Exploring GPT-4 for Pokémon Name Transcreation PDF
Document Details
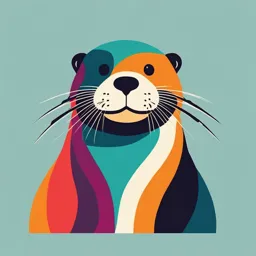
Uploaded by InventiveDrums
Luis Damián Moreno García & Carme Mangiron
Tags
Summary
This article explores the potential of GPT-4 as a tool for transcreation in game localization, using Pokémon names as a case study. The authors examine the use of prompt engineering and iterative development to assist translators in adapting fictional elements for different target cultures. The research also discusses limitations and ethical considerations related to using GPT models in such an application.
Full Transcript
Perspectives Studies in Translation Theory and Practice ISSN: (Print) (Online) Journal homepage: www.tandfonline.com/journals/rmps20 Exploring the potential of GPT-4 as an interactive transcreation assistant in game localisation: A case study on the translation of Pokémon names Luis D...
Perspectives Studies in Translation Theory and Practice ISSN: (Print) (Online) Journal homepage: www.tandfonline.com/journals/rmps20 Exploring the potential of GPT-4 as an interactive transcreation assistant in game localisation: A case study on the translation of Pokémon names Luis Damián Moreno García & Carme Mangiron To cite this article: Luis Damián Moreno García & Carme Mangiron (02 Sep 2024): Exploring the potential of GPT-4 as an interactive transcreation assistant in game localisation: A case study on the translation of Pokémon names, Perspectives, DOI: 10.1080/0907676X.2024.2378346 To link to this article: https://doi.org/10.1080/0907676X.2024.2378346 © 2024 The Author(s). Published by Informa UK Limited, trading as Taylor & Francis Group Published online: 02 Sep 2024. Submit your article to this journal Article views: 1217 View related articles View Crossmark data Full Terms & Conditions of access and use can be found at https://www.tandfonline.com/action/journalInformation?journalCode=rmps20 PERSPECTIVES https://doi.org/10.1080/0907676X.2024.2378346 Exploring the potential of GPT-4 as an interactive transcreation assistant in game localisation: A case study on the translation of Pokémon names a b Luis Damián Moreno García and Carme Mangiron a Department of Translation, Interpreting and Intercultural Studies, Hong Kong Baptist University, Kowloon, Hong Kong; bDepartment of Translation, Interpreting and East Asian Studies, Universitat Autònoma de Barcelona, Barcelona, Spain ABSTRACT ARTICLE HISTORY Generative Pre-trained Transformer (GPT) models are beginning to Received 14 March 2024 assist humans in complex creative endeavours. Transcreation is one Accepted 3 July 2024 of such creative processes, which in the context of translation KEYWORDS consists of linguistically and culturally adapting a text or a part of it, GPT-4; Video game including visuals, with the goal of maximising positive reception localisation; irrealia from a specific audience. Game localisation constantly engages in transcreation; prompt-based the transcreation of both real references (realia) and fictional conversational machine elements (irrealia). Irrealia, albeit imaginary, are essential for translation; prompt gamers’ successful immersion and, thus, need to be properly engineering; Pokémon video adapted. This paper examines the potential of GPT models as games assisting tools during game localisation processes. This exploratory study exemplifies how GPT-4 may be able to generate new language-specific irrealia via prompting to adapt fictional neologisms, focusing on pokémonikers, portmanteaus from the Pokémon game saga. It also describes how transcreation-related outputs can be used by translators as a pool of localisation options, for brainstorming, practice, and other purposes. The paper finds that the implementation of GPT chatbot-human interactions shows growing potential to support translators while adapting fictional elements, but also emphasises the current limitations of using GPT models, including accuracy, context-awareness, cultural sensitivity, and appropriateness, as well as potential ethical concerns. 1. Introduction Video games are a unique type of software defined by its special use of multimodality and interactivity. They typically incorporate a combination of visual, auditory, and textual elements, with which the player iteratively engages. Video game experiences are inextricably linked to world-building and storytelling and the creation of fictional elements is part and parcel of such world building. These virtual worlds CONTACT Luis Damián Moreno García [email protected] Department of Translation, Interpreting and Intercultural Studies, Hong Kong Baptist University, Kowloon, Hong Kong © 2024 The Author(s). Published by Informa UK Limited, trading as Taylor & Francis Group This is an Open Access article distributed under the terms of the Creative Commons Attribution-NonCommercial-NoDerivatives License (http://creativecommons.org/licenses/by-nc-nd/4.0/), which permits non-commercial re-use, distribution, and reproduction in any medium, provided the original work is properly cited, and is not altered, transformed, or built upon in any way. The terms on which this article has been published allow the posting of the Accepted Manuscript in a repository by the author(s) or with their consent. 2 L. D. MORENO GARCÍA AND C. MANGIRON may contain characters, objects and spaces that exist in the real world – realia – and others that are fictitious – irrealia. Realia often represent unique concepts, customs, or items that are deeply rooted in the source culture of game creators. Irrealia are ‘refer ences to fictional entities related to the fictional cultures of the game world’ (Pettini, 2021, p. 90). These tend to be represented via a variety of neologisms (Méndez Gon zález, 2019). This paper will focus on the translation of irrealia, and more precisely, portmanteaus, that is, novel words that combine the sounds and meanings of two existing words, from the Pokémon video game franchise. This topic was chosen to perform an experimental exploration due to the creativity required to adapt irrealia, and to preliminary findings pointing to the potential of GPT models to generate crea tive output (Guzik et al., 2023). Game localisation is a ‘cross-lingual and cross-cultural operation’ (O’Hagan, 2015, p. 747) that involves multiple layers and sequential steps, wherein the audiovisual and textual components of a game are modified to suit the preferences and cultural norms of a particular target market. Game localisers constantly engage in the adaptation of video games as entertainment experiences and therefore are often required to provide creative solutions in order to ensure that the players of the localised version will have a similar gameplay experience as those of the original one. For this reason, the term transcreation is often used to highlight the creative agency and the freedom granted to game localisers (Mangiron & O’Hagan, 2006). In the case of video games, transcreation may involve not only the adaptation of textual elements, but also the creative re-interpretation of certain visual components included as part of the game or as paratext to the game (cover, back, etc.). The complex world of a video game can be made of real or fictional elements, also known as realia and irrealia (Pettini, 2021, p. 90). Both are crucial for facilitating a player’s immersive experience and therefore require appropriate adaptation. One of the major challenges in game localisation is the translation of such fictional elements. Translators need to maintain the intent, style, tone, functionality or connotations of irrealia to keep a consistent and enjoyable gameplay experience (Pettini, 2021). More over, the names of fictional characters, objects, creatures and locations, tend to require more creativity than other in-game content (i.e., system messages, help messages, and tutorials), as well as a thorough understanding of both the source and target cultures from the part of