Adda Comprehensive Detail Notes - WD Practice Questions PDF
Document Details
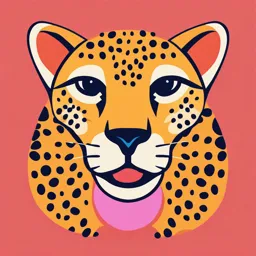
Uploaded by JudiciousNephrite2042
Tags
Summary
This document provides lecture notes and practice questions on psychological research and data analysis. It covers topics like research designs, data analysis techniques (e.g., SPSS), and issues like the file-drawer problem and replication crisis within psychology.
Full Transcript
LECTURE 1: PSYCHOLOGICAL RESEARCH AND DATA ANALYSIS A review of statistical inference issues What is the file-drawer problem? Refers to the bias introduced into the scientific literature by selective publication - chiefly by a tendency to publish positive results but not to publish negative or nonco...
LECTURE 1: PSYCHOLOGICAL RESEARCH AND DATA ANALYSIS A review of statistical inference issues What is the file-drawer problem? Refers to the bias introduced into the scientific literature by selective publication - chiefly by a tendency to publish positive results but not to publish negative or nonconfirmatory results. Why is the replication crisis centred on psychology? Gelman (2016) mentions five reasons: 1. Sophistication. Unlike other scientific fields psychology focuses very much on the concepts of validity and reliability and did so before many other fields of study. Because the focus on these key concepts was developed early in psychology it made the field more open to criticism than other fields. 2. Openness. Unlike other fields the psychology one is an open one where the sharing of data is common and therefore it is easier to find mistakes. 3. Overconfidence deriving from research designs. Clean designs lead to overconfidence. 4. Involvement of some prominent academics. Few leading findings and academics dragged into replication crisis therefore whole field is taken with them. 5. The general interest of psychology. More open to the public and there interests. Some routes to replication issues 1. Outright fraud (rare) 2. P-hacking, data dredging, data snooping, fishing expeditions (likely rarer than is commonly believed) This involves an individual going hunting for the smallest p-value in a data set then publishing results from this (p-hacking) 3. "The garden of forking paths" (likely more common than is generally realised) Idea that if one where to find different data you would then analyse it in a different form. E.g. It is well known publishing that there is a correlation between eating breakfast and school performance. You, as a researcher, performed this study however you found no significant relationship for this hypothesis. However, you did find one for maths. You then go on to publish an article about the relationship between eating breakfast and maths performance. ▪ This is overcome by preregistering hypotheses QUESTIONS LECTURE 1 1. What is meant by the 'file-drawer problem'? 2. Why is the replication crisis centred on psychology? 3. What is P-hacking? 4. Describe the term "the garden of forking paths". 5. How would you describe the taxonomy of routes to the current replication issues present in psychology? 6. Give one example of how we can overcome replication issues? 1 LECTURE 2: RESEARCH DESIGN AND MANAGING DATA GOALS OF THIS LECTURE To review basic psychological research designs To give you some preliminary information about models and the structure of data To explain some basic steps in data analysis using SPSS: o Entering data o Missing data o Transforming data o Computing scale totals Section 1: Research designs in psychology Varieties of psychological research Basic vs applied research Basic: explaining the fundamental principles of behaviour and processes e.g., Sherry Shadow Tasks. Leads to the development of models of behaviour. Considered more glamorous than applied. Applied: has some relevance to a real world issue. E.g. Driving tests while using a mobile. Laboratory (control) vs Field research (realism) Laboratory: greater control over variable and specify the conditions of the study. Field: realism *all begin with an empirical research question that can only be answered with statistical data analyses. Quantitative vs qualitative research Quantitative: the measurement consist of unordered or ordered (ranked) discrete categories e.g., religious backgrounds Quantitative: assumed to have underlying continuity. E.g. Height, temperature Empirical research questions Developing research From observations, Theory, And past research Experimental psychological research What are the essential features of experimental research? The researcher holds some factors constant, various others (independent variables) and observes the outcomes on another variable of interest (dependent variable) Experimental vs control groups What are typical methods of analysis? What are between-subject designs? Different participants give data for each variable. However, the difference seen may be the result of the inherent differences between the groups rather than manipulation by experimenter. How might you obtain equivalent groups for between-subjects designs? o Can be overcome with the use of a random design. Where participants are randomly assigned to groups. o Also achieved through participant matching on X variable. What are within-subjects designs? Each participant is exposed to each level of the variable e.g., completes a memory task after taking all doze levels of a particular medication. How might you control for sequencing effects? o Counterbalancing: each possible order applied to each individual participant. E.g. Take highest to lowest dose or reverse. Or a random assignment of doses. Single factor designs 2 One independent variable o Two or more levels Factorial designs Two, possibly more, factors (independent variables) Looks at main effects and interactions between factors o E., two way ANOVA Correlational research Correlation: an association between two (usually continuous) variables Regression: predicting a variable from other variables in a regression model Such designs are helpful when: Experiments cannot be carried out for practical or ethical reasons Ecological validity: generalisation in to the broader environment Applied research and Quasi-experimental designs The goal of applied research is to investigate real world problems Quasi-experimental: o Groups occur naturally in the world; there cannot be random assignment to groups ▪ E.g. Compares mean scores of men and women on some task Program evaluation: o Provides empirical data about effectiveness of government and other programs. Some important issues: Causality Be careful with claims like X causes Y, or X leads to Y. Casual claims might be possible with careful experimental design Or maybe with carefully designed longitudinal studies In particular, beware of conflating correlation with causation. Some important issues: Null hypothesis significance testing Some additional issues to those mentioned before o Large sample size = high statistical power If power is low, then there may be a difference but you don't see it = Type 2 error If power is really high, you will find even very small differences as significant = Type 1 error o Statistical power: the probability that a given test will find an effect assuming that one exists in the population. The opposite of the probability that a given test will NOT find an effect assuming that one exists in the population. E.g. Cohen recommends a.2 probability of failing to detect a genuine effect therefore, we aim to achieve a power of.8, and 80% chance of detecting an effect if one genuinely exists. Report effect sizes, and use confidence intervals, whenever you can, along with p values. Effect size: an objective and standardized measure of the magnitude of the observed effected. o E.g. Cohen's d or the correlation coefficient (Pearson correlation r) Depending on your supervisor you might use Bayesian methods. Section 2: Data and Models Multivariate Models 'multivariate' usually means multiple dependent variables o Sometimes, it just means there are more than one variable The multivariate models we ill fit to data: o Either seek to test the prediction of some variable from other variables ▪ Confirmatory (hypothesis testing) approach o Or they may be models that simply seek to account for the relationships between variables 3 ▪ Exploratory (hypothesis finding) approach Fitting Models to Data Both of these aims have a common simple conceptual model: DATA = MODEL + RESIDUAL (error) A simple example: 4 boys, 4 WISC subscale scores Each individual boy scores a variety of scores on each subset. As we never expect the model to fit the data perfectly, we have residual. A model is interesting when it doesn't fit perfectly: we want a model to be parsimonious and explain as much variation in the data as possible using the simplest structure. Different models handle residuals in different ways o If you use the mean of each person on different subsets as a model for their overall performance, you assume that each of the items are weighted equally in influencing the overall outcome ▪ In which case the residual account for random noise, measurement error, etc RESIDUAL = DATA - MODEL The residual gives us an indication of how well the model FITS the data. These 'differences' are then squared. This sum of squares is a standardised measure of how well the model fits. o If residual scores some to be very large, this is evidence that the model is wrong and does not fit the data well. Assessing the fit of a model Examine the residual o These provide a direct indication of the discrepancy between model and data Use a summary measure (such as percentage of variance accounted for) o Provides an overall indication of the variance accounted for by the model Use a statistical test o When a model is a null hypothesis, if the residual is too large (p.05), the data could have a plausibly arisen from such a model. The model FITS the data. Ways of representing models Regression model Here the model basically says that the outcome variable Y is a weighted average of the predictor variable X1 and X2 (with e being the residual) o Statistical assumption of the residual: these are normally distributed with a mean of 0 and are independent of each other The 'weights' are the regression coefficients b0, b1 and b2 - the parameters of the model It can be convenient to represent multivariate models in matrix form = Y = Xb + e Regression model is therefore a line in a 6 dimensional space, as you have 6 variables. What is a matrix? Regular array of numbers in a data spread. E.g. The variables for the BIG 5 are a matrix (with n rows and five columns) Variables in models Independent (predictor) vs dependent (outcome) variables 4 Discrete vs continuous variables o Discrete can be categorical (unordered) or ordinal (ordered) Exogenous vs endogenous variables o Exogenous are determined outside of the model (not predicted within the model - e.g. Independent variables) o Endogenous variables are determined within the model (e.g., dependent variables) Levels of measurement Nominal (categorical): made up of categories. The only way that nominal data can be used is to consider frequencies Ordinal: when categories are ordered. Tells us nothing about the difference between values. Has a logical order to it. Interval: equal intervals on the variable represent equal differences in the property being measured. Ratio: the ratios of values along the scale are meaningful e.g. A score of 16 on anxiety means an individual is twice as anxious as someone who scores 8. Some models require variables ate certain levels: ANOVA or independent t-test: the factors must be discrete Section 3: Handling Data Missing data Missing completely at random (MCAR) : missingness is not related to any other variable. This is the most ideal form of missingness. Missingness here is not systematic and related to variables in the analysis. Statistical test of this is Little's test: null hypothesis that the missingness in the data is completely at random. DO NOT want this to be significant! Establishment of missingness completely at random is a justification for performing the analysis, even though you have missing data. Missing at random (MAR) - missingness is related to another variable, but there is no pattern within the variable. E.g. Depressed people might be less inclined to report income, so reported income is related to depression (missingness in not MCAR) But suppose that within depressed people, the probability of reporting income is unrelated to income level. Then the data is considered MAR. Ignorability: if the missingness in MCAR or MAR, then it is ignorable. If it is not ignorable, then you have a serious problem. Make sure your research design enables good measurement of all variables. 