Cross-task Specificity of Cognitive Control Processes (PDF)
Document Details
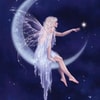
Uploaded by AppealingAmazonite
null
Yuchen Xiao, Chien-Chen Chou, Garth Rees Cosgrove, Nathan E. Crone, Scellig Stone, Joseph R. Madsen, Ian Reucroft, Yen-Cheng Shih, Daniel Weisholtz, HsiangYu Yu, William S. Anderson, Gabriel Kreiman
Tags
Summary
This research explores the neural mechanisms underlying cognitive control by comparing neurophysiological responses across different cognitive tasks. The study found that neural signatures of conflict monitoring are primarily task-specific, rather than reflecting generalizable abstract control mechanisms. Results suggest a complex interplay between sensory inputs, motor outputs, and task demands in cognitive control processes.
Full Transcript
HHS Public Access Author manuscript Author Manuscript Cell Rep. Author manuscript; available in PMC 2023 March 08. Published in final edited form as: Cell Rep. 2023 January 31; 42(1): 111919. doi:10.1016/j.celrep.2022.111919. Cross-task specificity and within-task invariance of cognitive control pro...
HHS Public Access Author manuscript Author Manuscript Cell Rep. Author manuscript; available in PMC 2023 March 08. Published in final edited form as: Cell Rep. 2023 January 31; 42(1): 111919. doi:10.1016/j.celrep.2022.111919. Cross-task specificity and within-task invariance of cognitive control processes Yuchen Xiao1, Chien-Chen Chou2,3, Garth Rees Cosgrove4, Nathan E. Crone5, Scellig Stone6, Joseph R. Madsen6, Ian Reucroft5, Yen-Cheng Shih2,3, Daniel Weisholtz4, HsiangYu Yu2,3, William S. Anderson5, Gabriel Kreiman6,7,8,* 1Harvard University, Cambridge, MA, USA Author Manuscript 2Department 3School of Neurology, Taipei Veterans General Hospital, Taipei, Taiwan of Medicine, National Yang Ming Chiao Tung University College of Medicine, Taipei, Taiwan 4Brigham 5Johns and Women’s Hospital, Harvard Medical School, Boston, MA, USA Hopkins School of Medicine, Baltimore, MD, USA 6Boston Children’s Hospital, Harvard Medical School, Boston, MA, USA 7Center for Brains, Minds and Machines, Cambridge, MA, USA 8Lead contact Author Manuscript SUMMARY Author Manuscript Cognitive control involves flexibly combining multiple sensory inputs with task-dependent goals during decision making. Several tasks involving conflicting sensory inputs and motor outputs have been proposed to examine cognitive control, including the Stroop, Flanker, and multi-source interference task. Because these tasks have been studied independently, it remains unclear whether the neural signatures of cognitive control reflect abstract control mechanisms or specific combinations of sensory and behavioral aspects of each task. To address these questions, we record invasive neurophysiological signals from 16 patients with pharmacologically intractable epilepsy and compare neural responses within and between tasks. Neural signals differ between incongruent and congruent conditions, showing strong modulation by conflicting task demands. These neural signals are mostly specific to each task, generalizing within a task but not across tasks. These results highlight the complex interplay between sensory inputs, motor outputs, and task demands underlying cognitive control processes. This is an open access article under the CC BY-NC-ND license (http://creativecommons.org/licenses/by-nc-nd/4.0/). * Correspondence: [email protected]. AUTHOR CONTRIBUTIONS The task was designed by Y.X. and G.K. All the data were collected by Y.X. with the help of C.-C.C., N.E.C., I.R., Y.-C.S., and D.W. C.R.G., S.S., J.R.M., H.-Y.Y., and W.S.A. performed the surgeries on the patients. All the data were analyzed by Y.X., with frequent discussions with G.K. The manuscript was written by Y.X. and G.K. and approved by all authors. DECLARATION OF INTERESTS The authors declare no conflicts of interest. SUPPLEMENTAL INFORMATION Supplemental information can be found online at https://doi.org/10.1016/j.celrep.2022.111919. Xiao et al. Page 2 Graphical Abstract Author Manuscript Author Manuscript Author Manuscript In brief Cognitive control involves flexibly routing information according to current goals. Xiao et al. demonstrate that the neural mechanisms underlying cognitive control generalize across different conditions within a task but are not task invariant. Instead, the neural signatures of conflict monitoring reflect task-dependent combinations of sensory inputs and motor outputs. INTRODUCTION Author Manuscript The ability to flexibly route information is central to daily activities, especially when faced with a complex and conflicting interplay of sensory information, choices, and goals. Cognitive control refers to the ability to regulate actions toward achieving overriding goals and is mentally effortful due to the necessity to suppress autonomous responses toward salient but goal-irrelevant stimulus attributes.1,2 Such costs are required for successful adaptation to various environments.3 Impairment in cognitive control is associated with a wide range of mental disorders, including addiction, depression, and schizophrenia.4-6 An essential component of cognitive control is conflict resolution, which entails mental operations involving conflict detection and monitoring,7 response selection and inhibition,8 performance monitoring and evaluation,9 and error-detection.9-11 Cell Rep. Author manuscript; available in PMC 2023 March 08. Xiao et al. Page 3 Author Manuscript Author Manuscript Many experimental tasks have been used to study cognitive control during conflict resolution. Paradigmatic examples include the Stroop task,12 the Eriksen-flanker task (referred to as "Flanker" throughout the text13), and the multi-source interference task (MSIT, referred to as "Number" throughout the text14). Common to all these tasks is the comparison between congruent and incongruent conditions (Figure 1). In the Stroop task, subjects name the font color of a color word (e.g., "red," "green," or "blue") when the semantic meaning of the word agrees (congruent condition) or disagrees (incongruent condition) with its font color. In the Flanker task, subjects have to recognize a symbol, such as a letter or an arrow, embedded among the same symbols (congruent condition) or different symbols (incongruent condition).13,15,16 The multi-source interference task14 combines multiple dimensions of cognitive interference from the Stroop, Flanker, and Simon17 tasks. The MSIT stimulus consists of three numbers (chosen from 0, 1, 2, or 3) in which one number (target) is always different from the other two numbers (distractors). Subjects are instructed to identify the target number under conditions where it is congruent (e.g., 100) or incongruent (e.g., 313) with its position. The behavioral signature of this family of tasks is the longer reaction time (RT) for incongruent stimuli (containing conflict) than congruent stimuli (conflict-free). For example, in the Stroop task, subjects take longer to name the font color of the word “RED” when shown in green or blue font than in red font. The increase in reaction time during incongruent conditions is due to interference from irrelevant but conflicting information and the selection among competing motor plans.2,8,12 Author Manuscript Multiple studies have examined brain signals associated with each one of these cognitive control tasks, including measurements derived from human neuroimaging,14,18-22 human scalp electroencephalography,21,23,24 human invasive neurophysiology,11,25-28 and monkey neurophysiology.29-32 These studies have described an extensive network of frontal and parietal regions, and to a lesser extent temporal and other regions, that demonstrate distinct activation patterns during congruent and incongruent trials. Author Manuscript Here, we evaluated whether there are shared mechanisms involved in conflict processing that are common across different sensory inputs and motor outputs. We focused on how conflict is represented in the brain by directly comparing neurophysiological responses during three cognitive control tasks, analyzing intracranial field potentials from 694 bipolarly referenced electrodes implanted in patients with pharmacologically intractable epilepsy. Our first hypothesis is that conflict-related responses should show invariance to the stimulus properties within each task (within-task invariance). For example, in the Stroop task, we would expect that neural responses would distinguish congruent (RED/ red, GREEN/green, or BLUE/blue) from incongruent (RED/green, RED/blue, GREEN/ red, GREEN/blue, BLUE/red, or BLUE/green) conditions, irrespective of the specific semantic/color combination. Extending this hypothesis of within-task invariance to the comparison among different tasks, the assumption of an abstract notion of conflict led to our second hypothesis, that neural responses would distinguish conflict irrespective of whether incongruency is dictated by color, shape, or number stimuli, and also regardless of the specific response modalities involved (cross-task invariance). Our results are consistent with the first hypothesis; neural signals that show modulation by conflict are invariant to stimulus Cell Rep. Author manuscript; available in PMC 2023 March 08. Xiao et al. Page 4 Author Manuscript attributes within a task. In contrast, our results are inconsistent with the second hypothesis; the majority of the neural conflict modulation is task specific and does not generalize across tasks. These observations are consistent with models of cognitive control2 that rely on the augmentation of task-specific processing pathways. RESULTS Author Manuscript We recorded intracranial field potentials from 16 epilepsy patients implanted with depth electrodes (Table S1). Subjects performed three cognitive control tasks: Stroop, Flanker, and Number (STAR Methods, Figure 1). Importantly, subjects performed the three tasks during the same session, therefore enabling direct comparison among the tasks. Each trial began with a fixation cross shown for 500 ms at the center of the screen. The Stroop task stimulus consisted of color words (“RED,” “GREEN,” or “BLUE,” STAR Methods) shown in red, green, or blue font. Subjects were instructed to name the font color (Figure 1A). Conflict arose when the font color did not match the meaning of the word. The Flanker task stimulus consisted of five arrows in a horizontal row, and subjects were asked to press the left or the right key to indicate the direction of the center arrow (Figure 1B). Conflict arose when the center arrow pointed in the opposite direction to the other four arrows. The Number task required subjects to say the position of the unique number (“one,” “two,” or “three”) among three numbers shown in a horizontal row (Figure 1C). Conflict arose when the position of the unique number did not match the actual number (e.g., number “3” in position 1 in the stimulus “322”). For all the tasks, congruent and incongruent conditions, as well as the stimulus dimensions (word, color, arrow direction, number identity, and position), were randomly interleaved and counterbalanced. Author Manuscript Subjects showed behavioral evidence of conflict in the three tasks Subjects showed high accuracy in all three tasks (Figures S1A-S1D): Stroop (congruent) = 96.8% ± 0.9%; Stroop (incongruent) = 90.1% ± 2.2%; Flanker (congruent) = 96.3% ± 2.8%; Flanker (incongruent) = 90.2% ± 2.9%; Number (congruent) = 