translators (Pettini, 2021). The evolution of the game localisation market has witnessed significant advancements over time, culminating in the present era, where translation technology and automatic translation are assuming an increasingly pivotal role. Until recently, creative tasks were a realm reserved for the human translator. However, in recent years, advancements in artificial intelligence (AI) and natural language processing (NLP) have led to the devel opment of powerful language models like Generative Pre-trained Transformers (GPT). These models have lately shown potential for creative tasks, including translation and cultural adaptation (Cao et al., 2024). A novel method of translation, to which we refer in this paper as prompt-based conver sational machine translation (PCMT), involves prompting chatbots in ways that they output translations or interlingual adaptations of a certain textual input. Chatbots are aimed at interacting with users in a conversational manner, and are powered by fine- tuned Large Language Models (LLMs). Translator-users can combine certain tokens (the PERSPECTIVES 3 individual units of text of varying length that the model processes) to provide a translation- related prompt, that the chatbot processes to then provide a translation output. This paper aims to contribute to computer-aided translation research by exploring how GPT chatbots could assist during game localisation processes, focusing on the tasks related to irrealia recreation and adaptation. After defining the concepts of tran screation, realia and irrealia in the context of game localisation, it focuses on GPT tech nology and introduces several tools for the study and exploration of GPT translational output. The methodology is of an exploratory and qualitative nature due to the topic at hand. Next, the paper builds upon previous research, specifically in relation to the implementation of GPT systems for translation purposes, in order to explore several transcreative tactics and to propose use cases based on the translation of pokemónikers, that is, the names of Pokémon creatures. Finally, the potential advantages of GPT implementation in game localisation, are discussed, as well as a number of limitations and caveats, and future research avenues. 2. Transcreation in video game localisation research In this section, the concepts of game localisation and transcreation are examined. Game localisation can be defined as ‘a set of procedures involved in adjusting games technically, linguistically and culturally to a given market so as to distribute them in territories other than their countries of origin’ (O’Hagan, 2015, p. 747). It is a specialised form of software localisation that possesses distinctive characteristics, notably an exceptionally high degree of linguistic and cultural adaptation arising from the intricate stories and numer ous cultural references, puns and play-on-words included in video games, which are commonly interactive and procedural by nature. These elements require the creativity of translators for them to be properly adapted to meet the target players’ expectations. Due to the highly creative nature of game localisation, the term transcreation is often used when referring to this type of translation. Transcreation has been a buzzword in the language industry for the last twenty years, but academics have been using it for decades, mainly in the field of literary translation and, more recently, in the translation of advertising (Benetello, 2017, p. 237). However, its scope and meaning are not unanimous (Benetello, 2017; p. 237). Mangiron and O’Hagan (2006) were the first authors to use this term in the context of game localisation, to celebrate its creative nature and highlight the freedom granted to translators in this field: translators are often given carte blanche to modify, adapt, and remove any cultural. references, puns, as well as jokes that would not work in the target language. Localisers are given the liberty of including new cultural references, jokes, or any other element they deem necessary to preserve the game experience and to produce a fresh and engaging translation (p. 15). Transcreation plays a central role in game localisation, as the user-oriented and input- driven nature of the video game medium requires a more creative approach to trans lation, including the adaptation of the game’s content, context, and culture to provide a coherent and smooth experience for players in the target locale (Mangiron, 2007). For Fernández Costales (2012), transcreation is a crosswise translation strategy, particu larly relevant to video games with a strong narrative component, which can be observed in the translation of dialogues, subtitles and proper nouns. 4 L. D. MORENO GARCÍA AND C. MANGIRON There are authors, such as Bernal-Merino (2006, 2007, 2008), who believe that the concept of transcreation is not necessary, as it clashes with the concept of translation itself. According to this author, ‘all translations are transcreations since they require a certain degree of crea tivity on the translators’ part, although they are not creating anything from scratch’ (Bernal- Merino, 2006, p. 6). However, as stated by Mangiron and O’Hagan (2006), game translators sometimes include cultural references, jokes and linguistic variation even when such elements are not present in the original text, leaving a creative and authorial imprint in the target text, which could be considered creating something from scratch. Although the conceptual and terminological debate regarding translation and tran screation is still ongoing, the term transcreation seems to be rooted both in the industry and Translation Studies. It has become predominant in the fields of marketing and adver tisement (Pedersen, 2017; Rike, 2013) and game localisation (see, among others, Man giron & O’Hagan, 2006; Fernández Costales, 2012; O’Hagan 2012; Czech 2013; O’Hagan & Mangiron 2013; Iaia 2016; Zhang 2021), although it can be applied to any type of translation (Gaballo, 2012). In this paper, transcreation is understood as a trans lation strategy that is used within a broader localisation project to adapt the original content to the target culture in order to achieve a similar reception of certain in-game elements and effects by the target players. 3. GPT technologies for translation purposes Due to their multilingual data training (Devlin et al., 2018) and context awareness (Radford et al., 2019), GPT technologies can accurately generate, translate, summarise and analyse both general and specialised texts (Brown et al., 2020). The field of GPT- powered translation is relatively nascent, but there have already been several encouraging research outcomes. Raunak et al. (2023a) found that large language models like GPT-3 produced less literal but qualitatively comparable machine translations from English compared to standard neural MT models, especially for sentences with idiomatic expressions. Another paper by Raunak et al. (2023b) explored the implementation of GPT-4 to automatically post-edit neural machine translation outputs, resulting in improved quality and fixed errors, but also hallucinated edits. Hendy et al. (2023) found that GPT models achieve very competitive translation quality for high-resource languages, but were limited when dealing with low-resource languages, while Jiao et al. tested GPT-4, the most advanced OpenAI model at the time of writing, finding that translation performance ‘is significantly boosted, becoming comparable to commercial translation products, even for distant languages’ (2023a, p. 6). In relation to interactive MT, Gao, Wang and Hou (2023) explored how to boost GPT machine translation performance via prompt engineering, noticing significant perform ance increases. Lyu, Xu and Wang (2023) suggest new directions for machine translation (MT) using LLMs. Relevant to this paper, interactive MT is defined as a workflow that allows trans lators to ‘actively participate in the translation process, either by correcting or refining automatic translations or by providing feedback on the translation quality’ (ibid, p. 2). This translation method allows interaction and feedback between models and users, and can be implemented via GPT chatbots. The term prompt-based conversational machine translation (PCMT) is proposed here to refer to Lyu, Xu and Wang’s definition PERSPECTIVES 5 of this method, to distinguish it from previous definitions of interactive MT, such as in the case of the software LILT, where ‘suggestions change with user actions and the system learns from edits made by the user’ (Daems and Macken, 2019, p. 121). 