Statistical Inference of Two Sample PDF
Document Details
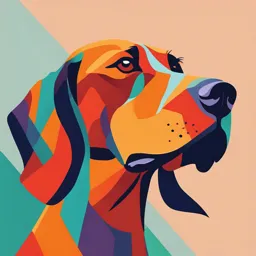
Uploaded by SelfDeterminationSaxophone
Anino, Mary Ann L. Fernandez, Amel C. Garcia, Enriquo Vicenzo P. Gloria, Marielle Osorio, Cathy P. Reyes, Joannah Maureen P. Sarausa, Jeff Oliver R. Tejoc, Lloyd O. Tuhoy, Mark Jay T.
Tags
Summary
This document outlines statistical inference for comparing two sample means, covering scenarios with known and unknown variances. It explores hypothesis testing, confidence intervals, and the choice of sample size. The document emphasizes the importance of identifying cause and effect, particularly in randomized experiments and observational studies.
Full Transcript
Statistical Inference of Two Sample UNIT 9 Anino, Mary Ann L. Fernandez, Amel C. Garcia, Enriquo Vicenzo P. Gloria, Marielle Osorio, Cathy P. Reyes, Joannah Maureen P. Sarausa, Jeff Oliver R. Tejoc,...
Statistical Inference of Two Sample UNIT 9 Anino, Mary Ann L. Fernandez, Amel C. Garcia, Enriquo Vicenzo P. Gloria, Marielle Osorio, Cathy P. Reyes, Joannah Maureen P. Sarausa, Jeff Oliver R. Tejoc, Lloyd O. Tuhoy, Mark Jay T. TOPIC OUTLINE: A. INFERENCE FOR A DIFFERENCE IN B. INFERENCE FOR A DIFFERENCE MEANS OF TWO NORMAL DISTRI- IN MEANS OF TWO NORMAL BUTIONS, VARIANCES KNOWN DISTRIBUTIONS, VARIANCES UNKNOWN Hypothesis Tests for a Difference in Means, Variances Known Hypothesis Tests for a Difference in Means, Variances Unknowm Choice of Sample Size Identifying Cause and Effect Choice of Sample Size Confidence Interval on a Difference in Confidence Interval on the Means, Variances Known Difference in Means TOPIC OUTLINE: C. INFERENCES ON THE VARIANCES D. INFERENCE ON TWO OF TWO NORMAL POPULATIONS POPULATION PROPORTIONS The F Distribution Large-Sample Test for H0: p1 p2 Development of the F Distribution (CD Only) Small-Sample Test for H0: p1 p2 (CD Only) Hypothesis Tests on the Ratio of Two Variances Error and Choice of Sample Size B - Error and Choice of Sample Size Confidence Interval for p1 p2 Confidence Interval on the Ratio of Two Variances I. INFERENCE ON THE DIFFERENCE IN MEANS OF TWO NORMAL DISTRIBUTIONS, VARIANCES KNOWN Reported By: Tejoc & Sarausa INFERENCE ON THE DIFFERENCE IN MEANS OF TWO NORMAL DISTRIBUTIONS, VARIANCES KNOWN Inference on the difference in means of two normal distributions with known variances is a common statistical procedure used to determine if there is a significant difference between the means of two populations. This can be done through hypothesis testing and confidence interval estimation. Assumptions for Two-Sample Inference In this section we consider statistical inferences on the difference in means μ1 - μ2 of two normal distributions, where the variances σ1^2 - σ2^2and are known. The assumptions for this section are summarized as follows: Hypothesis Tests on the Difference in Means, Variances Known It is used to determine if there is a statistically significant difference between the means of two populations. This method relies on the assumption that the populations are normally distributed and the variances of the populations are known. The process involves comparing the sample means from each population using a test statistic that follows a normal distribution under the null hypothesis. Example: Choice of Sample Size The choice of sample size is a critical aspect of designing a statistical study. It affects the accuracy and reliability of the results, the power of the test, and the ability to generalize findings to the broader population. Properly determining the sample size helps ensure that the study can detect a true effect if one exists while minimizing the risks of Type I and Type II errors. Sample size for two-sided test on the difference in means with n1 = n2, Variances known Sample size for one-sided test on the difference in means with n1 = n2, Variances known From the earlier Example, suppose that if the true difference in drying times is as much as 10 minutes, we want to detect this with probability at least 0.90. Under the null hypothesis, 0 0. We have a one-sided alternative hypothesis with 10, 0.05 (so z z0.05 