Chapter 5 (Part 1) - Statistical Inference PDF
Document Details
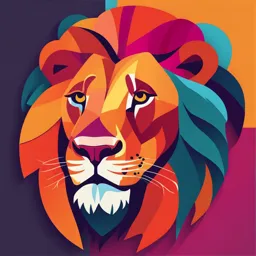
Uploaded by UnrivaledUnderstanding
Universiti Teknologi MARA, Johor
Nur Liyana Mohamed Yousop
Tags
Summary
This document presents a chapter on statistical inference, focusing on hypothesis testing. It covers the concepts and procedures of hypothesis testing, including different types of tests and provides examples.
Full Transcript
CHAPTER 5 (PART I) 2 S Statistical Inference Prepared by: Nur Liyana Mohamed Yousop STATISTICAL INFERENCE Statistical inference focuses on drawing conclusions about populations from samples. Statistical inference includes: • Estimation of population parameters and; • Hypothesis testing Which in...
CHAPTER 5 (PART I) 2 S Statistical Inference Prepared by: Nur Liyana Mohamed Yousop STATISTICAL INFERENCE Statistical inference focuses on drawing conclusions about populations from samples. Statistical inference includes: • Estimation of population parameters and; • Hypothesis testing Which involves drawing conclusions about the value of the parameters of one or more populations. HYPOTHESIS Hypothesis is a statement about population subject to verification. Where we: Make a claim (State in hypothesis form) Collect data Use data to test the claim (hypothesis). HYPOTHESIS TESTING Hypothesis Testing is a method to determine whether the hypothesis concerning the population parameter is a reasonable statement based on sample data. Hypothesis testing involves drawing inferences about two contrasting propositions (each called a hypothesis) relating to the value of one or more population parameters. TYPES OF HYPOTHESIS TESTING Hypothesis Testing One-Sample Hypothesis Tests • Mean • Proportion The two complement hypothesis statements: Null Hypothesis (H0) Two-Samples Hypothesis Test • Mean Two-Samples Test (Paired Samples) • Mean Alternative Hypothesis (H1) EXAMPLES Hypothesis Testing • One-Sample Hypothesis Tests • Average CGPA for group A is higher and equal to 3.00 • Hypothesis: µ ≥ 3.00 • Two-Samples Hypothesis Test • Average CGPA for group A is higher and equal to the average CGPA for group B • µA≥µB or µA - µB = 0 • Two-Samples Test (Paired Samples) • Pre and post test / crisis in Malaysia • Entrance and exit survey in UiTM • Slimming product (before and after consuming) • Measure difference (µd) HYPOTHESIS TESTING PROCEDURE Steps in conducting a hypothesis test: 1 2 3 4 5 • Formulate the hypotheses to test by stating H0 and H1. • Select a level of significance (the risk of drawing an incorrect conclusion). • Identify the test statistics • Determine the decision rule • Apply the decision rule and draw a conclusion. STEP 1 Formulate the Hypothesis Test by Stating H 0 and H 1 Three types of one-sample tests: • H0: parameter ≤ constant • H1: parameter > constant One tailed test → Upper-tailed • H0: parameter ≥ constant • H1: parameter < constant One tailed test → Lower-tailed • H0: parameter = constant • H1: parameter ≠ constant Two-tailed It is not correct to formulate a null hypothesis using >, <, or ≠. DETERMINING THE PROPER FORM OF HYPOTHESES Hypothesis testing always assumes that H0 is true and uses sample data to determine whether H1 is more likely to be true. • Statistically, we cannot “prove” that H0 is true; we can only fail to reject it. Rejecting the null hypothesis provides strong evidence (in a statistical sense) that the null hypothesis is not true and that the alternative hypothesis is true. Therefore, what we wish to provide evidence for statistically should be identified as the alternative hypothesis. HYPOTHESIS TESTING Using sample data, we either: Reject H0 and conclude the sample data provides Sufficient evidence to support H1. OR ; Fail to reject H0 and conclude the sample data Does not support H1. EXAMPLE 1 Analogy for Hypothesis Testing In the U.S. legal system, a defendant is innocent until proven guilty. • H0: Innocent • H1: Guilty If evidence (sample data) strongly indicates the defendant is guilty, then we reject H0. Note that we have not proven guilt or