4. Methodology We will focus on Pettini’s (2021) first category of irrealia, individual and collective refer ences in the form of proper nouns, and, more specifically, individual references in the form of proper nouns of Pokémon creatures. Several examples of relevant irrealia were extracted from the Pokémon video game saga, due to their highly creative nature. As the main method of interaction of users-cum-translators with these models are prompts, this paper explores instruction writing for transcreation and presents use cases for such instructions. The methods employed to perform this exploratory study were prompt engineering and iterative prompt development. Prompt engineering is the process of designing and refining input prompts to guide AI language models in gen erating more accurate, relevant, and useful responses. GPT best practices, instantiated via certain tactics such as temperature modification and control codes, were referred to during our explorations (OpenAI, 2023a). As recent studies have found, the quality and composition of the prompt can significantly impact the output generated by the model, which seems to also apply in the generation of translated output (Gao, Wang and Hou, 2023; Jiao et al., 2023). Iterative prompt development is a technique used to interact with GPT models to obtain more accurate and relevant output (Wei et al., 2022). Instead of relying on a single prompt, iterative prompting involves providing the model with a series of prompts, which are refined and built upon the generated output. It is particularly useful for complex tasks, such as transcreation, where preserving the meaning, style, and cultural nuances of a text are crucial and where the ideal output might not be obtained with a single prompt. It is a process of iterative refinement that might include rephrasing, expanding or providing more context. In this paper, the exploration of GPT-4’s potential has been divided into prompting tactics and prompting use cases for irrealia. All the examples included in the results section are output from OpenAI’s GPT-4, accessed via Poe1 from May to June 2023, with a monthly subscription. GPT-4 was selected over the 3.5 model due to its superior translational output quality, as detected by previous research (OpenAI, 2023b). Whenever chained prompting was not required, Poe’s GPT-4 clear context functionality, which erases the conversational context the bot saves from previous inputs, was used to ensure that previous conversations did not influence upcoming responses. Regarding the languages involved, all are high-resource languages with substantial data used for GPT-4 training. In terms of output, the source language was English, and the target languages were Spanish, French and Chinese, the working languages of the researchers, without specifying varieties or dialects. It should be explained that although the Spanish versions of the Pokémon games did not follow a transcreative approach for the translation of pokémonikers – the official term for Pokémon proper names – and kept the English translations, the Spanish language has been included, because of its potential for the training of video game localisers. 6 L. D. MORENO GARCÍA AND C. MANGIRON Prompts were improved, but not normally retried to avoid confirmation bias and cherry-picked results. Albeit certain prompts were retried when the model failed to provide correct output, output from such retries was not included. The transcreation outputs generated by the GPT-4 model for the selected game elements are presented here as is and briefly discussed, focusing on the potential utility of GPT-4 for transcrea tion in the context of game localisation. 5. Results The main results of the study, including general prompting tactics and prompting use cases for irrealia in the specific context of game localisation, are presented in this section. 5.1. Prompting tactics This section presents the procedure followed for single prompting, chained prompting, and other prompting tactics, such as using temperature and control codes. 5.1.1. Single prompting and chained prompting The Pokémon franchise is centred on fictional creatures called Pokémon that possess unique physical and magical traits. The Japanese and English names of Pokémon are typically compound words or portmanteaus made up of two or more words. The mean ings contained inside pokémonikers have been adapted by localisers into several languages (Miller, 2022), striving to retain both symbolism, sound and meaning in their transcreations (Shih et al., 2019). The creation of these sets of irrealia requires careful linguistic research, time, imagination, and creativity. One straightforward approach to generate transcreative output is to input a single prompt in natural language (see Table 1). The GPT-4 model is capable of producing tran screations by being conditioned on instructions that prompt creative translations or tran screations, without requiring any specific examples. This functionality is referred to as zero-shot prompting (Kojima et al., 2022). All the text included in the following tables was provided by GPT-4. Table 1. Translation of the name Cyndaquil via GPT-4 single prompting functionality. Prompt: Provide creative translations in Spanish, French and Chinese for a porcupine-like fire creature named Cyndaquil. Output: In each language, I’ve provided a creative translation that combines elements of the words for “porcupine” and “fire” or “burning” to create a unique name for the creature. Spanish: Puercofuego “Puercoespín” means porcupine, and “fuego” means fire. French: Héribrûle “Hérisson” means hedgehog (closest to porcupine), and “brûler” means to burn. Chinese: 火刺猬 (Huǒ Cìwèi) “火” (huǒ) means fire, and “刺猬” (cìwèi) means hedgehog (closest to porcupine). Please note that the Chinese name uses characters that represent the words for “fire” and “hedgehog” directly, while the names in the other languages are more creative adaptations. For example, the name Cyndaquil is a portmanteau of ‘cinder’ and ‘quill’, the latter referencing porcupines, which evokes in English the idea of a fiery, spiky creature. The prompt solicits inventive translations in multiple languages, specifies the name to be transcreated, and provides a concise description of the creature’s appearance and type. PERSPECTIVES 7 The resulting output consisted of a list of transcreations in each of the specified languages, presented in the order provided in the input prompt. The output also included explanations of the components of the newly created words, with more detailed expla nations given in the case of Chinese. Although single prompting can be useful, it is limited in its ability to provide the desired results in the case of transcreation due to the linguistic and cultural complexities of the task at hand. Chaining prompts may be used to obtain more relevant and varied transcreative output. The output of a previous prompt can be used as input and context for a subsequent prompt. For instance, in this scenario, the model is prompted (see Tables 2 and 3) with a series of simple prompts to generate more imaginative translations. Table 2. Translation of the name Cyndaquil via GPT-4 chained prompting functionality. Chained Prompt 1: Provide