greater – Are specific for – Tend to have more authority the purpose – Are usually quick to access – Are proprietary – Are easier to compare to other research that uses the same data – Are sometimes more accurate (e.g., data on competitors) Disadvantages – May be outdated – Are usually more – May not completely fit the problem expensive – There may be errors hidden in the data – difficult to assess – Take longer to data quality collect – Usually contains only factual data – No control over data collection – May not be reported in the required form (e.g., different units of measurement, definitions, aggregation levels of the data) Secondary and primary data have their own specific advantages and disadvantages, which we illustrate in Table 3.2. Generally, the most important reasons for using secondary data are that they tend to be cheaper and quick to obtain access to (although there can be lengthy processes involved). For example, if you want to have access to the US Consumer Expenditure Survey, all you have to do is point your web browser to http://www.bls.gov/cex/pumdhome.htm and down- load the required files. Furthermore, the authority and competence of some of these research organizations might be a factor. For example, the claim that Europeans spend 9% of their annual income on health may be more believable if it comes from Eurostat (the statistical office of the European Community) than if it came from a single survey conducted through primary research. However, important drawbacks of secondary data are that they may not answer your research question. If you are, for example, interested in the sales of a specific product (and not in a product or service category), the US Expenditure Survey may not help much. In addition, if you are interested in reasons why people buy products, this type of data may not help answer your question. Lastly, as you did not control the data collection, there may be errors in the data. Box 3.2 shows an example of inconsistent results in two well-known surveys on Internet usage. In contrast, primary data tend to be highly specific because the researcher (you!) can influence what the research comprises. In addition, primary research can be carried out when and where it is required and cannot be accessed by competitors. However, gathering primary data often requires much time and effort and, there- fore, is usually expensive compared to secondary data. As a rule, start looking for secondary data first. If they are available, and of acceptable quality, use them! We will discuss ways to gather primary and secondary data in Chap. 4. [email protected] 30 3 Data Box 3.2 Contradictory results in secondary data IAB Europe (http://www.iabeurope.eu) is a trade organization for media companies such as CNN Interactive and Yahoo! Europe focused on interac- tive business. The Mediascope study issued yearly by the IAB provides insight into the European population’s media consumption habits. For exam- ple, according to their 2008 study, 47% of all Germans were online every single day. However, this contradicts the results from the well-known Ger- man General Survey (ALLBUS) issued by the Leibniz Institute for Social Sciences (http://www.gesis.org), according to which merely 26% of Germans used the Internet on a daily basis in 2008. 3.2.2 Quantitative and Qualitative Data Data can be quantitative or qualitative. Quantitative data are presented in values, whereas qualitative data are not. Qualitative data can take many forms such as words, stories, observations, pictures, or audio. The distinction between qualitative and quantitative data is not as black-and-white as it seems, because quantitative data are based on qualitative judgments. For example, the questions on brand trust in Table 3.1 take the values of 1–7. There is no reason why we could not have used other values to code these answers, but it is common practice to code answers of a construct’s items on a range of 1–5 or 1–7. In addition, when data are “raw,” we often label them qualitative data, although researchers can code attributes of the data, thereby turning it into quantitative data. Think, for example, of how people respond to a new product in an interview. We can code this by setting neutral responses to 0, somewhat positive responses to 1, positive responses to 2, and very positive responses to 3. We have thus turned qualitative data into quantitative data. This is also qualitative data’s strength and weakness; qualitative data are very rich but can be interpreted in many different ways. Thus, the process of interpreting qualitative data is subjective. To reduce some of these problems, qualitative data should be coded by (multiple) trained researchers. The distinction between quantitative and qualitative data is closely related to that between quantitative and qualitative research, which we discuss in Box 3.3. Most people think of quantitative data as being more factual and precise than qualitative data, but this is not necessarily true. Rather, what is important is how well qualitative data have been collected and/or coded into quantitative data. 3.3 Unit of Analysis The unit of analysis is the level at which a variable is measured. Researchers often ignore this aspect, but it is crucial because it determines what we can learn from the data. Typical measurement levels include respondents, customers, stores, companies, or countries. It is best to use data at the lowest possible level, because [email protected] 3.3 Unit of Analysis 31 Box 3.3 Quantitative and qualitative research Market researchers often label themselves as either quantitative or qualitative researchers. The two types of researchers use different methodologies, dif- ferent types of data, and focus on different research questions. Most people regard the difference between qualitative and quantitative as one between numbers and words, with quantitative researchers focusing on numbers and qualitative researchers on words. This distinction is not accurate, as many qualitative researchers use numbers in their analyses. Rather, the distinction should be made according to when the information is quantified. If we know which possible values occur in the data before the research starts, we conduct quantitative research. If we only know this after the data have been collected, we conduct qualitative research. Think of it in this way: if we ask survey questions and use a few closed questions such as “Is this product of good quality?” and the respondents can choose between “Completely disagree,” “Somewhat disagree,” “Neutral,” “Somewhat agree,” and “Completely agree,” we know that the data we will obtain from this will – at most – contain five different values. Because we know all possible values before- hand, the data is quantified beforehand. If, on the other hand, we ask some- one, “Is this product of good quality?,” he or she could give many different answers, such as “Yes,” “No,” “Perhaps,” “Last time yes, but lately...”