innocence! DEVELOPING NULL AND ALTERNATIVE HYPOTHESES Example A new teaching method is developed that is believed to be better than the current method H1 - got changes The new teaching method is better. H1 Ho - no change H0 The new method is no better than the old method. New machine of sliding pineapple will produce more cans of the pineapple product. Ho: The new machine does not increase the number of cans of the pineapple product H1: The new machine does increase the number of cans of the pineapple product DEVELOPING NULL AND ALTERNATIVE HYPOTHESES Example The new bonus plan increase sales. A new sales force bonus plan is developed in an attempt to increase sales. H1 H0 The new bonus plan does not increase sales. STEP 2 Select a Significance Level After setting up the null hypothesis and alternate hypothesis, the next step is to state the level of significance. Level of significance(α) can be defined as the probability of rejecting the null hypothesis when it is true. Level of sig → 1%, 5%, 10% (normally 5%) STEP 2 Select a Significance Level Understanding Potential Errors in Hypothesis Testing Hypothesis testing can result in one of four different outcomes: H0 is true and the test correctly fails to reject H0 H0 is false and the test correctly rejects H0 H0 is true and the test incorrectly rejects H0 (called Type I error) H0 is false and the test incorrectly fails to reject H0 (called Type II error) STEP 2 Select a Significance Level No hypothesis test is 100% certain. Because the test is based on probabilities, there is always a chance of making an incorrect conclusion. Truth about the population Decision based on sample H0 is true H0 is false Reject H0 Type I Error Rejecting H0 when it is true (probability = α) Correct Decision (probability = 1 - β) Fail to reject H0 Correct Decision (probability = 1 - α) Type II Error Fail to reject H0 when it is false (probability = β) STEP 2 Select a Significance Level When the null hypothesis is true and you reject it, you make a type I error. The probability of making a type I error is α, which is the level of significance you set for your hypothesis test. TYPE I ERROR An α of 0.05 indicates that you are willing to accept a 5% chance that you are wrong when you reject the null hypothesis. To lower this risk, you must use a lower value for α. However, using a lower value for alpha means that you will be less likely to detect a true difference if one really exists. STEP 2 Select a Significance Level When the null hypothesis is false and you fail to reject it, you make a type II error. The probability of making a type II error is β, which depends on the power of the test. TYPE II ERROR You can decrease your risk of committing a type II error by ensuring your test has enough power. You can do this by ensuring your sample size is large enough to detect a practical difference when one truly exists. The probability of rejecting the null hypothesis when it is false is equal to 1–β. This value is the power of the test. EXAMPLE 2 Example of Error The director of the ABC hospital wants to formulate a hypothesis test that could use a sample of emergency response times to determine whether or not the service goal of 15 minutes or less is being achieved. H0 : µ ≤ 15 H1 : µ > 15 The emergency service is meeting the response goal; no follow-up action is necessary. The emergency service is not meeting the response goal; appropriate followup action is necessary. where: µ= mean response time for the population of medical emergency requests EXAMPLE 2 Example of Type I and Type II Error Population Conditions Conclusion H0 True H0 : µ ≤ 15 H0 False H0 : µ > 15 H0 : µ ≤ 15 Correct Decision Type II Error H1 : µ > 15 Type I Error Correct Decision STEP 3 Selecting the Test Statistic The decision to reject or fail to reject a null hypothesis is based on computing a test statistic from the sample data. The test statistic used depends on the type of hypothesis test. Test statistics for one-sample hypothesis tests for means: 2 2 Z-DISTRIBUTION REMEMBER CHAPTER 4 ???? STEP 3 z-Statistics