another set of yet even more creative translations. Output: Here’s a new set of creative translations inspired by the concept of a porcupine-like fire creature: Spanish: Espinoardiente “Espino” means thorn (resembling porcupine quills), and “ardiente” means burning. French: Flammépine “Flamme” means flame, and “épine” means spine or thorn (resembling porcupine quills). Chinese: 炽焰刺猬 (Chìyàn Cìwèi) “炽焰” (chìyàn) means blazing flame, and “刺猬” (cìwèi) means hedgehog (closest to porcupine). These new translations incorporate additional creative elements while still conveying the idea of a porcupine-like fire creature. Table 3. Translation of the name Cyndaquil via GPT-4 chained prompting functionality requesting a more creative translation. Chained Prompt 2: Still not creative enough. Output: I apologize for not meeting your expectations. Here’s another set of even more creative translations for a porcupine-like fire creature: Spanish: Fuegoquillamba Combining “fuego” (fire), “quilla” (keel, which suggests the shape of quills), and “llama” (flame). French: Étincepik Merging “étincelle” (spark) and “pik” (an adaptation from “pic,” meaning spike or peak, to resemble quills). Chinese: 火焰箭猬 (Huǒyàn Jiàn Wèi) Combining “火焰” (huǒyàn, flames), “箭” (jiàn, arrow, suggesting the shape of quills), and “猬” (wèi, a less common character for hedgehog). These translations are more inventive and less literal, aiming to capture the essence of a porcupine-like fire creature while providing a unique name in each language. The second output (Table 2) appears to deviate more from the original meanings of the Pokémoniker’s components, while the third (Table 3) combines three words instead of two. This approach of chaining prompts can be further extended to achieve more desirable transcreation levels, as needed. However, a risk of higher hallucinations was detected when forcing more creative outputs. For example, the Spanish translation in chained prompt 2 includes ‘llamba’ (non-sensical), instead of ‘llama’ (fire), as well as ‘quilla’, an uncommon word the meaning of which might not be easily recognised by average players. 5.1.2. Other prompting tactics The previous prompts followed a conversational approach to modifying transcreational output. However, the creativity of the output can be technically adjusted using various prompt engineering techniques, such as temperature or control codes. ‘Temperature’ is a parameter that controls the randomness of the model’s output during text generation 8 L. D. MORENO GARCÍA AND C. MANGIRON (OpenAI Developer Forum, 2022). By adjusting the temperature parameter, the model can produce transcreations that are either more conservative or more creative, depending on the specific requirements. In this particular instance (see Table 4), the model was instructed, via three separate prompts, to transcreate the name ‘Tentacool’ using a temperature value of 0.1, 0.5 and 1.0, resulting in transcreations with varied creativity. The specific prompts used were ‘Transcreate the name Tentacool to Spanish, French and Chinese. Temperature: 0.1’, ‘Transcreate the name Tentacool to Spanish, French and Chinese. Temperature: 0.5’ and ‘Transcreate the name Tentacool to Spanish, French and Chinese. Temperature: 1.0’. Table 4. Translation of Tentacool via GPT-4 using the temperature functionality. Temperature Merged output 0.1 Tentáculo/Tentacule/触手(tentacle) 0.5 Medusajel/GeléePsy/水母灵 (jellyfish spirit) 1.0 Psiquimarea/Aquaméduse /心浪蜇 (heart/mindwave jellyfish) The model may struggle when merging two words. For example, the 0.5 French output of ‘GeléePsy’ shows the second component capitalised, which is not common practice in the case of Pokémonikers. Moreover, the words ‘medusa’ and ‘gel’ were combined for the medium creativity Spanish transcreation, but ‘g’ may have been mis takenly transformed into a ‘j’, as this contravenes Spanish spelling rules. However, we cannot say for certain, as there are cases of official Pokémon names with incorrect spel ling, such as Eelektrik (instead of Eelectric). On a different note, the recurrence of psychic elements in the translations (psy, psiqui-, 心) might be explained by the fact that, although Tentacool is Water/Poison, it does have a Water/Psychic Relic form, which shows how the model exploits its background knowledge and leverages it for rel evant output. In addition, control codes, which are special tokens that can be inserted into prompts to provide guidance to GPT models, can also be used to fine-tune the translations or to look for synonyms with specific connotations. For example, when dealing with irrealia expected to contain either positive or negative undertones, control codes such as [POS] or [NEG] can be used, as shown in Table 5. Similar effects could also be reached by using natural language (i.e.: with a positive/negative tone/twist) instead of control codes. In order to obtain interlingual translations, languages may be specified as part of the prompt. Table 5. Use of control codes in GPT-4. Prompt: Provide 3 Pokémon transcreations [NEG] Output: I understand that you’re looking for transcreations of Pokémon names with a negative twist. Here are three examples: Charmander -> CharFlander Original meaning: Char (burn) + Salamander Transcreation: Char (burn) + Flander (derived from flounder, meaning to struggle) Negative twist: This Pokémon struggles with its fire abilities and may not be as effective in battles. Jigglypuff -> GloomyPuff Original meaning: Jiggly (soft, wiggly) + Puff (round, inflated) Transcreation: Gloomy (unhappy, dark) + Puff (round, inflated) Negative twist: This Pokémon is known for its melancholic demeanor and tends to dampen the mood when it appears. PERSPECTIVES 9 Pikachu -> Pikaboo Original meaning: Pika (onomatopoeic for a mouse’s sound) + Chu (onomatopoeic for a mouse’s sound in Japanese) Transcreation: Pika (onomatopoeic for a mouse’s sound) + Boo (expression to scare someone) Negative twist: This Pokémon is known for startling others with its sudden and unpredictable appearances. Prompt: Provide 3 Pokémon transcreations [POS] Output: I understand that you’re looking for transcreations of Pokémon names with a positive twist. Here are three examples: Charmander -> Flameander Original meaning: Char (burn) + Salamander Transcreation: Flame (fire) + Salamander Positive twist: This Pokémon is known for its impressive fire abilities and is highly effective in battles. Jigglypuff -> BreezyPuff Original meaning: Jiggly (soft, wiggly) + Puff (round, inflated) Transcreation: Breezy (light, carefree) + Puff (round, inflated) Positive twist: This Pokémon has a cheerful and uplifting presence, which can brighten the mood wherever it goes. Pikachu -> PikaJoy Original meaning: Pika (onomatopoeic for a mouse’s sound) + Chu (onomatopoeic for a mouse’s sound in Japanese) Transcreation: Pika (onomatopoeic for a mouse’s sound) + Joy (happiness, delight) Positive twist: This Pokémon is known for its joyful and playful nature, bringing happiness to those around it. Another useful technique for transcreation are mask tokens, which allow specific parts or subparts of an input sequence to be masked out. In the context of Pokémon name transcreation, mask tokens can be employed as wildcards to generate transcreations that incorporate particular elements. For example, in this scenario, the model is prompted to transcreate ‘Bulbasaur’ in Spanish using ‘saurio’ as the ending element, while generating its own unique first component of the Pokémoniker. The results are presented in Table 6. Table 6. Use of mask tokens in GPT-4. Prompt: Provide 3 Spanish transcreations of the Pokémon character “Bulbasaur” with the following structure “[MASK]saurio”. Output: Here are three Spanish transcreations of Bulbasaur using the requested structure: 1. Brotesaurio 2. Plantasaurio 3. Germinosaurio These transcreations attempt to capture the essence of Bulbasaur, a grass-type Pokémon with a plant bulb on its back, by incorporating elements related to plant growth and botanical concepts. 