. This means we have no idea what the possible answer values are. Therefore, this data is qualitative. We can, however, recode these qualitative data and assign values to each response. Thus, we quantify the data, allowing further statisti- cal analysis. Qualitative and quantitative research are equally important in the market research industry in terms of money spent on services.1 Practically, market research is often hard to categorize in qualitative or quantitative as it may include elements of both. Research that includes both elements is sometimes called hybrid or fused market research, or mixed methodology. this provides more detail and if we need these data at another level, we can aggregate the data. Aggregating data means that we sum up a variable at a lower level to create a variable at a higher level. For example, if we know how many cars all car dealers in a country sell, we can take the sum of all dealer sales, to create a variable measuring countrywide car sales. Aggregation is not possible if we have incomplete or missing data at lower levels. 1 See http://www.e-focusgroups.com/press/online_article.html [email protected] 32 3 Data 3.4 Dependence of Observations A key issue for any data is the degree to which observations are related. If we have exactly one observation from each individual, store, company, or country, we label the observations independent. That is, the observations are completely unrelated. If we have multiple observations of each individual, store, company, or country, we label them dependent. For example, we could ask respondents to rate a type of Cola, then show them an advertisement, and again ask them to rate the same type of Cola. Although the advertisement may influence the respondents, it is likely that the first response and second response will be related. That is, if the respondents first rated the Cola negatively, the chance is higher that they will continue to rate the Cola negative rather than positive after the advertisement. If the data are dependent, this often impacts what type of analysis we should use. For example, in Chap. 6 we discuss the difference between the independent samples t-test (for independent observations) and the paired samples t-test (for dependent observations). 3.5 Dependent and Independent Variables Dependent variables represent the outcome that market researchers study while independent variables are those used to explain the dependent variable(s). For example, if we use the amount of advertising to explain sales, then advertising is the independent variable and sales the dependent. This distinction is artificial, as all variables depend on other variables. For example, the amount of advertising depends on how important the product is for a company, the company’s strategy, and other factors. However, the distinction is frequently used in the application of statistical methods. While researching relationships among variables, we need to distinguish between dependent and independent variables beforehand, based on theory and practical considerations. 3.6 Measurement Scaling Not all data are equal! For example, we can calculate the average age of the respondents of Table 3.1 but it would not make much sense to calculate the average gender. Why is this? The values that we have assigned male (0) or female (1) respondents are arbitrary; we could just as well have given males the value of 1 and female the value of 0, or we could have used the values of 1 and 2. Therefore, choosing a different coding would result in different results. Measurement scaling refers to two things: the variables we use for measuring a certain construct (see discussion above) and the level at which a variable is measured which we discuss in this section. This can be highly confusing! [email protected] 38 3 Data 3.8 Population and Sampling A population is the group of units about which we want to make judgments. These units can be groups of individuals, customers, companies, products, or just about any subject in which you are interested. Populations can be defined very broadly, such as the people living in Canada, or very narrowly, such as the directors of large hospitals in Belgium. What defines a population depends on the research conducted and the goal of the research. Sampling is the process through which we select cases from a population. The most important aspect of sampling is that the sample selected is representative of the population. With representative we mean that the characteristics of the sample closely match those of the population. In Box 3.4, we discuss how to determine whether a sample is representative of the population. Box 3.4 Is my sample representative of the population? Market researchers consider it important that their sample is representative of the population. How can we see if this is so? – The best way to test whether the sample relates to the population is to use a dataset with information on the population. For example, the Amadeus and Orbis databases provide information at the population level. We can (statistically) compare the information from these databases to the sample selected. The Amadeus database is available at http://www.bvdinfo.com. – You can use (industry) experts to judge the quality of your sample. They may look at issues such as the type and proportion of organizations in your sample and population. – To check whether the responses of people included in your research do not differ significantly from non-respondents (which would lead to your sam- ple nor being representative), you can use the Armstrong and Overton procedure. This procedure calls for comparing the first 50% of respondents to the last 50% with regard to key demographic variables. The idea behind this procedure is that later