σ2 is known Case I n<30 Case II Population is normal n≥30 Case III Population is normal Use the normal distribution (z) to test the hypothesis about µ n<30, n≥30 Population is not normal Use a nonparametric method to test the hypothesis about µ STEP 3 t-Statistics σ2 is unknown Case I n<30 Case II Population is normal n≥30 Case III Population is normal Use the t-distribution (t) to test the hypothesis about µ n<30, n≥30 Population is not normal Use a nonparametric method to test the hypothesis about µ T-DISTRIBUTION The t-Distribution, also known as Student’s t-Distribution is the probability distribution that estimates the population parameters when the sample size is small and the population standard deviation is unknown. T-distribution is flatter than normal distribution but as a degree of freedom increases or n increases, it approaches the standard normal distribution. STEP 4 Determine the Decision Rule The decision rule states the conditions under which the null hypothesis will be failed to rejected or be rejected. It depends on whether the test statistics falls under the rejection area or non-rejection area. STEP 4 Determine the Decision Rule The critical value approach Two procedures to make a hypothesis test: The p-value approach D TS>CV Reject Ho STEP 4 TS<CV Fail to reject Ho CRITICAL VALUE APPROACH Determine the Decision Rule Critical value is the dividing point between the region where the H0 is rejected and the region where it is not rejected That is, it entails comparing the observed test statistic to some cut-off value, called the "critical value." If the test statistic is more extreme than the critical value, then the null hypothesis is rejected in favour of the alternative hypothesis. If the test statistic is not as extreme as the critical value, then the null hypothesis is failed to be rejected. FINDING CRITICAL VALUES (EXCEL) For a lower one-tailed test, the critical value is the probability to the left of the test statistic t in the t-distribution, and is found using the Excel function: • =T.INV(α, n-1) For an upper one-tailed test, the critical value is the probability to the right of the test statistic t, and is found using the Excel function: • T.INV(1-α, n-1) For a two-tailed test, the critical value is found using the Excel function: • T.INV(α/2, n-1) STEP 4 P-VALUE APPROACH Determine the Decision Rule A p-value (observed significance level) is the probability of obtaining a test statistic value equal to or more extreme than that obtained from the sample data when the null hypothesis is true. An alternative approach to Step 3 of a hypothesis test uses the p-value rather than the critical value: • Reject H0 if the p-value < α * p-value < a Reject Ho p-value > a Fail to reject Ho FINDING P-VALUES (EXCEL) For a lower one-tailed test, the p-value is the probability to the left of the test statistic t in the t-distribution, and is found using the Excel function: • =T.DIST(t, n-1, TRUE) For an upper one-tailed test, the p-value is the probability to the right of the test statistic t, and is found using the Excel function: • 1 - T.DIST(t, n-1, TRUE) For a two-tailed test, the p-value is found using the Excel function: • T.DIST.2T(t, n-1), if t > 0 • T.DIST.2T(-t, n-1), if t < 0 STEP 5 Drawing a Conclusion The conclusion to reject or fail to reject H0 is based on comparing the value of the test statistic to a “critical value” from the sampling distribution (zdistribution or t-distribution) of the test statistic when the null hypothesis is true and the chosen level of significance, a. The critical value divides the sampling distribution into two parts: Rejection region (reject the null hypothesis) Non-rejection region (failed to reject null hypothesis). REJECTION REGIONS H0: parameter ≥ constant H1: parameter < constant H0: parameter ≤ constant H1: parameter > constant H0: parameter = constant H1: parameter ≠ constant For a one-tailed test, if H1 is stated as <, the rejection region is in the lower tail; if H1 is stated as >, the rejection region is in the upper tail (just think of the