96.6% ± 1.7%; Number (incongruent) = 90.4% ± 2.3% (mean ± SEM). On average, performance was higher in the congruent condition than the incongruent condition in all three tasks; this difference reached statistical significance in the Stroop task (p = 0.007, permutation test, 10,000 iterations), but not in the Flanker (p = 0.33) or Number (p = 0.39) tasks. These observations are consistent with previous works,11-15,28,33 and are mostly ascribed to a ceiling effect.34 Since accuracy was high in all the tasks, there were not enough error trials to have sufficient power to distinguish incongruent from congruent trials statistically. We focused exclusively on correct trials for the remainder of the study. Author Manuscript A hallmark of conflict in cognitive control tasks is the longer reaction time associated with incongruent than congruent trials (Figure 2). As demonstrated in previous works,11,15,28 reaction times were longer during incongruent trials for all three tasks (Stroop: 1,122 ± 8 versus 953 ± 7 ms, p < 0.001; Flanker: 875 ± 11 versus 722 ± 9 ms, p < 0.001; Number: 1,110 ± 8 versus 972 ± 8 ms, p < 0.001; mean ± SEM, permutation test, 10,000 iterations). The longer reaction times during incongruent trials were also statistically significant at the individual subject level in the majority of cases (Stroop: 16/16 subjects; Flanker: 14/16 Cell Rep. Author manuscript; available in PMC 2023 March 08. Xiao et al. Page 5 Author Manuscript subjects; Number: 15/16 subjects). Subject number 4 showed no significant difference in the Flanker and Number tasks, but this subject completed only half of a standard session. Absolute reaction times differed across tasks because of the distinct response modalities (verbal or keypress) because of the different number of response options (2 or 3), and because of the different sensory properties (language, shape, or number). Therefore, to assess the difficulty of each task, we computed the ratio of reaction times in incongruent versus congruent trials. There was no significant difference in difficulty among the three tasks (Figure S1E, p = 0.16, non-parametric one-way ANOVA). In sum, behavioral results were consistent with previous works and demonstrated almost ceiling accuracy and longer reaction time associated with incongruent trials in all three tasks. Neural responses were modulated by conflict Author Manuscript Author Manuscript We recorded intracranial field potential activity from 1,877 electrodes (Table S1 reports the number of electrodes in each subject). We analyzed the activity from 694 bipolarly referenced electrodes that were not in the white matter (STAR Methods); Figure 3 and Table S2 report the distribution of electrode locations. We focused on the neural activity in the theta band (4–8 Hz) because it constitutes a key component of cognitive control35-38 and also in the high-gamma band (70–120 Hz) given its significance in sensory, motor, control, and other cognitive functions.11,27,39-41 Additional results in other frequency bands (alpha, beta, low-gamma) are reported in Table S8. In previous work,11 we reported that multiple electrodes showed activity in the high-gamma band that was modulated by the presence of conflict during the Stroop task. Consistently, Figure 4 (left) depicts the high-gamma activity during the Stroop task of an electrode, located in the left orbitofrontal cortex, that showed enhanced responses during incongruent trials compared with congruent trials when aligning the neural signals to the behavioral response. The differences between incongruent and congruent trials were highly robust and could even be discerned in individual trials (compare Figure 4C, left, versus Figure 4B, left). Notably, the enhancement associated with conflict was also evident when the neural responses were aligned to stimulus onset (Figure 4D, left). Author Manuscript An electrode was considered to be conflict-modulated if the band-filtered power during incongruent conditions was significantly different from that during congruent conditions for at least 150 consecutive milliseconds (permutation test, 5,000 iterations, α = 0.05) both when responses were aligned to the behavioral response (Figure 4A, left) and to the stimulus onset (Figure 4D, left, STAR Methods). These strict selection criteria using both alignment to behavior and stimulus were implemented to exclude potential false positives. For example, signals from a visually responsive electrode could be confused for conflict modulation when aligning the neural responses to behavior due to the different reaction times between congruent and incongruent trials (Figure 2). An example visually responsive electrode located in the right lateral occipital cortex is shown in Figures S2A and S2B. Even though there was a difference between incongruent and congruent conditions when neural signals were aligned to the behavioral response (Figure S2A), this difference was absent when the neural signals were aligned to the stimulus onset (Figure S2B). Therefore, we did not consider this type of response to reveal any conflict modulation. Similarly, a motor responsive electrode could also be confused for conflict modulation when aligning the neural signals to stimulus onset for the same reasons (Figures S2C and S2D). Thus, Cell Rep. Author manuscript; available in PMC 2023 March 08. Xiao et al. Page 6 Author Manuscript the evaluation criteria for conflict modulation excluded purely sensory and purely motor responses. Author Manuscript Figure 4 shows an example electrode that revealed conflict modulation in the high-gamma band during the Stroop task. Electrodes demonstrating robust conflict modulation were also observed during the Flanker and Number tasks. Figure 5A (middle) depicts the responses of an electrode in the right superior parietal lobule that showed enhanced activity during incongruent trials in the Flanker task only. As described for the Stroop task, conflict modulation was observed in single trials (Figure S3A, middle) and also when aligning the responses to stimulus onset (Figure S3B, middle). Figure 5B (right) depicts the responses of an electrode in the right precuneus that showed enhanced activity during incongruent trials in the Number task only. Figure S4A (right) shows conflict modulation for this electrode during single trials and Figure S4B confirms this conflict modulation when aligning neural activity to the stimulus onset. Similar results were observed when considering the theta frequency band. Figure S5 shows an example electrode in the right pars triangularis that demonstrated conflict modulation in the theta band during the Stroop task only. Such modulation can be appreciated both in response-aligned signals (Figure S5A) and stimulus-aligned signals (Figure S5C), as well as in individual trials (Figure S5B). Conflict modulation within the theta band also occurred in the Flanker and Number tasks. Author Manuscript Out of the total of 694 electrodes, we identified 134 electrodes (19%) that exhibited conflict modulation in at least one task in the high-gamma band (Table S3) and 109 electrodes (16%) when considering the theta band (Table S4). Table S5 displays the number of electrodes modulated by conflict in each subject. Given the heterogeneity in electrode locations, which are dictated by clinical criteria, the distribution of electrodes modulated by conflict varies among subjects. In most cases, conflict modulation was characterized by enhanced band power in the incongruent condition than the congruent condition, as illustrated in the three example electrodes in Figures 4 and 5. A few electrodes exhibited the reverse modulation direction where the congruent response was higher than the incongruent one (Figure S9A, middle). Figure S6 shows the distribution of locations of electrodes revealing conflict modulation in each task. In sum, using strict criteria, we found electrodes that demonstrated robust conflict modulation in each of the three tasks, considering both high-gamma and theta band signals, evident in both behavior-aligned and stimulus-aligned responses, and even in single trials. Author Manuscript Neural signals in the high-gamma band during incongruent trials correlated with reaction times Next we examined whether the neural signals are correlated with reaction times. For each conflict-modulated electrode, we plotted the mean high-gamma band power as a function of the reaction time in each trial (STAR Methods). Figure S7A shows an example electrode located in the left rostral middle frontal cortex that was modulated by conflict during the Stroop task. The mean high-gamma power was not correlated with reaction times during congruent trials (p = 0.3, Figure S7A, left), but there was a significant correlation during incongruent trials (p = 0.03, Figure S7A, right). Similarly, Figure S7B shows an example Cell Rep. Author manuscript; available in PMC 2023 March 08. Xiao et al. Page 7 Author Manuscript electrode in the right superior frontal cortex that showed a correlation with reaction times during the Flanker task and Figure S7C shows an example electrode in the right inferior temporal cortex that showed a correlation with reaction times during the Number task. In all, 8.3%, 12.2%, and 10.2% of the conflict modulated electrodes showed a correlation with reaction times during incongruent trials, but not congruent trials, for the Stroop, Flanker, and Number tasks, respectively. Author Manuscript These observations did not extend to the theta band. Signals in the theta band showed a weaker correlation with reaction times. We found only four conflict-modulated electrodes (two in the Flanker task, two in the Number task, and none in the Stroop task) that demonstrated statistically significant correlation between theta band power and reaction times. These results are consistent with a previous study that examined multiple frequency bands but only observed correlation with reaction times in the high-gamma but not theta or beta bands using the Stroop paradigm only.11 Conflict representation exhibited within-task invariance In each task, there were different stimuli that defined conflict. For example, in the Stroop task, there were six different word/color combinations that were incongruent and three that were congruent (Figure 6A-I). Our first hypothesis states that conflict modulation is invariant to the different stimuli defining incongruence within a task. To test this hypothesis, we evaluated whether the modulation of neural signals underlying conflict was evident only for certain stimuli defining incongruent trials but not other stimuli within each task, as opposed to a uniform modulation due to incongruent trials across the different stimuli within each task. Author Manuscript Author Manuscript One might expect stimulus specificity given the extensive documentation of selective responses to different sensory inputs (e.g., Liu et al.,40 among many others). For example, an electrode located in visual cortical area V4 might be selective for color and respond differentially to red but not to blue or green. Indeed, consistent with previous work, we found multiple visually selective electrodes (Stroop: 15 electrodes; Flanker: 8 electrodes; Number: 0 electrodes; total = 23 electrodes; STAR Methods; Table S6). Similarly, we found 36 motor-selective electrodes (verbal response: 26 electrodes; keypress response: 10 electrodes; total = 36 electrodes; STAR Methods; Table