Hastings aamp; Washington( 2010) The First of the Month Effect.pdf
Document Details
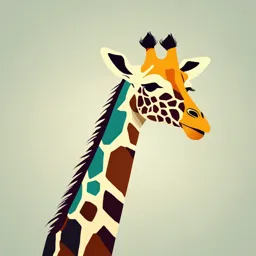
Uploaded by AppreciatedUranium
University of Bern
2010
Tags
Full Transcript
American Economic Journal: Economic Policy 2 (May 2010): 142–162 http://www.aeaweb.org/articles.php?doi=10.1257/pol.2.2.142 The First of the Month Effect: Consumer Behavior and Store Responses† By Justine Hastings an...
American Economic Journal: Economic Policy 2 (May 2010): 142–162 http://www.aeaweb.org/articles.php?doi=10.1257/pol.2.2.142 The First of the Month Effect: Consumer Behavior and Store Responses† By Justine Hastings and Ebonya Washington* Previous research has demonstrated that benefit recipients decrease expenditures on, and consumption of, food throughout the benefit month. Using detailed grocery store scanner data, we ask two ques- tions: whether cycling is due to a desire for variety that leads to within-month substitution across product quality, and whether cycling is driven by countercyclical retail pricing. We find that the decrease in food expenditures is largely driven by reductions in quantity, not quality, and that prices for foods purchased by benefit households vary pro-cyclically with demand, implying that house- holds could save money by delaying their food purchases until later in the month. (JEL D12, I38) T here is a growing line of empirical literature demonstrating the sensitivity of the timing of household purchases to the timing of the receipt of income. Melvin Stephens, Jr. (2003, 2006) finds evidence of a purchasing cycle in two distinct popu- lations: social security recipients in the United States, and paycheck recipients in the United Kingdom, both of whom receive checks monthly. In samples of both groups, he finds that spending on instantaneous consumption, which he defines as fresh food, food away from home, and entertainment, increases following income receipt. Stephens (2006) concludes that he finds evidence in violation of the per- manent income hypothesis. Other researchers, such as David Huffman and Matias Barenstein (2005), who examine the same United Kingdom data as Stephens (2006), push the interpretation further, concluding that the cycling patterns in purchases con- stitute real world evidence of short-run impatience or quasi-hyperbolic discounting.1 * Hastings: Yale University, Department of Economics, Box 208264, New Haven, CT 06520 and National Bureau of Economic Research (NBER) (e-mail: [email protected]); Washington: Yale University, Department of Economics, Box 208264, New Haven, CT 06520 and NBER (e-mail: ebonya.washington@yale. edu). We thank two anonymous referees for helpful comments. We also thank Joseph Altonji, Steven Berry, Keith Chen, Raj Chetty, Judy Chevalier, Nada Eissa, Tim Guinnane, Patrick Kline, Camille Landais, Jesse Shapiro, Sharon Oster, and seminar participants at Boston University, Brown University, Clemson University, Harvard University, Institute for International Economic Studies, the NBER TAPES Conference, Northwestern University, Notre Dame, Rutgers University, University of California at Berkeley, University of Chicago GSB, and the Yale Industrial Organization lunch for their valuable input. Lydia Ashton, Eric Chyn, Kimberly Tolland, and Alexander Torgovitsky provided excellent research assistance. We gratefully acknowledge funding from the Yale University Institution for Social and Policy Studies. All remaining errors are ours. † To comment on this article in the online discussion forum, or to view additional materials, visit the articles page at http://www.aeaweb.org/articles.php?doi=10.1257/pol.2.2.142. 1 David Laibson (1997) develops the notion of quasi-hyperbolic discounting in which individuals have distinct short- and long-run time preferences and evaluate trade offs with a present bias. The majority of empirically support for impatience has been found in laboratory settings (Shane Frederick, George Loewenstein, and Ted O’Donoghue 2002). 142 Vol. 2 No. 2 Hastings and washington: The first of the Month Effect 143 Researchers investigating the prevalence of short-run impatience have paid par- ticular attention to populations receiving government benefits, presumably because of the group’s policy relevance; utility losses suffered by recipients due to impatience could be inexpensively undone through public policy.2 Parke E. Wilde and Christine K. Ranney (2000) and Jesse M. Shapiro (2005) use survey data to document not only that food stamp recipients buy relatively more food at the beginning of their food stamp month, but, more importantly, that they consume relatively more calories as well. Shapiro (2005) estimates that calorie intake decreases by 0.45 percent per day within the food stamp month. He presents evidence against food spoilage, theft, and strategic considerations vis-à-vis other family members and community members, or naïveté driving the results. He interprets this as evidence of short-run impatience. His policy prescription is for localities to divide food stamp distribution into smaller, more frequent payments to each household. In this paper, we consider two alternative explanations for the benefit household food consumption cycle that could not be tested using survey data. First, recipients may have a preference for variation in the quantity and quality of food consumed, providing a rational explanation for expenditure and consumption patterns over the course of the month. Due to data limitations, Shapiro (2005) presents only indirect evidence against the desire for a few “feast” days. He points to survey data dem- onstrating cycling in food stamp recipients’ hypothetical willingness to accept a smaller immediate payment in place of a larger payment in the future. As the month goes on, recipients are more willing to accept the smaller payment. He interprets this finding as evidence that the marginal utility of income (to buy food) is not con- stant, but rather increases throughout the month, suggesting that benefit households do not desire this variance in food consumption. Households who show the greatest increases in marginal utility are the most likely to report food shortfalls at the end of the month. Second, cycling in purchasing behavior could also be consistent with rational behavior if, as in previous literature (e.g., Elizabeth J. Warner and Robert B. Barsky 1995; James M. MacDonald 2000; Judith A. Chevalier, Anil K. Kashyap, and Peter E. Rossi 2003), prices decline at times of peak demand, and therefore groceries are less expensive at the beginning of the month. Prior research relying on survey or diary data cannot address store pricing response to demand cycles, and lacks the detail to test for substitution across product quality within grocery category over the course of the month. Access to two years of item-level scanner data from three Nevada stores belong- ing to a national supermarket chain allows us to present direct evidence on the rel- evance of these alternative explanations. We first document, consistent with previous literature, that benefit recipients (defined as customers who ever purchase with Food Stamps or cash welfare assistance currency) do relatively more of their food shop- ping at the beginning of the month (defined as the date on which benefits are distrib- uted). We find a sharp decrease in benefit households’ food expenditures of nearly 20 percent from the first to the second week of the month. Expenditures continue to 2 For example, Carlos Dobkin and Steven L. Puller (2007) find that that SSI and DI recipients see an increase in drug related hospitalizations and mortality at the beginning of their payment month. C. Fritz Foley (forthcom- ing) shows that financially motivated crime is higher at the end of the Food Stamp/TANF payment month. 144 American Economic Journal: economic policy May 2010 decline less steeply through the remainder of the month. The decline in expenditures is robust across products from storable to perishable items. We see no such cycle in food expenditures amongst store patrons who do not receive benefits, whether low or high income. Second, we examine to what extent the decrease in food expenditures is driven by changes in quantities versus changes in the quality of food purchased. We find over- whelming evidence in favor of the former. In fact, point estimates suggest that quan- tity reductions can explain the full decrease in food expenditures. From week one to week four, benefit households reduce their quantities purchased by 32 percent. Quality reductions are much smaller. From week one to week four, benefit house- holds increase their relative (to nonbenefit households) propensity to buy generic by 1 percent and decrease their relative propensity to buy premium by 2 percent. Benefit households also exhibit a small increase (about 1 percent) in their relative propensity to purchase sale items. Thus, we find no evidence that the food spending cycle is driven by a desire for variation in food products consumed throughout the month. While some states stagger benefit delivery, in Nevada food stamps and cash benefits are distributed to all recipients on the same day. Thus, each month, gro- cery stores face a large and predictable increase in demand for goods most heavily purchased by benefit recipients. Finally, we explore the store’s pricing response to this demand shift. We do not find evidence of countercyclical pricing, but find that the increase in aggregate demand induced by benefit delivery results in food price increases. While the pricing response