respondents more closely match the characteristics of non-respondents. If these differences are not significant (e.g., through hypothesis tests, discussed in Chap. 6), we find some support that there is little, or no, response bias (see Armstrong and Overton 1977). This procedure is sometimes implemented by comparing the last wave of respondents in a survey design against earlier waves. There is some evidence this procedure is better than the original procedure of Armstrong and Overton (Lindner et al. 2001). – Using follow-up procedures, a small sample of randomly chosen non- respondents can be contacted again to ask for cooperation. This small sample can be compared against the responses that were obtained earlier to test for any differences. (continued) [email protected] 3.8 Population and Sampling 39 Box 3.4 (continued) http://www.bvdinfo.com When we develop a sampling strategy, we have three key choices: – Census, – Probability sampling, and – Non-probability sampling. If we get lucky and somehow manage to include every unit of the population in our study, we have conducted a census study (so, strictly speaking, this is not sampling). Census studies are rare because they are very costly and because missing just a small part of the population can have dramatic consequences. For example, if we were to conduct a census study among directors of banks in Luxemburg, we may miss out on a few because they were too busy to participate. If these busy directors happen to be those of the very largest companies, any information we collect would underestimate the effects of variables that are more important in large banks. Census studies work best if the population is small, well-defined, and accessible. Sometimes census studies are also conducted for specific reasons. For example, the US Census Bureau is required to hold a census of all persons resident in the US every 10 years. Check out the US Census Bureau’s YouTube channel using the mobile tag or URL in Box 3.5 to find out more about the US Census Bureau. [email protected] 40 3 Data Box 3.5 The US census http://www.youtube.com/uscensusbureau#p/ If we select part of the population, we can distinguish two types of approaches: probability sampling and non-probability sampling. Figure 3.2 provides an over- view of the different sampling procedures, which we will discuss in the following sections. Sampling procedures Probability sampling Non-probability sampling Simple random sampling Judgmental sampling Systematic sampling Snowball sampling Stratified sampling Quota sampling Other types of Cluster sampling convenience sampling Fig. 3.2 Sampling procedures 3.8.1 Probability Sampling Probability sampling approaches provide every individual in the population a chance (not equal to zero) of being included in the sample. This is often achieved by using an [email protected] 3.8 Population and Sampling 41 accurate sampling frame. A sampling frame is a list of individuals in the population. There are various sampling frames, such as Dun & Bradstreet’s Selectory database (includes executives and companies), the Mint databases (includes companies in North and South Americas, Italy, Korea, the Netherlands, and the UK), or telephone directories. These sampling frames rarely completely cover the population of interest and often include some outdated information, but due to their ease of use and availability they are frequently used. If the sampling frame and population are very similar, we have little sampling frame error, which is the degree to which sample frames represent the population. Starting from a good-quality sampling frame, we can use several methods to select units from the sampling frame. The easiest way is to use simple random sampling, which is achieved by randomly selecting the number of cases required. This can be achieved by using specialized software, or using Microsoft Excel or SPSS.3 Specifically, Microsoft Excel can create random numbers between 0 and 1 (using the RAND() function). Next you choose those individuals from the sampling frame where the random value falls in a certain range. The range depends on the percentage of respondents needed. For example, if you wish to approach 5% of the sampling frame, you could set the range from 0.00 to 0.05. Systematic sampling uses a different procedure. We first randomize the order of all observations, number them and, finally, select every nth observation. For exam- ple, if our sampling frame consists of 1,000 firms and we wish to select just 100 firms, we could select the 1st observation, the 11th, the 21st, etc. until we reach the end of the sampling frame and have our 100 observations. Stratified sampling and cluster sampling are more elaborate techniques of probability sampling, which require dividing the sampling frame into different groups. When we use stratified sampling, we divide the population into several different homogenous groups called strata. These strata are based on key sample characteristics, such as different departments in organizations or the area in which consumers live. Subsequently we draw a random number of observations from each strata. While stratified sampling is more complex and requires accurate knowledge of the sampling frame and population, it also helps to assure that the sampling frame’s characteristics are similar to those of the sample. Cluster sampling requires dividing the population into different heterogeneous groups with each group’s characteristics similar to those of the population. For example, we can divide the consumers of one particular country into different provinces, counties, or councils. Several of these groups can perhaps be created on the basis of key characteristics (e.g., income, political preference, household composition) that are very similar (representative) to those of the population. We can select one or more of these representative groups and use random sampling to select our observations from this group. This technique requires knowledge of the 3 By going to Data in the SPSS menu options, then Select Cases, followed by Random sample of cases, you can indicate what percentage or exact number of cases you want to be randomly selected from the sampling frame. The use of SPSS will be discussed in detail in Chap. 5 and beyond. [email protected] 42 3 Data sampling frame and population, but is convenient because gathering data from one group is cheaper and less time consuming. Generally, all probability sampling methods allow for drawing representative samples from the target population. However, simple random sampling and, in particular, stratified sampling are considered superior in terms of drawing representative samples. 