Elements of Analysis.pdf
Document Details
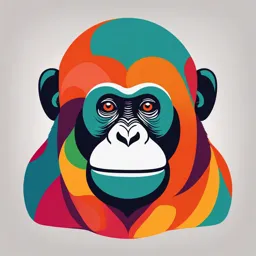
Uploaded by FasterBougainvillea
2011
Tags
Full Transcript
AACE International Certified Cost Technician Primer 1st Edition – January 2011 Updated September 2018 SUPPORTING SKILLS AND KNOWLEDGE OF A COST ENGINEER Based Upon AACE International R...
AACE International Certified Cost Technician Primer 1st Edition – January 2011 Updated September 2018 SUPPORTING SKILLS AND KNOWLEDGE OF A COST ENGINEER Based Upon AACE International Recommended Practice 11R‐88, Required Skills and Knowledge of Cost Engineering Part 1 Acknowledgments: Michael Pritchett, CCE CEP (Author) Pete Griesmyer Donald McDonald Jr., PE CCE PSP Valerie Venters, CCC Larry Dysert, CCC CEP Copyright 2011‐2018, AACE International, Inc. AACE International CCT Primer Population is the collection of all elements of interest to the decision‐maker. The size of the population is usually denoted by N. Very often, the population is so large that a complete census is out of the question. Sometimes, not even a small population can be examined entirely because it may be destructive or prohibitively expensive to obtain the data. Under these situations, we draw inferences based upon a part of the population (called a sample) [S&K 6th Ed., Chap 29]. Sample is a subset of data randomly selected from a population. The size of a sample is usually denoted by n [S&K 6th Ed., Chap 29]. Statistical inference is an estimation, prediction or generalization about the population based on the information from the sample [S&K 6th Ed., Chap 29]. Reliability is the measurement of the “goodness” of the inference [S&K 6th Ed., Chap 29]. 2. Descriptive Statistics 1. Basic Statistics: Mean (average) Mean is the sum of measurements divided by the number of measurements [S&K 6th Ed., Chap 29]. The best known and most reliable measure of central tendency is the mean. The mean is the arithmetic average of a group of scores [S&K 6th Ed., Chap 29]. Median Median is the middle number when the data observations are arranged in ascending or descending order. If the number n of measurements is even, the median is the average of the two middle measurements in the ranking [S&K 6th Ed., Chap 29]. The median is the middle point in a distribution. Half of the distribution is above this point and half is below. To find the median one arranges the scores in order [S&K 6th Ed., Chap 29]. The median of a set of numbers arranged in order of magnitude (i.e., in an array) is either the middle value (if the number of data values is odd) or the arithmetic mean of the two middle values (if the number of data values is even) [Schaum’s Statistics Crash Course, Murray R. Spiegel]. In a population or a sample, the median is the value that has just as many values above it as below it. If there is an even number of values, the median is the average of the two middle values. The median is a measure of central tendency. The median can also be defined as the 50th percentile. For symmetrical distributions the median coincides with the mean and the center of the distribution. For this reason, the median of a sample is often used as an estimator of the center of the distribution. If the distribution has heavier tails than the normal distribution, then the sample median is usually a more precise estimator of the distribution center than the sample mean [Statistics.com 2004‐ 2010]. Copyright 2011‐2018, AACE International, Inc. AACE International CCT Primer The median of a set of data is a value that divides the bottom 50 percent of the data from the top 50 percent of the data. To find the median of a data set, first arrange the data in increasing order. If the number of observations is odd, the median is the number in the middle of the ordered list. If the number of observations is even, the median is the mean of the two values closest to the middle of the ordered list [Schaum’s Beginning Statistics 2nd Edition, Larry J. Stephens, Ph.D.]. Mode Mode is the measurement that occurs most often in the data set. If the observations have two modes, the data set is said to have a bimodal distribution. When the data set is multi‐modal, the mode(s) is on longer a viable measure of the central tendency. In a large data set, the modal class is the class containing the largest frequency. The simplest way to define the mode will then be the midpoint of the modal class [S&K 6th Ed., Chap 29]. The mode is the simplest measure of central tendency. It is merely the score value or measure that occurs most often in a distribution of scores [S&K 6th Ed., Chap 29]. The mode of a set of numbers is that value which occurs with the greatest frequency: that is, it is the most common value. The mode may not exist, and even if it does exist it may not be unique [Schaum’s Statistics Crash Course, Murray R. Spiegel]. The mode is a value that occurs with the greatest frequency in a population or a sample. It could be considered as the single value most typical of all the values [Statistics.com 2004‐2010]. Range The range is defined as the difference between the lowest and highest score in a distribution of scores. The range is not considered a stable measurement of variability because the value can change greatly with the change in a single score within the distribution – either the high or low score [S&K 6th Ed., Chap 29]. The difference between the largest and the smallest values of the data set. The range only uses the two extreme values and ignores the rest of the data set [S&K 6th Ed., Chap 29]. The range for a data set is equal to the maximum value in the data set minus the minimum value in the data set. It is clear that the range is reflective of the spread in the data set since the difference between the largest and the smallest values is directly related to the spread in the data [Schaum’s Beginning Statistics 2nd Edition, Larry J. Stephens, Ph.D.]