1.645), and since the power is 0.9, 0.10 (so z z0.10 1.28). Therefore, we may find the required sample size Just Substitute! Identifying Cause and Effect Identifying cause and effect refers to the process of determining whether a specific factor (the cause) directly leads to a particular outcome (the effect). In scientific studies, this involves establishing a clear relationship where changes in the cause lead to predictable changes in the effect, demonstrating that the cause is responsible for the observed outcome. Types of Identifying Cause and Effect: Randomized Experiment Observational Study Randomized Experiment In randomized experiments, random assignment helps establish causality by controlling for other variables, making it easier to determine whether a specific factor is responsible for an observed outcome. Example the two different treatments are the two paint formulations, and the response is the drying time. The purpose of the study is to determine whether the new formulation results in a significant effect reducing drying time Observational Study In observational studies, researchers observe or compare natural groups without manipulating conditions or using random assignment. This makes it tricky to pinpoint causality due to factors like lifestyle or genetics that can influence outcomes. Example the September 1992 issue of Circulation (a medical journal published by the American Heart Association) reports a study linking high iron levels in the body with increased risk of heart attack. The study, done in Finland, tracked 1931 men for five years and showed a statistically significant effect of increasing iron levels on the incidence of heart attacks. Confidence Interval on a Difference in Means, Variances Known Example Example Example Choice of Sample Size If the standard deviations σ, and σ are known (at least approximately) and the two sample sizes n, and n, are equal (n1 = n2 = n, say), we can determine the sample size required so that the error in estimating μ1-μ2 by x1-x2 will be less than E at 100(1 - a)% confidence. Choice of Sample Size One- Sided Confidence Bounds Upper Confidence Bound One- Sided Confidence Bounds Lower Confidence Bound II. INFERENCE FOR A DIFFERENCE IN MEANS OF TWO NORMAL DISTRIBUTIONS, VARIANCES UNKNOWN Reported By: Gloria & Reyes Basic Idea We want to compare the average values (means) of two groups to see if there's a significant difference between them. The variances (how spread out the data is) of these groups are unknown. Hypotheses Tests on the Difference in Means, Variances Unknown Reported By: Gloria & Reyes Key Terms Reported By: Gloria, Marielle Concepts to Remember When testing if the means of two groups are different but their variances are unknown, we use a t-statistic. The test requires assuming that the data follows a normal distribution, but small deviations from normality don't impact the results significantly. There are two main scenarios/cases to consider. Reported By: Gloria, Marielle Case 1: Variances Unknown but Assumed Equal Reported By: Gloria, Marielle Scenario You want to compare the means of two groups (e.g., two different types of treatments or products). You don't know the exact variances, but you assume that the variability in both groups is similar. Reported By: Gloria, Marielle 1. Formulate Hypotheses: Reported By: Gloria, Marielle 2. Collect Data: Reported By: Gloria, Marielle 3. Pooled Variance: Combine the sample variances into one estimate using the formula: This gives a single estimate of the variance assuming both groups have similar variability. Reported By: Gloria, Marielle 4. Calculate the Test Statistic: Use the pooled variance to compute the t-statistic. Reported By: Gloria, Marielle 5. Determine Degrees of Freedom: Reported By: Gloria, Marielle 6. Compare with Critical Value: Reported By: Gloria, Marielle Case 2: Variances Unknown and Not Assumed Equal Reported By: Gloria, Marielle Scenario You want to compare the means of two groups, but you do not assume that the variability (variance) in the two groups is the same. Reported By: Gloria, Marielle Concepts to Remember in