S7). Among these 23 + 36 = 59 electrodes, there were only 5 electrodes (3 visually selective electrodes and 2 motor-selective electrodes) that showed both visual or motor selectivity and conflict modulation in the same task. These 5 electrodes constitute 8% of the visual/motor selective electrodes and 4% of all the electrodes that showed conflict modulation. Thus, the majority of electrodes that showed conflict modulation were not visually or motor selective. To further investigate whether conflict modulation generalized across different sensory inputs, we directly compared the responses to all possible stimuli within each task. Figures 6A-6I describe the responses of an electrode in the left inferior parietal cortex for every word/color combination during the Stroop task. Conflict modulation cannot be ascribed to responses to specific semantic/color combinations; that is, conflict modulation showed within-task invariance with enhanced responses during incongruent trials for the six different possible incongruent semantic and font color combinations compared with the Cell Rep. Author manuscript; available in PMC 2023 March 08. Xiao et al. Page 8 Author Manuscript three different possible congruent semantic and font color combinations. Similarly, Figures 6J-6M describe the responses of an electrode in the right superior frontal gyrus for every combination of central and peripheral arrow directions during the Flanker task. Conflict modulation in the Flanker task was also invariant within the task; that is, there was higher activity during the two incongruent target/flanker combinations compared with the two congruent combinations. Figures 6N-6S describe the responses of an electrode in the right superior parietal lobule, showing that conflict modulation was evident for all the different incongruent conditions in the Number task. Such within-task invariance was also observed in the theta frequency band. Author Manuscript Author Manuscript To characterize the degree of within-task invariance at the electrode ensemble level, we used a machine learning decoding approach to assess whether we could decode the presence of conflict in individual trials (Figures 6T and 6U). In all the decoding analyses, a support vector machine (SVM) classifier with a linear kernel was trained after concatenating all the conflict modulated electrodes in each task. We used two neural features: the maximum and the mean band power during each trial (STAR Methods), either for the high-gamma band (Figure 6T) or the theta band (Figure 6U). In all cases, we used cross-validation, separating the data into a training set and an independent test set and randomly subsampled the data to ensure that the number of congruent trials matched the number of incongruent trials. To evaluate within-task invariance, the classifier was trained using only a subset of the different stimulus combinations and tested on different stimulus combinations. For example, in the first bars in Figures 6T and 6U, the SVM classifier was trained with the neural responses to GREEN/red, GREEN/green, GREEN/blue, BLUE/red, BLUE/green, and BLUE/blue. The classifier’s performance was tested using the remaining conditions: RED/red, RED/green, and RED/blue. Even though the classifier was never exposed to the neural responses to any stimulus with the word “RED” during training, the classifier could extrapolate to identify conflict with those novel stimuli in the same task. Similar conclusions were reached for the other possible combinations of training and test stimuli within the Stroop task (Figures 6T and 6U, red bars) and also for the different combinations in the Flanker (yellow bars) and Number (blue bars) tasks. In sum, both at the individual electrode level and the electrode population level, and both in the high-gamma and theta bands, the results support the hypothesis that the neural signals modulated by conflict are largely independent of the specific sensory combination of stimuli that give rise to incongruence within each task. Conflict-modulated electrodes were task specific Author Manuscript Given the extrapolation across stimuli within a task, we next considered the hypothesis that neural signals representing conflict would also be independent of the specific sensory and motor characteristics of the task. We asked whether electrodes showing conflict modulation were task specific (i.e., showing activity modulation during incongruent trials in some but not all tasks) or task invariant (i.e., showing activity modulation during incongruent trials in all three tasks). The examples in Figures 4, 5, and S3-S5 illustrate example electrodes with high specificity in their degree of conflict modulation. The electrodes in Figures 4 and S5 only reveal conflict modulation during the Stroop task (compare leftmost column to middle and rightmost columns). Similarly, the electrodes in Figures 5A and S3 show conflict modulation only during the Flanker task (middle column), and the electrodes in Figures 5B Cell Rep. Author manuscript; available in PMC 2023 March 08. Xiao et al. Page 9 Author Manuscript and S4 only during the Number task (rightmost column). These types of neural responses were representative of the majority of the data. Out of the total of 134 electrodes that showed conflict modulation in the high-gamma band, 118 electrodes (88%) exhibited modulation in one task but not in the other two tasks. Similarly, out of the total of 109 electrodes that showed conflict modulation in the theta band, 92 electrodes (84%) exhibited modulation in one task but not in the other two tasks. Author Manuscript Although most electrodes demonstrated conflict modulation in one task only, there were 16 electrodes in the high-gamma band (12%) and 17 electrodes (16%) in the theta band that showed conflict modulation in two tasks. Three example electrodes that showed conflict modulation in two tasks are illustrated in Figures S8 and S9. In Figure S8A, an electrode in the left inferior parietal cortex exhibited conflict modulation during the Stroop and Flanker tasks, but not during the Number task. Similarly, Figure S8B shows an electrode at the right supramarginal gyrus, demonstrating conflict modulation in the Stroop and Number tasks, but not during the Flanker task. Figure S8C shows an electrode in the insula exhibiting conflict modulation during the Flanker and Number tasks, but not during the Stroop task. These electrodes also showed conflict modulation when the neural signals were aligned to stimulus onset (Figure S9). Tables S3 and S4 report the locations and task specificity for all the dual-task modulated electrodes for the high-gamma and theta bands, respectively. Author Manuscript Author Manuscript In sum, most electrodes demonstrated conflict modulation in one task and a few electrodes showed conflict modulation in two tasks. Remarkably, we did not find any electrode that was modulated by conflict in all three tasks. Given the absence of any task-invariant electrode, we asked whether it is possible that we missed indications of invariance due to our stringent criteria. First, we considered whether it is possible that having elevated activity in the congruent condition could be a prerequisite to observe conflict modulation. The electrode in Figure 4 showed conflict modulation in the Stroop task but not in the other two tasks. During the Flanker task, this electrode showed no elevated response whatsoever, and, during the Number task, there was a high response with respect to baseline starting about 0.7 s before the behavioral response, but this increase was very much the same for congruent and incongruent trials. Thus, there can be activation in the congruent condition without conflict modulation. Similarly, in the example electrode in Figure 5B, there was an elevated response in the congruent condition during all three tasks; however, conflict modulation occurred only in the Number task. In total, 302 electrodes showed elevated high-gamma band responses during the congruent condition in at least one task. Among these electrodes, only 80 (26%) also showed conflict modulation. Moreover, the majority of these electrodes (70 out of 80) did not share the same task specificity, i.e., tasks showing conflict modulation did not match tasks displaying responses during the congruent conditions. In sum, multiple electrodes responded during the congruent condition without conflict modulation, and multiple electrodes showed conflict modulation only in some task(s) while still showing a response during the congruent condition in other task(s). Thus, an elevated response during the congruent condition is neither necessary nor sufficient to show evidence of conflict modulation. Lack of task invariance cannot be attributed to lack of response during the congruent condition. Cell Rep. Author manuscript; available in PMC 2023 March 08. Xiao et al. Page 10 Author Manuscript Author Manuscript Second, we asked whether lack of invariance could be attributed to the behavioral performance by subjects in a given task. If conflict signals from a subject demonstrated Stroop specificity, one may ask whether this subject only performed the Stroop task adequately. In an extremely hypothetical situation, if a subject closed their eyes during the other two tasks we would not expect to observe conflict signals in the other tasks. Several pieces of evidence argue against this possibility. Most subjects showed high accuracy in all tasks, except subjects 1 and 6, who performed less well on the Number and Flanker tasks, respectively (Figures S1A-S1C). Subject 6 performed the Flanker task only slightly above chance, but this subject still had Flanker-modulated electrodes. Furthermore, almost all subjects showed a clear behavioral conflict effect in the three tasks (Figure 2), except for subject 4 who performed half of the sessions and did not show behavioral conflict in the Flanker and Number tasks (Figures 2B and 2C). All subjects experienced conflict during the Stroop task at the behavioral level (Figure 2A). However, electrodes, such as the ones shown in Figures 5, S3, S4, and S8C, clearly demonstrated no evidence of conflict modulation in the Stroop task. One may also ask whether Stroop-specificity could arise due to increased or reduced difficulty in the Stroop task, but this is not the case either. Subject 2 showed comparable reaction times with the Stroop and Number tasks and similar differences in reaction time between congruent and incongruent trials. However, this subject had zero Stroop-specific electrodes but 22 Number-specific electrodes considering the high-gamma band. Furthermore, by calculating the radio of incongruent RT to congruent RT as a proxy of task difficulty, there was no significant variation in how difficult these tasks were. Finally, and even more conclusively, there were many examples of different electrodes in the same subject showing task specificity for conflict modulation in different tasks. Therefore, lack of task invariance cannot be ascribed to cases where subjects showed adequate performance or conflict effect in one task but not others at the behavioral level. Author Manuscript Author Manuscript Third, the results presented thus far focus on the high-gamma and theta frequency bands. Although different frequency bands of intracranial field potential signals tend to be correlated,42 it is conceivable that some of the electrodes may reveal task invariance in conflict