is small, prices fall 3 percent as quantities purchased fall 32 percent, the fact that prices move pro-cyclically with demand rules out the desire to purchase food when it is relatively cheaper as an explanation for the food purchasing cycle. The fact that prices rise, even slightly, with demand provides additional support for impatience as the best explanation of the purchasing behavior. Even if households have nonconvex preferences, they would do better to shift their expenditure peak to a later point in the month. These findings add further support to the view that food stamp recipients display impatience. They also suggest that the bulk of food stamp subsidies accrue to the intended recipients, particularly in markets and products where subsidy recipients represent a lower overall percentage of demand. The relatively small price effects stand in contrast to estimates of incidence in other subsidy programs, such as the Earned Income Tax Credit (EITC), where recipients make up a large fraction of the market and their behavior is likely to have a large impact on equilibrium market prices, (e.g., labor supply and wages in Jesse Rothstein (2008), and used car prices in William Adams, Liran Einav, and Jonathan Levin (2009)). One key limitation of our study is that we only have data for one grocery store per neighborhood. If low-income shoppers’ choice of food retailer is correlated with timing within the month, then our purchasing pattern results will be biased. However, our expenditure results are of a similar magnitude to Shapiro (2005) who relies on survey data which is not prone to such bias. The second concern stemming from our use of grocery data concerns the generalizability of our pricing results. We know that grocery chains are far more likely to locate in low-poverty neighbor- hoods (Chanjin Chung and Samuel L. Myers, Jr. 1999). However, across the nation, 83 percent of food stamps are cashed in supermarkets, and 50 percent of food stamp Vol. 2 No. 2 Hastings and washington: The first of the Month Effect 145 recipients spend their benefits exclusively in supermarkets (Nancy Cole and Ellie Lee 2005). Thus, our pricing results are relevant for the majority of food stamp recipients. I. Data Previous investigations of the monthly food expenditure/consumption cycle have relied on household survey data. Such data have been extremely valuable in allowing researchers to carefully document cycling amongst benefit-receiving populations. However, because household survey data do not provide the full menu of products from which households are able to choose, nor the monthly variation in price of products chosen or unchosen, survey data do not allow for the investigation of two possible causes of such cycling. These two possible causes are: a desire to consume a variety of food products across the month, and a response to variation in food prices across the month. We are fortunate to have access to grocery store scanner data that will allow us to investigate both of these possibilities. Our scanner data cover transactions at three focal stores in a national grocery chain over the 26-month period from January 2006 to February 2008. The data include an observation for each item purchased, detailing the quantity, price, and any discounts applied. While our supermarket chain carries food and nonfood items, such as those found in a drug store, in keeping with previous literature, we analyze only food pur- chases. Our dataset includes an observation for each food item scanned at the register during our 26-month period. Each item is uniquely identified by its Universal Product Code (UPC), an identifier specific to the product and size of container where appli- cable (e.g., extra large red delicious apples, or General Mills Cheerios Cereal 18 oz. box). There are thousands of UPCs for food items. For each scanned item, the data also record the form of payment, which can include cash, credit, debit and, most importantly for our purposes, currency from the social programs, food stamps, cash welfare, and The Special Supplemental Nutrition Program for Women, Infants, and Children (WIC). Finally, each record includes a household loyalty card identifier. We use the loyalty card number and payment infor- mation to identify benefit recipients. In particular, we categorize a loyalty card num- ber as belonging to a welfare recipient if that loyalty card was associated with any purchases settled with currency from social programs over the 26-month period. We will refer to such loyalty card holders as “benefit households” from here forward.3 We analyze purchases at three stores located in the state of Nevada. We focus on Nevada because food stamp and cash assistance benefits are delivered to all recipi- ents on the first day of the month in that state.4 This concentrated benefit delivery schedule is important for our ability to identify the start of the benefit recipient income month and to be able to chart the pattern of expenditures throughout that month. 3 Because people cycle on and off of government assistance programs, we have also run specifications in which we defined benefit recipients as loyalty card holders who had paid with cash welfare or food stamps within the past year. The results are robust to the change in definition. 4 WIC benefits are distributed upon request. Our results are robust to excluding WIC recipients from the benefit household category. 146 American Economic Journal: economic policy May 2010 Table 1—Summary Statistics Benefit households Non-benefit households (N = 29,367) (N = 229,643) First Second First Second All weeks two weeks two weeks All weeks two weeks two weeks Percent store purchases 0.257 0.286 0.226 0.743 0.714 0.774 Average weekly expenditure 34.98 38.30 31.29 28.08 27.96 28.21 Purchased product quality Percent generic 0.120 0.118 0.123 0.090 0.090 0.091 Percent mainstream 0.546 0.541 0.553 0.570 0.568 0.571 Percent premium 0.335 0.342 0.325 0.340 0.342 0.338 Percent purchases on sale 0.594 0.589 0.601 0.575 0.571 0.579 Notes: A household is defined by an anonymous loyalty card identification number. Transactions from benefit households are defined as any transaction made with a loyalty card number that was used with food stamp pay- ment method at any time during our sample period. Product quality categories were constructed from product classifications and product descriptions provided with the scanner data. On sale items are items that registered a discount off the list price in the transaction record. In Nevada, both food stamps and cash assistance are distributed electronically to a benefits card that works like a debit card. Benefits do not expire, instead, they may be carried over from month to month. Nevada’s cash assistance program is less gen- erous than the national average. In 2002, the maximum benefit for a family of three with no income was $348 compared to a national mean of $413 and median of $390 (Gretchen Rowe and Victoria Russell 2004). Even including food stamp benefits, which are determined according to a single national formula that takes cash benefits into account, Nevada residents’ maximum benefit level is below the national median (U.S. House of Representatives Committee on Ways and Means 2004). Within Nevada, we select our three focal stores based on the demographic char- acteristics of their patrons. In particular, we select stores in which a relatively large share of food purchases (14 to 45 percent) were made by benefit recipient house- holds.5 The stores are all located in zip codes in which Latinos and families in pov- erty are overrepresented (by two to three times) and educational attainment levels are lower than both state and national averages. In all neighborhoods, our focal store faces competition from small ethnic grocers as well as larger chain retailers.6 Table 1 presents summary statistics of household expenditures for benefit and nonbenefit households over the 26-month period. The first three columns show expenditure patterns pooled across all three stores over the course of the month for benefit households, while the last three columns show expenditure patterns for nonbenefit households. Twenty-six percent of all purchases are made by benefit- receiving households. Looking at percent of purchases by time of the month, we see that the share of store purchases for benefit-households falls by 6 percentage points from the first to the second half of the month. Benefit households spend an average of $35 dollars per week on food items at our supermarket, while nonbenefit 5 Food prices are set weekly and can vary by store. 