5 Solutions to ignorable missing data First step: determine the extent of the problem Delete cases: o Listwise deletion: ignore any case with any missing data. Loss of power. o Pairwise deletion: remove pairs of cases relevant to an analysis (e.g. Correlation) Estimate missing values o Using the mean of the mean of the variable - often criticised o Missing value analysis in SPSS - regression and EM algorithm o Multiple imputation procedure in SPSS - generates possible values, creating several "complete" sets of data. Analytic procedures that work with multiple imputation datasets produce output for each 'complete' data set, plus pooled output ▪ The preceding methods use data from other participants to estimate missing data. But when a participant misses some items in a scale but responds to others, the data from just that participant should be used to estimate the missing data. Transformations Variables can be recoded if this make theoretical sense Sometimes we need to transform our data fro model related purposes. As the model we consider are all linear in one way or another, we may wish to transform the scale on which our variable is measured in order to: o Optimize the linear relations in our model o Or to ensure that our data conform to the assumptions demanded by the statistical tests associated with the particular model (normality distributions) We transform the variables to change the symmetry of the distribution Totals There are several advantages in putting item level data into the computer and computing totals later: Estimate the reliability of the test scores Check the validity of any subscales And if necessary develop new subscales Form empirically weighted totals Drop 'dud' items and cope with missing data Reliability coefficients 6 Coefficient alpha (Cronbach's alpha) is the most common but not the best - this is an internal consistency coefficient All reliability estimates are pessimistic: they are lower bound estimates. The best estimate of true reliability is the greatest of the lower bound estimates. Guttman coefficients allow this choice - provides many difference coefficients: choose the greatest of these. SYNTAX E.g. 0001 19 F 164978237855 5.6 0002 23 M 174527211184 9.2 -< ID, age, sex, responses on 12 item questionnaire, average learning score QUESTIONS LECTURE 2 1. Describe the key differences between the following terms: basic vs applied research; laboratory vs field research; and quantitative vs qualitative research. Give one example for each 2. What are between-subject designs? 3. What are within-subject designs? 4. How does a single factor design differ from factorial designs? 5. How does correlation differ from regression? 6. What are some important issues with causality? 7. Describe three issues of NHST? 8. What is the difference between type 1 and type 2 errors? 9. Define statistical power. 10. Define effect size. Give two examples. 11. Describe the confirmatory and exploratory approaches of multivariate models. 12. What is the common simple conceptual model? What does each term mean? 13. How to we assess the fit of a data model? 14. What does parsimonious mean? 15. Write the equation for a regression model. 16. Write the matric form of a regression model. 17. What is a matrix array? 18. What are the six types of variables that can be present in a model? 19. How does an exogenous variable differ from an endogenous variable? Give an example for each. 20. Describe the level of variable measurement giving an example for each level. 21. Describe MCAR. 22. What test can we use to establish MCAR? What does it mean if this is significant? 23. Describe MAR. Give an example. 24. What are the steps taken if missing data is found not to be ignorable? 25. Describe listwise and pairwise deletion. 26. Describe three means of estimating missing values in data. 27. Why would a research decide to transform variable data? 28. What effect does transforming data have? 29. Give four examples of how we can transform ‘x’ and the corresponding change to the variable. 30. Describe some key advantages of putting item level data in to SPSS rather than totals. 31. What is the best reliability coefficient to estimate reliability? 7 32. What is meant by “all reliability measures are pessimistic”? 33. Interpret the syntax: 0002 23 M 174527211184 9.2. 8 LECTURE 3: REGRESSION, MEDIATION AND MODERATION REGRESSION Theory of error In regression and statistics in general, we should preserve the notion that there is some truth out there. We observe something, and hopefully that which we observe reflects the truth. o There is always some error in our observations. We want to be able to separate the truth/signal, for the error/noise. The science of psychology requires a theory of error to find the truth o E.g. Gaussian distribution/ normal distribution OBSERVATION = TRUTH + ERROR/ THEORY + ERROR Variables Suppose we've got variables X, Y, Z, etc. We might combine them in some way. Perhaps we could add them up: X+Y+Z+… But then maybe they are not all equally important, so perhaps a weighted sum? aX + bY + cZ + … the weighted sum of coefficients is the basis of the general linear model in regression A Model theory: intelligence increases with age. Suppose everyone is born with an IQ of 90 and IQ increases by ½ point for every year of age. We can then write a model: IQ = 90 + 0.5xAGE. This is an example of a general linear model – adding up of variables by a weighted sum = regression model Problem: no variation. Not everyone is born with 90 IQ, nor is everyone IQ to increase at same rate. Correction mode: IQ = 90 + 0.5(AGE) + (error) The error term covers all other causes, measurement errors, individual differences If the error is random in the sample, we expect that it will cancel out across all subjects in the sample. This is because we assume that error is normally distributed with a mean of zero Theory of error: It is the error term that requires statistical analyses The real question: the relationship between age and IQ – is not inherently statistic Residuals are estimates of error ▪ Residuals are errors that relate to our data/sample, errors are at the population level Regression to predict positive affect To predict positive affect from the Big 5 in regression, we need six regression coefficients: This model represents a straight line in 6-dimensional space Statistical assumptions: the residual are normally distributed with a mean of zero and are independent of each other 9 Randomised control trials = careful experimental design helps with the important assurance that the residuals are independent of each other R squared is the variation explained by the regression model. Variation in the DV that is explained by the IVs – an index of how accurate the model is compared to the noise Significant ANOVA result – the R square statistics is significant. Partial coefficients – indicate the independent effect of each IV on the DV, while taking into the account the effects of other variables in the model Listwise vs pairwise deletion of cases – if you get the same inference from both, it doesn’t matter which deletion method you use. Statistical plots to check assumptions – as long as there is no systematic departure from normality in the histogram, no obvious patterning in the PP plot, and no patterning in the scatterplot, then the assumptions are reasonably being met. But why Linear Models? You’ve often modelled the mean and variance of some outcome variable(s) as an additive combination of other variable(s). Lots of advantages to these models. They: Are easy to fit. Are commonly used. Have lots of practical applications (prediction, description, etc) Provide a descriptive model that is very flexible (corresponds to lots of possible underlying processes) Have assumptions are often broadly reasonable The family 10 Modelling Predictor(s) Outcome(s) Normal errors assumed? ANOVA 1+ categorical 1 continuous Yes ANOVA 1 categorical 1 continuous Yes 2 categorical 1 continuous Yes 2+ continuous and/or categorical 1 continuous Yes Technique [One-Way ANOVA] ANOVA [Two-Way ANOVA] Multiple regression (min 1 continuous) Simple regression 1 continuous 1 continuous Yes t-test 1 categorical (max 2 levels) 1 continuous Yes [Student’s t-test] All fundamentally the same kind of model, but terminology varies widely But why normal errors? Two broad justification for building models around the assumption of normal errors: Ontological justification – they occur naturally in the environment e.g. distribution of height. Epistemological justification – normal distribution relates to a state of knowledge, and it is better to go with what you know! Five assumptions of our model Validity – relevance of measure to the phenomenon you are trying to analyse. Is the sample representative of the population etc. Additivity and linearity – non-error to be a linear production of the model Independence of errors – model assumes that errors are independent. This however, can be violated! Equal variance of errors – also known as homogeneity of variance, heteroscedasticity Normality of errors MEDIATION EFFECTS 11 What is mediation? Mediation is important in many psychological studies. It is the process whereby one variable acts on another through an intervening (or mediating) variable. When one variable intervenes between two others, X affects Y. but X only affects Y by affecting another variable M, in between. EG. Theory of reasoned action: attitudes leads to intentions which leads to behaviour. Simplest mediation model: Independent variable X, mediating variable M, dependent variable Y X -> M -> Y Mediation regression equations – conceptualise the mediation effect in terms of regression modelling Predictor X, outcome Y, mediator M Equation 1: X predicts Y, with an error term explaining deviation from model. C is the strength of the effect – regression coefficient. You need this relationship, because if X does not predict Y, there is no relationship to mediate. (THIS CAN BE CONCEPTUALLY INCORRECT) Equation 2: X must predict M, because this logically needs to hold it X is to effect Y through M. Equation 3: the new regression coefficient for X -> Y = c’. ▪ If this is small, but not zero, you have a partial mediation, as the mediating variable does not fully explain the relationship between X and Y ▪ If this is zero, you have full mediation (WHAT YOU WANT), because there is no left over direct relationship between X and Y once the mediating variables is considered ▪ If c’ is the same as c, you have no mediation effect at all In terms of the TRA, full mediation would results in the following: attitudes predict intentions, which predict behaviour with no direct effect of attitude on behaviour. Baron & Kenny (1986) – The casual steps approach 12 For mediation effect to be present, there exists 4 requirements: The IV directly predicts the DV (coefficient c is significant) The IV directly predicts the MV (coefficient a is significant) The MV directly predicts the DV (coefficient b is significant) CRUCIAL When both the IV and MV predict the DV, the effect of the IV is either: Significantly reduced (coefficient c’ is significantly smaller than c), and there is partial medication; or Eliminated (coefficient c’ is not significant) and there is full mediation Types of effects: The direct effect of IV on DV is c’ Indirect effect of IV on DV via MV is axb The total effect of the IV on the DV is the sum: c’ + a x b Suppose the direct effect c’ is not significant, but the indirect effect axb is significant: an indirect effect of IV on DV, but no direct effect. This is not mediation unless c is also significant. This is distinguishable step for the Baron & Kenny approach C also represents the total effect of IV on DV, so c = c’ + a x b. Or rearranging a x b = c – c’. For mediation to be present, the total effect has to be significant. Testing the significance of the indirect effect a x b is equivalent to testing whether mediation occurs Ways of testing a.b. Sobel test – tests the null hypothesis that the population indirect effect equal zero. Ho: (a x b) = 0 If p 1.0 ▪ This intuitively means that any retained factor should account for at least as much variation as any of the original variables. Each observed variable contributes one unit of variance to the total variance. If the eigenvalue is greater than 1, then each principal component explains at least as much variance as 1 observed variable ▪ It tends to choose one third of the variables as the number of components 2. The Scree Plot o Good for graphically conceptualising the idea of signal and noise o The Scree Plot graphs the number of components (x) against the eigenvalues (y). At some point the plot turns, and this is the point at which the eigenvalues for your components diminish, and at this point is where you should stop extracting components o Obtain the Scree Plot under the 'extraction' option in SPSS. From these different tests, you will obtain a number of different criteria for selecting the different components, and you need to make an assessment about how many to extract. 