modulation in other frequency bands. To evaluate this possibility, we repeated all the analyses in the following frequency bands (STAR Methods): alpha (8–12 Hz), beta (12–35 Hz), and low-gamma (35–70 Hz). Table S8 reports the number of electrodes that showed conflict modulation for each task and for each frequency band. Summarizing Table S8, we found conflict modulation in all frequency bands, although the total number of electrodes that showed modulation was highest in the high-gamma band, followed by the theta band. Consistent with the results described in the previous sections, the vast majority of electrodes revealed conflict modulation only in one task: alpha, 88%; beta, 94%; low-gamma, 93% (cf. 88% for the high-gamma band and 84% for the theta band). In all frequency bands, we observed a small fraction of electrodes that showed conflict modulation in two tasks. Importantly, we did not find any electrode that showed task invariance in any of these other frequency bands. Finally, the results presented thus far relied on highly rigorous pre-processing through bipolar referencing and stringent selection criteria by requiring a long window of 150 ms to identify significant differences between incongruent and congruent trials and 5,000 iterations of a permutation test. We relaxed all of these constraints by using global referencing, Cell Rep. Author manuscript; available in PMC 2023 March 08. Xiao et al. Page 11 Author Manuscript by evaluating a shorter duration threshold of 50 ms, and using a t test. We analyzed 748 electrodes (this is more than the 694 electrodes reported so far because bipolar referencing reduces the number of electrodes). Using these more liberal criteria, we found two electrodes in the high-gamma band that showed task invariance, one located in the left superior frontal gyrus and the other in the right rostral middle frontal gyrus. Neural responses from one of these two electrodes is shown in Figure S10. Although the neural signals from this electrode are less compelling than the examples showing task modulation in one or two tasks, these observations hint at the possibility of a weaker signal common across tasks. Yet, even under these liberal selection criteria, only 0.3% of the total number of electrodes that we studied demonstrated task invariance in the high-gamma band and none in the other frequency bands. Author Manuscript In sum, these observations show that most of the electrodes reveal conflict modulation in only one task, and few electrodes show conflict modulation in two tasks. These results led us to reject our second hypothesis of task invariance in cognitive control at the level of individual electrodes in most part of the brain. Electrode population level responses revealed task-specific conflict modulation in individual trials Author Manuscript It is conceivable that individual electrodes could show task specificity while multiple electrodes might reflect task invariance. First, task specificity relied on a statistical threshold and there could be weak modulation in other tasks that did not pass our strict statistical criteria. Second, machine learning classifiers show more power in detecting small changes in activity in individual trials when combining data across electrodes. To evaluate the possibility that task invariance may appear within electrode ensembles, we investigated whether we could decode the presence of conflict at the electrode population level in individual trials following the same procedure described in Figures 6T and 6U. Depending on the specific question about task specificity, each calculation used different combinations of training and test sets, as described below. Author Manuscript As a sanity check to evaluate the methodology, we first tested classifiers trained using all conflict-modulated electrodes (Figure S11). These classifiers were able to detect conflict at above chance levels in all three tasks and showed minimal extrapolation across tasks. Similar conclusions are reached when considering all electrodes (regardless of whether conflict-modulated or not) in specific regions that may be thought to be involved in cognitive control, such as the prefrontal cortex (Figure S12). However, this analysis does not allow us to assess whether there is a task-invariant representation because the classifier includes multiple electrodes that show modulation in different tasks. Therefore, we asked whether the population of electrodes modulated by one task could classify the presence of conflict from another task. We trained nine different classifiers using the features of high-gamma (Figure 7A) and theta (Figure 7C) band power. Following the methods in the decoding analysis for assessing within-task invariance, we used the maximum and the mean power as the training and test features for each trial. The first three bars used Stroop-only electrodes (like the one in Figure 4), the middle three bars used Flanker-only electrodes (like the one in Figure 5A), and the last three bars used Cell Rep. Author manuscript; available in PMC 2023 March 08. Xiao et al. Page 12 Author Manuscript Number-only electrodes (like the one in Figure 5B). The classifiers were trained and tested using cross-validation incorporating features from the Stroop task only (red), Flanker task only (yellow), or Number task only (blue). The Stroop-only electrode population yielded classification performance that was significantly higher than chance when trained and tested on the Stroop task (p < 0.001, permutation test, 10,000 iterations, one-sided, Figures 7A and 7C, bar 1), and the Flanker-only population yielded significant classification performance when trained and tested on the Flanker task (p < 0.001, Figures 7A and 7C, bar 5). The population of Number-only electrodes yielded significant classification performance when trained and tested on the Number task (p < 0.001, Figures 7A and 7C, bar 9), but also when trained and tested on the Stroop task (p < 0.01, Figures 7A and 7C, bar 7). Although the Number-only electrode population could detect conflict in the Stroop task, the performance on the Number task was still significantly higher than that on the Stroop task (p < 0.001). Author Manuscript Author Manuscript We next sought to assess whether a classifier trained only with data from one task could extrapolate to detect conflict in different tasks. We trained three classifiers, one taking features from the Stroop task only, one taking features from the Flanker task only, and one taking features from the Number task only for high-gamma (Figure 7B) and theta (Figure 7D) bands. Then we tested each classifier with data from the Stroop, Flanker, and Number tasks, respectively. We performed this analysis using Stroop-only, Flanker-only, and Number-only electrodes. Note that this was different from the analyses in Figures 7A and 7C, where the training and test data were always from the same task for each classifier, whereas, here, the training and test data could be from different tasks. Here, even when the classifier was trained and tested using features from the same task, we still performed cross-validation among trials to avoid overfitting. The results of these crosstask classification analyses are presented in Figures 7B (high-gamma) and 7D (theta). The highest classification accuracies were observed for within-task training and testing (diagonal tiles). These three conditions not only exhibited better than chance accuracies (high-gamma: 82.6% ± 6.9%; theta: 79.5% ± 8.2%, mean ± SEM), but also significantly higher performance than all the corresponding cross-task accuracies (high-gamma: 55.3% ± 4.3%, p < 0.001; theta: 53.6% ± 5.0%, p < 0.001, permutation test, 10,000 iterations, one-sided). In sum, even at the electrode population level, we observed minimal ability to detect conflict when a decoder was trained and tested in different tasks. In contrast, using exactly the same approach but even fewer trials in each condition, there was high accuracy in distinguishing conflict in individual trials within each task. These results show that, at the same electrode population level, within-task invariance is significantly more prominent than cross-task invariance. Author Manuscript DISCUSSION We studied the neural mechanisms underlying conflict resolution during cognitive control by recording intracranial field potentials from 694 electrodes in 16 subjects who performed three different tasks: Stroop, Flanker, and Number (Figure 1). Subjects showed increased reaction times during incongruent trials compared with congruent trials (Figure 2), a hallmark of cognitive control.12-14,16 Consistent with previous studies,11,25,26,43 we found robust modulation of neural signals in the high-gamma frequency band when comparing incongruent versus congruent trials (Figures 4 and 5). Conflict modulation was also present Cell Rep. Author manuscript; available in PMC 2023 March 08. Xiao et al. Page 13 Author Manuscript in the theta band (Figure S5) and other frequency bands (Table S8). Modulation was evident both when aligning neural signals to the behavioral responses and to stimulus onsets (Figures 4, S3-S5, and S9), could be appreciated even in single trials (Figures 4 and S3-S5), and showed within-task invariance to the different combinations of visual inputs (Figure 6). Surprisingly, despite this robust within-task invariance, most of the electrodes showed task specificity, with clear incongruent/congruent modulation in only one task but not in the other two (Figures 4 and 5). A few electrodes showed task-modulation in two tasks but not the third task (Figures S8 and S9). Author Manuscript Author Manuscript We were concerned about multiple potential factors that could masquerade as task specificity. First, if subjects failed to perform a given task adequately or failed to show conflict at the behavioral level in a given task, one may wrongly conclude that there is task specificity but no task invariance in the neural responses. However, all subjects revealed high accuracy in the three tasks (Figures S1A-S1D), and the vast majority of subjects showed conflict at the behavioral level (Figure 2). We observed task specificity in the neural signals even in subjects that showed conflict at the behavioral level in all three tasks. Furthermore, there were electrodes in the same subject with different task specificity, ruling out an explanation of task specificity based on behavioral differences. Another potential behavioral factor that could correlate with task specificity would be if one task showed more "conflict" or was more difficult than the other tasks. However, the reaction times showed that the three tasks were comparable in terms of their degree of behavioral conflict (Figure S1E). We used stringent criteria to ascribe conflict modulation to an electrode (STAR Methods). We considered whether it is possible that task specificity could be dictated by a lack of response in certain tasks even during the congruent trials, but it turned out that this is not the case. We showed that an elevated response during the congruent condition was neither necessary nor sufficient to show evidence of conflict modulation. We also considered whether our strict selection criteria could have biased the interpretation of our results. However, an inspection of individual trials reveals robust modulation during incongruent trials compared with congruent trials in some tasks but not others (Figures 