6 Zip code demographic data are drawn from the 2000 census using the American Fact finder Web site. Competition data from www.switchboard.com. Vol. 2 No. 2 Hastings and washington: The first of the Month Effect 147 households spend less at $28.7 Previewing our results, we see that average weekly expenditures fall between the first and second half of the month for benefit house- holds, but remain constant for nonbenefit households. The remaining rows of the table examine purchasing patterns by purchase type. We divide all products into three quality categories: generic, mainstream, and pre- mium. Our categorization is based on product descriptions provided to us by the grocery chain. We code as premium any product that is so categorized in the store’s description. We code as generic nonpremium items that are marketed under the store’s private label.8 Any premium private-label products are classified as premium. Mainstream is the residual category capturing all items that are neither generic nor premium. Table 1 indicates that the share of purchases devoted to each product cat- egory is quite similar for benefit and nonbenefit households. About 55 percent of pur- chases for both groups are of mainstream products, about 10 percent are generic, and the remainder are premium. Dividing products simply according to whether they are on promotion (on sale) or not, we see that 59 percent of products purchased by benefit households are on sale, compared with 58 percent of purchases by nonbenefit households.9 Benefit households are only slightly more likely than other households to purchase generics or sale items. Further, that the shares of purchases that are generic, mainstream, premium, and sale items are consistent across the month pre- views our results that benefit households do not substitute across product types over the course of the month.10 II. Regression Analysis of Expenditures Table 2 documents monthly food expenditure patterns for benefit recipient and nonrecipient households. The table cells report coefficients from regressions of the following form: (1) Yits = beni × weekt β′ + weekt δ′ + hi λ′ + ss η′ + εits, where Yits is the log of total expenditures for household i in week t at store s; beni is an indicator for whether household i is classified as a benefit household; weekt is a row vector of dummies for each seven day period beginning at the first of each month (week of the pay month dummies, where we exclude from the sample the days past the twenty-eighth day of the month); hi is a row vector of household fixed-effects; 7 This difference in expenditures is generated somewhat by a difference in family size for benefit and non- benefit households. The demographic data include the number of children and number of adults per household. When we normalize expenditures by number of household members for the limited sample for which we have demographic data, the gap in expenditures between benefit and nonbenefit households shrinks further. Benefit households may still spend more at the grocery store for two reasons. First, nonbenefit households may shop over more stores because they have better access to transportation. Second, benefit households consume a much larger portion of their food as food at home versus food away from home (Dora Gicheva, Justine Hastings, and Sofia Villas-Boas Forthcoming). 8 We also included items categorized as “bulk” or “economy” in the detailed product description. 9 The scanner data include information on markdowns and discounts for each item. If an item is purchased and a discount is posted at the register, we consider that item to be “on sale.” 10 An earlier version of this paper (Justine Hastings and Ebonya Washington 2008) shows how expenditure patterns differ between benefit and nonbenefit households across detailed product categories. 148 American Economic Journal: economic policy May 2010 Table 2—Change in Expenditures across Stores Alcohol and All Storable Perishable Splurge tobacco Benefit household × week 2 −0.189** −0.201** −0.190** −0.112** −0.029* (0.007) (0.009) (0.008) (0.008) (0.015) Benefit household × week 3 −0.264** −0.261** −0.244** −0.180** −0.037* (0.008) (0.009) (0.009) (0.008) (0.014) Benefit household × week 4 −0.299** −0.285** −0.272** −0.206** −0.029* (0.008) (0.010) (0.009) (0.009) (0.015) Week 2 −0.017** −0.010** −0.019** −0.002 −0.016* (0.003) (0.004) (0.003) (0.004) (0.006) Week 3 −0.006* −0.005 −0.011** 0.009* 0.003 (0.003) (0.004) (0.003) (0.004) (0.006) Week 4 −0.005 −0.023** −0.016** 0.011** 0.002 (0.003) (0.004) (0.003) (0.004) (0.006) Mean expenditures by Benefit households 34.51 14.19 19.19 9.11 13.99 Non-Benefit households 27.95 11.67 16.29 8.00 16.57 Observations 1,395,925 876,610 1,097,039 915,550 242,187 Notes: Robust standard errors in parentheses. Columns present results from an OLS regression of log weekly expenditures per household and store on an indicator if the household is a benefit household, the week number in the month, and their interactions. All regressions include household fixed effects with standard errors clustered at the household level. A household is defined by an anonymous loyalty card identification number. Transactions from benefit households are defined as any transaction made with a loyalty card number that was used with food stamp payment method at any time during our sample period. Regressions pooling data from all three stores also include store-level fixed effects. ** Significant at the 1 percent level. * Significant at the 5 percent level. and ss is a row vector of store fixed-effects. The first week of the pay month and its interaction with the benefit household indicator are omitted so that each coefficient (multiplied by 100) is the percentage decline in total expenditures relative to the first week. Each column of Table 2 presents the coefficients for β and δ. Standard errors, clustered at the household level, are reported in parentheses. The first column pres- ents results for all products pooled together, while the subsequent columns present results by broad product classifications: storable goods, perishable goods, “splurge” goods, and alcohol and tobacco.11 Turning to the first column, we see that benefit households decrease their grocery purchases throughout the month. Relative to nonbenefit receiving households, the total purchases of benefit households (first row) decline by 18.9 percent from week one to week two, and continue to decline, though not as steeply, as we move to week three and week four, when the reduction is 29.9 percent relative to week one. In contrast, nonbenefit households exhibit almost no decline in expenditures over the For results by detailed product type and by store, see Hastings and Washington (2008). Storable goods 11 include canned fish and meat; canned fruits; canned vegetables; cereal and breakfast; cookies and crackers; flour, sugar, and cornmeal; frozen breakfast items; frozen fruits; frozen juices; frozen vegetables; Hispanic products; jams, jellies, and spreads; pasta and pasta sauce; prepared frozen foods; ready-to-eat foods; rice and beans; salty snacks; shelf juices and drinks; and soups. Perishable items include cheese, fresh bread, fresh produce, in-store fresh bread, meat, refrigerated dairy, refrigerated foods, refrigerated juice and drinks, and seafood. Splurge items include candy, gum and mints, sweet baked goods, dessert and baking mixes, desserts, frozen desserts, ice cream, in-store sweet baked goods, and soft beverages. Vol. 2 No. 2 Hastings and washington: The first of the Month Effect 149 course of the month.12 The coefficients on the week dummies are generally an order of magnitude smaller than the coefficients on the interactions indicating a much smaller decrease (off a smaller mean) in purchases across the month for nonbenefit recipient purchasers. Our results are consistent with Wilde and Ranney (2000) and Shapiro (2005) who document food spending decreases across the month for low-income house- holds. The magnitude of the decline we demonstrate is similar to Shapiro (2005) who focuses exclusively on food stamp recipients and finds a decrease in the market value of purchased food of 20 percent from the first to the twenty-eighth day of the month. Shapiro’s market value of food is not measured directly through prices, however. The Nationwide Food Consumption Survey, whose data he uses, assigns this value based on the food the household reports that it consumes. That our results are slightly greater in magnitude is not surprising given that we focus on a popula- tion that receives both their food stamp and their cash welfare benefits on the exact same day of the month. The similarity of our results to those of Shapiro (2005) lends credence to our claim that despite our focus on only one retail outlet per community, we are capturing representative grocery purchases of benefit households.13 We place foods into four groups (storable, perishable, splurge, and alcohol and tobacco) to examine whether results vary by food type. Results are displayed in the remaining columns of Table 2. Expenditures on both storable and perishable items exhibit steep declines from week one to week four, which suggests a violation of the permanent income hypothesis. The decline for splurge items is slightly less steep, while alcohol and tobacco (items not covered by food stamps) experience the small- est decline in expenditures over the course of the month. Table 2 documents the cycle in expenditure patterns on the intensive margin. In Table 3, we consider how variation in the extensive margin purchasing decision impacts our findings. We combine linear probability models of the purchase/no pur- chase decision with OLS models of expenditures on the intensive margin to address selection into the purchase decision in two steps, following John F. McDonald and Robert A. Moffitt (1980). First, we estimate a linear probability model of the prob- ability of purchasing in a given week as a function of the week of the month, week of the month interacted with an indicator if the household is a benefit household, and household fixed-effects.14 We then estimate the expected expenditure level con- ditional on positive expenditures as a function of these same variables. We then 12 We rely on nonbenefit households as a control because this population’s income receipt is not concentrated on the first of the month. Paydays may be every Friday, every other Friday, the first of the month, and the fifteenth of the month. We do not have this information. Thus, the small magnitude of the weekly main effects does not indicate that the grocery purchases of nonbenefit households are not sensitive to the timing of receipt of income. However, because the average standard deviation of weekly grocery expenditures within a household/month is smaller for nonbenefit households as compared to benefit households, we do know that non-benefit households exhibit smoother purchasing patterns than benefit households. 