21 3. The Parallel Test This test enables you to move beyond a superficial examination of the Scree plot. This test generates random data of the same dimensions - this is definitely noise. You can then look at the eigenvalues for your dataset compared to the random data, and if they are larger, then this represents signal in direct comparison to noise Where the Scree Plot (red line) is above the 95th percentile (blue line) you can be sure this is significant signal This is beneficial as it removes the subjectivity of simply looking at the Scree plot, and gives you a specific decision point It is not in SPSS, but you can use syntax script to run the parallel test OUTPUT From this, you extract components where your data (raw data column) is greater than the 95th percentile of the random data (pctile column). 2 is the last component where the eigenvalue for our observed data is greater than the random noise, so you select 2 components 22 3. The MAP Test Based on partial correlations known as Minimum Average Partial Correlation test After each component is extracted, it and those extracted before it are partialled out of the correlation matrix of original variables, and the average of the resulting partial correlations calculated As more components are partialled out, the resulting partial correlations would approach zero But at some point components that reflect noise would be partialled out, and the average partial correlation would begin to rise. Based on this, you choose the number of components corresponding to the minimum average partial correlation You use syntax for the MAP test as well. OUTPUT You can see from the middle column that the average partial correlation decreases, and then increases once the noise has been partialled out. Choose the number of components at the minimum point of this value. Here, the map test and the parallel test agree on 2 principal components, so you can have confidence that 2 is a good number 23 If there was a discrepancy - make a decision. Here you can run both and see if you gain differing interpretations, or refer to Schmitt (2011) and say that the parallel test is more accurate and go with that, or see what is the most interpretable option etc. ▪ FACTOR LOADING: correlation between the original variables and the factors, and the key to understanding the nature of a particular factor. Squared factor loadings indicate what percentage of the variance in an original variable is explained by the factor Component/ Factor Loading Now that we now the number of factors to be extracted is 2, you can specify this under "extraction" on SPSS This gives you a component matrix, with various different loadings for the different variables within each component Can see the weight by component - each item with a greater weight is more important than those with a lesser weight, in the given component Signs of the weight - this is all standardized, so if you get a high score on a positive weighted item, then you get a higher score on component 1. if you get a higher score on a negatively weighted item, your score on component 1 decreases. This is still fairly complicated with loadings for all 20 items on the individual components. You want to work out what component 1 actually means. We need another step to make this simpler - rotate again. Component rotation: simplifying interpretation With n variables, you can have n components - these are completely determined and follow directly from the matrix operations But if we only use a smaller number of components, then there is some freedom in the final solution o In particular we can rotate components to get a simpler structure o With large component loadings for some variables, and small component loadings for others On SPSS you can do this under the 'rotations' option. Varimax is an orthogonal rotation method - keeps the axes at right angles and does not allow correlation between the components. Some rotation methods allow correlation, others don't This provides a "rotated components matrix" in the output - increases the larger loading, and decreases the smaller loadings to aid interpretation. To aid this further, you can ask SPSS to remove small coefficients. o These are still included in the analysis, they are just not reported in the output FACTOR ROTATION: process of manipulating or adjusting the factor axes to achieve a simpler and pragmatically more meaningful factor solution Component interpretation You look at the loadings greater than 0.6, with reference to the original items in the question, and derive what the commonality among all the items might be which represents the component variable o THIS IS A UNIQUE WAY OF ADDING UP THE DIFFERENT VARIABLES WITHIN EACH COMPONENT, WITH DERIVED WEIGHTS THAT AID INTERPRETATION OF TEH DATA Types of rotation Orthogonal rotation - components/ factors DO NOT correlate. Components are at right angles to one another. With an orthogonal rotation, loadings are equivalent to correlations between observed variables and components ▪ ORTHOGONAL FACTOR ROTATION: factor rotation in which the factors are extracted so that their axes are maintained at 90 degrees. Each factor is independent of, or orthogonal to, all other factors. The correlation between the factors is determined to be 0. 24 Oblique rotation - components/factors DO correlate. Components converge on one another in the graph. With oblique rotations you always get a correlation matrix in SPSS as well which tells you the extent to which your factors correlate - and a pattern matrix and structure matrix. Focus on the PATTERN MATRIX. The structure matrix is a product of the pattern matrix and the factor correlation matrix. But you use the pattern matrix for interpretations. ▪ OBLIGUE FACTOR ROTATION: factor rotation computed so that the extracted factors are correlated. Rather than arbitrarily constraining the factor rotation to an orthogonal solution, the oblique rotation identifies the extent to which each of the factors is correlated. Schmitt - strongly recommends oblique rotation methods as in practice components usually correlate ("results in more realistic and more statistically sound structures" ) In practice - try both rotation methods. If the correlation between the components is quite small, revert back to orthogonal rotations EXPLORATORY FACTOR ANALYSIS Factor analysis is a technique to search for such underlying factors - a method related to measurement. Factor analysis assumes these latent constructs exist - you need a theoretical argument to justify what construct might exist. Particularly useful when you want to estimate underlying factors or constructs that cause the associations among your variables, which cannot be measured directly. 25 The common factor model We have the common factor, which each individual measure relates to, and the specific factor for each measure, which explains variation form the common factor (the error term) We have observed variables, and assume there are k common factors that explain the observations on these variables Each variable represents the product of the common factor, the degree to which the variable relates to the common factor, with the error added in Assumptions of this EXPLORATORY factor analysis (these differ for confirmatory factor analysis): o Common factors are standardised (variance = 1) o Common factors are uncorrelated o Specific factors are uncorrelated o Common factors are uncorrelated with specific factors The common factor model uses structural equation modelling interpretations Underlying rationale: partial correlations This technique uses the correlations between items, given the influence of the explanatory factor Suppose a correlation of 0.615 between items on an extraversion scale: o 1 = don't mind being the centre of attention o 2 = feel comfortable around people o Correlation of 0.82 between item 1 and extraversion o Correlation of 0.75 between item 2 and extraversion 26 The goal of factor analysis therefore, is to find a latent variable which will account for observed correlation - find the point at which the partial correlations are zero. We will try and find a model (which PCA does not do, this is simple mathematics), which best captures the covariance matrix, and renders the partial correlations zero The aim is to find a latent or unobserved variable, which then correlated with out observed variables, leads to partial correlations between observed variables that are as close to zero as possible If each variable is to vary, it can only vary by the extent to which it relates to the latent factor, or the specific factor. Conceptualised below: We want high communality - this is the variation due to the common factor TECHNICAL ISSUES WITH RUNNING EFA Sample size - research has attempted to provide rules of thumb for sample size to ensure beneficial results Absolute sample size and communalities (size of factor loadings) are the most important thing If there are conditions were the component loadings are high (above 0.60), and there were four or more variables per component, the sample size could be as low as 50. Generally though, you would want around 150 participants for this to work. Costello & Osborne state the ideally EFA should have: High communalities for each itme (>0.8 would be excellent, but 0.4-0.7 is more common). If an item has communality lower than 0.4, it could be removed, as it doesn't fit with other items. But don't remove items too liberally Few cross loadings - not many items that load more than 0.32 on more than one factor. In reality, you will have items that cross load. You can tolerate some cross-loading, but you wouldn't have them in a deal dataset More than three strongly loading items per factor o If these conditions do not apply, a bigger sample size could help alleviate this. Communality Communalities are important, but these are only known after finding factor loadings. Thus, there are various different diagnostics you can use to whether your covariance matrix will show communality prior to finding factor loadings: o Low correlations leads to low factor loadings o Barlett's test of whether correlations = 0. want to reject this o Anti-image correlation matrix o Kaiser's measure of sampling adequacy COMMUNALITY: total amount of variance an original variable shares with all other variables included in the analysis 27 ANTI-IMAGE CORRELATION: matrix of the partial correlations among variables after factor analysis, representing the degree to which the factors explain each other in the results. The diagonal contains the measures of sampling adequacy (MSA) for each variable, and the off-diagonal values are partial correlations among variables. MEASURE OF SAMPLING ADEQUANCY (MSA): measure calculated both for the entire correlation matrix and each individual variable evaluating the appropriateness of applying factor analysis. Values above.50 for either the entire matrix or an individual variable indicate appropriateness. Guttman - Kaiser Image Approach Image analysis involves partitioning of the variance of an observed variable into common and unique parts: Produce correlations due to common parts - image correlations Produce correlations due to unique parts - anti image correlations. Want these to be NEAR_ZERO In the table of output in SPSS - in the diagonal of the anti-image correlations, you want big numbers. Diagonal is the MSA value for the variable (want close to 1), and the off-diagonal is the anti-image correlations (want close to 0). This tells you that the correlations/covariances in the data should be factorisable. Good to report this: anti-image correlations were close to zero.... None were greater than... The diagonal smallest was 0.8. ▪ BATLETT TEST OF SPHERICITY: statistical test for the