4, 5, and S3-S5). Even after relaxing pre-processing by using a global reference and a much less stringent statistical threshold, only two electrodes (less than 1%) revealed task invariance. Similar conclusions were reached when examining other frequency bands (Table S8). Author Manuscript We focused on the time window within a trial, from the stimulus onset to the behavioral response time, when conflict resolution processes take place. We did not consider prestimulus and post-response time periods, which may represent estimation of the conflict element in upcoming trials and post-response feedback signals. Neural responses in these distinct windows may carry different operations and should not be confused with each other, which requires methods with high temporal resolution, such as intracranial recordings. We also considered an electrode ensemble machine learning decoding approach (Figures 6 and 7). Population-based decoding is highly sensitive and could, in principle, uncover a task-invariant representation even if we mainly observe specificity in individual trials and individual electrodes. However, the decoding results also support the conclusion of clear within-task invariance (Figures 6T and 6U) and a largely task-specific representation (Figure 7). It is important to note that we used the same electrode populations, covering exactly Cell Rep. Author manuscript; available in PMC 2023 March 08. Xiao et al. Page 14 Author Manuscript the same brain regions, in the two analyses. These decoding results cannot be ascribed to drifting neural signals or non-stationarities in the data. First, previous work showed that intracranial field potentials tend to be very stable within a session, and even across recording sessions spanning multiple days.42 Second, the total of 18 blocks with different tasks were randomly interleaved and the signals were still more consistent within a task than across tasks. Author Manuscript The task-specific electrodes were located in multiple regions within the frontal, parietal, and temporal lobes, and, to a lesser degree, the occipital lobe. Several studies documented differential activation during incongruent versus congruent trials in the frontal cortex.11,18,20,21,25,28,44,45 The locations shown in Figure S6 and Tables S3 and S4 are also consistent with many studies documenting responses during cognitive control in the parietal cortex,14,18-20 temporal cortex,14,20 occipital cortex,20,24,46 and other brain areas, such as the insula.47 These results suggest that cognitive control processes recruit distributed and task-specific networks rather than a single brain region.20,48-50 Furthermore, the results are consistent with the theoretical notion that conflict enhances selective and task-relevant signals in cortex. Author Manuscript Several previous studies reported signals that differ between incongruent and congruent trials at the level of individual neurons,28,51 intracranial field potentials,11,25-27 in scalp electroencephalography signals,21,23,24 and in functional neuroimaging signals.8,18,44,45 Most previous studies leveraged a single task, precluding the possibility to assess task invariance and task specificity in conflict modulation. It is possible to draw inferences about potential invariance by comparing results in different studies; however, precise anatomical comparisons across subjects can be challenging, especially when considering coarse signals that smooth over large numbers of neurons. Inferences across studies do not necessarily imply that the same neural circuits represent conflict in an abstract format. Another potential confound is the distinction between signals aligned to the stimulus or to the behavioral response, which requires a careful comparison of the temporal dynamics of the neural responses. Stimulus-specific neural signals could be misconstrued as conflict modulation if neural responses are aligned to the motor output (e.g., Figures S2A and S2B), and motorspecific neural signals could be misconstrued as conflict modulation if neural responses are aligned to the stimulus onset (e.g., Figures S2C and S2D). Thus, either due to single-task studies or spatial and temporal averaging, it is difficult to differentiate whether the conflictassociated neural activities in many previous studies reflected task-specific modulation or an abstract conflict signal. Author Manuscript We deliberately designed the tasks to be different in terms of the sensory inputs and motor outputs. Conflict relies on a discrepancy between color and semantic meaning (Stroop), comparison between shapes (Flanker), and the meaning of numbers and positional encoding (Number). Subjects used either verbal responses (Stroop, Number) or keypress responses (Flanker) as output. We conjectured that a general, abstract, signature of cognitive control should be independent of the inputs and outputs that define conflict. However, we found no such task-invariant conflict signals. It is tempting to assume that neural signals from electrodes that show conflict modulation in two tasks (e.g., Figures S8 and S9) correlate with the common aspects of two tasks. For example, electrodes that showed modulation Cell Rep. Author manuscript; available in PMC 2023 March 08. Xiao et al. Page 15 Author Manuscript exclusively during the Stroop and Number tasks (e.g., Figure S8B) might be involved in conflict expressed through verbal output. However, caution should be exercised in this type of interpretation because we did not test the Flanker task using a verbal output. Future experiments may incorporate diverse output and input modalities within and across tasks to address this question. The majority of electrodes responded in a task-specific manner, arguably demonstrating engagement in conflict through specific sensory-motor combinations. These task-specific electrodes exhibit generalized conflict signals within a task. Collectively, our results indicate that cognitive control is orchestrated by largely distinct and distributed networks dictated by shared processes within a task and the specific demands of each task. Limitations of the study Author Manuscript The results on within-task invariance are based on demonstrating similar responses for different sensory-motor combinations in each task. The results showing task specificity are based on positive evidence of electrodes that separately show modulation by conflict in each of the three tasks. In contrast, the results on lack of cross-task invariance are based on not finding any regions demonstrating an abstract representation of conflict. We performed extensive controls to search for such an abstract representation (single trials, averages over trials, different frequency bands, behavioral controls, and electrode population analyses based on machine learning). However, as often stated, absence of evidence does not imply evidence of absence. Author Manuscript First, our sampling of brain locations was extensive but certainly not exhaustive (Figure 3). By combining multiple subjects we achieved a fairly good coverage of most regions (Figure 3). However, it is still quite possible that there are other brain regions that represent conflict in a task-invariant fashion that we could not sample here. Author Manuscript Second, our study focuses on intracranial field potentials; these signals combine the activity of large numbers of neurons. It is conceivable that individual neurons might show more, or less, task invariance than the results reported here. Studies examining single-unit activity in the frontal cortex are also consistent with a lack of task invariance in cognitive control.10,51,52 Several studies have shown that frontal cortex neurons demonstrate “mixed selectivity.53” Such mixed selectivity is a good summary of the results described here at the level of intracranial field potentials, which seem to reflect a combination of conflict and task-specific demands. However, the contrast between within-task invariance and cross-task specificity could not be easily predicted from the theory of mixed selectivity. Our results are partially echoed by two recent single-neuron studies. One study54 examining neurons in the dACC and pre-SMA, regions that were traditionally believed to be primarily involved in domain-general cognitive control processes, found clear evidence of task-specific singleneuron activity. This study also showed conflict responses common to two tasks, Stroop and Number, when using the same behavioral response mode (button press). These observations are consistent with those electrodes that show conflict modulation in two tasks (e.g., Figure S8). It is possible that the responses in both tasks highlight the common sensorimotor transformation component, especially given that dACC neurons are specialized for representing task-state variables relevant for behavior and are tuned for a variety of Cell Rep. Author manuscript; available in PMC 2023 March 08. Xiao et al. Page 16 Author Manuscript sensory and motor elements.55 In contrast, we compared three tasks with either keypress or verbal responses and the fact that a great proportion of dual-task electrodes were selective for the Stroop and Number tasks but not the Flanker task adds weight to this interpretation. Another single-unit study52 concluded that firing rates of dACC neurons more likely amplify task-relevant sensorimotor information to facilitate conflict resolution than signal conflict abstractly. Furthermore, the role of the dACC during cognitive control has been called into question given that dACC ablation does not lead to changes in interference-dependent behavior.28 STAR★METHODS RESOURCE AVAILABILITY Author Manuscript Lead contact—Further information and requests should be directed to and will be fulfilled by the lead contact, Gabriel Kreiman ([email protected]). Materials availability—This study did not use or generate any reagents. Data and code availability—All the behavioral and neurophysiological data are publicly available at: https://klab.tch.harvard.edu/resources/XiaoEtAl_CognitiveControl.html and https://doi.org/10.5281/zenodo.7317346. All the code used to analyze the data are publicly available at: https://klab.tch.harvard.edu/ resources/XiaoEtAl_CognitiveControl.html. and https://doi.org/10.5281/zenodo.7317346. Any additional information required to reanalyze the data reported in this work paper is available from the lead contact upon request. Author Manuscript EXPERIMENTAL MODEL AND SUBJECT DETAILS Author Manuscript Subjects and recording procedures—Subjects were 16 patients (8 female, ages 12– 62, Table S1) with pharmacologically intractable epilepsy treated at Taipei Veterans General Hospital (TVGH), Boston Children’s Hospital (BCH), Brigham and Women’s Hospital (BWH), and Johns Hopkins Medical Hospital (JHMH). The electrode locations are purely dictated by clinical considerations, precluding any quantitative estimation of sample size at study design. The target number of subjects, 16, was decided during study design based on historical data of electrode distributions from previous studies. This study was approved by the institutional review board in each hospital and was carried out with subjects’ informed consent. Subjects were implanted with intracranial depth electrodes (Ad-Tech, Racine, WI, USA). The electrode locations were dictated by the clinical needs to localize the seizure focus in each patient.59 The total number of electrodes