CASE 2 Steps 1 (Formulate Hypotheses) and 2 (Collect Data) have the same application in Case 1 Reported By: Gloria, Marielle 3. Calculate the Test Statistic: Since the variances are not assumed equal, use the formula for the t-statistic that accounts for this: Reported By: Gloria, Marielle 4. Determine Degrees of Freedom: This is known as the Welch-Satterwaite equation. Reported By: Gloria, Marielle 5. Compare with Critical Value: Reported By: Gloria, Marielle EXAMPLE : Comparing Tensile Strengths of Two Types of Steel Scenario: The UrbanEdge Group wants to compare the tensile strengths of two different types of steel, Steel A and Steel B, to determine which one has a higher mean tensile strength. The tensile strength is measured in Megapascals (MPa). Pooled t-test (assuming equal variances) 1. State the Hypothesis H0: Steel A and Steel B have no difference in the mean tensile strengths. H1: There is a difference in the mean tensile strengths of Steel A and Steel B. Pooled t-test (assuming equal variances) 2. Calculate the pooled variance Pooled t-test (assuming equal variances) 3. Calculate the test-statistic (t) Pooled t-test (assuming equal variances) 4. Determine the degrees of freedom (df) Pooled t-test (assuming equal variances) 4. Determine the degrees of freedom (df) 5. Determine the critical value Pooled t-test (assuming equal variances) 6. Decision Compare t with the critical value. Pooled t-test (assuming equal variances) 6. Decision Compare t with the critical value. Pooled t-test (assuming equal variances) 7. Conclusion There is significant evidence to suggest that the mean tensile strengths of Steel A and Steel B are different. EXAMPLE : Comparing Tensile Strengths of Two Types of Steel Scenario: The UrbanEdge Group wants to compare the tensile strengths of two different types of steel, Steel A and Steel B, to determine which one has a higher mean tensile strength. The tensile strength is measured in Megapascals (MPa). Welch’s t-test (assuming unequal variances) 1. State the Hypothesis H0: Steel A and Steel B have no difference in the mean tensile strengths. H1: There is a difference in the mean tensile strengths of Steel A and Steel B. Welch’s t-test (assuming unequal variances) 2. Calculate the test-statistic (t) Welch’s t-test (assuming unequal variances) 2. Calculate the test-statistic (t) Welch’s t-test (assuming unequal variances) 2. Calculate the test-statistic (t) Welch’s t-test (assuming unequal variances) 4. Determine the degrees of freedom (df) 4. Determine the degrees of freedom (df) 4. Determine the degrees of freedom (df) 5. Determine the critical value Welch’s t-test (assuming unequal variances) 6. Decision Compare t with the critical value. SUMMARY SUMMARY Both tests suggest that the mean tensile strengths of the two types of steel are significantly different. Choice of Sample Size Reported By: Gloria & Reyes Why is Sample Size Important? Accuracy: Too small a sample size may not capture the true effect and can lead to incorrect conclusions (false negatives). Efficiency: Too large a sample size wastes resources and time. Confidence: Adequate sample size increases confidence that the results reflect the true population characteristics. Reported By: Gloria, Marielle Key Concepts 1. Effect Size (Δ): The effect size is the magnitude of the difference you expect to find between the groups. For example, in a medical study, it might be the difference in blood pressure reduction between a new drug and a standard drug. Reported By: Gloria, Marielle Key Concepts 2. Standard Deviation (σ): A measure of the variability or spread of the data points. In the blood pressure example, it represents how much the blood pressure readings vary among participants. Reported By: Gloria, Marielle Key Concepts 3. Significance Level (α): The probability of rejecting the null hypothesis when it is actually true (a false positive). Commonly set at 0.05, meaning a 5% risk of concluding that a difference exists when there is none. Reported By: Gloria, Marielle Key Concepts 4. Power (1 - β): The probability of correctly rejecting the null hypothesis when it