HIS Module - Week 7 PDF
Document Details
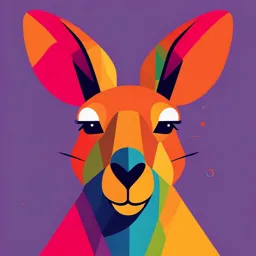
Uploaded by IssueFreePipeOrgan3951
St. Dominic College of Asia
Tags
Summary
This module covers data quality within the context of health information systems (HIS) for medical lab science. It outlines the importance of data quality in various applications and introduces tools for assessing and improving data quality, such as the Routine Data Quality Assessment (RDQA) and Lot Quality Assurance Sampling (LQAS).
Full Transcript
ONLINE LEARNING MODULE HEALTH INFORMATION SYSTEM FOR MED LAB SCIENCE WEEK 7 – HMIS Data Quality DATA QUALITY Over the years, data quality has become a major concern for large com...
ONLINE LEARNING MODULE HEALTH INFORMATION SYSTEM FOR MED LAB SCIENCE WEEK 7 – HMIS Data Quality DATA QUALITY Over the years, data quality has become a major concern for large companies especially in the areas of customer relationship management (CRM), data integration, and regulation requirements. Aside from the fact that poor data quality generates costs, it also affects customer satisfaction, company reputation, and even the strategic decisions of the management. Routine Data Quality Assessment (RDQA) The Routine Data Quality Assessment (RDQA) tool is a Data quality is the overall utility of a dataset(s) as a simplified version of the Data Quality Audit (DQA) tool function of its ability to be processed easily and analyzed which allows programs and projects to verify and assess for a database, data warehouse, or data analytics system. the quality of their reported data. It also aims to strengthen data management and reporting systems. The objectives of RDQA are as follows (RDQA User Manual, 2015): 1. Rapidly verify the quality of reported data for key Data quality signifies the data’s appropriateness to serve indicators at selected sites. its purpose in a given context. Having quality data means 2. Implement corrective measures with action plans for that the data is useful and consistent. Data cleansing can strengthening data management and reporting system be done to raise the quality of available data (Rouse, and improving data quality. 2005). 3. Monitor capacity improvements and performance of data management and reporting system to produce Lot Quality Assurance Sampling (LQAS) quality data. Lot Quality Assurance Sampling (LQAS) is a tool that The RDQA is a multipurpose tool that is most effective allows the use of small random samples to distinguish when routinely used. Following are the uses for the between different groups of data elements (or lots) with RDQA tool (RDQA User Manual, 2015): high and low data quality. For health managers and supervisors, using small samples makes the conduct of surveys more efficient. This tool has been widely applied in the health care industry for decades and has been primarily used for quality assurance of products. Data Quality Tools A data quality tool analyzes information and identifies incomplete or incorrect data. Data cleansing follows after the complete profiling of data concerns, which could range anywhere from removing abnormalities to merging repeated information. Gartner (2017) explains how these data quality tools are used to address problems in data quality : Development Implementation Plan An implementation plan is a project management tool that illustrates how a project is expected to progress at a high level. It helps ensure that a development team is working to deliver and complete tasks on time (Visual Paradigm, 2009). It is also important in ensuring the efficient flow of communication between those who are involved in the project so as to minimize issues that would delay delivery of the project. It validates the estimation and schedule of the project plan. As data quality continues to become increasingly all- encompassing, data integration tools are further An implementation plan is developed through the developed to include data quality management following key steps (Smartsheet, 2017): functionality. Define Goals/Objectives. Address the question, “What do you want to accomplish?” Application/Scope of Data Quality tools Schedule Milestones. Outline the deadline and The first generation of data quality tools was timelines in the implementation phase. characterized by dedicated data cleansing tools designed Allocate Resources. Determine whether you to address normalization and reduplication. However, in have sufficient resources, and decide how you the last 10 years, it was observed that there is a will procure those missing. generalization of Extract, Transform, Load (ETL) tools Designate Team Member Responsibilities. which allow the optimization of the alimentation Create a general team plan with overall roles process. Recently, these tools started to focus on Data that each team member will play. Quality Management (DQM), which generally integrates Define Metrics for Success. How will you profiling, parsing, standardization, cleansing, and determine if you have achieved your goal? matching processes (Goasdue, Nugier, Duquennoy, and Laboisse, 2007). Root Cause Analysis FMEA is used when there is a new product or process or when there are changes or updates in a product and A root cause analysis is a problem solving method that when there is a problem reported through customer identifies the root causes of problems or events instead feedback. of simply addressing the obvious symptoms. The aim is to improve the quality of products and services by using Pareto Analysis systematic ways to address problems in order to be effective (Bowen, 2011). Techniques in Root Cause Analysis Root cause analysis is among the core building blocks in the continuous improvement efforts of an organization in terms of its operation dynamics, especially in the way it handles information. However, root cause analysis alone will not produce any valuable results. The organization should seek to improve a at every level and in every department for this to work. The analysis will help develop protocols and strategies to address Figure 8.1 Pareto Chart showing causes of malnutrition underlying issues and reduce future errors. Bowen (2011) suggest that “to address the root cause of a Fault Tree Analysis problem, one must identify the problem and ask “why” five times, to determine the proper strategies to address its root cause.” Failure Mode and Effects Analysis (FMEA) The Failure mode and effects analysis (FMEA) aims to find various modes for failure within a system and addresses the following questions for execution: 1. What is the mode in which an observed failure occurs? 2. How many times does a cause of failure occur? 3. What actions are implemented to prevent this cause from occurring again? 4. Are the actions effective and efficient? Current Reality Tree (CRT) Kepner-Tregoe Technique Figure 8.2 Current Reality Chart on a bus that is overcrowded. Fishbone or Ishikawa or Cause-and-Effect Diagrams RPR Problem Diagnosis Another technique for root cause analysis is the Rapid Problem Resolution (RPR Problem diagnosis) which diagnoses the causes of recurrent problems by following the three phases below: Discover – data gathering and analysis of the findings Investigate – creation of a diagnostic plan and identification of the root cause through careful Figure 8.3 Ishikawa chart showing analysis on why analysis of the diagnostic data patients leave the clinic. Fix – fix the problem and monitor to confirm and validate that the correct root cause was identified. Sustaining culture of information use Choo, Bergeron, Detlor, and Heaton (2008) state that information culture affects outcomes of information use. The information culture is determined by the following variables: mission, history, leadership, employee traits, industry, and national culture. It can also be shaped by cognitive and epistemic expectations which are influenced by the way tasks are performed and decisions are made. KEY POINTS TO REMEMBER: Data quality is the overall utility of a dataset(s) as a function of its ability to be processed easily and analyzed for a database, data warehouse, or data analytics system. Lot Quality Assessment (LQAS) is a tool that allows the use of small random samples to distinguish between different groups of data elements (or lots) with high and low data quality. The Routine Data Quality Assessment Tool (RDQA) is a simplified version of the Data Quality Audit (DQA) which allows programs and projects to verify and assess the quality of their reported data. The development of an implementation plan is important in ensuring that the communication between those who are involved in the project will not encounter any issues and work will also be delivered on time. A root cause analysis is a problem solving method aimed at identifying the root causes of problems or events instead of simply addressing the obvious symptoms. Techniques in root cause analysis include Failure Mode and Effects Analysis (FEMA), Pareto Analysis, Fault Tree Analysis, Current Reality Tree (CRT), Fishbone or Ishikawa or Cause-and- Effect Diagrams, Kepner-Tregoe Technique, and RPR Problem Diagnosis.