3.8.2 Non-probability Sampling Non-probability sampling procedures do not give every individual in the population an equal chance of being included in the sample. This is a drawback, because the resulting sample is most certainly not representative of the population, which may bias results of subsequent analyses. Nevertheless, non-probability sampling procedures are frequently used as they are easily executed, and are typically less costly than probability sampling methods. Judgmental sampling is based on researchers taking an informed guess regarding which individuals should be included. For example, research companies often have panels of respondents who are continuously used in research. Asking these people to participate in a new study may provide useful information if we know, from experience, that the panel has little sampling frame error. Snowball sampling is predominantly used if access to individuals is difficult. People such as directors, doctors, or high-level managers often have little time and are, consequently, difficult to involve. If we can ask just a few of these people to provide names and details of others in a similar position, we can expand our sample quickly and access them. Similarly, if you post a link to an online questionnaire on your Facebook page (or send out a link via email) and ask your friends to share it with others, this is snowball sampling through referrals to people who would be difficult to access otherwise. In quota sampling, we select observations according to some fixed quota. That is, observations are selected into the sample on the basis of pre-specified characteristics so that the total sample has the same distribution of characteristics assumed to exist in the population being studied. In other words, the researcher aims to represent the major characteristics of the population by sampling a proportional amount of each (which makes the approach similar to stratified sampling). Let’s say, for example, that you want to obtain a quota sample of 100 people based on gender. First you would need to find out the proportion of the population that is men and the proportion that is women. If you found out the larger population is 40% women and 60% men, you would need a sample of 40 women and 60 men for a total of 100 respondents. You would start sampling and continue until you got those proportions and then you would stop. So, if you’ve already got 40 women for the sample, but not 60 men, you would continue to sample men and discard any female respondents that came along. What makes quota sampling a non-probability technique is that the selection of the observations does not occur randomly. That is, once the quota has been fulfilled for a certain characteristic (e.g., females), you do not allow any more observations with this specific characteristic in the sample. This systematic component of the [email protected] 3.9 Sample Sizes 43 sampling approach can introduce a sampling error. Nevertheless, quota sampling is very effective for little cost, making it the most prominent sampling procedure in practitioner market research. Finally, convenience sampling is a catch-all term for methods (including the three non-probability sampling techniques just described) in which the researcher makes a subjective judgment. For example, we can use mall intercepts to ask people in a shopping mall if they want to fill out a survey. The researcher’s control over who ends up in the sample is limited and influenced by situational factors. 3.9 Sample Sizes After determining the sampling procedure, we have to determine the sample size. Larger sample sizes increase the precision of the research, but are also much more expensive to collect. The gains in precision decrease as the sample size increases (in Box 6.3 we discuss the question whether a sample size can be too large in the context of significance testing). It may seem surprising that relatively small sample sizes are precise, but the strength of samples comes from accurately selecting samples, rather than through sample size. Furthermore, the required sample size has very little relation to the population size. That is, a sample of 100 employees from a company with 100,000 employees can be nearly as accurate as selecting 100 employees from a company with 1,000 employees. There are some problems in selecting sample sizes. The first is that market research companies often push their clients towards accepting large sample sizes. Since the fee for market research services is often directly dependent on the sample size, increasing the sample size increases the market research company’s profit. Second, if we want to compare different groups, we need to multiply the required sample by the number of groups included. That is, if 150 observations are sufficient to measure how much people spend on organic food, 2 times 150 observations are necessary to compare singles and couples’ expenditure on organic food. The figures mentioned above are net sample sizes; that is, these are the actual (usable) number of observations we should have. Owing to non-response (discussed in Chaps. 4 and 5), a multiple of the initial sample size is normally necessary to obtain the desired sample size. Before collecting data, we should have an idea of the percentage of respondents we are likely to reach (often fairly high), a percentage estimate of the respondents willing to help (often low), as well as a percentage estimate of the respondents likely to fill out the survey correctly (often high). For example, if we expect to reach 80% of the identifiable respondents, and if 25% are likely to help, and 75% of those who help are likely to fully fill out the questionnaire, only 15% (0.800.250.75) of identifiable respondents are in this case likely to provide a usable response. Thus, if we wish to obtain a net sample size of 100, we need to desired sample size send out ¼ 100=0:15 ¼ 667 surveys. In Chap. 4, we will likely usable responses discuss how we can increase response rates (the percentage of people willing to help). [email protected]