was 1,877 (Table S1). Neurophysiological data were recorded using XLTEK (Oakville, ON, Canada), Bio-Logic (Knoxville, TN, USA), Nihon Kohden (Tokyo, Japan), and Natus (Pleasanton, CA). The sampling rate was 2048 Hz at BCH and TVGH, 1000 Hz at JHMH, and 512 Hz at BWH. All data were bipolarly referenced, unless stated otherwise. There were no seizure events in any of the sessions. Electrodes in epileptogenic foci, as well as pathological areas, were removed from analyses. Cell Rep. Author manuscript; available in PMC 2023 March 08. Xiao et al. Page 17 METHOD DETAILS Author Manuscript Author Manuscript Task procedures—Each subject completed three tasks in a single recording session: Stroop, Flanker, and Number. A schematic rendering of the tasks is shown in Figure 1. Each session contained 18 blocks, with 30 trials of one task (Stroop, Flanker, or Number) per block. The target number of trials was pre-defined based on the results of one of our previous studies11 and based on a pilot study with 4 healthy volunteers where we confirmed that conflict (i.e., reaction time difference between congruent and incongruent trials) could be robustly detected with this number of trials. Per our IRB protocols, subjects can stop testing at any time; subjects who completed different numbers of blocks are indicated in Table S1 in bold font. Subjects completed the tasks in normally lighted and quiet rooms. The experiments were written and presented using the Psychtoolbox extension in Matlab_R2016b (Mathworks, Natick, MA). Subjects viewed and completed the experiment using a 13-inch Apple Mac laptop. Stimuli subtended approximately 5 degrees of visual angle and were centered on the screen. Before each experiment started, each subject went over a short practice session until the instructions were fully understood. During the actual experiment, no correct/incorrect feedback was provided. All trials started with 500 ms of fixation, followed by stimulus presentation. The stimulus was presented for 2,000 ms (Stroop, Number), or until the minimum of 2,000 ms and the subject’s key response time (Flanker). The stimuli were presented in white (Flanker, Number) or red/green/blue font color (Stroop), on a black background. For those subjects in Taipei, Stroop task stimuli were presented in traditional Chinese characters. Subjects provided a verbal response recorded using a Yeti microphone with an 8,192 Hz sampling rate (Stroop, Number), or a two-alternative keypress response using the left and right keys on the experiment laptop (Flanker). Author Manuscript Author Manuscript Electrode localization—We used the iELVis56 pipeline to localize the depth electrodes. Pre-implant MRI (T1, no contrast) was processed and automatically segmented by Freesurfer,57,60 followed by co-registering the post-implant CT to the processed MR images. Electrodes were then identified visually and marked in each subject’s co-registered space using the BioImage Suite.61 Each electrode was assigned an anatomical location (parcellated cortices,62 white matter, subcortical regions, or unknown locations) using the Freesurfer localization tool. Unknown locations could be due to brain lesions or pathological brain areas. Electrodes in the white matter, ventricles, cerebellum, and unknown locations were excluded from analyses. Out of a total of 1,877 electrodes, we included 694 bipolarly referenced electrodes in the analyses. To show the position of electrodes from different subjects (Figures 3 and S6), electrode locations were mapped onto the MNI305 average human brain via an affine transformation.63 QUANTIFICATION AND STATISTICAL ANALYSIS Behavioral analyses—The content of verbal responses (Stroop and Number tasks) was transcribed offline. The transcription was blind to the ground truth answers as well as neural responses. The behavioral reaction time for verbal response (Stroop and Number tasks) was determined as the first time the energy of the soundtrack was three standard deviations above the mean energy of the whole trial. Any noise (e.g., door slam, coughing, etc.) before Cell Rep. Author manuscript; available in PMC 2023 March 08. Xiao et al. Page 18 Author Manuscript the actual trial response was carefully identified and smoothed to prevent false automatic identification of behavioral response time. The keypress reaction time (Flanker task) was recorded by the Psychtoolbox code. Reaction times are shown in Figure 2. Preprocessing of intracranial field potential data—A zero-phase digital notch filter (Matlab function "filtfilt") was applied to the broadband signals to remove the AC line frequency at 60 Hz and harmonics. For each electrode and each task, trials with amplitudes (max-min voltage from fixation onset to stimulus off) larger than three standard deviations above the mean amplitude across all trials were considered as containing artifacts and excluded from analysis.42 The percentage of trials excluded by this criterion was 1.05% (Stroop), 1.25% (Flanker), and 1.29% (Number). Author Manuscript Single electrode analysis of modulation by conflict—We computed the highgamma band (70–120 Hz), low-gamma band (35–70 Hz), beta band (12–35 Hz), alpha band (8–12 Hz), and theta band (4–8 Hz) power of the intracranial field potential signals by using a multi-taper moving-window spectral estimation method implemented in the Chronux toolbox.58 The time-bandwidth product, number of tapers, and size of moving window used for each frequency band are listed in Table S7.11 Throughout the paper, we focus on the high-gamma band and theta band signals. The power in the corresponding frequency band was z-scored by subtracting the mean high-gamma power during the baseline period (500 ms before stimulus onset) and dividing by the standard deviation of the high-gamma power during the baseline. Only correct trials were included in the analyses. Author Manuscript Author Manuscript First, we examined whether an electrode exhibited any response at all to the stimuli. An electrode was defined as "responsive" if the z-scored high-gamma power during the congruent condition was larger than 1 for at least 150 consecutive milliseconds (15 × 200 ms window shifted by 10 ms), starting from stimulus onset to average behavioral response time. To determine whether an electrode showed conflict modulation (Tables S3 and S4, and S7), we compared the band power between the congruent and incongruent conditions of each task. For each time bin (200 ms shifted by 10 ms), we compared the band power of incongruent versus congruent trials using a permutation test with 5,000 iterations (α = 0.05). An electrode was denoted as showing conflict modulation if the following two criteria were satisfied: (1) The band power of incongruent trials was significantly different from the high-gamma power in congruent trials for at least 150 consecutive milliseconds (15 × 200 ms window shifted by 10 ms); (2) Criteria (1) was satisfied in both behavioral response-aligned and stimulus-aligned conditions.11 When the band power was aligned to behavioral response, selection criteria were applied to the time window starting from the average stimulus onset to the behavioral reaction time. When the band power was aligned to the stimulus, the time window was from stimulus onset to average behavioral reaction time. An electrode was considered to be visually responsive if the maximum z-scored highgamma band power was larger than 2 during the 300 milliseconds after stimulus onset. An electrode was considered showing motor responsive if the maximum z-scored high-gamma band power was larger than 2 during 300 milliseconds before behavioral response and the power continually increased during this time window. An electrode was considered to be visually selective for a particular task if it was visually responsive to stimuli only in one Cell Rep. Author manuscript; available in PMC 2023 March 08. Xiao et al. Page 19 Author Manuscript task. An electrode was deemed to show motor selectivity if it was motor responsive to verbal output (Stroop and Number) only or keypress (Flanker) only. These analyses are summarized in Tables S5 and S6. To assess the correlation between conflict responses and reaction times (Figure 6), a linear regression (“fitlm” function in Matlab) was performed between reaction time and the mean high-gamma or theta band power of each trial for all conflict-modulated electrodes. An electrode was considered to show a significant correlation if the p value of the linear regression slope was smaller than 0.05. Author Manuscript Classifier analyses—We quantified whether we could distinguish between congruent and incongruent trials in individual trials based on the activity of pseudo-populations formed by multiple electrodes.40 We used a linear-kernel support vector machine with ten-fold crossvalidation for all the classifier analyses (Figures 7 and 8). Two features were calculated for each trial from each electrode: the mean and maximum band power from average stimulus onset to the behavioral response. These analyses were conducted separately for the high-gamma and theta frequency bands. All data were normalized to zero mean and standard deviation 1 before each training and testing session. All the classifier performance results reported are based on cross-validated test data. The main text and Figures 7 and 8 describe all the different combinations of training and test data used, which are critical to evaluate within-task and between-task invariance. Supplementary Material Refer to Web version on PubMed Central for supplementary material. Author Manuscript ACKNOWLEDGMENTS This work was supported by NIH grant R01EY026025 and by the Center for Brains, Minds and Machines, funded by NSF Science and Technology Center Award CCF-1231216. REFERENCES Author Manuscript 1. Gratton G, Coles MG, and Donchin E (1992). Optimizing the use of information: strategic control of activation of responses. J. Exp. Psychol. Gen 121, 480–506. 10.1037//0096-3445.121.4.480. [PubMed: 1431740] 2. Miller EK, and Cohen JD (2001). An integrative theory of prefrontal cortex function. Annu. Rev. Neurosci 24, 167–202. 10.1146/annurev.neuro.24.1.167. [PubMed: 11283309] 3. Diamond A (2013). Executive functions. Annu. Rev. Psychol 64, 135–168. 10.1146/annurevpsych-113011-143750. [PubMed: 23020641] 4. Goschke T (2014). Dysfunctions of decision-making and cognitive control as transdiagnostic mechanisms of mental disorders: advances, gaps, and needs in current research. Int. J. Methods Psychiatr. Res 23 (Suppl 1), 41–57. 10.1002/mpr.1410. [PubMed: 24375535] 5. Lesh TA, Niendam TA, Minzenberg MJ, and Carter CS (2011). Cognitive control deficits in schizophrenia: mechanisms and meaning. Neuropsychopharmacology 36, 316–338. 10.1038/ npp.2010.156. [PubMed: 20844478] 6. Zilverstand A, Huang AS, Alia-Klein N, and Goldstein RZ (2018). Neuroimaging impaired response inhibition and salience attribution in human drug addiction: a systematic review. Neuron 98, 886– 903. 10.1016/j.neuron.2018.03.048. [PubMed: 29879391] Cell Rep. Author manuscript; available in PMC 2023 March 08. Xiao et al. Page 20 Author Manuscript Author Manuscript Author Manuscript Author Manuscript 7. Botvinick MM, Braver TS, Barch DM, Carter CS, and Cohen JD (2001). Conflict monitoring and cognitive control. Psychol. Rev 108, 624–652. 10.1037/0033-295x.108.3.624. [PubMed: 11488380] 8. Goghari VM, and MacDonald AW 3rd. (2009). The neural basis of cognitive control: response selection and inhibition. Brain Cognit. 