. Quartile Deviation The quartile deviation is more stable than the range because it is based on the spread of the scores through the center of the distribution rather than through the two extremes. Since the quartile deviation is an index that reflects the spread of scores throughout the middle part of the distribution, it should be sued whenever extreme scores may distort the data. Thus, the median and the quartile deviation are both insensitive to extreme scores in the distribution and Copyright 2011‐2018, AACE International, Inc. AACE International CCT Primer should be used accordingly. The major disadvantage of the quartile deviation is that it does not take into account the value of each of the raw scores in the distribution [S&K 6th Ed., Chap 29]. Standard Deviation Is a more reliable indicator of the spread of a distribution. It determines the amount each score deviates from the mean of the distribution [S&K 5th Ed., 30.6]. The positive square root of the variance [S&K 6th Ed., Chap 29]. The standard deviation is a measure of dispersion. It is the positive square root of the variance. An advantage of the standard deviation (as compared to the variance) is that it expresses dispersion in the same units as the original values in the sample or population. For example, the standard deviation of a series of measurements of temperature is measured in degrees; the variance of the same set of values is measured in “degrees squared” [Statistics.com 2002‐2010]. Variance The average of the squared deviations from the mean. The variance has a squared unit and is in a much larger scale than that of the original data [S&K65th Ed., Chap 29]. Variance is a measure of dispersion. It is the average squared distance between the mean and each item in the population or in the sample. An advantage of variance (as compared to the related measure of dispersion – the standard deviation) is that the variance of a sum of independent random variables is equal to the sum of their variances [Statistics.com 2004‐2010]. Normal Distribution: [Review S&K 6th Edition, Chapter 29, for more detailed discussion on this topic]. Normal Distribution One of the most important examples of a continuous probability distribution is the normal distribution. Normal curve or Gaussian distribution [Schaum’s Statistics Crash Course, Murray R. Spiegel]. The normal distribution is a probability density which is bell‐shaped, symmetrical, and single peaked. The mean, median and mode coincide and lie at the center of the distribution. The two tails extend indefinitely and never touch the X‐ axis (asymptotic to the X‐axis). A normal distribution is fully specified by two parameters – mean and the standard deviation [Statistics.com 2004‐2010]. The most important continuous distribution in statistical decision making is the normal distribution. A graph of a normal distribution is called a normal curve and it has the following characteristics” a. It is bell‐shaped and is symmetrical about the mean. The mean, median and mode are all equal. Probability density decreases symmetrically as X values move from the mean in either direction. b. The curve approaches but never touches the horizontal axis. However, when the value of X is more than three standard deviations from the Copyright 2011‐2018, AACE International, Inc. AACE International CCT Primer mean, the curve approaches the axis so closely that the extended area under the curve is negligible [S&K 6th Ed., Chap 29]. Normal Probability Distribution The most important and widely used of all continuous distributions is the normal probability distribution. The larger the standard deviation, the more disperse are the values about the mean [Schaum’s Beginning Statistics 2nd Edition, Larry J. Stephens, Ph.D.]. Standard Normal Distribution The standard normal distribution is the normal distribution having mean equal to 0 and standard deviation equal to 1 [Schaum’s Beginning Statistics 2nd Edition, Larry J. Stephens, Ph.D.]. 2. Non‐Normal Distributions: be able to describe the following concepts: a. Skewness A symmetric histogram in which each class has the same frequency is called a uniform or rectangular histogram. A skewed to the right histogram has a longer tail on the right side. A skewed to the left histogram has a longer tail on the left side [Schaum’s Beginning Statistics 2nd Edition, Larry J. Stephens, Ph.D.]