overall significance of all correlations within a correlation matrix Kaiser's measure of sampling adequacy The higher the value the better The sampling adequacy statistic here is very high - this is a good result. Bartlett's test - you want this to be significant and it usually is. It is good to report this, but it is better to understand and report the KMO with reference to the anti-image correlation matrix. These diagnostic techniques give you a sense of weather you can factorise your variables SUMMARY Bartlett test acceptable (as always) Overall MSA value is.901 Low anti-image correlations Reasonably high MSA values Conclusion: this should be OK for factor analysis 28 Methods for finding factors Two SPSS methods for EFA: 1. Maximum likelihood (ML), and 2. Principal axis factoring (PA) Try different methods - if you obtain consistent results, this is good The maximum likelihood method has a test of fit. Statistical interpretation of the fit statistics requires the data to be multivariate normal, but factor loading can always be calculated whether the data is normal or not Heywood cases.999 in factor loadings This is a technical problem - you have probably extracted too many factors. This means the computer has inserted a correction because the loading is 1.000 - there is no unique variance SUGGESTED STEPS IN EFA Check your data by examining the MSA values Find the most likely number of factors, using one of the better rules such as the parallel test. Where there is some doubt, find solutions for your best estimate and one more and one less numbers of factors Use a common factor method when you want to interpret the factors. If you just want a weighted sum of variables use principal components. Try oblique rotation first. If there are no correlations between the factors consider using a simpler orthogonal rotation Use un-rotated factor matrix to look for Heywood cases Increase the number of iterations when doing rotation [change 25 to 250] For easier interpretation on the 'option' submenu check - sorted by size, and suppress absolute values less than [say 0.32] Factor scores Having identified factors, we can estimate factor scores for each case o One option - form sum of scores for each item that load on the factor. This assumes equal weights for each item (tau-equivalent test). If this is not true, alpha is a serious underestimate o Another option - with the assumption of varying factor loadings - known as congeneric tests. ▪ SPSS provide three ways of doing this: regression is the default; Bartlett method (recommended); and Anderson-Ruben (misleading for oblique solutions as this assumes uncorrelated scores) In practice, the method you use may not matter much COMPARING PCA AND EFA Principal components analysis: o You do not claim that there is some underlying construct that you are measuring. There are simply themes in the data that you extract. o Works with observed variables o Components are weighted composites of the observed variables, so are also observed variables o If a variable is added to or removed from the analysis, the component may change o If another component is added or removed, the other component loadings, do not change Exploratory factor analysis o You can claim that you are investigating an underlying factor that cases correlations among your variables o Factors are latent variables - superordinate to the observed variables: they causes the observed variables to correlate 29 o o If an observed variable is asses to or removed from the analysis, the others should not change If another factor is added or removed, then the factor loadings of the others will change Why the difference? This is an issue of the diagonal elements of the correlational matrix o In components analysis, the value of 1.0 is used, and the aim is to explain all the variance of the variable o In factor analysis, the diagonal element is the communality, and the aims is to explain only the common variance of an element Comparing EFA and PCA for the data in the example - the interpretation is similar Using PCA over EFA - perhaps factor analysis will not work for technical collapsing observed variables. If you cannot get a factor analysis, report this, and say that you will run a PCA instead. If you are only looking for themes in the data, then PCA is acceptable. But where you measure correlations with the idea that these reflect some underlying or latent variable, you should run an EFA. Run FA if you assume or wish to test a theoretical model of latent factors causing observed variables. Run PCA if you want to simply reduce your correlated observed variables to a smaller set of important uncorrelated composite variable QUESTIONS LECTURE 4 1. 2. 3. 4. 5. 6. 7. 8. 9. 10. 11. 12. 13. 14. 15. 16. 17. 18. 19. 20. 21. 22. 23. 24. 25. 26. 27. Describe the terms component, factor and latent variable What is principle component analysis? What does the first principal component tell us? How does this differ from the second principal component? How many principal components could a given data set have? Describe the covariance matrix in a principal component. How does this change after we 'flip' the principal component? How do we determine the 'fit' of a principal component? What is the eigenvector? What is an eigenvalue? Where is it derived from? What does it mean if the spread of values in large along the principal component? Describe the algebraic interpretation of PCA. What is the one key difference between the PCA linear equation and the FA equation? Why is PCA a mathematical technique and not a statistical one? How does the equation for the first principal component differ from the second? How are they the same? What are the four methods we can use to determine how many components we should extract from a data set? What are the parameters of the Kaiser-Guttman rule? Is this an effective means of extraction? Why, why not? Describe a Scree plot. Which extraction method is the most sound, parallel test or MAPS? Give a reason. In a parallel test how do you determine the number of factors? Describe MAPS. How do we perform the analysis? Why do we perform component rotation? What are the two forms of rotation? Give an example for each. How does orthogonal differ from oblique rotation? How to you choose which form of rotation is the best for your data? How do we interpret the output from a rotational analysis for both types of rotation? What is the difference between the strucural matrix and the pattern matrix? Describe the term 'simple structure'. What is factor modelling (EFA)? What is the goal? Write the statistical equation for EFA. 30 28. 29. 30. 31. 32. 33. 34. 35. 36. 37. 38. 39. 40. 41. 42. 43. 44. Draw a simple common factor model, labelling all the key parts and what it means. What are the four assumptions of exploratory factor analysis? What is the main goal of EFA in relation to partial coefficient values? What is the equation for partial correlation in EFA? Describe the terms: unique and common variance. What are the parameter that ideally should be met for an EFA according to Costello and Osborne? How do we determine the correct sample size for EFA? What is communality? How does communality effect sample size? What is the Kaiser Image Approach? What is measured by the diagonal and off-diagonal of an anti-image matrix? Describe the Kaiser measure of sampling adequacy. What are the two SPSS methods for EFA? Which one is better or more effective, and in which scenarios? Describe Haywood cases? What do they mean? What are the steps you should take when they appear? What are some methods for generating factor scores? What are the key differences and similarities between PCA and EFA? When would you use PCA and when would you use EFA? 31 LECTURE 5: STRUCTURAL EQUATION MODELLING 1: CONFIRMATORY FACTOR ANALYSIS While ANOVA, regression, chi square etc are all useful techniques, they share a common limitation: they can only investigate one relationship at a time. But often inter-related questions are more useful. Confirmatory factor analysis (CFA) is one type of SEM model - enables you to fit a comprehensive model to data that would otherwise require you to use multiple different techniques. For this lecture, think of it as a combination of regression and factor analysis. Combining factor analysis and regression analysis E.g. Does satisfaction with life at time point 1, predict satisfaction with life at time point 2? To get a score for each participant at time 1 on SWL, and time 1 on SWL, you could just add up their scores. But this is problematic as it assumes equal weights among the variables Instead to get a more meaningful analysis you could perform a factor analysis of the scale at each time point, save the factor scores, then use these new variables in a regression predicting time 2 (DV) from time 1 SWL (IV) Running a regression on these factor score, you can see that SWL at T1 significantly predicts SWL at T2. Constant in this analysis is close to zero, as the factor scores are standardized, so you would expect this. This method is advantageous in comparison to simply running a regression on the summed scores, as using the factor scores loads the observed variables in a more meaningful way How did we get this factor score: o Using the weighted average: score on each factor multiplied by the factor value, then summed o SPSS values are standardised (0 = average; therefore, negative scores indicated less than average [i.e., dissatisfaction with life]) What have we done here We first combined the observed variables in terms of an underlying factors - SWL at time 1 (oval). The squares represent the observed (or manifest) variables, which are explained by the factor. The underlying factor predicts the observed variable scores 32 Extra variances for each item on the left - the observed variables can be though of as having two causes: the latent factor, and the error term, which comprises measurement error and any other influence on the observed variable not captured. Each variable will have residual variance, as it is not fully explained by the factor This is the common factor model - there is a common factor for five items, and individual variation on each observed variable. We are doing this for each time point on SWL. We then use the factor scores for each time point to predict time 2 from time 1 There will be some error here as well - the error variance is enclosed in a circle as it is not directly observed. This represents not only measurement error, but everything else on which SWL may depend but is not captured by the observations o This is essential for the path diagram, as this is meant to be comprehensive. If you excluded the error variance, you would be making the implausible claim that SWL at time 2 is purely a function of time 1 and nothing else. The latent factor is a hypothesised and not directly observed construct that we represent through our observed variables. The latent factor is examined through our analysis of the consistency among the observed variables precise calculations related to this are not important here The idea is that the 5 manifest variables will tests the latent factor better than any one variable could. As they are meant to test the same underlying construct, they should be highly correlated. If they are, we believe that they are measuring the same underlying construct We have run a factor analysis one ach side, and then a regression connecting analysis and regression together, in comparison to doing them separately, over and above simply saving time Conceptually, in the structural equation modelling, you have the above two models. o The measurement model depicts how the observed variables come together to represent the latent construct o The structural model depicts the dependent relationships among constructs 33 The measurement models coincide with a theory, that firstly there is something called satisfaction with life that we can represent indirectly through measurement of manifest variables. Then the structural model depicts satisfaction with life as something that maintains itself over time. When you take these hypotheses, you get a measurement model which depicts how SWL can be represented, and a structural model embodying your theory about how SWL changes over time Confirmatory factor analysis (CFA) is a measurement model (left diagram) - you want to be able to confirm that your model is a plausible representation of the data. If you can show using this technique that your data is consistent with the measurement