is false (detecting a true effect). Typically set at 0.80 (80%), meaning there's an 80% chance of detecting a true difference if it exists. Reported By: Gloria, Marielle Key Concepts 5. Effect Size (d): Reported By: Gloria, Marielle CONFIDENCE INTERVAL ON THE DIFFERENCE IN MEANS A confidence interval for the difference in means is a range of values that, with a specified level of confidence, is likely to contain the true difference between the population means. It provides a way to quantify the uncertainty in estimating the difference between two population means based on sample data. Reported By: Garcia, Enriquo Case 1: Reported By: Garcia, Enriquo Case 2: Reported By: Garcia, Enriquo Example: Reported By: Garcia, Enriquo Example: Get the pool estimate of the common standard deviation: Reported By: Garcia, Enriquo Example: Use our equation: Just substitute: Reported By: Garcia, Enriquo III. INFERENCE ON THE VARIANCES OF TWO NORMAL POPULATION Inferences on the variances of two normal populations involve comparing their variances to determine if they are significantly different from each other. III. INFERENCE ON THE VARIANCES OF TWO NORMAL POPULATION The F Distribution Development of the F Distribution (CD Only) Hypothesis Tests on the Ratio of Two Variances B - Error and Choice of Sample Size Confidence Interval on the Ratio of Two Variances The F Distribution Central to comparing the variances of two normal populations. When conducting an F-test, we aim to determine if the variances of two independent normal populations are equal. This test involves the following steps: STEP 1: Formulate Hypotheses STEP 2: Calculate the F-Statistic The F-statistic is computed as the ratio of the sample variances where and are the sample variances of the two populations, and is the larger of the two to ensure the F value is greater than or equal to 1. STEP 3: Determine the Critical Value The F-distribution has two degrees of freedom: where and are the sample sizes. Using these degrees of freedom, you find the critical value from the F-distribution table at a chosen significance level (e.g., 0.05). STEP 3: Determine the Critical Value The F-distribution has two degrees of freedom: where and are the sample sizes. Using these degrees of freedom, you find the critical value from the F-distribution table at a chosen significance level (e.g., 0.05). STEP 4: Decision Rule If the calculated F-statistic is greater than the critical value from the F-distribution table, we reject the null hypothesis, indicating that the variances are significantly different. If the F-statistic is less than or equal to the critical value, we fail to reject the null hypothesis, indicating that there is no significant difference in the variances. EXAMPLE Two different teaching methods are being compared to determine if they result in different levels of variability in student test scores. We have data from two independent random samples of students taught by these methods. GIVEN Method A: = 15 students, =25 Method B: =12 students, =10 We will conduct a hypothesis test at the 0.05 significance level to determine if the variances of the two methods are equal. SOLUTION WITH FORMULA STEP 1: Formulate Hypotheses STEP 2: Calculate Sample Variances STEP 3: Compute the F-Statistic STEP 4: Determine Degrees of Freedom STEP 5: Find the Critical Value For a two-tailed test at α=0.05, the critical values can be found using an F-distribution table or software: STEP 4: Decision Rule Compare the calculated F-statistic to the critical values: STEP 5: Conclusion The calculated F-statistic is 2.5, which lies between 0.26 and 3.87. Therefore, we fail to reject the null hypothesis. Final Conclusion There is not enough evidence to conclude that the variances of test scores between the two teaching methods are significantly different at the 0.05 significance level. Development of the F Distribution (Critical Detail Only) The F-distribution is developed based on the ratio of two independent chi-square distributions, each divided by their respective degrees of freedom. This distribution is particularly useful for making