. The reason for constructing histograms and frequency polygons is to reveal how scores are distributed along the score scale. That is, the form of the distribution is shown. A distribution is symmetrical if one side is a mirror image of the other. If not, it is asymmetrical. Asymmetrical curves can be skewed either positively or negatively. For negative skewness, the tail travels to the left, for positive skewness, the tail travels to the right. Skewness measures the lack of symmetry of a probability distribution. A curve is said to be skewed to the right (or positively skewed) if it tails off toward the high end of the scale (right tail longer than the left). A curve is skewed to the left (or negatively skewed) if it tails off toward the low end of the scale. Skewness of the distribution whose density is symmetrical around the mean is zero. The reverse is not true – there are asymmetrical distributions with zero skewness. Skewness characterizes the shape of a distribution – that is, its value does not depend on an arbitrary change of the scale and location of the distribution. For example, skewness of a sample (or population) of temperature values in Fahrenheit will not change if you transform the values to Celsius (the mean and the variance will, however, change) [Statistics.com 2004‐2010]. b. Kurtosis Kutosis measures the “heaviness of the tails” of a distribution (in compared to a normal distribution). Kutosis is positive if the tails are “heavier” than for a normal distribution and negative if the tails are “lighter” than for a normal distribution. The normal distribution has kurtosis of zero [Statistics.com 2004‐2010]. 3. Histograms, Cumulative Frequency: Copyright 2011‐2018, AACE International, Inc. AACE International CCT Primer Histograms A histogram is a graph that displays the classes on the horizontal axis and the frequencies of the classes on the vertical axis. The frequency of each class is represented by a vertical bar whose height is equal to the frequency of the class. A histogram is similar to a bar graph. However, a histogram uses classes or intervals and frequencies while a bar graph uses categories and frequencies [Schaum’s Beginning Statistics 2nd Edition, Larry J. Stephens, Ph.D.]. In a histogram, the frequency of each score or class is represented as a vertical bar. When developing the histogram, the ¾ rule should be applied. That is, the highest frequency should be laid out so that the height is approximately ¾ the length of the horizontal axis. Otherwise, the viewer may obtain the wrong impression based on graph appearance rather than on graph data. The bar width should be the same as the “real limit” of a class. In addition, the graph should be titled in a descriptive fashion to indicate what the graph is showing [S&K 6th Ed., Chap 21 & 29]. Histograms and frequency polygons are two graphic representations of frequency distributions. A histogram or frequency histogram, consists of a set of rectangles having (a) bases on a horizontal axis (the X axis), with centers at the class marks and lengths equal to the class interval sizes, and (b) areas proportional to the class frequencies [Schaum’s Statistics Crash Course, Murray R. Spiegel]. 3. Inferential Statistics 1. Probability: Probability is a measure of the likelihood of the occurrence of some event [Schaum’s Beginning Statistics 2nd Edition, Larry J. Stephens, Ph.D.]. Hint: Given a curve of normal distribution and an accompanying table of areas under the curve, be able to determine the probability of: a) The variable being between two given numbers. b) Not being higher than a given number, or lower than that number. c) Given a confidence interval or range in terms of percentage probability, give the corresponding low and high number of the interval or range. 2. Regression Analysis: Regression analysis provides a “best‐fit” mathematical equation for the relationship between the dependent variable (response) and independent variable(s). There are two major classes of regression – parametric and non‐parametric. Parametric regression requires choice of the regression equation with one or a greater number of unknown parameters. Linear regression, in which a linear relationship between the dependent variable and independent variables is posited, is an example. The aim of parametric regression is to find the values of these parameters which provide the best fit to the data. The number of parameters is usually much smaller than the number of data points [Statistics.com 2004‐2010]. 3. Statistical Significance: Copyright 2011‐2018, AACE International, Inc. AACE International CCT Primer a. Chi‐squared The chi‐square test can be used to determine how well theoretical distributions (such as the normal and binomial distributions) fit empirical distributions (i.e., those obtained from sample data) [Schaum’s Statistic Crash Course, Murray R. Spiegel]. Chi‐squared test is a statistical test for testing the null hypothesis that the distribution of a discrete random variable coincides with a given distribution. It is one of the most popular goodness‐of‐fit tests. For small samples, the classical chi‐squared test is not very accurate – because the sampling distribution of the statistic of the test differs from the chi‐square distribution. In such cases, Monte Carlo simulation is a more reasonable approach [Statistics.com 2004‐2010]. b. T‐tests A t‐test is a statistical hypothesis test based on a test statistic whose