model, you have confirmed that the model is at least plausible. CONFIRMATORY ANALYSIS VERSUS EXPLANATORY ANALYSIS CFA is a way of testing how well variables represent a smaller number of constructs EFO could be described as orderly simplification of interrelated measures. EFA, traditionally, has been used to explore the possible underlying factor structure of a set of observed variables without imposing a preconceived structure on the outcome. By performing EFA, the underlying factor structure is identified. CFA is a statistical technique used to verify the factor structure of a set of observed variables. CFA allows the researcher to test the hypothesis that a relationship between observed variables and their underlying latent constructs exists. The researcher uses knowledge of the theory, empirical research, or both, postulates the relationship pattern a priori and then tests the hypothesis statistically. EFA can impose two kinds of restrictions: restrict the number of factors, and constrain the factor loadings to be uncorrelated with an orthogonal rotation You can do this and more with CFA - this can restrict factor loadings (or factor correlations or variance) to take certain values: a common value such as zero. If a factor loading was set to zero, the hypothesis is that the observed variable score was not due to the factor at all. o Can also fix the factor loadings to be equal with one another - if you do this the model fits quite well, you would conclude that simply summing up your variables is a good way of reducing them o With CFA you can get the degree of change in the fir of your model depending on which variables you allow to load on one another, the loadings you fix, and the variables you load as zero etc Following the purest form of CFA, if your predefined model did not fit, you would give up. But more realistically, you would adjust the model slightly using the information provided by SPSS, and then test the fit of the revised model on the same set of data (if you could, you should test this on a new data set) You need to try and find a model that still makes theoretical sense, is parsimonious without added complicated elements, and you will decently fit you data. Ideally then, you would cross-validate your revised model by testing it on your data It is not as though there are no exploratory components to CFA, and no confirmatory components to EFA - they differ in their rationale. In CFA, you have a predefined model you wish to test. In EFA, you wish to establish a model to test. Key advantage of CFA 0 using maximum likelihood and generalised least squares estimation, CFA has a test of model fit. So it is possible to test the null hypothesis that your factor loading is zero (your observed variables are not influenced by the underlying factor). You aim to confirm the fit of your model, hence confirmatory factor analysis Example: 11 subtest of the WISC ▪ Two factors: verbal IQ and performance IQ. Difference subtests (manifest variables) loading on the two factors Running an EFA on this: can see that each variable has a loading on each factor. With low loadings suppressed on the left, it appears that your model fits the data quite well, however, you need to remember that everything is 34 allowed to load on everything in EFA. Looking at the table, it is clear that some variables have quite high loadings on both sides (e.g., comprehension) CFA is going to do away with this model wherein every variable load on every factor, to get a more specific test of the hypothesised model Two model: hypothesised WISC on the right (to test in CFA) and EFA model on the left (poor for testing the model proposed by the WISC, as the WISC does not assume that each variables loads on each factor) Left is the model that the WISC hypothesised. Two correlated latent factors (verbal and performance IQ), which are each respectively measures by a set of subtasks. The model supposes that each subtask is loading on one of the factors, but not the other (no lines connecting the factors) o Strong model lots of assumed loadings of zero (e.g. Between picture completion and verbal intelligence - assumed nil relationship) Right is the EFA model - all variables load on all factors. This is much more complex, and with this model and EFA you cannot test the theory proposed by the WISC. With CFA, you can specifically test the hypothesised model proposed by the WISC, and see how well the model fits the data. In EFA, supressing low loading variables does not fully remove them from the analysis. With CFA, you are actually preventing variables from loading on the other factor. In CFA, you need to specify beforehand not only how many factors you will have, but also which factor each manifest variable will load on. In CFA, the researcher (or the theory), not the program, assigns variables to factors, and CFA provides a confirmatory test of your theory. Here there is close consistency between loading for CFA and EFA - this is a good sign of a well-designed scale. If the values were extremely different, this would tell you that your assumption of zero loadings for variables across factors is problematic. 35 SEVEN ISSUES FOR CFA 1. Sample size Nothing definitive is written about sample size in CFA Absolute sample size is important - recommendation of around 200 cases Also need to have around 20 cases for each parameter you are estimating (parameters are statistical estimates you require the computer to male based on your data). Less than 10 cases per parameter is highly problematic. Many indices of fit have been shown to be asymptomatic - as the sample size gets larger (400+), they resemble the actual distribution Maximum likelihood is the usual method for conducting CFA - you may need a larger sample depending on which technique you use. Also need a larger sample with more missing data. 2. Distributional assumptions Most estimation techniques assume multivariate normality of data. Maximum likelihood, the usual method, assumes this. With severe departure from normality you might investigate this and transform your variables Maximum likelihood estimation appears relatively robust to moderate violations of distributional assumptions. There are tests of these distributional assumptions (discussed below) Robust methods of estimation exist for drastic violations of this assumption 3. Levels of measurement Observed variables can be categorical, ordinal or continuous, however, continuous variables are generally assumed. As we generally work with the variances and covariances (unstandardized correlations) in our data, continuous variables are assumed. o The program doesn't mind if you feed it raw data or variances and covariances from that data Latent variables in SEM are ALWAYS assumed to be continuous 36 You also don't need to normalise the scale of your variables. You might have one variable scored out of 2000, and another scored out of 20 - the program will standardised the output for you 4. Identification Key issues Estimation of parameters is done in CFA through the solving of a number of complex equations (which we don't need to worry about) Constraints need to be placed on the model (not the data) in order for these equations to be solved unambiguously. This is not a problem with the data, it just means the model is not well defined. A model that can be estimated unambiguously is referred to as identified. An identified model exists when you can provide enough information for the structural equations to be solved unambiguously. An unidentified model cannot be estimated - program will be prevented from producing results The computer program takes in a variances and covariances matrix from the data. Based on the model we have specified, it will then some up with parameters (factor loadings, factor correlations and unique variances [residuals]). It then uses these parameters to calculate an estimated variances and covariances matrix (the model covariance matrix). If the generated variances and covariances matrix is similar to the raw matrix from the data, there is good fit of your model. The number of cells in the variance-covariance matrix can be calculated from the number of variables in the analysis - if the number of variables is n, then the number of cells is n(n + 1)/2 o A model in which the number of parameters is equal to the number of cells is said to be a just-identified model. This is a model wherein the number of estimated parameters is equal to the amount of information provided Underdetermined - this occurs when there are more parameters that need estimating than the data variances and covariances you gave to SPSS. More information requires estimation based on your model, than can be done with the amount of information your data provides o Here, you need to redesign you model. Gaining more data will not help Just determined - exactly the number of equations to estimate all the parameters. This means the program will use all the information provided to reproduce the sample variance-covariance matrix identically. This is guaranteed to have perfect fit: the model has zero degrees of freedom, and is saturated o Just identified models are just as complex as the data they are wishing to explain. You are not simplifying anything here - the perfect fit you get with a model like this is not interesting in terms of testing a theory Overdetermined - more than enough equations to estimate all the parameters. You have sent in more unique variances and covariances than the number of parameters to be estimated. There is enough information to estimate all the parameters, and some variances and covariances will be left over o Here, for any given measurement model, a solution can be found with positive degrees of freedom, and a corresponding chi square goodness of fit value o If you have at least 2 factors, then 1 items per factor should suffice, but 3 is recommended. Having more items per factor will only strengthen the condition o The goal in CFA and SEM in general is to have an over-identified model. In this case, you will be able to adjust the model to find the best fit with the data. If you have two models with comparable fit to the data, you prefer the simpler model. Over-identified models enable you to find the best fit. Why you prefer over identified to just identified? A major goal of model testing is falsifiability, and the just identified model cannot be falsified. The over-identified model will always be wrong to some degree, and the degree of wrongness of your over identified model tells you how well your model will fit o Only over-identified models provide fit statistics that we can interpret 37 ▪ o Under identified - 4 parameters to estimate, but only 3 unique variances/covariances (left) ▪ Just identified - 6 parameters to estimate, and 6 unique variances/covariances (right) Over identified model: 8 parameters to estimate, and 10 unique variances and covariances in the matrix. This is the best possible scenario. How to get identified CFA models o If each factor has at least three observed variables loading on it, and the loadings on one of the variables is fixed at a non-zero value (usually 1.0), then the model will be identified ▪ This follows from the fact that the scale of the unobserved variable is unknown. By fixing the loading of one observed variable to 1, you are using the scale of the observed variable to represent the latent factor. ▪ The scale of error variances (unique variances for each manifest variable) is also fixed at 1 - so each of these are set in the same scale as they observed variable to which they correspond o If there are at least two factors, then the model will be identified for only two variables loading per factor, if the factor is correlated with another factor, and one of the variable loadings (or factor variances) is fixed to a non-zero value ▪ For some more complex models these rules may not apply. Computer programs are often able to detect unidentified models and will compute an error message *****setting a factor loading to one does not mean there is a perfect correlation - this is a regression coefficient 5. Methods of estimation Most commonly used method of parameter estimation are: 38 o o o Unweighted least squares Generalised least squares Maximum likelihood Maximum likelihood is the preferred method for statistical tests, and is generally robust Other more esoteric methods can generally be ignored 6. Assessment of fit Model test statistics - these test how well the model specified by the research reproduces the