5.2. Prompting use cases for irrealia Prompts can also be utilised to overcome specific challenges or achieve particular objec tives in transcreation tasks. For instance, prompts can be used to compare different irrea lia-related output, create a cohesive feeling among transcreations, handle rhetorical figures, support decision-making, check transcreational output, etc., as further explained in the next subsections. 5.2.1. Comparison and brainstorming GPT-4 can also be instructed to generate multiple transcreations for the same irrealia simultaneously, providing a range of possible options for further consideration. In this example, GPT-4 is prompted to produce three Spanish (see Table 7) and three Chinese names (see Table 8) for the Pokémon Pikachu, offering different levels of transcreation. 10 L. D. MORENO GARCÍA AND C. MANGIRON Table 7. Gradation of creative translations of Pikachu into Spanish. Prompt: Provide 3 different transcreations, from less creative to more creative, for the Pokémon Pikachu in Spanish, taking into account the traits of this Pokémon. Output: Here are three different transcreations for the Pokémon Pikachu in Spanish, ranging from more creative to less creative, based on the traits of being an electric mouse Pokémon: Chisparatón (combining “chispa” for “spark” and “ratón” for “mouse”) Relámpagón (combining “relámpago” for “lightning” and “ratón” for “mouse”) Electroratín (combining “electro” for “electric” and “ratín” for “little mouse”) Please note that these are original transcreations and not the official names used in the Spanish version of Pokémon. In the official Spanish localisation, Pikachu retains its original name. Table 8. Gradation of creative translations of Pikachu into Chinese. Prompt: Provide 3 different transcreations, from less creative to more creative, for the Pokémon Pikachu in Chinese, taking into account the traits of this Pokémon. Output: Here are three different transcreations for the Pokémon Pikachu in Chinese, ranging from more creative to less creative, based on the traits of being an electric mouse Pokémon: 闪电鼠 (shǎndiàn shŭ) (combining “闪电” for “lightning” and “鼠” for “mouse”) 电花鼠 (diànhuā shŭ) (combining “电花” for “electric spark” and “鼠” for “mouse”) 电鼠宝宝 (diàn shŭ bǎobǎo) (combining “电鼠” for “electric mouse” and “宝宝” for “baby”) Please note that these are original transcreations and not the official names used in the Chinese version of Pokémon. In the official Chinese localisation, Pikachu is called 皮卡丘 (píkǎqiū). These transcreative options can be compared, and the preferred choice, if any, can be selected. The explanations of the constituent parts of the transcreated name can assist in further inspiring transcreators. However, mistakes may occur when creating portmanteaus, as in the case of the double accent in ‘Relámpagón’, which is not possible in Spanish (see Table 7). 5.2.2. Consistency and cohesiveness As irrealia are integral components of a unified fictional world, preserving coherence is of utmost importance. Video games encompass a variety of in-game elements that evolve, as well as fictional languages employed to name locations and individuals (Furtak, 2019). Customised prompts can generate transcreated names that maintain common elements (see Table 9), thereby fostering a cohesive feel among interconnected irrealia and promoting world consistency. Table 9. Examples of consistency and cohesiveness. Prompt: Provide transcreations for the names “Charmander”, “Charmeleon” and “Charizard”, which are from the same Pokémon during different stages. You should keep common elements in all the names so that players know that they are the same Pokémon in different stages of evolution. Do this in Spanish, French and Chinese. Output: In order to create transcreations that maintain the meaning and charm of the original English names while also being appealing and understandable in each target language, I have come up with the following suggestions: Spanish: 1. Charmander: Brasillama 2. Charmeleon: Brasileón 3. Charizard: Brasidraco French: 1. Charmander: Flamézard 2. Charmeleon: Flaméléon 3. Charizard: Flamédraco Chinese (Simplified): 1. Charmander: 火蜥火 (Huǒ xīhuǒ) 2. Charmeleon: 火炎蜥 (Huǒyán xī) 3. Charizard: 火焰龙 (Huǒyàn lóng) In each language, the transcreations keep a common element to show that they are the same Pokémon in different stages of evolution: “Brasi-” in Spanish, “Flamé-” in French, and “火” (meaning “fire”) in Chinese. The second part of each name refers to the creature’s appearance or characteristics, using words related to lizards, lions, or dragons. PERSPECTIVES 11 This example demonstrates how GPT-4 can be utilised to transcreate interconnected irrealia. In this scenario, three related Pokémon are creatively translated into three languages. By specifying that the transcreations should have shared elements, the desired output is achieved. The model also clarifies what these common elements are, increasing the usefulness of the output from a transcreational perspective. However, as seen previously, the output shows certain issues, such as incongruent double accents in the case of the French portmanteau ‘Flaméléon’ and a non-sensical translation of Charmander in Chinese as 火蜥火(fire lizard’s fire). 5.2.3. Constrained localisation The term constrained translation here refers to the practice of translating in-game text while adhering to specific constraints or guidelines. In game localisation, these con straints may include preserving playability through language, ensuring that translations fit the available screen space, or maintaining consistency with established terminology or character names. The summarising features of GPT technology could be employed to deal with space limitations in relation to irrealia. In many occasions, especially in mobile games, text expansion can be a problem and a reduction of character length is required to avoid display problems, such as overlapping, truncations, container overflow, etc. (O’Hagan & Mangiron, 2013). Character limits can be prompted to control the length of the translated output (see Table 10). Table 10. Translating with character limitations. Prompt 1: Translate into Spanish and condensate the following with a 12-character limit: “Thunder Punch”, “Extreme Speed”, “Pokémon Black and White”. Output 1: GolpeTrueno, VelExtrema, PKMN-B&W Prompt 2: Translate into Spanish and condensate the following with a 10-character limit: “Thunder Punch”, “Extreme Speed”, “Pokémon Black and White”. Output 2: TruenoPñ, VelExtrem, PokéBN The prompt followed the tactic of using delimiters to indicate distinct parts of the input, which included Pokémon attacks and the titles of a Pokémon game paired version. The source text was included after a colon, each string was separated with commas, contained between inverted commas. Notwithstanding that fact, the first output may show language confusion (B&W instead of B&N), as the title of this Pokémon video game was translated into Spanish and not retained in English, which has been common practice for Pokémon entries named after colours. The second output shows how the model manages to condensate accurately, even within 10 charac ters, although the proposed terms would probably not be used in a professional trans lation environment due to the fact that in game localisation abbreviations are often avoided (O’Hagan and Mangiron, 2013, p. 122). In addition, in the first case, GPT-4 has kept ‘Thunder’ as the first element of the portmanteau in Spanish, ‘Trueno’, when it should go in second place, as it modifies ‘Punch’. According to the literature, more complex prompts have shown better results for complex tasks related to language, logic or mathematics (Espejel et al., 2023). However, in this study prompts which asked the model to not only translate and condense, but 12 L. D. MORENO GARCÍA AND C. MANGIRON also to explain what