71, 72–83. 10.1016/j.bandc.2009.04.004. [PubMed: 19427089] 9. Ridderinkhof KR, Ullsperger M, Crone EA, and Nieuwenhuis S (2004). The role of the medial frontal cortex in cognitive control. Science 306, 443–447. 10.1126/science.1100301. [PubMed: 15486290] 10. Fu Z, Wu DAJ, Ross I, Chung JM, Mamelak AN, Adolphs R, and Rutishauser U (2019). Singleneuron correlates of error monitoring and post-error adjustments in human medial frontal cortex. Neuron 101, 165–177.e5. 10.1016/j.neuron.2018.11.016. [PubMed: 30528064] 11. Tang H, Yu HY, Chou CC, Crone NE, Madsen JR, Anderson WS, and Kreiman G (2016). Cascade of neural processing orchestrates cognitive control in human frontal cortex. Elife 5, e12352. 10.7554/eLife.12352. [PubMed: 26888070] 12. Stroop JR (1935). Studies of Interference in Serial Verbal Reactions (Ph D (George Peabody College for Teachers)). 13. Eriksen BA, and Eriksen CW (1974). Effects of noise letters upon the identification of a target letter. Percept. Psychophys 16, 143–149. 14. Bush G, and Shin LM (2006). The Multi-Source Interference Task: an fMRI task that reliably activates the cingulo-frontal-parietal cognitive/attention network. Nat. Protoc 1, 308–313. 10.1038/ nprot.2006.48. [PubMed: 17406250] 15. Davelaar EJ, and Stevens J (2009). Sequential dependencies in the Eriksen flanker task: a direct comparison of two competing accounts. Psychon. Bull. Rev 16, 121–126. 10.3758/PBR.16.1.121. [PubMed: 19145021] 16. Mayr U, Awh E, and Laurey P (2003). Conflict adaptation effects in the absence of executive control. Nat. Neurosci 6, 450–452. 10.1038/nn1051. [PubMed: 12704394] 17. Simon JR, and Berbaum K (1990). Effect of conflicting cues on information processing: the ’Stroop effect’ vs. the ’Simon effect. Acta Psychol. 73, 159–170. 10.1016/0001-6918(90)90077-s. 18. Bunge SA, Hazeltine E, Scanlon MD, Rosen AC, and Gabrieli JDE (2002). Dissociable contributions of prefrontal and parietal cortices to response selection. Neuroimage 17, 1562–1571. 10.1006/nimg.2002.1252. [PubMed: 12414294] 19. Coulthard EJ, Nachev P, and Husain M (2008). Control over conflict during movement preparation: role of posterior parietal cortex. Neuron 58, 144–157. 10.1016/j.neuron.2008.02.009. [PubMed: 18400170] 20. Fan J, Flombaum JI, McCandliss BD, Thomas KM, and Posner MI (2003). Cognitive and brain consequences of conflict. Neuroimage 18, 42–57. 10.1006/nimg.2002.1319. [PubMed: 12507442] 21. Robertson JA, Thomas AW, Prato FS, Johansson M, and Nittby H (2014). Simultaneous fMRI and EEG during the multi-source interference task. PLoS One 9, e114599. 10.1371/ journal.pone.0114599. [PubMed: 25490131] 22. Sani I, Stemmann H, Caron B, Bullock D, Stemmler T, Fahle M, Pestilli F, and Freiwald WA (2021). The human endogenous attentional control network includes a ventro-temporal cortical node. Nat. Commun 12, 360. 10.1038/s41467-020-20583-5. [PubMed: 33452252] 23. Hanslmayr S, Pastötter B, Bäuml KH, Gruber S, Wimber M, and Klimesch W (2008). The electrophysiological dynamics of interference during the Stroop task. J. Cognit. Neurosci 20, 215– 225. 10.1162/jocn.2008.20020. [PubMed: 18275330] 24. Janssens C, De Loof E, Boehler CN, Pourtois G, and Verguts T (2018). Occipital alpha power reveals fast attentional inhibition of incongruent distractors. Psychophysiology 55, e13011. 10.1111/psyp.13011. 25. Caruana F, Uithol S, Cantalupo G, Sartori I, Lo Russo G, and Avanzini P (2014). How action selection can be embodied: intracranial gamma band recording shows response competition during the Eriksen flankers test. Front. Hum. Neurosci 8, 668. 10.3389/fnhum.2014.00668. [PubMed: 25206328] Cell Rep. Author manuscript; available in PMC 2023 March 08. Xiao et al. Page 21 Author Manuscript Author Manuscript Author Manuscript Author Manuscript 26. Koga S, Rothermel R, Juhász C, Nagasawa T, Sood S, and Asano E (2011). Electrocorticographic correlates of cognitive control in a Stroop task-intracranial recording in epileptic patients. Hum. Brain Mapp 32, 1580–1591. 10.1002/hbm.21129. [PubMed: 20845393] 27. Oehrn CR, Hanslmayr S, Fell J, Deuker L, Kremers NA, Do Lam AT, Elger CE, and Axmacher N (2014). Neural communication patterns underlying conflict detection, resolution, and adaptation. J. Neurosci 34, 10438–10452. 10.1523/JNEUROSCI.3099-13.2014. [PubMed: 25080602] 28. Sheth SA, Mian MK, Patel SR, Asaad WF, Williams ZM, Dougherty DD, Bush G, and Eskandar EN (2012). Human dorsal anterior cingulate cortex neurons mediate ongoing behavioural adaptation. Nature 488, 218–221. 10.1038/nature11239. [PubMed: 22722841] 29. Blackman RK, Crowe DA, DeNicola AL, Sakellaridi S, MacDonald AW 3rd, and Chafee MV (2016). Monkey prefrontal neurons reflect logical operations for cognitive control in a variant of the AX continuous performance task (AX-CPT). J. Neurosci 36, 4067–4079. 10.1523/ JNEUROSCI.3578-15.2016. [PubMed: 27053213] 30. Li YS, Nassar MR, Kable JW, and Gold JI (2019). Individual neurons in the cingulate cortex encode action monitoring, not selection, during adaptive decision-making. J. Neurosci 39, 6668– 6683. 10.1523/JNEUROSCI.0159-19.2019. [PubMed: 31217329] 31. Nakamura K, Roesch MR, and Olson CR (2005). Neuronal activity in macaque SEF and ACC during performance of tasks involving conflict. J. Neurophysiol 93, 884–908. 10.1152/ jn.00305.2004. [PubMed: 15295008] 32. Cole MW, Yeung N, Freiwald WA, and Botvinick M (2009). Cingulate cortex: diverging data from humans and monkeys. Trends Neurosci. 32, 566–574. 10.1016/j.tins.2009.07.001. [PubMed: 19781794] 33. MacLeod CM (1991). Half a century of research on the Stroop effect: an integrative review. Psychol. Bull 109, 163–203. 10.1037/0033-2909.109.2.163. [PubMed: 2034749] 34. Carter CS, and van Veen V (2007). Anterior cingulate cortex and conflict detection: an update of theory and data. Cognit. Affect Behav. Neurosci 7, 367–379. 10.3758/cabn.7.4.367. [PubMed: 18189010] 35. Gratton G, Cooper P, Fabiani M, Carter CS, and Karayanidis F (2018). Dynamics of cognitive control: theoretical bases, paradigms, and a view for the future. Psychophysiology 55. 10.1111/ psyp.13016. 36. Cavanagh JF, and Frank MJ (2014). Frontal theta as a mechanism for cognitive control. Trends Cognit. Sci 18, 414–421. 10.1016/j.tics.2014.04.012. [PubMed: 24835663] 37. Helfrich RF, and Knight RT (2016). Oscillatory dynamics of prefrontal cognitive control. Trends Cognit. Sci 20, 916–930. 10.1016/j.tics.2016.09.007. [PubMed: 27743685] 38. Widge AS, Heilbronner SR, and Hayden BY (2019). Prefrontal cortex and cognitive control: new insights from human electrophysiology. F1000Res 8. 10.12688/f1000research.20044.1. 39. Crone NE, Miglioretti DL, Gordon B, and Lesser RP (1998). Functional mapping of human sensorimotor cortex with electrocorticographic spectral analysis. II. Event-related synchronization in the gamma band. Brain 121 (Pt 12), 2301–2315. [PubMed: 9874481] 40. Liu H, Agam Y, Madsen JR, and Kreiman G (2009). Timing, timing, timing: fast decoding of object information from intracranial field potentials in human visual cortex. Neuron 62, 281–290. 10.1016/j.neuron.2009.02.025. [PubMed: 19409272] 41. Norman Y, Yeagle EM, Khuvis S, Harel M, Mehta AD, and Malach R (2019). Hippocampal sharp-wave ripples linked to visual episodic recollection in humans. Science 365, eaax1030. 10.1126/science.aax1030. [PubMed: 31416934] 42. Bansal AK, Singer JM, Anderson WS, Golby A, Madsen JR, and Kreiman G (2012). Temporal stability of visually selective responses in intracranial field potentials recorded from human occipital and temporal lobes. J. Neurophysiol 108, 3073–3086. 10.1152/jn.00458.2012. [PubMed: 22956795] 43. Gaetz W, Liu C, Zhu H, Bloy L, and Roberts TPL (2013). Evidence for a motor gamma-band network governing response interference. Neuroimage 74, 245–253. 10.1016/ j.neuroimage.2013.02.013. [PubMed: 23454050] Cell Rep. Author manuscript; available in PMC 2023 March 08. Xiao et al. Page 22 Author Manuscript Author Manuscript Author Manuscript Author Manuscript 44. Milham MP, and Banich MT (2005). Anterior cingulate cortex: an fMRI analysis of conflict specificity and functional differentiation. Hum. Brain Mapp 25, 328–335. 10.1002/hbm.20110. [PubMed: 15834861] 45. Parris BA, Wadsley MG, Hasshim N, Benattayallah A, Augustinova M, and Ferrand L (2019). An fMRI study of response and semantic conflict in the Stroop task. Front. Psychol 10, 2426. 10.3389/fpsyg.2019.02426. [PubMed: 31736827] 46. Egner T, and Hirsch J (2005). Cognitive control mechanisms resolve conflict through cortical amplification of task-relevant information. Nat. Neurosci 8, 1784–1790. 10.1038/nn1594. [PubMed: 16286928] 47. Menon V, and Uddin LQ (2010). Saliency, switching, attention and control: a network model of insula function. Brain Struct. Funct 214, 655–667. 10.1007/s00429-010-0262-0. [PubMed: 20512370] 48. Dosenbach NUF, Fair DA, Miezin FM, Cohen AL, Wenger KK, Dosenbach RAT, Fox MD, Snyder AZ, Vincent JL, Raichle ME, et al. (2007). Distinct brain networks for adaptive and stable task control in humans. Proc. Natl. Acad. Sci. USA 104, 11073–11078. 10.1073/pnas.0704320104. [PubMed: 17576922] 49. Dosenbach NUF, Visscher KM, Palmer ED, Miezin FM, Wenger KK, Kang HC, Burgund ED, Grimes AL, Schlaggar BL, and Petersen SE (2006). A core system for the implementation of task sets. Neuron 50, 799–812. 10.1016/j.neuron.2006.04.031. [PubMed: 16731517] 50. Marek S, and Dosenbach NUF (2018). The frontoparietal network: function, electrophysiology, and importance of individual precision mapping. Dialogues Clin. Neurosci 20, 133–140. [PubMed: 30250390] 51. Smith EH, Horga G, Yates MJ, Mikell CB, Banks GP, Pathak YJ, Schevon CA, McKhann GM 2nd, Hayden BY, Botvinick MM, and Sheth SA (2019). Widespread temporal coding of cognitive control in the human prefrontal cortex. Nat. Neurosci 22, 1883–1891. 10.1038/ s41593-019-0494-0. [PubMed: 31570859] 52. Ebitz RB, Smith EH, Horga G, Schevon CA, Yates MJ, McKhann GM, Botvinick MM, Sheth SA, and Hayden BY (2020). Human dorsal anterior cingulate neurons signal conflict by amplifying task-relevant information. Preprint at bioRxiv. 10.1101/2020.03.14.991745. 53. Rigotti M, Barak O, Warden MR, Wang XJ, Daw ND, Miller EK, and Fusi S (2013). The importance of mixed selectivity in complex cognitive tasks. Nature 497, 585–590. 10.1038/ nature12160. [PubMed: 23685452] 54. Fu Z, Beam D, Chung JM, Reed CM, Mamelak AN, Adolphs R, and Rutishauser U (2022). The geometry of domain-general performance monitoring in the human medial frontal cortex. Science 376, eabm9922. 10.1126/science.abm9922. [PubMed: 35511978] 55. Heilbronner SR, and Hayden BY (2016). Dorsal anterior cingulate cortex: a bottom-up view. Annu. Rev. Neurosci 39, 149–170. 10.1146/annurev-neuro-070815-013952. [PubMed: 27090954] 56. Groppe DM, Bickel S, Dykstra AR, Wang X, Mégevand P, Mercier MR, Lado FA, Mehta AD, and Honey CJ (2017). iELVis: an open source MATLAB toolbox for localizing and visualizing human intracranial electrode data. J. Neurosci. Methods 281, 40–48. 10.1016/j.jneumeth.2017.01.022. [PubMed: 28192130] 57. Dale AM, Fischl B, and Sereno MI (1999). Cortical surface-based analysis. I. Segmentation and surface reconstruction. Neuroimage 9, 179–194. [PubMed: 9931268] 58. Mitra P, and Bokil H (2008). Observed Brain Dynamics (Oxford University Press). 59. Fried I, Rutishauser U, Cerf M, and Kreiman G (2014). Single Neuron Studies of the Human Brain : Probing Cognition (The MIT Press). 