13 These results are also robust to several specification checks. In particular, we limited our sample to frequent shoppers (those who shopped at least once in the first half and once in the second half of the month) and found the same decline in expenditures. In addition, to address the concern that results may be driven by events concurrent to the first, we found that the regression results were robust to the inclusion of month effects, time trends, holiday dummies, and gasoline prices due to the fact that the monthly cycle has such a sharp and particular pattern. 14 For tractability, and because of the possibility of household relocation, we eliminate household-weeks that occur in months in which the household made no purchases in a focal store. 150 American Economic Journal: economic policy May 2010 Table 3—Decomposition of Total Expenditure Changes: Weeks 1 to 4, Benefit Households Change in Change in Change in Change in unconditional expenditures on expenditures on intensive/change Type of goods mean expenditure extensive margin intensive margin in total All goods −9.603 −3.005 −5.794 0.603 (0.685) (0.501) (0.236) Storable −3.495 −1.265 −1.861 0.532 (0.268) (0.192) (0.099) Perishable −4.712 −1.586 −2.675 0.568 (0.357) (0.256) (0.125) Splurge −1.501 −0.754 −0.642 0.428 (0.134) (0.096) (0.062) Alcohol and tobacco −0.197 −0.229 0.034 −0.172 (0.107) (0.081) (0.060) Notes: Let E(y) be the expected value of expenditures, E(y*) be the expected value of expenditures conditional on y being greater than zero and F(z) be the probability that y is greater than zero. Column 1 gives dEy/dx calculated as F(z)dEy*/dx + Ey*(dF(z)/dx. Column 2 and 3 decompose this total derivative into changes on the extensive (Ey*(dF(z)/dx) and intensive (as F(z)dEy*/dx) margins respectively. Columns 2 and 3 may not sum to 1 because of rounding. The standard deviation of 100 estimates from 10 percent random samples is provided for each sta- tistic in parentheses. recover the total (not conditional on expenditures greater than zero) derivative of expenditures with respect to benefit weeks by following the procedure laid out by McDonald and Moffitt (1980).15 We follow this two-step approach for two reasons. First, maximum likelihood estimation of nonlinear panel data models, such as Tobit analysis, has been shown to be biased and inconsistent, particularly when T is small (James J. Heckman and Thomas E. MaCurdy 1980). Second, the two step approach allows us to express the unconditional expected change in expenditures as a function of changes in the extensive and intensive margins, allowing for further economic interpretation of the empirical results. Table 3 presents results from the McDonald-Moffitt decomposi- tion. The first row shows the decomposition for all products, while the next four rows show the decomposition for the four product subcategories of interest. The first column of results gives the total change in unconditional expected expenditures from week one to week four. The second and third columns of results decompose this unconditional change into changes along the extensive and intensive margins: the change in the expected probability of purchase from week one to week four, and the expected change in expenditures, given expenditures are greater than zero from week one to week four, respectively. The first row of Table 3 shows the results for all products. We find that uncondi- tional mean expenditures fall by $9.60 from week one to week four for benefit house- holds. This fall represents a 27.4 percent decline by week four off a base of $34.98. 15 Specifically, let E (y) be the expected value of y; E (y*) be the expected value of y, conditional on y being greater than zero; and F (z) be the probability that y is greater than zero. Then E (y) = F (z)E (y*) and dEy/dx = F (z)dEy*/dx + Ey*(dF (z)/dx). Note that because weeks are discrete instead of continuous, we calculate the change as the difference in E (y) between week one and week four. McDonald and Moffitt (1980) demonstrate this decomposition using examples of Tobit estimates that could yield more economic insights by separately examin- ing changes on the extensive and intensive margins. Joseph G. Altonji and Ernesto Villanueva (2007) provide an additional empirical example using this approach. Vol. 2 No. 2 Hastings and washington: The first of the Month Effect 151 This is very similar in magnitude to the point estimate of 29.9 percent presented in Table 2. Of this $9.60 decline, approximately two-thirds is generated by a decrease in expected expenditures conditional on nonzero expenditures. We find very similar percentage declines for all three food product categories across Tables 2 and 3. The unconditional percentage decline in expenditures for alcohol and tobacco is smaller than what we find in Table 2, suggesting that purchasers decrease the probability of purchasing toward the end of the month rather than purchasing less given positive expenditures.16 Table 4 employs information on household income to investigate whether the identified decline in expenditures generalizes to low-income households who may be budget constrained, but do not depend on the monthly benefits cycle for income. We use estimated household income data that the supermarket chain collects for a sample of loyalty card holders, and add interactions between weekly dummies and income quartiles to equation (1). The first specification in Table 4 (columns 1 and 2) repeats the base specification in Table 2, column 1 for the subsample of households with demographic data, and confirms that the same expenditure decline is present in this subsample. For the second specification (columns 3 and 4), we add interactions between income quartiles and weekly effects among nonbenefit households. Coefficients on these interactions, even for the least well off quartile (1), are an order of magnitude smaller than our focal benefit households × week coefficients. Our results indicate that the purchasing pattern is particular to benefit households. This may be because low-income wage earners receive wages weekly or bi-weekly. It also may be because different wage earners receive monthly com- pensation on different days of the month. Stephens (2006) finds cycling on food, both for consumption at home and away, amongst monthly paycheck recipients in the United Kingdom. However, in contrast to our findings for benefit households, he finds that food-for-home purchases only decline in the fourth week of the month and not between the first and second weeks, suggesting smoother purchasing patterns for nonaid recipients. Our results thus far provide a robust replication of findings in previous literature; benefit-receiving households’ grocery expenditures fall across the month. Because we have replicated this finding with scanner data as opposed to survey data, we can now explore patterns in household expenditures over the monthly cycle that would not be possible to investigate with data on expenditures from the Consumer Expenditure Survey, for example. We can examine if benefit households display further nonstandard income effects. For instance, do they feel relatively wealthy at the beginning of the month, substituting toward premium and brand-name products and away from generics (Greg M. Allenby and Rossi 1991)? Given that they do not smooth consumption, do they shift toward inferior goods or become more sen- sitive to promotional items as the month proceeds and their relative income level 16 To construct the unconditional mean, we had to assume that all products should be purchased weekly, which may not be true for many products such as canned vegetables or alcohol and tobacco. For products purchased less frequently than every week, the unconditional mean may overstate changes in the extensive margin. In fact, if households adjust purchases on extensive versus intensive margins differently, we would expect that the ratio of intensive to extensive margins for total expenditures would be larger than for any one category alone. For analysis by product category, please see Hastings and Washington (2008). 