was condensed and why, did not manage to make the model output correctly during the first try (Table 11), nor during other subsequent attempts. Table 11. Output of constrained translation tasks with more complex prompts. Prompt: Translate into Spanish and condensate the following with a 12-character limit: “Thunder Punch”, “Extreme Speed”, “Pokémon Black and White”. Explain what was condensed and why. Output: 1. “Trueno Puñ” 2. “Velo. Extremo” 3. “Poké BNyB” Explicación: “Thunder Punch” se traduce como “Puño Trueno”, pero se acortó a “Trueno Puñ” para respetar el límite de 12 caracteres. “Extreme Speed” se traduce como “Velocidad Extrema”. Se abrevió a “Velo. Extremo” para cumplir con el límite. “Pokémon Black and White” se traduce como “Pokémon Negro y Blanco”. Se acortó a “Poké BNyB”, utilizando las iniciales de Negro y Blanco (N y B) y uniendo con “y” para indicar “y”. As can be seen, there are several errors in the output, as well as a language switch in the explanation section. Errors include keeping the English word order in ‘Trueno Puñ’, as well as using a strange abbreviation, ‘Puñ’. Another error can be found in ‘Extremo’, where there is a lack of concordance between noun and adjective, as ‘velocidad’ is a feminine noun in Spanish. In the third case, there is a problem with ‘BNyB’, which seems to have mixed ‘Blanco’ (white) (abbreviated by GPT4 as BN), in Spanish, and ‘Black’ (B), in English. Such errors would not be present in human output. This highlights the potential issue of ran domness in the output generated by GPT-4 when dealing with constrained translation tasks. 6. Findings and discussion This exploratory study about the potential implementation of GPT chatbots in game localisation yields several findings. First, the GPT-4 model shows a similar understanding of the keywords ‘transcreation’ and ‘creative translation’, as the inclusion of such con cepts in prompts seems to provide similar results in terms of output. After being asked why its output is similar when including those commands as part of input, GPT-4 replied ‘while these terms have different emphases, they all share a common goal: to ensure that the content is engaging, appropriate, and effective for the target audi ence’ (GPT-4, June 2023). Also, apart from the capability to prompt the GPT-4 model to provide transcreative options with general, single prompting, prompts can be also fine- tuned via iterative prompting and specific prompting techniques, such as temperature modification, control codes and mask tokens. In particular, the model did not perform well when faced with complex prompts that involved character constraints typically present in, for example, mobile game localisation. The results show a degree of potential for the translation and transcreation of certain types of irrealia, specifically individual and collective references with semantical charge. In general, the model is capable of generating sensible portmanteaus for fictional crea tures. Results show that the GPT-4 model is capable of providing multilingual output in a structured manner. The output for pokémonikers seems to mostly follow the struc ture [Introduction-transcreation-explanation-summary/caveat]. GPT-4 is prone to offer explanations when asked to transcreate irrealia that contain meaning. The explanatory output tends to be written in the input language and includes key words used, which may help translators understand the components that conform pokémonikers. PERSPECTIVES 13 In relation to its background knowledge, the GPT-4 model provided caveats, explain ing that the irrealia created were not official, whenever official translations were included as part of the training data. Moreover, GPT-4 demonstrated having information of certain Pokémon descriptions, abilities and types, and leveraging such information to provide relevant output. Moreover, GPT-4 was aware of the fact that the names of Pokémon creatures have not been translated into the Spanish versions of the game. The fact that no official versions of the Pokémonikers exist in Spanish makes the adapted portmanteaus ideal candidates for the teaching of game localisation, as there are no official translations students can refer to. Students can also experiment with the options offered by GPT-4 and compare them with their own. The GPT-4 model seems to have the capacity to support the tackling of transcreation- related challenges, such as brainstorming, comparison and cohesiveness, although results are not always satisfactory and there are errors, including conceptual repetition, format ting issues and incongruent or uncommon portmanteaus. As far as the quality of the output is concerned, the English-to-Chinese combination seems less creative in compari son to the adaptations from English into French or Spanish. However, further compara tive studies of a qualitative and quantitative nature, as well as a reception study with users to find out about their preferred translations, are required to come to any conclusions in this respect, as creativity is a very subjective matter. Notwithstanding the positive results obtained, the GPT-4 model output and its per formance seem strongly influenced by the specific arrangement of tokens. This intro duces challenges for translation and transcreation, particularly with regard to maintaining consistency, ensuring replicability in research outputs and developing best practices, as detected by previous scholars (Raunak et al., 2023a). In the near future, the context-awareness, multilingual capabilities and creative nature of GPT-based chatbots may assist translators in generating appropriate translations for certain irrealia for specific locales. Additionally, advanced models could prove valuable in assessing the linguistic and cultural appropriateness of inputs (Raunak et al., 2023b). However, there are certain limitations and concerns that need to be taken into account. Firstly, the limited context windows of available models restrict the amount of context that can be primed to improve consistency. This can lead to transcreations that deviate from previous work or are incongruous with the video game’s setting. Context windows may restrict the ability of the model to capture long-range dependencies and patterns in the data, thus limiting the amount of chained prompting possible. Models that include bigger context windows such as Gemini 1.5 Pro might prove more useful in this respect. Moreover, GTP models may also produce hallucinated or non-sensical output (Raunak et al. 2023a, 2023b) or include grammar or spelling mistakes, as seen in tables 3, 4, 7, 9, 10 and 11. GPT models may also fail to be aware of culturally sensitive issues. Their output could help perpetuate, replicate and even reinforce stereotypes and biases that were present in their training data, leading to inappropriate output (Ferrara, 2023). In relation to this, irrealia may well be words that lack semantic content, that do not correspond to any real-world referent, or that are part of fictional languages that have not been included in the training data. Models may thus lack the ability to handle, transform or transcreate them. Human-GPT collaboration is highly encouraged to address these concerns and ensure that the generated content is accurate, respectful, inclusive, and free of harmful biases. 