inferences about the variances of two normal populations. STEP 1: Chi-Square Distributions If are independent random samples from two normal populations with variances respectively, then the sample variances and follow chi-square distributions: STEP 2: Formation of the F-Statistic To compare these variances, we form the ratio of the two chi-square distributed variables, each normalized by its degrees of freedom: STEP 2: Formation of the F-Statistic Simplifying, we get: STEP 3: Under the Null Hypothesis Under the null hypothesis the F-statistic simplifies to: This F-statistic follows an F-distribution with and degrees of freedom. STEP 4: Critical Value and Decision Making We compare the calculated F-statistic to the critical value from the F-distribution table at a chosen significance level (e.g., 0.05). If exceeds the critical value, we reject the null hypothesis, indicating that the variances are significantly different. If does not exceed the critical value, we fail to reject the null hypothesis, indicating no significant difference in variances. EXAMPLE We have two independent samples from two normal populations and want to determine if their variances are equal. Here is the data: SOLUTION WITH FORMULA STEP 1: Formulate Hypotheses STEP 2: Calculate Sample Variances STEP 3: Compute the F-Statistic STEP 4: Determine Degrees of Freedom STEP 5: Chi-Square Distributions and F- Distribution The sample variances and follow chi-square distributions: STEP 5: Chi-Square Distributions and F- Distribution The F-statistic follows an F-distribution when we take the ratio of these chi-square variables normalized by their degrees of freedom: Under so: STEP 6: Find the Critical Value For a two-tailed test at α=0.05, the critical values from the F- distribution table are: STEP 7: Decision Rule Compare the calculated F-statistic to the critical values: STEP 8: Conclusion The calculated F-statistic is 2, which lies between 0.21 and 4.88. Therefore, we fail to reject the null hypothesis. Final Conclusion There is not enough evidence to conclude that the variances of the two populations are significantly different at the 0.05 significance level. Hypothesis Tests on the Ratio of Two Variances The F-distribution is developed based on the ratio of two independent chi-square distributions, each divided by their respective degrees of freedom. This distribution is particularly useful for making inferences about the variances of two normal populations. STEP 1: Formulate Hypotheses STEP 2: Calculate the Sample Variances Obtain two independent random samples from the populations and calculate their sample variances, STEP 3: Calculate the F-Statistic The F-statistic is computed as the ratio of the sample variances: Ensure is the larger sample variance to keep the F value greater than or equal to 1. STEP 4: Determine Degrees of Freedom The degrees of freedom for the numerator is The degrees of freedom for the denominator is Here, are the sample sizes of the two populations. STEP 5: Conclusion Based on the comparison, draw a conclusion about the equality of the population variances. TYPE II ERROR AND CHOICE OF SAMPLE Appendix Charts VIIo, VIIp, VIIq, and Vllr provide operating characteristic curves for the F-test given in Section 10-5.1 for a = 0.05 and a = 0.01, assuming that n1 = n2 = n. Charts VIIo and VIIp are used with the two-sided alternate hypothesis. They plot b against the abscissa parameter (10-30) for various nl = n2 = n. Charts VIIq and VIIr are used for the one-sided alternative hypotheses. EXAMPLE For the semiconductor wafer oxide etching problem in Example 10-13, suppose that one gas resulted in a standard deviation of oxide thickness that is half the standard deviation of oxide thickness of the other gas. If we wish to detect such a situation with probability at least 0.80, is the sample size n1 = n2 = 20 adequate ? FORMULA Note that if one standard deviation is half the other, GIVEN Standard deviation ratio (λ) between the two gases: λ = 1/2 Desired power of the test (1 - β): 0.80 Sample size for each group: n1 = n2 = 20 Level of significance (α): 0.05 SOLUTION 1. Understanding Lambda (λ) The ratio of standard deviations between the two gases is given by: λ = σ₁ / σ₂ = 1/2 This means the standard deviation of the first gas is half of the standard deviation of the second gas. 2.Calculating Beta (β) Beta (β) is the probability of making a type II error, which is failing to detect a true difference between the gases when it actually exists. From statistical tables (such as Appendix Chart VIIo): For n1 = n2 = 20 and α = 0.05, the critical value of β is found to be 0.20. This means there is a 20% chance of not detecting a true difference in standard deviations between the gases. 