152 American Economic Journal: economic policy May 2010 Table 4—Expenditures Patterns, All Stores, with Income Interactions Base model for Interactions with subsample with income income quartiles Coefficient SE Coefficient SE Benefit households Week 2 − 0.173** (0.011) − 0.194** (0.010) Week 3 − 0.234** (0.012) − 0.244** (0.011) Week 4 − 0.259** (0.013) − 0.265** (0.012) Non-benefit households Week 2 − 0.021** (0.005) — — Week 3 − 0.010* (0.004) — — Week 4 − 0.006 (0.005) — — Week 2 × income quartile 1 — — − 0.027** (0.010) Week 3 × income quartile 1 — — − 0.011 (0.009) Week 4 × income quartile 1 — — − 0.015 (0.010) Week 2 × income quartile 2 — — − 0.016 (0.009) Week 3 × income quartile 2 — — − 0.020* (0.009) Week 4 × income quartile 2 — — − 0.015 (0.009) Week 2 × income quartile 3 — — − 0.028** (0.009) Week 3 × income quartile 3 — — − 0.005 (0.009) Week 4 × income quartile 3 — — 0.002 (0.009) Week 2 × income quartile 4 — — − 0.015 (0.009) Week 3 × income quartile 4 — — − 0.003 (0.009) Week 4 × income quartile 4 — — 0.002 (0.009) Observations 571,057 R2 0.45 Mean expenditures 30.29 Mean expenditures benefit HH 34.46 Mean expenditures non-benefit 29.11 Notes: Robust standard errors in parentheses. Columns present coefficients and standard errors from OLS regres- sions of household-level log weekly expenditures on the independent variables listed in each row. All regressions include household fixed effects with standard errors clustered at the household level. Household income is defined based on the retailer’s demographic data for loyalty card holders. Approximately 40 percent of the observations in our scanner data had income information present in the demographic data. Quartiles of the income distribution are defined using the retailer demographic data for club card holders purchasing from a representative subsam- ple of the retailer’s stores. The cut-points for the first 3 quartiles are $18,000, $37,000, and $72,000. The income information is top-coded at $500,000. The categories are not additive, implying that the coefficients in column 3 give the effect of each successive week on the expenditures for people in each of 5 categories: benefit households, non-benefit households with income in quartile 1, non-benefit households with income in quartile 2, etc. ** Significant at the 1 percent level. * Significant at the 5 percent level. decreases, in an attempt to stretch their marginal dollars further (Gicheva, Hastings, and Villas-Boas Forthcoming)? Our scanner data allow us to move beyond what previous literature has been able to do with diary data. We are able to decompose this change in expenditures to examine to what extent cycling is driven by changes in quantity versus quality of food items purchased. This distinction is important. One unexamined explanation for the monthly expenditure cycle is that households have a desire for a variety of products throughout the month. If the decrease in expenditures is driven by quality adjustments—households moving from the purchase of high to low quality products throughout the month—that would lend support to this theory. On the other hand, if the decrease in expenditures is driven primarily by quantity adjustments, then that would be further evidence of impatience on the part of benefit recipients. In the first column of Table 5, we examine how quantity purchased changes throughout the month. Because different items have different sizes, we report all Vol. 2 No. 2 Hastings and washington: The first of the Month Effect 153 Table 5—Change in Quantity and Quality Purchased and Change in Percent Purchases across Stores Quantity Quality measures Income index Generic Premium On Sale (1) (2) (3) (4) (5) Benefit household × week 2 − 0.232** 0.005** − 0.011** 0.000 − 0.0080** (0.011) (0.001) (0.002) (0.002) (0.0008) Benefit household × week 3 − 0.304** 0.009** − 0.016** 0.003 − 0.0129** (0.011) (0.001) (0.002) (0.002) (0.0008) Benefit household × week 4 − 0.322** 0.011** − 0.018** 0.007** − 0.0141** (0.012) (0.001) (0.002) (0.002) (0.0009) Week 2 − 0.017** 0.001 0.001 0.002* − 0.0008* (0.005) (0.001) (0.001) (0.001) (0.0004) Week 3 0.019** − 0.002** 0.006** 0.006** − 0.0008* (0.005) (0.001) (0.001) (0.001) (0.0004) Week 4 0.007 − 0.001* 0.007** 0.002** − 0.0002 (0.005) (0.001) (0.001) (0.001) (0.0004) Mean Average Average Average Average quantity percent percent percent index quality Benefit households 199.55 0.15 0.24 0.62 34,403.45 Non-benefit households 144.62 0.12 0.25 0.60 37,145.94 Observations 1,378,237 1,395,925 1,395,925 1,395,925 1,395,879 Notes: Robust standard errors in parentheses. Column 1 presents OLS results from a regression of household- level log weekly quantity purchased in ounces on an indicator if the household is a benefit household, the week number in the month, and their interactions. Columns 2, 3, and 4 present results from an OLS regression of frac- tion of weekly purchases (standardized weight) from Generic, Premium, or On Sale items on weekly dummies and their interactions with an indicator if the household is a benefit household. Column (5) presents OLS results from a regression of the log of the quantity-weighted income index by UPC product on an indicator if the house- hold is a benefit household, the week number in the month and their interactions. All regressions include store and household fixed effects with standard errors clustered at the household level. A household is defined by an anon- ymous loyalty card identification number. Transactions from benefit households are defined as any transaction made with a loyalty card number that was used with food stamp payment method at any time during our sample period. Product categories for Generic, Premium and On Sale were created based on the categorization made by the retailer and the product names. ** Significant at the 1 percent level. * Significant at the 5 percent level. quantities in ounces. We have sizes for approximately 90 percent of the items pur- chased so the sample size decreases slightly. The specification remains of the form (1), but the dependent variable is now log weekly quantity purchased (measured in total ounces). The coefficients show the decline in total number of ounces purchased by household i in week t at store s as we move from the first to the last week of the month. The results of the first column demonstrate that benefit recipients exhibit a large relative decline in total quantity purchased across the month. The results are of a similar magnitude to the results for log expenditures in Table 2, column 1. Across all food types, benefit households reduce their relative quantity purchased by 23 percent from week one to week two and by 32 percent from week one to week four. Recall that these same households reduce total expenditures by 30 percent across the four weeks. This suggests that the decline in expenditures is coming solely from a decline in quantity purchased, rather than in price savings from substitution toward less expensive products. In the remaining columns of Table 5, we examine four measures of the quality margin. We examine how families substitute over the course of the month among mainstream, generic, and premium products; between sale and nonsale items; and 154 American Economic Journal: economic policy May 2010 across items typically purchased by high- versus low-income patrons, using regres- sions of the form (1) in which the dependent variable is either the quantity-weighted fraction of purchases for household i in week t from store s that are classified as “generic,” “premium,” or “on sale” (columns 2–4), and the quantity-weighted income level of people who typically purchase that product (column 5).17 Classic income effects may lead households to substitute away from normal and luxury goods toward inferior goods as income drops, and this has been proposed as a reason for disparate cross-price elasticities between private label and brand name products (Allenby and Rossi 1991). While benefits households are not experiencing a change in permanent income over the course of the month, it is interesting to compare their substitution patterns across products to those identified elsewhere in response to changes in permanent income. Columns 2–4 of Table 5 indicate that across the month, benefit receiving house- holds do very little substitution toward generic items, away from premium products, or from nonsale to sale items. This inert purchasing pattern is not driven by the fact that benefit households have a substantial base fraction of products purchased from generic and sale items already. In fact, the mean fraction of purchase coming from generic and sale items is very similar across benefit and nonbenefit households.18 The last column of Table 5 presents results for one final measure of product quality. We generate an expenditure-weighted average income for each UPC in our data over the entire sample period. This statistic measures the expected income of a person who purchases this particular product. We may think that our categorizations of pre- mium or generic do not sufficiently capture quality. If higher income shoppers sub- stitute up toward higher quality goods, this index will capture quality by exploiting the revealed product choice of patrons with high-versus low-income. When we use the log of this index as the dependent variable, we find statistically significant, but economically small, declines in the quality of item purchased for benefit households over the course of the month. The results of this section indicate that benefit recipients show a steep drop in food expenditures over the course of the month. Grocery expenditures in week four are 30 percent smaller than those in week one. The results are true for both storable and per- ishable items, and thus constitute a violation of the permanent income hypothesis. Our results are consistent with those found in prior literature using survey and diary data on household food expenditures. Authors of earlier work have theorized that the purchase cycling is due to irrationality or impatience on the part of low-income households. Two alternative explanations for these purchasing patterns could not be explored with survey data. The first is that benefit households enjoy a “feast day” at the start of the month, purchasing premium products at the beginning of the month, and substituting 17 Because the extent to which generic (premium/sale) products are available differs by product and over time, we control for share of products available to consumer i at store s in week t in category k that are generic (premium/sale). 