observed data. The reason CFA is good is because you can test fit - you cannot do this in EFA (test model fit with zero loadings across factors etc - everything loads on everything in EFA so you cannot specifically test your model) There will be a discrepancy between the data and your model, but will the discrepancy be sufficiently small that it can be attributed to sampling error? Chi square is the initial test of fit of your model. Tests the null hypothesis that the model fits the data o A significant chi square (bad fit of model, reject null hypothesis that model fits) should only be taken as preliminary evidence against your model, and you should investigate further to understand why there is a discrepancy o A non-significant chi-square (good fit of model, favour the null hypothesis that the model fits) is good preliminary evidence against your model, but there could still be other influences driving this With the chi square test, if you have a large number of participants, it will tend to say your model doesn't fit (significant result), even if the discrepancy between your model and the data is trivial. Also, if you have few participants, you may have insufficient power to reject and false model o Don't ignore chi-square, just treat it as a preliminary investigation of the fir of your model Approximate fit indices - these have a different intention to model fit indices. These do not try and distinguish between sampling error and real evidence against the model, and they also do not present us with a dichotomous accept/ reject model based on the significant test. The lines between the different indices are blurred - might be referred to differently in different papers o Absolute - standardised root mean square residual (SRMR). This refers to the proportions of covariances in the sample data that are explained by the model ▪ Analyses the standardised residual covariances - the discrepancy between your sample data variance/covariance matrix, and the one generated with estimated parameters based on the mode. The discrepancy is the residual. ▪ SRMR gives you an overall residual value, by taking the square root of the mean of the squared residual covariances ▪ It is suggested that SRMR should be less than 0.08 on average. This has been criticised because and overall value of 0.08 can hide many individual values that exceed 0.08. Go beyond these benchmarks in thesis. o Comparative - comparative fit index (CFI). This is the relative improvement in fit compared to a baseline ▪ The CFI compares your model with a terrible model, and asks how much better your model is. You compare the model you just fitted, with a model which states that there are no common factors, and every variable is independent (this is the baseline against which we judge the fit of our model). o Parsimony adjusted - root mean square error of approximation (RMSEA). This assess model-sample discrepancy adjusted for sample size and number of parameters. ▪ These correct for model complexity. Larger sample sizes and simpler models, leads to smaller RMSEA values. RMSEA less then 0.05 is close fit o Best to cite an index of each kind: cite chi square, SRMR, CFI, RMSEA for a comprehensive analysis. Use these 4 on the assignment 7. Setting up a CFA - How to specify a CFA model CFA in AMOS 39 Drawing conventions Observed variable is a rectangle Unobserved common variable is an ellipse (oval) Unobserved unique or residual component is a circle Relationship: o Correlation is indicated by a curved, double-headed arrow o Regression is indicated by a straight single-headed arrow, aligned with the direction of prediction Left: uncorrelated factors. Right: correlated factors. Can see that the model fits slightly better when you allow the factors to correlate. DON"T FORGET TO CORRELATE YOUR FACTORS - 3 factors, you need correlations between all of them For uncorrelated factors squared multiple correlations appear in the top right corner of each observed variable. On left - 0.61 for information o This means that 61% of the variance on this information test, is accounted for by verbal intelligence. The remaining 39% is accounted for by the unique factor e1 o If e1 is purely represented by measurement error, you could say the reliability of the information test is 0.61, but e1 refers to many more influences that just measurement error Rule of thumb - you want the factors to explain at least 50% of the variation in your observed variables Interpreting the output from AMOS 40 Interpreting unstandardized coefficient (LEFT) : 1.02 for vocab indicated that an increase of one unit on verbal intelligence predicts an increase of 1.02 units on the vocabulary subtest, holding constant scores on all other factors (only one other factor here, so it would be 'holding constant scores on performance intelligence') Interpreting standardised coefficients (RIGHT): these standardised coefficients are independent of the units in which they are measured. 0.60 for picture comprehension - a 1 standard deviation increase in performance intelligence, predicts an increase of 0.60 standard deviations in the picture completion subtest, holding constant scores on verbal intelligence. Text output: o Distinct sample moments - refer to the sample means, variances and covariances. AMOS tends to ignore means, through, so this refers to the number of unique variances and covariances o Distinct parameters to be estimated - this is the number of parameters you are asking the program to estimate. If this is less than the distinct sample moments, you have degrees of freedom and an overidentified model o Minimum was achieved - AMOS successfully estimated the lowest value (best fit for the model) Assessment of normality output o CR stands for the critical value. Here we are looking for anything above 1.96 or below -1.96 41 o o We want multivariate normality - an extension of normality to multiple variables. AMOS provides tests of both univariate normality for each manifest variable, and also multivariate normality across the whole analysis. This is useful as univariate normality is a precondition for multivariate normality Multivariate CR statistic is the one to look at - you don't want it to be above 1.96. But if it is above it won't stop you from proceeding, just good to acknowledge this. Maximum likelihood is robust to violation of this. Parameter estimates - can see the regression weights (unstandardized and standardized) here, and also the correlations between the factors o SEM is fundamentally regression, and factor analysis is regression wherein the predictor variables are unobserved Measures of fit: default model is the picture we drew - assessment of fit of our model Saturated model is where the variances and covariances are allowed to freely vary Independence model is where all covariances are constrained to zero CMIN is the chi square analysis RMR is the root mean square residual value Baseline comparisons is CFI 42 Modification indices - useful feature for assessing which additional covariances would improve the model. AMOS assessed the adjustments to the overall fit it you adjust your model and allow extra covariances o MI is the modification index - this constitutes a conservative estimate of the DECREASE in chi-square (improvement in fit), that will occur if you adjust the model by the method concerned o Parameter change indicates what the parameter would change to o E.g. You can let chi square decrease by 8.955 if you let e2 co-vary with performance. The parameter would change to 0.978 o BUT you need to justify this on theoretical grounds if you are going to allow additional covariances. Do not change you model simply to fit idiosyncratic changes in the dataset. It you do this, your model won't fit well when you try and apply new datasets to it. 43 If you "load comprehension on performance", that means you are allowing performance intelligence to predict comprehension. Doing this improves the fit of the model Approach additional covariances or loadings with caution - they need to be well justified by the context of the research. If you add too many in, the model will become meaningless in terms of simplifying your data and will simply look like an EFA model With CFA, we are trying to find a model that makes theoretical sense, is decently simple, and fits the data reasonably well. Theoretical justification is always important, but we might need to trade off simplicity and fitting the data with each other to find the best model ASSESSMENT OF FIT - SUMMARY 1. Chi square ranges from 0 to infinity. Higher Chi Square value = worse model fit 2. SRMR ranges from 0 to 1. Higher SRMR = worse model fit 3. CFI ranges from 0 to 1. Higher CFI = better model fit 4. RMSEA ranges from 0 to 1. Higher RMSEA = worse model fit QUESTION LECTURE 5 1. Why is it beneficial to use CFA over regression analyses/ANOVA etc? 2. What are two ways of getting a weighted score or factor score? Why is one better than the other? 3. Why is the intercept in a regression analysis using factor score close to 0? 4. What is r1? 5. What is represented by the squares and circles in a CFA diagram? 6. What does the direction of the arrows mean between latent variables and variable measures in a CFA diagram? 7. Define measurement model? How does this differ from structural model? 8. What is the purpose of CFA? 9. How does CFA differ from EFA? Give an example. 10. What effect does constraining loadings to zero in CFA have on the factor loadings themselves, in comparison to those in EFA? 11. List, briefly, the seven key issues for CFA. Do not explain. 12. What is the perfect sample size for CFA? 13. What are some of the distributional assumptions of CFA? 14. Define Underdetermined. Give an example. 15. Define Just determined. Give an example. 16. Define Over determined. Give an example. 17. Which of the above three do we prefer and why? 18. How do we calculate the degrees of freedom in a CFA model? 19. Define saturated. 20. Give three examples of method of estimation. Which is the best? 21. Why do we pre-set a variable value to 1.0? 22. Give some examples for assessments of model fit. 23. What are some of the key issues with using Chi square assessment of fit tests? 24. What is meant by approximate fit indices? 25. Gives an example of an absolute, comparative and parsimony adjusted measure of model fit. 26. What does the squared multiple correlation value represent? 27. Describe the term "distinct sample moments" and "distinct parameters to be estimated". 28. How would you describe the output from AMOS? Use the example in the lecture. 29. Describe CR 44 30. Describe CMIN 31. How could we use modification indices as a means of strengthening models of fit? What are some problems with using Mis? 32. Give a brief summary of the four assessment of fit methods. 33. What is the differences between model fit statistics and approximate fit indices? Give an example of each. 45 LECTURE 6: BASIC IDEAS IN STRUCTURAL EQUATION MODELLING (SEM) Model and Equations Equation1 – the regression model o Tells us the relationship between a set of IVs and a DV o Each of the independent variables is summed by their weight in predicting the DV, with some residual or error variation on the end o All linear models can be expressed as some form of this equation Equation 2 – the factor model o An observed variable (x) is being predicted by a series of unobserved latent factors/ variables, denoted as f. Lambda coefficients replace beta coefficients here o A person's score on the observed variable (x) is given by their score on factor 1 multiplied by the loading of factor one, plus their score on factor 2 multiplied by the loading for factor 2, ect o U represents the unique variance for each factor. This is variance specific to X and not shared with any other variables or measures Matrix form – not important Notice that we have the observed variable (x) in both equations We could build a composite by substituting for (x) in from (x) in 2 and carry out the kind of predictive modelling we do in the regression model with the latent variables involved in the factor model. o That is, we can carry out our prediction using latent variables rather than observed variables, by substituting equation 2 (x) in place of equation (x). o There are several advantages to using a factor related to (x) to predict (y), rather than (x) itself AN ADVANTAGE OF LATENT VARIABLE MODELLING The main advantage is that models the characteristic of interest (dealing with constructs), rather than scores on an observed test This is often confused in research- researchers will say the control group were more depressed than the experimental group, when really they mean the control group displayed higher scores on the depression scale than the experimental group o This is confusion in measurement of manifest variables and latent variables o With latent variable modelling, you are able to discuss differences in the characteristic of interest, rather than just the score This can be understood with classical measurement theory (above) An observed score (o) is composed of a true score (T) and an error (E) o T represents the construct we are interested in (depression). o O represents the person's score on a construct measure (Beck Depression Inventory) o E represents a random error component – variation not captured by the latent construct T. This is analogous to the combination of the regression model with the factor model. o The observed score O replaces (x) in both equations 1 and 2 above. o The true score T represents the latent variable F in equation 2. 