3. Interpreting Power of the Test The power of the test (1 - β) is the probability of correctly detecting a true difference. Power = 1 - β = 1 - 0.20 = 0.80 This indicates an 80% probability of correctly identifying a true difference in standard deviations between the gases. 4.Conclusion Based on the computed values: Beta (β) = 0.20, which aligns with the critical value from statistical tables. Power of the test = 0.80, indicating that the sample size of n1 = n2 = 20 is adequate to detect a true difference in standard deviations between the two gases with 80% probability. CONFIDENCE INTERVAL ON THE RATIO OF TWO VARIANCE If and are the sample variances of random samples of sizes n1 and n2, respectively, from two independent normal populations with unknown variances and , then a 100(1- a)% confidence interval on the ratio is CONFIDENCE INTERVAL ON THE RATIO OF TWO VARIANCE where and are the upper and lower a/2 percentage points of the F distribution with n2 – 1 numerator and n1 – 1 denominator degrees of freedom, respectively..A confidence interval on the ratio of the standard deviations can be obtained by taking square roots in Equation 10-33. EXAMPLE A company manufactures impellers for use in jet-turbine engines. One of the operations involves grinding a particular surface finish on a titanium alloy component. Two different grinding processes can be used, and both processes can produce parts at identical mean surface roughness. The manufacturing engineer would like to select the process having the least variability in surface roughness. A random sample of n1 = 11 parts from the first process results in a sample standard deviations s1 =5.1 microinches, and a random sample of n1 = 16 parts from the second process results in a sample standard deviation of s2 = 4.7 microinches. Find a 90% confidence interval on the ratio of the two standard deviations, s1 / s2. Assuming that the two processes are independent and that surface roughness is normally distributed, we can use Equation 10-33 as follows: FORMULA GIVEN n1=11 n2=16 s1=5.1 s2=4.7 SOLUTION or upon completing the implied calculations and taking square roots, Notice that we have used Equation 10-30 to find SOLUTION f0.95,15,10 = 1/f0.05,10,15 = 1/2.54= 0.39. Interpretation: Since this confidence interval includes unity, we cannot claim that the standard deviations of surface roughness for the two processes are different at the 90% level of confidence. IV. INFERENCE ON TWO POPULATION PROPORTIONS This refers to statistical methods used to conclude the difference between two population proportions based on sample data. Large sample test for Ho: p1 = p2 Suppose that two independent random samples of sizes n1 and n2 are taken from two populations, and let X1 and X2 represent the number of observations that belong to the class of interest in samples 1 and 2, respectively. Furthermore, This method assumes large sample sizes 𝑛 1 and 𝑛 2 , ensuring that the sampling distribution of the difference in proportions approximates a normal distribution for accurate hypothesis testing. Tuhoy, Mark Jay Tuhoy, Mark Jay Example Extracts of St. John’s Wort are widely used to treat depression. An article in the April 18, 2001 issue of the Journal of the American Medical Association (“Effectiveness of St. John’s Wort on Major Depression: A Randomized Controlled Trial”) compared the efficacy of a standard extract of St. John’s Wort with a placebo in 200 outpatients diagnosed with major depression. Patients were randomly assigned