inequality as an arrow pointing to the proper tail direction). DEGREE OF FREEDOM Simple Explanation The Freedom to Vary Imagine you’re a fun-loving person who loves to wear hats. You couldn't care less what a degree of freedom is. You believe that variety is the spice of life. Unfortunately, you have constraints. You have only 7 hats. Yet you want to wear a different hat every day of the week. On the 1st day, you can wear any of the 7 hats. On the 2nd day, you can choose from the 6 remaining hats, on day 3 you can choose from 5 hats, and so on. DEGREE OF FREEDOM Simple Explanation When day 6 rolls around, you still have a choice between 2 hats that you haven’t worn yet that week. But after you choose your hat for day 6, you have no choice for the hat that you wear on Day 7. You must wear the one remaining hat. You had 7-1 = 6 days of “hat” freedom—in which the hat you wore could vary! That’s kind of the idea behind degrees of freedom in statistics. Degrees of freedom are often broadly defined as the number of "observations" (pieces of information) in the data that are free to vary when estimating statistical parameters. ONE-SAMPLE HYPOTHESIS TESTS (MEAN | ONE-TAILED) STEP 1: Formulating a One-sample Test of Hypothesis by Stating H 0 and H 1 Data: CadSoft CadSoft receives calls for technical support. In the past, the average response time has been at least 25 minutes. It believes the average response time can be reduced to less than 25 minutes. If the new information system makes a difference, then, data should be able to confirm that the mean response time is less than 25 minutes; this defines the alternative hypothesis, H1. • H0: mean response time ≥ 25 • H1: mean response time < 25 STEP 2: Select a Significance Level After setting up the null hypothesis and alternate hypothesis, the next step is to state the level of significance. For this example, we will choose 5% level of significance STEP 3: Select and Compute the Test Statistic In the CadSoft example, sample data for 44 customers revealed a mean response time of 21.91 minutes and a sample standard deviation of 19.49 minutes. t = -1.05 indicates that the sample mean of 21.91 is 1.05 standard errors below the hypothesized mean of 25 minutes. STEP 4 & 5: Determine the Decision Rule and Drawing the Conclusion CRITICAL VALUES APPROACH H0: mean response time ≥ 25 H1: mean response time < 25 ➢ n = 44; df = n −1 = 43 ➢ t-statistic= -1.05, α=0.05 Critical value = T.INV(α , n −1) = T.INV(0.05, 43) = -1.68 T-statistic = -1.05 does not fall in the rejection region. Result: Fail to reject H0. Even though the sample mean of 21.91 is well below 25, we have too much sampling error to conclude the that the true population mean is less than 25 minutes. STEP 4 & 5: Determine the Decision Rule and Drawing the Conclusion P-VALUES APPROACH The p-value is the left tail area of the observed test statistic, t = -1.05. p-value =TDIST(-1.05, 43, true) = 0.1498 Failed to reject H0 because the p-value ≥ α, i.e., 0.1498 ≥ 0.05 STEP 4 & 5: Determine the Decision Rule and Drawing the Conclusion For Z-Test Excel Function (Critical Value Approach and P-Value Approach) please refer to your textbook ONE-SAMPLE HYPOTHESIS TESTS (MEAN | TWO-TAILED) STEP 1: Conducting a Two-tailed Hypothesis Test for The Mean Excel file Vacation Survey One of the tourism staff claims that average age of respondents going for vacation is different from 35 years old. H0: mean age = 35 H1: mean age ≠ 35 n = 34; sample mean = 38.677; sample standard deviation = 7.858. STEP 2: Select a Significance Level In this case, the sample mean is 2.73 standard errors above the hypothesized mean of 35. However, because this is a two-tailed test, the rejection region and decision rule are different. For a level of significance α, we choose 5%. For a level of significance α, we reject H0 if the t-test statistic falls either below the negative critical value, − tα/2,n-1, or above the positive critical value, tα/2,n-1. STEP 3: Select and Compute Test Statistics In the Excel function T.INV.2T(0.025,33) or based on