14 L. D. MORENO GARCÍA AND C. MANGIRON In relation to training data, an additional challenge involves the varying quantities of data available in different languages, which subsequently influences the quality of results for specific language pairings when performing translation and cultural adaptation. As regards Spanish, since the names of Pokémon creatures have not been localised and retain their English names, the model could not pull from that pool of knowledge. This is different to the case of French and Chinese, where Pokémon names were adapted in the official versions. In addition, legal and copyright issues, as well as ethical implications, represent additional factors that discourage GPT usage for transcreation. GPT models run the risks of infringing upon copyright laws and violating intellectual property rights, which may expose users to financial and legal repercussions. Although opting out of input data sharing is a possibility offered by some AI companies (OpenAI, 2023c), the potential use of input data for model training represents another significant concern that dissuades the use of GPT for the translation of sensitive and copyrighted content. The authorship of transcreations that have been created with some help from the model is also another contentious point of debate, as is the potential of this technology to displace human agency during creative practices. Yet another critical challenge that may impede the practicality of implementing GPT for transcreation pertains to access and model limitations. The most advanced GPT models are currently restricted by paywalls, and the number of monthly messages permitted by the more powerful models is limited. Moreover, disparity in access could potentially affect the market and workforce. Furthermore, the non-deterministic nature of the model may result in outputs that are inconsistent or unexpected, which can pose challenges for a highly-precise task such as translation. Another factor that needs to be taken into account is efficiency and productivity. Prompting and fine-tuning is a time-consuming endeavour, and it remains to be seen whether PCMT could increase productivity or not, especially in the case of seasoned translators who have experience in transcreation. Experimental studies with translators, tracking their progress and productivity, as well as probing their opinions about PCMT, would be necessary to confirm whether GPT models can be useful for translating highly creative content in a professional game localisation context with tight deadlines. All these issues must be taken into account when considering the feasibility of utilising GPT for transcreation tasks, as the above-mentioned constraints can impact output quality, deployment feasibility, and the level of appropriateness of using GPT models for transcreation in video game localisation. PCMT could be useful for translation train ing and for translators who have just started, while it may not be useful to experienced professional game localisers, who may come up with creative solutions faster. In the game localisation classroom, this tool could be used for brainstorming sessions to gain a sense of transcreation of cultural concepts and to provide students with strategies to think about creative names. In the future, comparative studies involving students can be con ducted to evaluate its potential, with one group translating highly creative content from scratch and the other using GPT as a tool to aid in the process. Finally, the limitations of this study should be taken into consideration. Firstly, the GPT-4 model is a developing black box, and there is no information available regarding the amount or types of irrealia used to train the model. The model may be updated, fine- tuned or downgraded at any time without any previous notification. This opacity, along PERSPECTIVES 15 with output randomness, limits the interpretability and applicability of the model, as well as the replicability of results. Secondly, the exploratory analysis conducted in the study is mostly limited to portmanteaus that combine the meanings of two existing words to gen erate pokémonikers. Further investigations that include a wider range of irrealia types are necessary to fully explore the capabilities and limitations of GPT-4 for irrealia recreation. Additionally, the effectiveness of GPT-4 for dealing with irrealia coming from other fran chises or contexts remains to be investigated. 7. Conclusion By exploring various transcreation strategies and tactics in relation to irrealia transcrea tion, this study has shed light on possibilities of GPT-powered localisation and transcrea tion. GPT technologies can serve as a tool by offering suggestions and alternatives for irrealia recreation, translation, and adaptation (Brown et al., 2020). By leveraging the capabilities of GPT models, trainee and novel localisation professionals may be able to enhance their productivity and creativity while maintaining control over the output via prompt-based conversational machine transcreation. The successful recreation of irrealia can help provide immersive experiences for players within fictional worlds. GPT technologies may be leveraged to generate multilin gual content that displays different levels of creativity. In conjunction with human exper tise and human creative power, these tools can assist with irrealia translation. The potential for these tools in transcreation is expected to significantly increase with the incorporation of multimodal input and output capabilities, such as those of GPT-4V, and the general improvement of the models. The integration of visual elements, such as images and videos, is anticipated to bring both new challenges and possibilities in terms of transcreative outputs. Experimental studies examining the speed, performance, and productivity of transla tors with varying levels of experience and backgrounds would be necessary to check whether using GPT for transcreation could contribute to increasing their productivity. Additionally, investigating player reception of textual content adapted by GPT is another promising avenue for future research, which will be explored in subsequent stages of the study. Future studies will also aim at probing human-machine interactions during transcrea tive practices in the field of game localisation, experimenting with new prompt engineer ing techniques, exploring GPT 4’s capability to deal with localisation constraints, rhetorical figures or wordplay, prompting models to generate output in specific language varieties or dialects, investigating the impact of such tools in transcreation, and drafting best practices for transcreation via prompt engineering. Note 1. Poe is a service that provides access to several chatbots from different AI companies (www. poe.com). Disclosure statement No potential conflict of interest was reported by the author(s). 16 L. D. MORENO GARCÍA AND C. MANGIRON Funding Luis Damián Moreno García’s contribution to this work is supported by Hong Kong Baptist Uni versity. Carme Mangiron’s contribution to this work is supported by the project 2021SGR00077, funded by the Generalitat de Catalunya. Notes on contributors Luis Damián Moreno García, PhD and FHEA, is an assistant professor at Hong Kong Baptist Uni versity, a Chinese-Spanish freelance translator specialised in film subtitling and game localisation, and an English-Spanish sworn translator. He holds a BA in Spanish-English Translation, an MA in Multimedia Translation, an MA in Teaching Spanish as a Foreign Language and a PhD in Audio visual Translation. His teaching and research interests lie in the fields of software localisation, translation technologies and audiovisual translation from and into Mandarin Chinese, Cantonese, English and Spanish. His research has been published in reputed journals such as Babel, Perspec tives, Translation, Cognition & Behavior and Translation Spaces, as well as part of edited volumes by Routledge and Palgrave Macmillan. Carme Mangiron, PhD, is an Assistant Professor, a member of the research group TransMedia Catalonia and a member of the knowledge transference network AccessCat at the Universitat Autònoma de Barcelona (UAB). She was awarded the Excellence in Teaching Award at UAB in 2022. She has extensive experience as a translator, specialising in software and game localisation. Her main research areas are game localisation, game accessibility, audiovisual translation and accessibility to the media. She has published extensively in international journals and participated in several national and