to two groups; one group received the St. John’s Wort, and the other received the placebo. After eight weeks, 19 of the placebo-treated patients showed improvement, whereas 27 of those treated with St. John’s Wort improved. Is there any reason to believe that St. John’s Wort is effective in treating major depression? Use 0.05 confidence level. Tuhoy, Mark Jay Using the hypothesis testing procedure; 1. Parameters of interest 2. Ho(Null hypothesis) 3. H1(Alternate hypothesis) 4. Choose level of significance 5. Test statistic 6. Computations 7. Reject Ho(Null hypothesis) Tuhoy, Mark Jay 8. Come into a conclusion Solution 1. Parameters of interest are P1:St John’s wort, P2: Placebo 2. Ho: P1 = P2 ; St John’s wort is not effective in treating major depression 3. H1: P1 ≠ P2; St John’s wort is effective in treating major depression 4. SL: 0.05 Tuhoy, Mark Jay 5. Test Statistic Tuhoy, Mark Jay 6. Computations =0.23 X1=19 P=0.23 X2=27 n1=100 n2=100 Tuhoy, Mark Jay 7. Reject Ho Since the Zo=1.35, it does not exceed the boundary of 1.96, we cannot reject the null hypothesis. Tuhoy, Mark Jay 8. Conclusion We can conclude that St John’s wort is not effective in treating major depression. Tuhoy, Mark Jay Small sample test for Ho: P1=P2 (CD only) Many problems involving comparison of proportions (P1,P2). have relatively large sample sizes, However, occasionally, a small sample size problem is encountered, in such cases Z-tests are inappropriate and an alternative procedure based on hypergeometric distribution is required. Tuhoy, Mark Jay Hypergeometric Distribution Tuhoy, Mark Jay Example Insulating cloth used in printed circuit boards is manufactured in large rolls. The manufacturer is trying to improve the process yield, that is, the number of defect-free rolls produced. A sample of 10 rolls contains exactly 4 defect-free rolls. From analysis of the defect types, process engineers suggest several changes in the process. Following implementation of these changes, another sample of 10 rolls yields 8 defect-free rolls. Do the data support the claim that the new process is better than the old one, using 0.10? Tuhoy, Mark Jay Solution Ho (Null Hypothesis) Ho: P1 ≤ P2 Tuhoy, Mark Jay Solution H1 (Alternate Hypothesis) H1: P1 > P2 Tuhoy, Mark Jay Test Statistic Tuhoy, Mark Jay Computations Tuhoy, Mark Jay Reject Ho The P-value is P=.0750 +.0095 +.0003 =.0848. Thus, at the level 0.10, the null hypothesis is rejected Tuhoy, Mark Jay Conclusion We can conclude that the engineering changes have improved the process yield. Tuhoy, Mark Jay B-Error and Choice of Sample Size The computation of the B-error for the large- sample test of Ho: p1 = p2 is more involved than in the single-sample case. The problem is that the denominator of the test statistic Zo is an estimate of the standard deviation of P1 - P2 under the assumption that p1 = p2 = p. Reporter: Amel C. Fernandez When Ho: p1 = p2 is false, the standard deviation of P1 - P2 is Reporter: Amel C. Fernandez Reporter: Amel C. Fernandez For a specified pair of values p1 and p2, we can find the sample sizes n1 = n2 = n required to give the test of size alpha that has specified type II error beta. Reporter: Amel C. Fernandez Reporter: Amel C. Fernandez Confidence Interval for p1 - p2 Reporter: Amel C. Fernandez Example 1 Consider the process of manufacturing crankshaft bearings. Suppose that a modification is made in the surface finishing process and that, subsequently, a second random sample of 85 axle shafts is obtained. The number of defective shafts in this second sample is 8. Therefore, since n1=85, p1=0.12, n2=85, and p2=8/85=0.09, we can obtain an approximate 95% confidence interval on the difference in the proportion of defective bearings produced under the two processes from Equation 10-39 as follows: Reporter: Amel C. Fernandez Reporter: Amel C. Fernandez This confidence interval includes zero, so, based on the sample data, it seems unlikely that the changes made in the surface finish process have reduced the proportion of defective crankshaft bearings being produced. Reporter: Amel C. Fernandez Thank you for listening