46 In test theory, reliability is the proportion of observed score variation that is true score variance. This never equals.0, therefore our observed score never fully captures the information in the latent variable. STRUCTURAL EQUATION MODELLING The joint modelling of data by equations 1 and 2 is known as structural equation modelling. This is when you combine the measurement and structural model to use latent factors to predict dependent variables variance. The aspect of the model concerned with equation 2 (factor predict observed score variance) is the measurement model The aspect of the model concerned with equation 1 (observed score predict dependent variable variance) is the structural model o Another term for this is path analysis. If we leave the latent variables out, and stick to models that only deal with observed variables, we are performing path analysis Structural model: set of one or more dependence relationships linking the hypothesised model's constructs. The structural model is most useful in representing the interrelationships of variables between constructs. Measurement model: a SEM model that (1) specifies the indicators for each construct and (2) enables an assessment of construct validity. The first of the two major steps in complete structural model analysis. Path analysis: general term for an approach that employs simple bivariate correlations to estimate relationships in a SEM model. Path analysis seeks to determine the strength of the paths shown in path diagrams. Structural equation modelling (SEM): multivariate technique combining aspects of factor analysis and multiple regression that enables the researcher to simultaneously examine a series of interrelated dependence relationships among the measured variables and latent constructs (variates) as well as between several latent constructs. CORRELATION AND REGRESSION As the correlation coefficient is a symmetric measure, it doesn't distinguish between predicting a from b and b from a However, regression does predict We cannot predict the increase/ decrease in a dependent variable from an increase/ decrease in an independent variable from a correlation coefficient alone. And if a correlation coefficient came to be 1.0, we could not infer a perfect relationship from a beta coefficient alone. These provide different information. A key point in regression when predicting Y from X is that we assume X variables to be fixed, and that there is sampling variation in Y o You can fix X values by assignment of groups, administration of a standard dose of a drug, etc Later when we predict Y from X, we do not second guess the X values – these are treated as fixed. By contrast, the model is saying that there is sampling error in Y. This creates an asymmetry – using X to predict Y is not the same as using Y to predict X. o In the line of best fit, 'best' means you are minimising the sum of squared deviations from the line, relative to the Y axis (when predict Y from X) o When you predict X from Y, you are minimizing the sum of squared deviations from the line, relative to the X axis. You would then say there is sampling variation in X, not in Y. 47 The correlation coefficient between X and Y will not be the same as the regression coefficient (beta, standardised) predicting Y from X unless the standard deviations of X and Y are identical When you standardise both variables, they are both centred on the origin (0), so the beta coefficient predicting Y from X and the correlation coefficient between X and Y will be the same o This is also why the constant disappears when we use standardised regression – it is centred on zero so the constant disappears Therefore regression and correlation are the same thing, just scaled differently CORRELATION AND CAUSATION There are some circumstances under which correlation is sufficient for causal claims o "in the biological sciences, especially, one often has to deal with a group of characteristics of conditions with are correlated because of a complex of interacting, uncontrollable and often obscure causes. The degree of correlation between two variables can be calculated by well-known methods, but when it is found it gives merely the resultant of all connecting paths of influence". Correlation is consistent with causation under the following conditions: 48 1. There is temporal ordering of the variables. The cause X needs to occur before the effect Y. this happens quite naturally in experimental studies – you administer a drug (cause X) and measure the results (effect Y). In crosssectional studies, it is up to the researcher to affirm that X comes first and causes Y, rather than the opposite. 2. Covariation or correlation is present among the variables. Here, causation necessitates correlation. This is not necessarily linear correlation of the sort we are used to. 3. Other causes are controlled for. This is extremely difficult to defend in practice, but it is imperative for claims that correlation is sufficient for causation. 4. Variables are measured on at least an interval scale (NOT TRUE, THIS IS NOT A REQUIREMENT FOR CORRELATION TO BE CONSISTENT WITH CAUSATION) CONTEMPORARY PATH MODELS In these models, you need to include residual variation to make them complete. Otherwise you are claiming that your variables are purely a function of those on which they depend Path models are expressed as diagrams. The drawing conventions are the same as in CFA: o Straight line single-head arrows are used to indicate causal or predictive relationships o Curved double-head arrows indicate a non-directional relationship such as correlation or covariance Bivariate regression – this is simple regression where X predicts Y. There are two observed variables here – bivariate regression. The residual is represented as a latent variable E as this is not observed, only estimated. Assume here that X is measured without error. TWO KINDS OF PATH MODELS Recursive models are simpler: o The paths (causal effects) are unidirectional o The residual (error) terms are independent o Such models can be tested with standard multiple regression Non-recursive models are more complex: o Bidirectional paths (causal effects) o Correlated errors o Feedback loops Non-recursive models can have feedback loops. These arise if you have a path diagram which can to go a variable, and then trace along single headed arrows to the original variable. 49 Indirect feedback loop – you can trace back to the original predictor from the final predictor Direct feedback loop – where you have one variable simultaneously predicting another, and being predicted by the other (bidirectional path) Non-recursive models can also have correlated errors Endogenous construct: latent, multi-item equivalent to dependent variables. An endogenous construct is represented by a variate of dependent variables. In terms of a path diagram, one or more arrows lead into the endogenous construct. Exogenous constructs: latent, multi-item equivalent of independent variables. They are constructs determined by factors outside the model. MODELLING DATA FOR PATH ANALYSIS This can be done via multiple regression, or structural equation modelling Example – data from attitude modelling of factors affecting perceived risk in genetically modified food (DV). Scores on four attitude scales (measuring attitudes to technology, to nature, neophobia and alienation, IVs) were used to predict scores on perceived risk. Running this in standard multiple regression in SPSS, the following results are produced for model: Regression model equations are the first two, and the 3rd is the full regression equation, modelling actual risk scores (the entire data) as the error is included. R square from SPSS is 0.387. AMOS model – the four predictors are predicting actual perceived risk, and what they don't predict is accounted for by the error term 50 Comparing results from SPSS regression and AMOS SEM: o Regression weight are the same o Standard errors differ o Standardised regression weights differ o Squared multiple correlation is less in AMOS ▪ This is all due to uncorrelated predictors in AMOS For AMOS SEM results to match regression results perfectly, you need to include correlations among exogenous variables (variables that predict others, but are not themselves predicted). Conclude from this that multiple regression must model the correlations among the independent variables, however this is not shown. A path analytic representation is therefore much more accurate and provides more conclusive information For example, if we model the associations among the exogenous variables, we can see that not all are statistically different from zero and could be deleted from the model 51 We now have a more parsimonious model now that some of the double headed arrows have been removed. Because those correlations were non-significant, not much changes in the model parameters. However, this shows that you have more flexibility to closely examine the model when using AMOS for regression Another advantage is that once you have removed some of the exogenous variable correlations, you have an overidentified model, which can be tested for significance. Model fit is now possible using the chi square test LATENT VARIABLE REGRESSION Some real advantages become clear when we have latent variables in the model – combined factor/ regression analysis Comparing results for observed variable regression vs latent variable regression: On the left, when scores are included as predictor variables, these represent equally weighted sums of observed variables. Affiliation score is just the sum of four affiliation items. On the right is the model for when you have individual item data as well. Here the affiliation score is modelled by a factor, which predicts the observed variables by varying factor loadings. Here we are not just summing up scores evenly to get an affiliation score, we create an affiliation score latent variable. o Here, the beta regression coefficients and the squared semipartial correlation are both higher From this comparison it is clear that the relationship between the characteristics is much stronger than the relationship between the scores. Comparing the two models o In the first model: regression of manifest variables to predict activity score. You make the assumption here that all of your items are worth equal weight in making up the 'score' for each predictor variable. Assumes all items assessing affiliation are worth the same. o In the second model: when you use latent factors, you do not assume they all carry equal weight. This helps you explain more variance in the predicted variable and increase R square. Each of the individual variables is weighted differently in comprising the factor in this model. 52 SECTION 2 – MULTI STEP REGRESSIONS The most important use of path analysis is not the mino improvement in regression variance explained, but in dealing with much more complex situations MULTI-STEP PATH ANALYSIS In multiple regression, some variables are predictors (A), some variables are predicted (B), and some variables are both predictors and predicted (M). o A -> M -> B Intervening variables like M may be considered as mediating the relationship between A and B Example – women's healthy study. One interest was in the usage of health facilities – frequency of visit to health professionals (this is DV = timdrs) o Predictor variables are age, stress, self-esteem, self reported physical health The hypothesised model is that self-esteem predicts doctor visits indirectly, through its prediction of physical health. Physical health is therefore the mediating variable in this relationship. Age, stress and physical health all predict DV directly. 