international research projects. She is currently one of the PIs of the WEL project (From written to oral texts in Easy Language: easy audios in cultural visits and video games, PID2022-137058NB-I00). She is co-author of Game Localization: Translat ing for the Global Digital Entertainment Industry (O’Hagan and Mangiron, 2013), and the main organiser of the Fun for All Conference, about game translation and accessibility, which is held at UAB every two years. ORCID Luis Damián Moreno García http://orcid.org/0000-0003-1046-8574 Carme Mangiron http://orcid.org/0000-0002-6421-8581 References Benetello, C. (2017). Transcreation as the creation of a new original. A Norton™ case study. In Cordisco, M., Di Martino, E., Bogenç Demirel, E., Le Disez, J-Y., Regattin, F., Segers, W. (Eds). (2017). Exploring creativity in translation across cultures (pp. 237-247). Roma: Aracne. Bernal-Merino, M. Á. (2006). On the translation of video games. JosTrans, 6, 22–36. Bernal-Merino, M. Á. (2007). Challenges in the translation of video games. Revista Tradumática, 5, 1–7. Bernal-Merino, M. Á. (2008). Creativity in the translation of video games. Quaderns de Filologia: Estudis Literaris, 13, 57–70. Brown, T. B., Mann, B., Ryder, N., Subbiah, M., Kaplan, J., Dhariwal, P., Neelakantan, A., Shyam, P., Sastry, G., Askell, A., Agarwal, S., Herbert-Voss, A., Krueger, G., Henighan, T., Child, R., Ramesh, A., Ziegler, D. M., Wu, J., Winter, C., … Amodei, D. (2020). Language models are few-shot learners. arXiv:2005.14165. Cao, Y., Kementchedjhieva, Y., Cui, R., Karamolegkou, A., Zhou, L., Dare, M., Donatelli, L., & Hershcovich, D. (2024). Cultural adaptation of recipes. Transactions of the Association for Computational Linguistics, 12, 80–99. https://doi.org/10.1162/tacl_a_00634 PERSPECTIVES 17 Czech, D. (2013). Challenges in video game localization: An integrated perspective. Explorations: A Journal of Language and Literature, 1, 3–25. Daems, J., & Macken, L. (2019). Interactive adaptive SMT versus interactive adaptive NMT: A user experi ence evaluation. Machine Translation, 33(1/2), 117–134. https://doi.org/10.1007/s10590-019-09230-z Devlin, J., Chang, M. W., Lee, K., & Toutanova, K. (2018). BERT: Pre-training of deep bidirec tional transformers for language understanding. arXiv:1810.04805. Espejel, J. L., Ettifouri, E. H., Alassan, M. S. Y., Chouham, E. M., & Dahhane, W. (2023). GPT-3.5, GPT-4, or BARD? Evaluating LLMs reasoning ability in zero-shot setting and performance boosting through prompts. Natural Language Processing Journal, 5, 100032. https://doi.org/ 10.1016/j.nlp.2023.100032 Fernández Costales, A. (2012). Exploring translation strategies in video game localization. MonTI. Monografías de Traducción e Interpretación, (4), 385–408. https://doi.org/10.6035/MonTI.2012.4.16 Ferrara, E. (2023). Should ChatGPT be biased? Challenges and risks of bias in large language models. https://arxiv.org/pdf/2304.03738.pdf Furtak, K. (2019). Cultural representation in video games: Building worlds and identities. Routledge. Gaballo, V. (2012). Exploring the boundaries of transcreation in specialized translation. ESP Across Cultures, 9, 95–113. Gao, Y., Wang, R., & Hou, F. (2023). How to design translation prompts for ChatGPT: An empiri cal study. arXiv:2304.02182. Guzik, E., Byrge, C., & Gilde, C. (2023). The originality of machines: AI takes the torrance test. Journal of Creativity, 33(3), 1–8. https://doi.org/10.1016/j.yjoc.2023.100065 Hendy, A., Abdelrehim, M., Sharaf, A., Raunak, V., Gabr, M., Matsushita, H., … Awadalla, H. H. (2023). How good are gpt models at machine translation? A comprehensive evaluation. arXiv:2302.09210. Iaia, P. L. (2016). Creativity and readability in game localisation. A case study on “Ni No Kuni”. Lingue e Linguaggi, 17, 121–132. Jiao, W., Wang, W., Huang, J. T., Wang, X., & Tu, Z. (2023). Is ChatGPT a good translator? A preliminary study OR Yes With GPT-4 As The Engine. arXiv:2301.08745. Kojima, T., Gu, S. S., Reid, M., Matsuo, Y., & Iwasawa, Y. (2022). Large language models are zero- shot reasoners. arXiv:2205.11916. Lyu, C., Xu, J., & Wang, L. (2023). New trends in machine translation using large language models: Case examples with chatgpt. arXiv:2305.01181. Mangiron, C. (2007). Video games localisation: Posing new challenges to the translator. Perspectives, 14(4), 306–323. https://doi.org/10.1080/09076760708669046 Mangiron, C., & O’Hagan, M. (2006). Game localisation: Unleashing imagination with ‘restricted’ translation. The Journal of Specialised Translation, 6, 10–21. Méndez González, R. (2020). Specialized terminology in the video game industry: Neologisms and their translation. Vertimo Studijos, 12(12), 71–86. https://doi.org/10.15388/VertStud.2019.5 Miller, K. (2022). Would a Bulbasaur by any other name be as iconic? Analyzing motivations in Pokémon name localisation [Doctoral dissertation]. The Ohio State University. Newmark, P. (1995). Truth and culture in translation. Lebende Sprachen, 40(2), 49–51. https://doi. org/10.1515/les.1995.40.2.49 O’Hagan, M., & Mangiron, C. (2013). Game localisation: Translating for the global digital enter tainment industry. John Benjamins Publishing Co. O’Hagan, M. (2012). Transcreating Japanese video games: Exploring a future direction for trans lation studies in Japan. In N. Sato-Rossberg, & J. Wakabayashi (Eds.), Translation and trans lation studies in the Japanese context (pp. 183–201). Bloomsbury. O’Hagan, M. (2015). Game localisation as software-mediated cultural experience: Shedding light on the changing role of translation in intercultural communication in the digital age. Multilingua, 34(6), 747–771. OpenAI. (2023a). GPT best practices. https://platform.openai.com/docs/guides/gpt-best-practices? utm_source=www.theneurondaily.com&utm_medium=newsletter&utm_campaign=openai-s- chatgpt-tips OpenAI. (2023b). GPT-4 technical report. https://openai.com/research/gpt-4 18 L. D. MORENO GARCÍA AND C. MANGIRON OpenAI. (2023c). Enterprise privacy at OpenAI. https://openai.com/enterprise-privacy#:~:text= Does%20OpenAI%20train%20its%20models,outputs%20for%20training%20our%20models OpenAI Developer Forum. (2022). Mastering temperature and Top_p in ChatGPT API. https:// community.openai.com/t/cheat-sheet-mastering-temperature-and-top-p-in-chatgpt-api-a-few -tips-and-tricks-on-controlling-the-creativity-deterministic-output-of-prompt-responses/172 683 Pedersen, D. (2017). Translation practice in the field. Translation Spaces, 6(1), 44–61. https://doi. org/10.1075/ts.6.1.03ped Pettini, S. (2021). The translation of realia and irrealia in game localisation: Culture-specificity between realism and fictionality. Routledge. Radford, A., Wu, J., Child, R., Luan, D., Amodei, D., & Sutskever, I. (2019). Language models are unsupervised multitask learners. OpenAI, 1, 9. Raunak, V., Menezes, A., Post, M., & Awadallah, H. H. (2023a). Do GPTs produce less literal trans lations? https://doi.org/10.48550/arXiv.2305.16806 Raunak, V., Sharaf, A., Awadallah, H. H., & Menezes, A. (2023b). Leveraging GPT-4 for automatic translation post-editing. arXiv:2305.14878. Rike, S. M. (2013). Bilingual corporate websites-from translation to transcreation? Jostrans, 20, 68–85. Shih, S. S., Ackerman, J., Hermalin, N., Inkelas, S., Jang, H., Johnson, J., & Yu, A. (2019). Cross- linguistic and language-specific sound symbolism: Pokémonastics. Unpublished manuscript. University of Southern California, University of California, Merced, University of California, Berkeley, Keio University, National University of Singapore and University of Chicago. Singh, N., & Pereira, A. (2005). The culturally customized web site. Routledge. Wei, J., Tay, Y., Bommasani, R., Raffel, C., Zoph, B., Borgeaud, S., … Fedus, W. (2022). Emergent abilities of large language models. arXiv:2206.07682. Zhang, X. (2021). Video game localization: Translating interactive entertainment. In E. Bielsa (Ed.), The Routledge Handbook of Translation and Media (pp. 369–383). Routledge.