18 This makes an interesting contrast to income effects among nonbenefit households identified in Gicheva, Hastings, and Villas-Boas (2007) in response to sharp changes in gasoline prices. They use scanner data to examine how grocery expenditures shift in response to changes in permanent income generated by gasoline price spikes, and find that households respond by substituting toward promotional items, increasing the fraction of items purchased on sale by 10 to 50 percent when gasoline prices double, saving approximately 5 to 10 percent on grocery expenditures. Vol. 2 No. 2 Hastings and washington: The first of the Month Effect 155 toward inferior products toward the month’s end. However, using detailed scanner data, we have found no evidence to support this view. We find that the vast majority of the expenditure declines result from declines in quantities purchased, rather than substitution to lower quality or even sale items. The second untested explanation is that the purchasing cycle can be rationalized by changes in stores’ prices. We explore this possibility in the next section. III. Regression Analysis of Retail Response to Cyclical Demand In this section, we examine the firms’ response to benefit households’ predict- able demand cycles. There are three possible responses. First, retailers may price countercyclically, decreasing prices during periods of peak demand. This strat- egy has been documented in several settings, including retail grocery, where both MacDonald (2000) and Chevalier, Kashyap, and Rossi (2003) find large decreases in the price of food items during their high demand season. MacDonald (2000) shows that across 48 products with seasonal fluctuation in demand, prices fall an average of 8 percent as demand rises by 199 percent. Chevalier, Kashyap, and Rossi (2003) demonstrate that the price of tuna drops 13 percent during lent and that the prices of snack crackers and cheese fall by more than 10 percent during the Christmas season. The authors argue that their findings are consistent with a loss leader model of pricing in which stores compete for customers through advertised prices on items of high relative demand. However, Aviv Nevo and Konstantinos Hatzitaskos (2005) decompose the seasonal price paid reductions into substitution (to less expensive brands) and price reduction effects. They find that the substitution effects are the larger of the two, which is less consistent with the loss leader model. While the loss leader model makes no predictions for pricing during increases in aggregate demand for all products, which we document occur monthly amongst benefit households, Warner and Barsky (1995) show that countercyclical pricing may be optimal if con- sumer demand elasticity increases in periods of peak demand due to economies of scale in search. Consumers who make one large shopping trip at the start of the month, search more for the lowest price retailer for their large purchases at the start of the month than they do for their small purchases at the end of the month. At the start of the month consumers have more expenditures and items over which to reap the gains of finding the lowest price store. This makes demand more elastic during peak-demand, generating countercyclical retailer pricing. Countercyclical pricing on the part of our grocery retailers would provide a rational explanation for the food purchase cycle—benefit-receiving shoppers purchase relatively more food at the beginning of the month because this is when prices are lowest. Such a finding would provide evidence against irrationality or impatience on the part of low-income households as an explanation for cycling behavior. The second possibility is that stores do not respond to this early month increase in aggregate demand. Shapiro (2005) makes this assumption. Empirical evidence of constant within month food prices would offer no evidence against his conclusion that monthly food consumption cycling is due to short-run impatience. Finally, grocery retailers may pursue the traditional response to an increase in demand or decrease in demand elasticity. They may raise prices cyclically as 156 American Economic Journal: economic policy May 2010 demand rises. Cyclical pricing would mean that benefit households could increase welfare by delaying their purchases until later in the month when prices fall. For example, Adams, Einav, and Levin (2009) show that demand for sub-prime used auto loans increases by 30 percent at the time of EITC receipt and required cash down-payments increase in response by 20 percent, implying that liquidity-con- strained purchasers could save by slightly delaying their car purchase after receiving their EITC check. Hence, cyclical demand accompanied by cyclical pricing would provide additional support for short-run impatience on the part of welfare recipient households as an explanation for their food purchase behavior.19 To examine store pricing behavior, and whether benefit recipients are responding to prices rationally or whether they could save money from altering purchase tim- ing, we create a price index that measures the change in the within-month variation in the cost of benefit households’ typical food basket. The price index is constructed as follows: (2) ln pricets = ∑ ωk ln ( ptsk), k where ωk is the total share, across the three stores, that benefit-receiving households spend on product k (identified by UPC) over the 26-month period; ptsk is the price for product k at store s on day t. Hence, this price index gives the share-weighted price on each day in each store for the typical basket of groceries for a benefit household. Table 6 presents results from regressions of the form (3) ln pricets = β1 + β 2 week2 + β 3 week3 + β 4 week4 + εts, ts ts ts where weeki is an indicator for the week of the month that each store-day observa- tion falls into. We exclude data from days after the twenty-eighth day of the month, so the first week becomes the omitted category. The top panel of the table uses the basket based on purchases of benefit-receiving households regardless of timing. The bottom panel uses the basket based solely on purchases made during the first week of each month. The coefficients in the first column of the top panel indicate that the basket of goods purchased by benefit recipient households is 1.8 percent less expensive in week two compared to week one. The basket’s price falls to 2.5 percent less expen- sive in week four, compared to week one.20 This finding suggests the opportunity for welfare gains on the part of benefit households by substituting intertemporally and/ or across products in their food purchase behavior. 19 Cyclical demand, or over-responsiveness of consumptions to predictable changes in income is difficult to generate in the absence of impatience since credit constraints themselves arise from insufficient precautionary savings. For example, in Adams, Einav, and Levin (2009), households who could save their EITC check at some point in time could save considerable money by changing the timing of their car purchase. 20 In the bottom panel of the table, we also calculate the weights in the price index, ω k , as the share that benefit households spend on product k during the first week of the month for any month in our 26-month sample. This second weighting allows us to hone in on how prices vary for those goods that benefit households purchase dur- ing their most active shopping period. This weighting allows us to answer the question of whether households could save money by altering the timing of that most active shopping period. We find stronger effects with point estimates of − 0.031, − 0.019, − 0.034, and − 0.040 as we move from pooled results to results for Stores 1–3, respectively. Vol. 2 No. 2 Hastings and washington: The first of the Month Effect 157 Table 6—Change in Prices Across the Month All Store 1 Store 2 Store 3 Log (price index) Week 2 − 0.018** − 0.016** − 0.020** − 0.018** (0.002) (0.004) (0.003) (0.004) Week 3 − 0.023** − 0.013** − 0.025** − 0.031** (0.002) (0.004) (0.003) (0.004) Week 4 − 0.025** − 0.016** − 0.028** − 0.034** (0.002) (0.004) (0.003) (0.004) Log (first week price index) Week 2 − 0.021** − 0.018** − 0.024** − 0.022** (0.002) (0.005) (0.003) (0.004) Week 3 − 0.027** − 0.015** − 0.031** − 0.037** (0.002) (0.005) (0.003) (0.004) Week 4 − 0.031** − 0.019** − 0.034** − 0.040** (0.002) (0.005) (0.003) (0.004) Observation 2,086 723 723 640 Percent store benefit purchases 0.257 0.141 0.259 0.454 Notes: Standard errors in parentheses. Each column presents results from OLS regressions of log price index, calculated according to equation (2) in the text, on weekly dummies. Specifications that pool stores also include store-level fixed effects. ** Significant at the 1 percent level. * Significant at the 5 percent level. Turning to the store-by-store results, we see that the magnitude of the price decline increases with the percentage of store’s merchandise purchased by benefit recipients.21 Store 1, at which benefit recipients account for 14 percent of purchases, reduces the prices of the food basket 1.6 percent in week four compared to week one. At Store 2, with 26 percent of purchases made by benefit recipients, the figure is 2.8 percent. At Store 3, with 45 percent of purchases made by benefit households, the number rises to 3.4 percent. The difference between the four week price change at Store 1 and Store 3 is statistically significant. Stores with a higher fraction of purchases by benefit households face larger fluctuations in aggregate demand. These results imply that the variation in the price of the benefit households’ food bundle is driven by fluctuations in aggregate demand.22 Figure 1 provides an additional piece of evidence in support of the view that fluc- tuations in demand drive our pricing results. Here, we explore how the high poverty stores’ pricing varies across product categories. To do so, we construct price indexes based solely on the products in a particular category at our core set of Nevada high- poverty stores. Note that within each broad category, such as “Fresh Produce,” there are many individual products. The figure shows a scatter plot of the change in quan- tity purchased by benefit households from week one to week four against the change in product pricing across the three stores over the same time period. The figure dis- 21 Note that we also ran specifications in Table 2 by store and found very similar overall declines in expendi- tures for benefit households in all three stores. 