60. Reuter M, Schmansky NJ, Rosas HD, and Fischl B (2012). Within-subject template estimation for unbiased longitudinal image analysis. Neuroimage 61, 1402–1418. 10.1016/ j.neuroimage.2012.02.084. [PubMed: 22430496] 61. Joshi A, Scheinost D, Okuda H, Belhachemi D, Murphy I, Staib LH, and Papademetris X (2011). Unified framework for development, deployment and robust testing of neuroimaging algorithms. Neuroinformatics 9, 69–84. 10.1007/s12021-010-9092-8. [PubMed: 21249532] 62. Desikan RS, Ségonne F, Fischl B, Quinn BT, Dickerson BC, Blacker D, Buckner RL, Dale AM, Maguire RP, Hyman BT, et al. (2006). An automated labeling system for subdividing the human Cell Rep. Author manuscript; available in PMC 2023 March 08. Xiao et al. Page 23 Author Manuscript cerebral cortex on MRI scans into gyral based regions of interest. Neuroimage 31, 968–980. [PubMed: 16530430] 63. Wu J, Ngo GH, Greve D, Li J, He T, Fischl B, Eickhoff SB, and Yeo BTT (2018). Accurate nonlinear mapping between MNI volumetric and FreeSurfer surface coordinate systems. Hum. Brain Mapp 39, 3793–3808. 10.1002/hbm.24213. [PubMed: 29770530] Author Manuscript Author Manuscript Author Manuscript Cell Rep. Author manuscript; available in PMC 2023 March 08. Xiao et al. Page 24 Author Manuscript Highlights Neural signals reveal incongruency during multiple cognitive control tasks The neural circuits that detect conflict generalize within a task The neural mechanisms underlying cognitive control are not task invariant Cognitive control relies on specific combinations of sensory inputs and motor outputs Author Manuscript Author Manuscript Author Manuscript Cell Rep. Author manuscript; available in PMC 2023 March 08. Xiao et al. Page 25 Author Manuscript Author Manuscript Author Manuscript Figure 1. Experimental paradigms Author Manuscript (A–C) Subjects performed the Stroop (A), Flanker (B), and Number (C) tasks in one session during intracranial neurophysiological recordings with depth electrodes. A standard session contained 18 blocks and each block comprised 30 trials of one task. (A) The Stroop task required subjects to say the font color. In the congruent condition, the semantic meaning coincided with the font color, while the two conflicted in the incongruent condition. (B) The Flanker task required subjects to press the left or the right key to indicate the direction of the central arrow. In the congruent condition, all the arrows pointed to the same direction while in incongruent condition, the arrow in the middle (target) pointed oppositely from the others (flankers or distractors). (C) The Number task required subjects to say the position (“one,” “two,” or “three”) where the unique number was located. In the congruent condition, the target number and its position were the same while in the incongruent condition these were different. All trials in this figure show incongruent conditions. Cell Rep. Author manuscript; available in PMC 2023 March 08. Xiao et al. Page 26 Author Manuscript Author Manuscript Author Manuscript Figure 2. Subjects were slower in incongruent trials in the three tasks (A–C) Violin plots showing distribution of reaction times for each subject for congruent trials (gray) and incongruent trials (red) during the Stroop (A), Flanker (B), and Number (C) tasks. Only correct trials are shown. Black bars indicate mean reaction times. The asterisks denote statistically significant differences (permutation test, 10,000 iterations, α = 0.05). Author Manuscript Cell Rep. Author manuscript; available in PMC 2023 March 08. Xiao et al. Page 27 Author Manuscript Author Manuscript Figure 3. Electrode locations (A–D) Each sphere reflects one of each pair of nearby electrodes that were bipolarly referenced (n = 694), overlaid on the Desikan Killiany Atlas with different views: (A) left lateral; (B) right lateral; (C) left medial; (D) right medial. Colors denote brain regions in the Desikan Killiany Atlas. Author Manuscript Author Manuscript Cell Rep. Author manuscript; available in PMC 2023 March 08. Xiao et al. Page 28 Author Manuscript Author Manuscript Author Manuscript Figure 4. Example electrode in the left orbitofrontal cortex showing conflict modulation in the high-gamma band during the Stroop task only Author Manuscript (A) The traces show the mean ± SEM Z-scored high-gamma power aligned to behavioral response time for incongruent trials (red) and congruent trials (black) for each of the three tasks (column 1, Stroop; column 2, Flanker; column 3, Number). The vertical dashed lines denote the average stimulus onsets. Yellow background indicates statistically significant power differences between congruent and incongruent trials (permutation test, 5,000 iterations, α = 0.05, STAR Methods). Legend shows the number of congruent (C) and incongruent (I) trials. The electrode location is shown on the right. (B and C) Raster plots showing the neural signals in individual trials (see color scale on the right) for congruent (B) and incongruent (C) trials. The white dashed lines show the average stimulus onsets. These lines are shifted to the left in (C) compared with (B), reflecting the longer reaction times during incongruent trials (see Figure 2). Gray and white bars on the left represent different blocks. (D) Z-scored high-gamma power (mean ± SEM) aligned to stimulus onset. Vertical dashed lines denote the average behavioral response times. Yellow background indicates statistically significant power difference between congruent and incongruent trials (permutation test, 5,000 iterations, α = 0.05, STAR Methods). Cell Rep. Author manuscript; available in PMC 2023 March 08. Xiao et al. Page 29 Author Manuscript Author Manuscript Figure 5. Example Flanker-specific (A) and Number-specific (B) electrode in the high-gamma band (A and B) The traces show mean ± SEM Z-scored high-gamma power aligned to behavioral response time for incongruent trials (red) and congruent trials (black) for each of the three tasks (column 1, Stroop; column 2, Flanker; column 3, Number). The vertical dashed lines denote the average stimulus onset. Yellow background indicates statistically significant differences between congruent and incongruent trials (permutation test, 5000 iterations, α = 0.05, STAR Methods). Legend indicates the number of congruent (C) and incongruent (I) trials. Electrode locations are shown on the right: (A) right superior parietal; (B) right precuneus). Author Manuscript Author Manuscript Cell Rep. Author manuscript; available in PMC 2023 March 08. Xiao et al. Page 30 Author Manuscript Author Manuscript Author Manuscript Figure 6. Neural signals showed within-task invariance Author Manuscript (A–S) Example task-specific electrodes demonstrating within-task invariance (Stroop (A–I), left inferior parietal; Flanker (J–M), right superior frontal; Number (N–S), right superior parietal). Z-scored high-gamma power (mean ± SEM) aligned to behavioral response time (black for congruent and red for incongruent). Vertical dashed lines denote the average stimulus onsets. Subplot titles indicate specific stimulus types. Conflict modulation occurred in all incongruent color/word (Stroop), target/flanker (Flanker), and target/distractor (Number) combinations. (T and U) Accuracy of support vector machine (SVM) classifier in discriminating incongruent from congruent trials extrapolating across conditions within each task (withintask invariance) using high-gamma (T) and theta (U) band power. Stroop task: the first bar labeled “RED” was trained using the “GREEN” trials (as in (D–F)) and “BLUE” trials (as in (G–I)), and tested on “RED” trials (as in (A–C)). A similar procedure was followed for the other combinations. In bar 2, the SVM was trained using “RED” and “BLUE” trials, and tested on “GREEN” trials. In bar 3, the SVM was trained using RED and GREEN trials, and tested on BLUE trials. Flanker task: in the fourth bar, the SVM was trained on “.” In the fifth bar, training and testing data were reversed. Number task: in the sixth bar, the SVM was trained on trials where the correct answer was “two” (as in (P and Q)) or “three” (as in (R and S)), and tested Cell Rep. Author manuscript; available in PMC 2023 March 08. Xiao et al. Page 31 Author Manuscript on trials where the correct answer was “one” (as in (N and O)). Similarly in bar 7, the SVM was trained on “one” and “three,” and tested on “two.” In bar 8, the SVM was trained on “one” and “two,” and tested on trials whose target answer was “three.” For each task, the training and testing data for each condition were randomly subsampled to contain an equal number of congruent and incongruent trials. Electrodes that had very few correct trials in any condition were removed from this analysis. Error bars indicate SEM over 50 sessions. The dashed line indicates chance performance (50%). Asterisks denote higher than chance accuracy (permutation test with 10,000 iterations, p < 0.001 for all bars). Author Manuscript Author Manuscript Author Manuscript Cell Rep. Author manuscript; available in PMC 2023 March 08. Xiao et al. Page 32 Author Manuscript Author Manuscript Figure 7. Task specificity in populationbased decoding of conflict in single trials Author Manuscript Author Manuscript (A and C) Accuracy of SVM classifier in congruent/incongruent discrimination when using a population of Stroop-specific electrodes (first three bars), Flanker-specific electrodes (next three bars), or Number-specific electrodes (last three bars). The SVM classifier was trained and tested with 10-fold cross-validation × 50 sessions of random sampling of trials using the high-gamma (A) and theta (C) band power data from the Stroop (red), Flanker (yellow), or Number (blue) tasks. Asterisks indicate that performances are significantly higher than chance (permutation test, 10,000 iterations, one-sided, p < 0.001, α = 0.05). (B and D) Cross-task training and testing using high-gamma (B) and theta (D) band power. Here, we used the same three populations from parts (A) and (C). The SVM classifier was trained on one task and tested on the other two tasks. The diagonal corresponds to training and testing within the same task and the off-diagonal entries show cross-task extrapolation. p values indicate the comparison between within-task and cross-task testing performances in each electrode population (permutation test with 10,000 iterations, one-sided). Accuracy is reflected by the color of each square (see color map on the right). Cell Rep. Author manuscript; available in PMC 2023 March 08. Xiao et al. Page 33 KEY RESOURCES TABLE Author Manuscript REAGENT or RESOURCE SOURCE IDENTIFIER Behavioral data for this study https://klab.tch.harvard.edu/resources/ XiaoEtAl_CognitiveControl.html https://doi.org/10.5281/zenodo.7317346 Neural data for this study https://klab.tch.harvard.edu/resources/ XiaoEtAl_CognitiveControl.html https://doi.org/10.5281/zenodo.7317346 Code used in this study https://klab.tch.harvard.edu/resources/ XiaoEtAl_CognitiveControl.html https://doi.org/10.5281/zenodo.7317346 MATLAB R2016b The MathWorks, Inc., Natick, MA https://www.mathworks.com/ Intracranial Electrode Visualization (iELVis) Toolbox (Groppe et al., 2017)56 https://github.com/iELVis/iELVis Freesurfer 6 (Dale et al., 1999)57 https://surfer.nmr.mgh.harvard.edu/ Chronux Toolbox (Mitra and Bokil, 2008)58 https://www.chronux.org Deposited data Software and algorithms Author Manuscript Author Manuscript Author Manuscript Cell Rep. Author manuscript; available in PMC 2023 March 08.