table t0.025,33 (α=0.05/2, because of two-tailed), we obtain 2.3483. Thus, the critical values are ±2.3483. Because the t-test statistic does not fall be- tween these values, we must reject the null hypothesis that the average age is 35 STEP 4 & 5: Determine the Decision Rule and Drawing the Conclusion Test statistic: o Critical value = T.INV.2T(0.025, 33) = 2.3483 o p-value = T.DIST.2T(2.69, 33) = 0.0111 < α/2 = 0.025 o Reject H0. ONE-SAMPLE HYPOTHESIS TESTS (PROPORTION) EXAMPLE 3 One-sample Test for the Proportion Excel file CadSoft CadSoft sampled 44 customers and asked them to rate the overall quality of a software package. Sample data revealed that 35 respondents (a proportion of 35/44 = 0.795 @ 79.5%) thought the software was very good or excellent. In the past, this proportion has averaged about 75%. Is there sufficient evidence to conclude that this satisfaction measure has significantly exceeded 75% using a significance level of 0.05? ONE-SAMPLE TESTS FOR PROPORTIONS STEP 1 – STEP 5 → Same as before Test statistic: p0 is the hypothesized value and 𝑝Ƹ is the sample proportion EXAMPLE 3 One-sample Test for the Proportion (Answer) EXAMPLE 3 One-sample Test for the Proportion (Answer) TWO-SAMPLES HYPOTHESIS TESTS TWO-SAMPLE SITUATIONS We may need to compare 2 treatments or 2 conditions. It is important to determine if the 2 samples are independent or not. Independent samples: • The individuals in both samples are chosen separately (“independently”) ! Paired (Dependent) samples: • The individuals in both samples are related (for example, the same subjects assessed twice, or siblings) TWO-SAMPLE SITUATIONS Examples of (Paired) Dependent Samples Pre and post lab test Before and after economic crisis Before and after consuming slimming product Entrance and exit survey on course TWO-SAMPLE HYPOTHESIS EXAMPLES Examples of Independent Samples Average score for A class is difference with B class • H0: µA = µB • H1: µA ≠µB OR; • H0: µA - µB = 0 • H1: µA-µB ≠ 0 Average score for A class is more than B class • H0: µA ≤ µB • H1: µA > µB OR; • H0: µA - µB ≤ 0 • H1: µA-µB > 0 Average score for A class is less than B class • H0: µA ≥ µB • H1: µA <µB OR; • H0: µA - µB ≥ 0 • H1: µA-µB < 0 TWO-SAMPLE SITUATIONS Independent samples • σ2 known • Z-Test • σ2 unknown (equal variance) • Choose equal variance if you have equal numbers of data points or numbers are nearly the same or conduct F-test • T-test • σ2 unknown (unequal variance) • Choose unequal variance if you have unequal numbers of data points or numbers are not the same or conduct F-test • T-test Paired (Dependent) samples • T-test EXCEL ANALYSIS TOOLPAK Procedures for Two-sample Hypothesis Tests These tools calculate the test statistic, the p-value for both a one-tail and twotail test, and the critical values for one-tail and two-tail tests. INTERPRETING EXCEL OUTPUT Rule 1 • If the test statistic is negative, the one-tailed p-value is the correct p-value for a lower-tail test; however, for an upper-tail test, you must subtract this number from 1.0 to get the correct p-value. Rule 2 • If the test statistic is nonnegative (positive or zero), then the p-value in the output is the correct p-value for an upper-tail test; but for a lower-tail test, you must subtract this number from 1.0 to get the correct p-value. Rule 3 • For a lower-tail test, you must change the sign of the onetailed critical value. TWO-SAMPLE HYPOTHESIS TESTS (Independent Samples) TWO-SAMPLE HYPOTHESIS TESTS LOWER-TAILED TEST H0: population parameter (1) - population parameter (2) ≥ D0 H1: population parameter (1) - population parameter (2) < D0 This test seeks evidence that the difference between population parameter (1) and population parameter (2) is less than some value, D0. When D0 = 0, the test simply seeks to conclude whether population parameter (1) is smaller than population parameter (2). In most applications D0 = 0, and we are simply seeking to compare the population parameters. However, there are situations when we might want to determine if the parameters differ by some non-zero