1. Proposed theoretical model requires two regressions (if done separately): Regress physical health on age, stress, self-esteem 2. Regress timdrs on age, stress and physical health SPSS results for the two regressions are presented below. Regression (phyhealth on age, stress, esteem) is on left, and regression 2 (timdrs on age, stress, phyheal) is on right HOW DO WE COMBINE THE TWO REGRESSIONS? Limitation of doing this as two separate regressions, is that it does not provide a measure of the overall fit of the theoretical model presented above. You are only permitted to assess the fit of each of the models (left and right) here, not overall with the two combined. To get an overall measure of fit from two separate regressions, you can estimate the generalised squared multiple correlation, which uses the R square value for each regression: Rm2 = 1 - π(1-R2) Here, -R squared represents the unexplained variance of each regression equation 0.32 indicates that 32% of the variation in timdrs is explained by the relations in the path model. However, this is an old fashion way of assessing overall model fit. 53 What about the effects of antecedent variables on dependent variables? These can be broken down into direct and indirect effects. o Directly antecedent variables: where one variable directly impacts another. Degree of impact is beta. Age, stress and physical health all have direct effects on timdrs. o Indirect antecedent variables: where one variables predicts another, through an intervening variable. Degree of impact in an indirect effect involves multiplying the beta coefficients together for the sequential paths. Stress has a direct effect on timedrs, but also has an indirect effect on timdrs via prediction of physical health The full effect of one variable on another is a combination of the direct and indirect effects. To obtain indirect affects you take the standardised coefficients for the two paths and multiply them together. From this you can build claims that stress works more on timdrs through physical health. Doing the two regressions separately is a very old fashioned way of doing things. It is simpler and more efficient to run this in AMOS. STRUCTURAL EQUATION MODEL – TWO REGRESSIONS COMBINED IN AMOS 54 Unstandardized estimates from SPSS up the top, and the estimates from AMOS underneath Comparing these, we see that the regression coefficients are the same. The standard errors differ slightly as we have uncorrelated predictors in our SEM, but if we had correlated them they would be the same Squared multiple correlations are now higher from AMOS – more variance in the predicted variables is explained by variation in the predictors When correlations among exogenous variables are included, the results from AMOS match the SPSS regression results exactly This illustrates that SEM and regression are quite similar, but SEM gives you more flexibility to allow your predictors to correlate or not. Regression forces you to have them correlate, which might not be advantageos as in this case the explained variance was better with uncorrelated predictors. Chi-Square: statistical measure of difference used to compare the observed and estimated covariance matrices. It is the only measure that has a direct statistical test as to its significance, and it forms the basis for many other goodness-of-fit measures SECTION 3 – MODEL FIT Absolute fit indices: measures of overall goodness-of-fit for both the structural and measurement models. This type of measure does not make any comparison to a specified null model (increment fit measure) or adjust for a number of parameters in the estimated model (parsimonious fit measures ASSESSING FIT A good model exhibits good fit between the sample covariance matrix and the estimated population covariance matrix o This could be indicated by a non-significant chi square. Chi square test is an absolute fit index, testing the model against the data o However, with large samples, trivial differences between model and data may be driving the significant result o Also, with small samples, the computed statistic may not be distributed exactly, with chi square giving inaccurate probability levels. o Significant chi square is insufficient in itself to determine whether a model should be rejected, but can be treated as a 'smoke alarm' in relation to model fit o Chi is strongly affected by multivariate non-normality, correlation size, the size of unique variance, and sample size 55 Many alternative fit indices have been proposed. SEM assesses fit through convergent validity – use different indices of fit and hope they all agree APPROXIMATE FIT INDICES Approximate fit indices ignores the issue of sampling error and take different perspectives on providing a continuous measure of model-data correspondence. Three main flavours available under ML estimation: o Absolute fit indices (proportion of the observed variance-covariance matrix explained by model) e.g., SRMR o Comparative fit indices (relative improvement in fit compared to a baseline) e.g., CFI o Parsimony-adjusted-indices (compare model to observed data but penalise models with greater complexity) e.g., RMSEA ABSOLUTE FIT INDICES RMRL root mean square residuals o Average difference between observed and model-estimated covariance matrices o Small indicates good fit, 0 = perfect fit o Hard to interpret because RMR's range depends on the range of the observed variables SRMR: standardized RMR o Transforms the sample and model-estimated covariance matrices into correlation matrices o Ranges from 0 to 1, with 0 = perfect fit o SMRS < 0.08 regarded as good fit by Hu and Bentler, 1999 – beware that this average value can hide some big residuals COMPARATIVE FIT INDICES NFI: Bentler – Bonnett Normed Fit Index o Compares the chi square of the model to the chi square of an independence model o High values (>0.95) indicates good fit but may underestimate fit in small samples CIF: Bentler's Comparative Index o Some similarity to NFI but adjusted for non-centrality in the chi square distributions o High values (>0.95) indicate good fit PARSIMONY-ADJUSTED INDICES RMSEA: Root mean square error of approximation o Acts to 'reward' models analysed with larger samples, and model with more degrees of freedom o Badness of fit statistic – lower is better and zero is best o If it turns out to be less than zero, treat it as zero REPORTING FIT Good fitting models produce consistent results on many different indices. If these generally agree, the choice on which to report is largely personal preference. Often people will report the indices that describe the best fit. CFI and RMSEA are frequently reported, and Hu & Bentler (1999) suggest SRMR o A nested model is a model that uses the same variables (and cases!) as another model but specifies atleast one additional parameter to be estimated. The model with fewer restrictions or more free parameters (I.e., fewer degree of freedom), which could be called a reduced model, is nested within the more restricted model, which could be called the full model. LIMITATIONS OF FIT STATISTICS Kleine (2016) lists six main limitations of fit statistics: 1. They test only the average/overall fit of a model 2. Each statistic reflects only a specific aspect of fit 3. They don't relate clearly to the degree/type of model misspecification 56 4. Well-fitting models do not necessarily have high explanatory power 5. They cannot indicate whether results are theoretically meaningful 6. Fit statistics say little about person-level fit. Underfitting occurs when the model is overly simple Overfitting occurs when the model is overly complex (this results in a model that fits the data too well – almost perfectly) SECTION 4 – PATH MODELS WITH LATENT VARIABLES EXAMPLE – Women's Health Study. Research question involves the usage of health facilities. We assume the latent constructs: o Sense of self (self), o Perception of health (perchealth), and o Use of health facilities (usehealth). Latent constructs are measured by: o Self: self-esteem (esteem), attitudes to marriage (attmar), locus of control (control) o Perchealth: self-reported mental health problems (menhealth), physical health problems (physhealth) o Usehealth: use of medicines (druguse), visits to health professionals (timdrs) Control variables: o Age, stress will be used as manifest control variables Latent constructs predict variation in manifest variables. This is not a complete factor model as we don't have many indicator variables, but those we do have seen sensible Control variables – these are manifest as they are control variables. We assume these variables are stable, so we don't need multiple indicators to treat them as latent constructs. The path model includes both manifest and latent variables in a regression. Assessing model fit indices, the above default model does not fit well. o Chi square = 99.942. DF = 20. P =.000 o CFI =.881 o RMSEA =.093 o SRMR =.0592 o AIC = 149.942 Assessing modification indices, we don't want the model too much as this is not supposed to be an exploratory technique. If alterations do not violate good sense or theory, there can be scope for their use. 57 o Most interesting suggested extra path is predicting usehealth from self (two latent constructs) o Adding this extra loading in did not work – produced negative variables in the model. Variances cannot be negative – if the model is estimating negative variances, we have a big problem with the model. One risk factor for this is when you have latent variables that only predict a few observed variables Assessing the modification indices for extra covariances in the model indicates improvements from correlating residuals. Correlations among the residuals of measures induces correlations among latent variable outcomes. o Including correlations among residuals might make theoretical sense if the two variables both predict positive or negative residual values (countries # of people murdered example) 58 With these additional covariances among error terms, there was a big improvement in model fit This comparison can be made for nested models – models are nested if one is a subset of the other. The model without correlations among unique variances is nested inside the model where the correlations are included. The default model without the error correlations is simpler, so is nested inside the new model without them included. This is a typical process for identifying the best fitting model that is not too distinct from your originally theoretically proposed model BOOTSTRAPPING This technique is important for when the assumptions of SEM are violated – multivariate normally When assessing normality assumptions, the critical statistics is kurtosis o Practically, very small assumptions, the critical statistic is kurtosis, considered negligible while values ranging from one to ten often indicate moderate non-normality. Values that exceed ten indicate severe nonnormality 59 Severe problems with non-normality and kurtosis here Mahalanobis distance – looks at how close each case is to the centroid. The centroid is analogous to the multivariate version of the mean – we want to identify people that are far away from the centroid o P1 indicates, assuming normality, that the probability of any arbitrary case exceeding a value of 44.01 is very low. We want low values in P1. o P2 indicates that assuming normality, the probability is.001 that the largest mahalanobis distance value would exceed 44.010. That is, given the sample we have, what is the probability that the largest value will exceed 44.010. Low values in P2 are bad – indicates we have some observations that are very far from the centroid under the assumption of normality. This therefore questions the assumption of normality in the data. Looking at the P2 column for person 277 – this is the probability that in your sample, the second biggest d square value is bigger than 42.30. This is even less likely – less likely that the 2nd biggest d square value would be as big as what we have. Indicates we have issues with some of these observations in our sample. When the assumption of normality is not met: o The model can be incorrectly rejected as not fitting o Standard errors will be smaller than they really are (parameters may seem significant when they are not) The