sampling distribution is a t‐distribution. Various t‐tests, strictly speaking, are aimed at testing hypotheses about populations with normal probability distribution. However, statistical research has shown that t‐test often provide quite adequate results for non‐normally distributed populations too. The term “t‐test” is often used in a narrower sense – it refers to a popular test aimed at testing the hypothesis that the population mean is equal to some value [Statistics.com 2004‐2010]. c. T‐statistic T‐statistic is a statistic whose sampling distribution is a t‐distribution [Statistics.com 2004‐2010]. Recommendation: Reference books, Schaum’s Beginning Statistics 2nd Edition, Larry J. Stephens, Ph.D.; Schaum’s Statistics Crash Course, Murray R. Spiegel. These books provide graphs, charts and sample problems for basis statistics. b. Economic and Financial Analysis 1. Economic Cost: Opportunity Cost (of capital) The rate of return available on the next best available investment of comparable risk [RP 10S‐90]. 2. Cash Flow Analysis: Cash Flow Inflow and outflow of funds within a project. A time‐based record of income and expenditures, often presented graphically [RP10S‐90]. Hint: be able to calculate simple and compound interest rates and solve interest problems using the basic single payments, uniform series, and gradient formulas. Hint: given a set of cost and revenue forecasts calculate a cash flow for an asset investment option 3. Internal Rate of Return: The compound rate of interest that when used to discount study period costs and benefits of a project, will make their time‐ values equal [RP10S‐90]. 4. Present/Future Value Analysis: Present Value Copyright 2011‐2018, AACE International, Inc. AACE International CCT Primer The value of a benefit or cost found by discounting future cash flows to the base time. Also, the system of compounding proposed investments, which involves discounting at a known interest rate (representing a cost of capital or a minimum acceptable rate of return) in order to choose the alternative having the highest present value per unit of investment. This technique eliminates the occasional difficulty with profitability index of multiple solutions, but has the troublesome problem of choosing or calculating a “cost of capital” or minimum rate of return [RP10S‐90]. Future Value The value of a benefit or a cost at some point in the future, considering the time value of money [RP10S‐90]. c. Optimization 1. Model: An optimization model is used to find the best possible choice out of a set of alternatives. It may use the mathematical expression of a problem to maximize or minimize some function. The alternatives are frequently restricted by constraints on the values of the variables [Answers.com]. 2. Linear Programming: Mathematical techniques for solving a general class of optimization problems through minimization (or maximization) of a linear function subject to linear constraints. For example, in blending aviation fuel, many grades of commercial gasoline may be available. Prices and octane ratings, as well as upper limits on capacities of input materials which can be used to produce various grades of fuel are given. The problem is to blend the various commercial gasolines in such a way that: 1) Cost will be minimized (profit will be maximized; 2) A specified optimum octane rating will be met; and 3) The need for additional storage capacity will be avoided [RP 10S‐90]. 3. Simulation: Application of a physical or mathematical model to observe and predict probable performance of the actual item or phenomenon to which it relates [RP10S‐90]. Modeling – Creation of a physical representation or mathematical description of an object, system or problem that reflects the function or characteristics of the item involved. Model building may be viewed as both a science and an art. Cost estimate and CPM schedule development should be considered modeling practices and not exact representations of future costs, progress and outcomes [RP10S‐90]. Monte Carlo Method – A simulation technique by which approximate evaluations are obtained in the solution of mathematical expressions so as to determine the range or optimum value. The technique consists of simulating an experiment or model to determine some probabilistic property of a model, system or population of objects or events by use of random sampling to the components of the model, system, objects, or events. The method can be applied to cost estimates and schedules when they are expressed as a model [RP10S‐90]. 4. Sensitivity Analysis: A test of the outcome of an analysis by altering one or more parameters from an initially assumed value [RP10S‐90]. Copyright 2011‐2018, AACE International, Inc. AACE International CCT Primer d. Physical Measurements: Hint: Be able to convert basic metric and imperial weight and dimensional measurements. Be able to convert temperature from Fahrenheit degrees to Celsius. Be able to convert square feet to square yards, square feet to cubic yards. Be able to convert square feet into square inches. Be able to convert gallons to liters. [See Appendix B, SI Units, S&K 5th Ed., for conversion exercises]. Copyright 2011‐2018, AACE International, Inc. AACE International CCT Primer