22 For a robustness check, we selected three low-poverty stores in Nevada (3–6 percent of purchases coming from benefit households) and found no price pattern in those stores over the course of the month. 158 American Economic Journal: economic policy May 2010 –0.09 –0.08 –0.07 –0.06 –0.05 –0.04 –0.03 –0.02 –0.01 0 0.01 0.02 0.1 0.05 0 Quantity –0.05 –0.1 –0.15 –0.2 Price index –0.25 Figure 1. Scatterplot of Change in Quantities Purchased and Change in Price by Product Category Notes: 1. Candy, Gum and Mints; 2. Cookies/Crackers and Misc Snacks; 3. Jams, Jellies and Spreads; 4. Soft Beverages; 5. Salty Snacks; 6. Cereal and Breakfast; 7. Desserts; 8. Desserts and Baking Mixes; 9. Flour/Sugar/ Corn Meal; 10. Shelf Stable Juices and Drinks; 11. Canned Vegetables; 12. Canned Fruits; 13. Canned Fish and Meat; 14. Ready-to-Eat Prepared Foods; 15. Soups; 16; Rice & Beans; 17. Pasta and Pasta Sauce; 18. Hispanic; 19. Tobacco and Smoking Needs; 20. Refrigerated Dairy; 21. Cheese; 22. Refrigerated Foods; 23. Refrigerated Juice and Drinks; 24. Ice Cream; 25. Frozen Dessert; 26. Frozen Fruits; 27. Frozen Breakfast Items; 28. Frozen Juices; 29. Frozen Vegetables; 30. Prepared Frozen Foods; 31. Fresh Produce; 32. Seafood; 33. Meat; 34. Alcoholic Beverages; 35. In Store Fresh Bread; 36. In Store Bakery Fresh Snacks; 37. Commercial Fresh Bread; 38. Snacks. plays results using the weights generated by benefit household purchases during any first week of any month throughout our 26-month time period. The figure is notable for the positive slope of the points. As quantity purchased increases, so does price. Across categories, the correlation between within-month quantity changes and change in price is 0.33. For instance, products such as meat, and rice and beans, for which quantities purchased fall by more than 15 percent across the month, also see some of the greatest (in magnitude) price changes across the same time period. Products for which there is less variation in benefit house- holds’ demand such as canned fruits, frozen juices, in-store fresh bread, and alcohol and tobacco, see less price change. The figure provides further evidence that the cyclical price changes are driven by cyclical increases in product demand. Thus, our pricing results reveal that the prices of food items purchased by benefit households fall by about 3 percent from the first to the fourth week of the month. Relative to the more than 30 percent decrease in quantities purchased over this period, the price fluctuations are small. Our average, benefit household spends approximately $140 per month at our grocery store. A savings of 3 percent is only $3.50. Nonetheless, our findings are notable for two reasons. First, our pricing results indicate that benefit households could stretch their food dollar a little further by shifting food purchases to later in the month. In the previous section, we find that benefit households could lower their food spending by shifting purchases across products (from premium to mainstream, mainstream to generic, or from nonsale to sale items). Taken together, our findings suggest that benefit households are fairly inert in their substitution patterns. These households could smooth consumption and minimize the costs of their food basket through intertemporal and/or cross-product substitution. Vol. 2 No. 2 Hastings and washington: The first of the Month Effect 159 Second, our pricing results speak to the question of whether the observed food expenditure cycle among low-income households should be interpreted as short-term impatience on the part of these households. While countercyclical pricing would have provided an alternative explanation for the purchasing patterns, our results of slightly pro-cyclical food pricing provide additional support for impatience as an explanation. Furthermore, while one alternative explanation for monthly expendi- ture patterns is nonconvex preferences, adding in evidence on prices shows that even if benefit households prefer lumpy expenditures, which vary in quantity or quality of food consumed across the month given their budget constraint, they would be significantly better off to shift their consumption peak slightly to take advantage of lower prices at periods of lower aggregated demand. These results add to the growing literature in Behavioral Industrial Organization, showing that profit maximizing actions of firms that are aware of behavioral biases among their customers typically lower the welfare of the biased agents, but also induce a potential cross-subsidization from biased consumers to nonbiased consumers who are aware of the actions of biased customers and the firm’s response (see Stefano DellaVigna and Ulrike Malmendier 2004, Glenn Ellison 2006, Xavier Gabaix and Laibson 2006, and DellaVigna 2009, among others). To the extent that public policy design induces behavioral response among biased agents, these results add to the grow- ing literature on subsidy or tax incidence and optimal design of cash-transfer programs. Programs that have large and predictable impacts on retail demand, such as tax credits (e.g., EITC) or food stamps, and target recipients who may have overly responsive consumption to changes in income (due to impatience or naïve hyperbolic discounting, for example), may generate optimal responses from retail firms that decrease the frac- tion of the subsidy reaching its intended recipients (Wojciech Kopczuk and Cristian Pop-Eleches 2007; Rothstein 2008; Adams, Einav, and Levin 2009). Small changes in policy design, such as staggering benefits receipt, that mitigate biased responses, could provide relatively low-cost, but first-order, impacts on welfare. IV. Conclusion Using detailed scanner data from a national grocery retailer, we document a food expenditure cycle among benefit recipients in the state of Nevada. Households that receive food stamps and/or cash welfare increase their food expenditures in the first week following benefit distribution. The decline in food expenditures from week one to week two is 19 percent among these households, with smaller additional declines across the remainder of the month. By week four, food expenditures, relative to non- benefit recipients, have fallen 30 percent from expenditures in week one. We identify no such expenditure cycle for nonbenefit recipients. Our findings for benefit house- holds are robust across stores at which benefit recipients purchase a varying share of the food items. Results are also robust across food categories, from perishable to storable, and thus constitute a violation of the permanent income hypothesis. Our results are consistent with those of Wilde and Ranney (2000) and Shapiro (2005), who use expenditure survey data to document a food spending cycle among food stamp recipients. The latter author concludes that this pattern is evidence of hyper- bolic discounting or short-run impatience on the part of benefit recipients. 160 American Economic Journal: economic policy May 2010 Our scanner data allow us to explore two alternative explanations that could not be tested using survey data. First, we consider the possibility that benefit recipi- ents desire variety in their food consumption and thus purchase splurge items at the beginning of the month and shift to lower cost items at month’s end. Second, we investigate the store’s pricing response to understand whether the expenditure cycle is a rational response to decreased food prices early in the month. We find support for neither of these possibilities. Benefit households do little cross-product substitution as the month progresses. The decreased expenditure at month’s end can be entirely accounted for by a change in quantities purchased. Point estimates indicate that the relative ounces of food product purchased by benefit households fall 32 percent from week one to week four, while benefit households are only 1 percent more likely to purchase generic items and about 2 percent less likely to purchase premium items over the same time period. The relative tendency for benefit households to purchase sale items also increases only slightly (about 1 percent) throughout the month. Thus, there is no evidence that benefit households food expenditure cycle is driven by a desire for higher quality feast days upon check receipt. We also find no evidence that the purchasing cycle is spawned by lower prices during the high demand early month period. We compute a price index for the basket of food goods consumed by benefit-recipient