amount; for example, “job classification A makes at least $5,000 more than job classification B.” TWO-SAMPLE HYPOTHESIS TESTS UPPER-TAILED TEST H0: population parameter (1) - population parameter (2) ≤ D0 H1: population parameter (1) - population parameter (2) > D0 This test seeks evidence that the difference between population parameter (1) and population parameter (2) is greater than some value, D0. When D0 = 0, the test simply seeks to conclude whether population parameter (1) is larger than population parameter (2). TWO-SAMPLE HYPOTHESIS TESTS TWO-TAILED TEST H0: population parameter (1) - population parameter (2) = D0 H1: population parameter (1) - population parameter (2) ≠ D0 This test seeks evidence that the difference between the population parameters is equal to D0. When D0 = 0, we are seeking evidence that population parameter (1) differs from population parameter (2). In most applications, D0 = 0, and we are simply seeking to compare the population parameters. EXAMPLE 4 Comparing Supplier Performance Excel file Purchase Order Determine if the mean lead time for Alum Sheeting (µ1) is greater than the mean lead time for Durrable Products (µ2). EXAMPLE 4 Testing the Hypotheses for Supplier Lead-time Performance Excel file Purchase Order t-Test: Two-Sample Assuming Unequal Variances Variable 1 Range: Alum Sheeting data Variable 2 Range: Durrable Products data EXAMPLE 4 Testing the Hypotheses for Supplier Lead-time Performance EXAMPLE 4 Testing the Hypotheses for Supplier Lead-time Performance TWO-SAMPLE HYPOTHESIS TESTS (Paired Sample) TWO-SAMPLE TEST FOR MEANS WITH PAIRED SAMPLES In many situations, data from two samples are naturally paired or matched. When paired samples are used, a paired t-test is more accurate than assuming that the data come from independent populations. Hypotheses (mD is the mean difference between the paired samples): Excel Data Analysis tool: t-Test: Paired Two-Sample for Means EXAMPLE 5 Using the Paired Two-sample Test for Means Excel file Pile Foundation Test for a difference in the means of the estimated and actual pile lengths (two-tailed test). EXAMPLE 5 Using the Paired Two-sample Test for Means EXAMPLE 5 Using the Paired Two-sample Test for Means F-DISTRIBUTION TEST FOR EQUALITY OF VARIANCES Test for equality of variances between two samples using a new type of test, the F-test. To use this test, we must assume that both samples are drawn from normal populations. Hypotheses: F-test statistic: Excel tool: F-test for Equality of Variances F-DISTRIBUTION The F-distribution has two degrees of freedom, one associated with the numerator of the F-statistic, n1 - 1, and one associated with the denominator of the F-statistic, n2 - 1. Appendix A provides only upper-tail critical values, and the distribution is not symmetric. Characteristics of F-distribution F-DISTRIBUTION F-distribution is skewed to the right Values of F always greater than 0 The shape of F-distribution is determined by: • Degree of freedom numerator • Degree of freedom denomenator CONDUCTING THE F-TEST Although the hypothesis test is really a two-tailed test, we will simplify it as an upper-tailed, one-tailed test to make it easy to use tables of the F-distribution and interpret the results of the Excel tool. • We do this by ensuring that when we compute F, we take the ratio of the larger sample variance to the smaller sample variance. Find the critical value Fa/2,df1,df2 of the F-distribution, and then we reject the null hypothesis if the F-test statistic exceeds the critical value. Note that we are using a/2 to find the critical value, not a. This is because we are using only the upper tail information on which to base our conclusion. EXAMPLE 6 Applying the F-test for Equality of Variances Determine whether the variance of lead times is the same for Alum Sheeting and Durrable Products in the Purchase Orders data. The variance of the lead times for Alum Sheeting is larger than the variance for Durable Products, so this is assigned to Variable 1. EXAMPLE 6 Applying the F-test for Equality of Variances