households. The price of this basket falls about 3 percent from week one to week four. While this price decrease is small relative to the change in quantities purchased, it provides strong evidence against the expenditure cycle being driven by a desire to purchase food items at lower prices. In fact, even had we found evidence that benefit households substitute across quality within the month, or even if benefit households define splurge days as increases in only quantity, the fact that the greater expenditures occur during the time of highest prices would mean that the substitution patterns could not be interpreted as rational behavior. Thus, both our food quality/quantity results and our pricing results lend support to the notion that food expenditure cycling is due to short-run impatience on the part of low-income households. Evidence of short-run impatience has also been found in other populations in labor market behavior (DellaVigna and M. Daniele Paserman 2005), health club plan choice (DellaVigna and Malmendier 2006), and credit card usage (Laibson, Andrea Repetto, and Jeremy Tobacman 2003). Shapiro (2005) makes a policy prescription based on benefit recipients’ impa- tience. He proposes that food stamp benefits, which are currently distributed to each household monthly in all states, be distributed more frequently, in smaller amounts. Our store pricing results suggest a second policy innovation should be considered if the goal is to improve the welfare of benefit recipients. While in Nevada, and eight other states, food stamp benefits are distributed to all recipients on a single day, in other states benefit delivery is staggered, generally across a week or two.23 Staggering the delivery of food stamp and cash benefits across the full month would eliminate the large swings in aggregate demand among benefit recipients, and would likely curtail the variation in the price of their food basket. Staggering is low 23 Email from the Department of Agriculture dated February 26, 2007. Vol. 2 No. 2 Hastings and washington: The first of the Month Effect 161 cost given that benefits are now delivered electronically. However, what cannot be inferred based on this investigation is at what price level the food basket would settle under staggering. Would the equilibrium food prices that result be closer to the lower cost end of the month price or the slightly higher cost beginning of the month price? Calculating the equilibrium prices over the course of the month and the welfare implications for staggering benefits would require estimating the parameters of indi- vidual demand for grocery products (i.e., does food stamp receipt increase demand, make it less elastic, or both), as well as the pricing decision of a multiproduct firm that prices both national and private label products. Not much is known about how firms learn about the biases of their consumers and how they develop profit max- imizing strategies in response (DellaVigna and Malmendier 2004; Ellison 2006; Gabaix and Laibson 2006; Steven D. Levitt 2006). Future research exploring how firm policies change when there are sharp changes in benefits receipt among clients may provide insights into firm learning and policy design in the presence of biased consumers. REFERENCES Adams, William, Liran Einav, and Jonathan Levin. 2009. “Liquidity Constraints and Imperfect Information in Subprime Lending.” American Economic Review, 99(1): 49–84. Allenby, Greg M., and Peter E. Rossi. 1991. “Quality Perceptions and Asymmetric Switching between Brands.” Marketing Science, 10(3): 185–204. Altonji, Joseph G., and Ernesto Villanueva. 2007. “The Marginal Propensity to Spend on Adult Chil- dren.” B.E. Journal of Economic Analysis & Policy, 7(1): 1–50. Chevalier, Judith A., Anil K. Kashyap, and Peter E. Rossi. 2003. “Why Don’t Prices Rise During Peri- ods of Peak Demand? Evidence from Scanner Data.” American Economic Review, 93(1): 15–37. Chung, Chanjin, and Samuel L. Myers, Jr. 1999. “Do the Poor Pay More for Food? An Analysis of Gro- cery Store Availability and Food Price Disparities.” Journal of Consumer Affairs, 33(2): 276–96. Cole, Nancy, and Ellie Lee. 2005. “Analysis of EBT Redemption Patterns: Methods and Detailed Tables.” Prepared for the U.S. Department of Agriculture, Food and Nutrition Service, Office of Analysis, Nutrition and Evaluation. Cambridge, MA: Abt Associates. http://www.fns.usda.gov/ ora/menu/Published/snap/FILES/ProgramOperations/EBTRedemptionTables.pdf. DellaVigna, Stefano. 2009. “Psychology and Economics: Evidence from the Field.” Journal of Eco- nomic Literature, 47(2): 315–72. DellaVigna, Stefano, and Ulrike Malmendier. 2004. “Contract Design and Self-Control: Theory and Evidence.” Quarterly Journal of Economics, 119(2): 353–402. DellaVigna, Stefano, and Ulrike Malmendier. 2006. “Paying Not to Go to the Gym.” American Eco- nomic Review, 96(3): 694–719. DellaVigna, Stefano, and M. Daniele Paserman. 2005. “Job Search and Impatience.” Journal of Labor Economics, 23(3): 527–88. Dobkin, Carlos, and Steven L. Puller. 2007. “The Effects of Government Transfers on Monthly Cycles in Drug Abuse, Hospitalization and Mortality.” Journal of Public Economics, 91(11–12): 2137–57. Ellison, Glenn. 2006. “Bounded Rationality in Industrial Organization.” In Advances in Econom- ics and Econometrics, Theory and Applications, Ninth World Congress, Volume II, ed. Rich- ard Blundell, Whitney K. Newey, and Torsten Persson, 142–74. New York: Cambridge University Press. Foley, C. Fritz. Forthcoming. “Welfare Payments and Crime.” Review of Economics and Statistics. Frederick, Shane, George Loewenstein, and Ted O’Donoghue. 2002. “Time Discounting and Time Preference: A Critical Review.” Journal of Economic Literature, 40(2): 351–401. Gabaix, Xavier, and David Laibson. 2006. “Shrouded Attributes, Consumer Myopia, and Information Suppression in Competitive Markets.” Quarterly Journal of Economics, 121(2): 505–40. Gicheva, Dora, Justine Hastings, and Sofia Villas-Boas. Forthcoming. “Revisiting the Income Effect: Gasoline Prices and Grocery Purchases.” American Economic Review. Hastings, Justine S., and Ebonya L. Washington. 2008. “The First of the Month Effect: Consumer Behavior and Store Responses.” National Bureau of Economic Research Working Paper 14578. 162 American Economic Journal: economic policy May 2010 Heckman, James J., and Thomas E. MaCurdy. 1980. “A Life Cycle Model of Female Labour Supply.” Review of Economic Studies, 47(1): 47–74. Huffman, David, and Matias Barenstein. 2005. “A Monthly Struggle for Self-Control? Hyperbolic Discounting, Mental Accounting, and the Fall in Consumption between Paydays.” Institute for the Study of Labor (IZA) Discussion Paper 1430. ftp://ftp.iza.org/dps/dp1430_rev.pdf. Kopczuk, Wojciech, and Cristian Pop-Eleches. 2007. “Electronic Filing, Tax Preparers and Participa- tion in the Earned Income Tax Credit.” Journal of Public Economics, 91(7–8): 1351–67. Laibson, David. 1997. “Golden Eggs and Hyperbolic Discounting.” Quarterly Journal of Econom- ics, 112(2): 443–77. Laibson, David, Andrea Repetto, and Jeremy Tobacman. 2003. “A Debt Puzzle.” In Knowledge, Information, and Expectations in Modern Macroeconomics: In Honor of Edmund S. Phelps, ed. Philippe Aghion, Roman Frydman, Joseph Stiglitz, and Michael Woodford, 228–66. Princeton, NJ: Princeton University Press. Levitt, Steven D. 2006. “White-Collar Crime Writ Small: A Case Study of Bagels, Donuts, and the Honor System.” American Economic Review, 96(2): 290–94. MacDonald, James M. 2000. “Demand, Information, and Competition: Why Do Food Prices Fall at Seasonal Demand Peaks?” Journal of Industrial Economics, 48(1): 27–45. McDonald, John F., and Robert A. Moffitt. 1980. “The Uses of Tobit Analysis.” Review of Econom- ics and Statistics, 62(2): 318–21. Nevo, Aviv, and Konstantinos Hatzitaskos. 2005. “Why Does the Average Price of Tuna Fall During Lent?” National Bureau of Economic Research Working Paper 11572. Rothstein, Jesse. 2008. “The Unintended Consequences of Encouraging Work: Tax Incidence and the EITC.” Princeton University Center for Economic Policy Studies Working Paper 165. Rowe, Gretchen, and Victoria Russell. 2004. “The Welfare Rules Databook: State Policies as of July 2002.” The Urban Institute Discussion Paper 04-06. Shapiro, Jesse M. 2005. “Is There a Daily Discount Rate? Evidence from the Food Stamp Nutrition Cycle.” Journal of Public Economics, 89(2–3): 303–25. Stephens, Melvin, Jr. 2003. “‘3rd of Tha Month’: Do Social Security Recipients Smooth Consumption between Checks?” American Economic Review, 93(1): 406–22. Stephens, Melvin, Jr. 2006. “Paycheque Receipt and the Timing of Consumption.” Economic Jour- nal, 116(513): 680–701. U.S. House of Representatives Committee on Ways and Means. 2004. 2004 Green Book: Background Material and Data on the Programs within the Jurisdiction of the Committee on Ways and Means. Prepared for the use of the Members of the Committee on Ways and Means. 108th Congress, 2nd Session. Ways and Means Committee Prints (WMCP) 108-6. Washington, DC: U.S. Government Printing Office. http://www.gpoaccess.gov/wmprints/green/2004.html. Warner, Elizabeth J., and Robert B. Barsky. 1995. “The Timing and Magnitude of Retail Store Mark- downs: Evidence from Weekends and Holidays.” Quarterly Journal of Economics, 110(2): 321–52. Wilde, Parke E., and Christine K. Ranney. 2000. “The Monthly Food Stamp Cycle: Shopping Fre- quency and Food Intake Decisions in an Endogenous Switching Regression Framework.” Ameri- can Journal of Agricultural Economics, 82(1): 200–213.