PSY 200 Study Guide PDF
Document Details
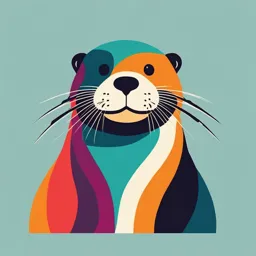
Uploaded by InnovativeByzantineArt1681
Tags
Summary
This study guide provides an overview of psychological research methodologies and concepts. It covers the theory-data cycle, scientific thinking, and common biases in everyday thinking. The guide also includes discussions on design confounds, different types of research, and statistical analysis. Perfect for psychological science students.
Full Transcript
PSY 200 - Slone - Study Guide Notes: Know all boldface terms from the chapters and the lecture notes, in addition to the below focal concepts. Don’t just know definitions; be able to apply these concepts. Not all of these topics may appear on an exam. Rather, students who have a strong...
PSY 200 - Slone - Study Guide Notes: Know all boldface terms from the chapters and the lecture notes, in addition to the below focal concepts. Don’t just know definitions; be able to apply these concepts. Not all of these topics may appear on an exam. Rather, students who have a strong grasp of the below topics will likely perform well on the exam. APA style specifics and the content of our class observation/experiment will not be covered on exams (this is “tested” in your APA paper and other written exercises). Unit 1: Ch1-5 1. Four goals of psychological research To DESCRIBE human behavior/thoughts/feelings To EXPLAIN “” To PREDICT To CHANGE Darling elephants predict change! 2. Elements and process of the Theory-Data cycle (e.g., what makes a good theory?) Theory → set of statements that describe general principles about how variables relate to one another Hypothesis - set of predictions about study outcomes Data - set of observations → do they support or refute the theory? A good theory is supported by the weight of evidence (many studies) - Falsifiable, has parsimony (simple = best) - But…cannot be “proved” 3. Everyday thinking (experience, intuition) vs scientific thinking (research), ways intuition is biased Personal experience has no control group; experience is confounded Ways intuition can be biased ○ Swayed by good story ○ Availability heuristic (what comes easily to mind guides our thinking) ○ Failure to think about what we can’t see ○ present/present bias – failure to consider appropriate comparison groups Example: when someone believes they have a psychic connection because they thought about a friend right before receiving a text message from them, but they fail to consider all the times they thought about friends who didn't text them or received texts when not thinking about anyone in particular ○ Confirmation bias – seeking/accepting information about something we already believe ○ Biased about being biased Scientific thinking/research: ○ Asks, “compared to what?”; has systematic comparison ○ Controls for confounds ○ Research → probabilistic and relies on averages; explains majority but not all cases ○ Scientific method helps us avoid bias; systematic (control for biases) and empirical (direct observation and experimentation, with comparison groups) ○ 2x2 matrix: 4. Observation vs Experimentation Observational research - watching behavior and recording it; no interference Experimentation - at least one variable is manipulated and another is measured ○ Manipulated by assigning participants to different levels of that variable (some variables can’t be manipulated for logistical or practical reasons) 5. Basic, Applied, Translational research Basic research - contributes to general knowledge; can apply to anyone, any population Applied research - addresses a practical problem; targets specific population (e.g., classroom program study) Translational research - uses lessons from basic research to test applications (think “evidence-based strategies) →aspects of both basic and applied research 6. How to evaluate studies and claims reported in the media Ask: ○ How good is the study behind the story? From peer-reviewed journal? ○ Is the story accurate? 7. Be able to describe what results (data) we would expect to see if a certain theory or explanation applies (e.g., Exercise 1) Would expect to see some kind of statistically significant data; for example, a strong correlation between 2 variables that the theory would predict and explain Research results are probabilistic →do not explain all findings all the time 8. Be able to consider alternative explanations to claims (e.g., Exercise 1) - Example - cannot for sure say that bed-sharing caused someone to have lower anxiety, could be genetic component (calmer parents choose to bed-share, have calmer kids) - Gum chewing → does it actually cause people to appear friendlier? Or is it an alternative explanation that explains why people preferred those chewing gum (e.g., smiling muscles activated) 9. Define operationalization and create operational definitions of conceptual variables Operationalization - turning a concept into a measured or manipulated variable Types of measures: observational, physiological, self-report Think of ways to measure sleep, stress, and job satisfaction for each type of measure (operationalize them!) Exercise 3 part 1 - Possible operational definitions of attention span: eye tracking device, brain scan, self-reports 10. Be able to identify all elements of IVs & DVs (construct? manipulated or measured? levels? operational definition?) from a description of research construct/conceptual variable - used in discussion of theories/journalist research Operational definition of a variable - used when testing hypotheses with empirical research IV - what we are conceptualizing as the predictor → usually manipulated in an experiment (the cause) DV - what we’re predicting ○ Always measured, the effect 11. Measured vs. manipulated variables. Can all variables be manipulated? When are IVs manipulated vs measured? Measured - have levels that are observed and recorded Manipulated - controlled by researchers, usually by randomly assigning study participants to different levels; participants are assigned to; no, not all variables can be manipulated for ethical and logistical reasons (ex. - age, trauma responses, etc.) 12. Confounds - be able to identify them in a study and explain how they relate to internal validity Confounds = alternate explanations of results Controlled for via random assignment to different levels of an independent variable 13. Scales of measurement (Categorical, Ordinal, Interval, Ratio) Categorical - aka nominal variables; levels are categories (ex. - age, sex, species) Quantitative - aka continuous variables; levels are coded with meaningful numbers (height, weight, IQ score, wellbeing measured w scale) ○ 3 types: ○ Ordinal scale - numerals represent ranked order Ex - ranked list of top 10 bestsellers\ Intervals may be unequal ○ Interval Numerals represent equal intervals (dist btw levels) There is no true zero; person can get a score of 0 but 0 does NOT = nothing (ex. - intelligence score; score of 0 does not mean “no intelligence”) Researchers cannot say things like “twice as hot” or “3x happier” ○ Ratio Equal variables, value of 0 truly means none Ex - how many questions answered correctly Researchers can say, “twice as much”, etc. 14. Ethical standards in psychological science, and where they come from Needed because of historical atrocities (Tuskegee Syphilis Study, Milgram Obedience Study) Come from personal standards and APA Ethics Code, which governs professional standards of research 5 principles of psych researching (1978 Belmont Report + 2): ○ Respect for persons/ people’s rights and dignity - individuals should be treated as autonomous agents Informed consent, no coercion (threats) or undue influence Special protection for those with less autonomy (children, disabled, prisoners) ○ Beneficence (and nonmaleficence) - precautions to protect participants from physical and emotional harm; research should ideally benefit them Researchers cannot withhold treatments known to be effective Info must be protected (anonymity, confidentiality) ○ Justice Fair balance between kinds of ppl who benefit from study and ppl who participate in study ○ Fidelity and responsibility - relationships based on trust; accept responsibility for professional behavior; no conflicts of interests between therapists/patients ○ Integrity - professionals must teach accurately, stay updated on current research, accurately represent data and give credit when due 15. Risk/benefit ratio; are ethical decisions subjective or objective? Balancing priorities for research Subjective evaluation of costs and benefits of a research project (to participants, society, researchers) ○ Includes… what’s the risk if we don’t do this research? 16. [Ethical] risk in a study - minimal risk, at risk, types of potential risks (Physical, Social, Psychological) Minimal risk = the harm or discomfort participants may experience is not more than they they would be expected to experience in everyday life during routine physical/psychological tests 17. Informed consent - what it is? when/why use it? how to do it? Informed consent - obligation to explain study in everyday language ○ Needed in some kinds of studies to meet principle of respect for persons ○ Must include basic info about study, risks (anything that might affect willingness to participate) ○ Do NOT need to inform about hypotheses Not required for studies not likely to cause harm, or for observational studies Must tell participants whether data is kept private or confidential or not NOT necessary for 18. Deception - what it is? when/why use it? how to do it? Deception = lying, withholding information from participants Participants cannot be told all aspects of a study in order to ensure validity of results ○ Deception through omission (some study details withheld) ○ Deception through commission (outright lying) Often necessary to obtain meaningful results Guidelines: use as last resort, must debrief immediately afterward if it occurs 19. Debriefing - what it is? when/why use it? how to do it? Occurs at end of a study when participants are informed about purposes of study, specific details about how info will be used Necessary when deception has occurred, must occur immediately at the end of a study to prevent long-term psych harm 20. Institutional Review Board (IRB) Committees responsible for reviewing studies and ensuring research involving humans is conducted ethically; universities, research hospitals have them Must be made up of @ least: 1 scientist not affiliated with research in question, academic outside of sciences, community member, prison advocate (if applicable) IRBs attempt to balance welfare of participants and researchers’ goals to contribute to body of knowledge, benefit society 21. Three claims (Frequency, Association, Causal claims): number of variables involved, how to identify them, how they are connected to the four goals of psychological research Claims = arguments someone is trying to make Frequency claims - describes rate or degree of a single variable (variable involved is a measured variable) Association claims - argues 1 level of a variable is likely to be associated with a particular level of another variable ○ At least 2 variables ○ Associated variables - said to correlate or co-vary ○ +/- correlations ○ Allow us to make predictions Causal ○ At least 2 variables; at least 1 manipulated ○ 1 variable responsible for changing the other How they’re related to 4 goals of psych research (describe, explain, predict, change human behavior): frequency claims → describe, association claims predict, causal can allow us to explain and change 22. Four validities (construct, internal, external, and statistical validities): definitions, how to evaluate them from a description of a study Construct validity - are the variables measuring what they are supposed to? How well is variable operationalized? Internal validity - are confounds/alternate explanations controlled for? External validity - how generalizable is the conclusion to a wider population? Statistical validity - how well do the data support the claim? Are statistical conclusions precise, reasonable, replicable? 23. Three claims and the four validities: Which validities would we use to interrogate frequency claims? Association claims? Causal claims? How? Which validities are most important for each type of claim? Interrogating frequency claims: ○ Construct validity ○ External validity ○ Statistical validity Interrogating association claims: ○ Same validities as above (**internal validity only applies to causal claims!!) Interrogating causal claims: ○ Construct, external, statistical PLUS internal validity →must meet three additional criteria for causation: Correlation Temporal precedence Internal validity ○ Only experiments can support causal claims 24. Ways to measure variables (self-report, observational, physiological) - Self-report (surveys, interviews → people’s answers about themselves) - Observational (paying attention to behavior → records of observable behavior) - Physiological (monitoring biological measures – heart rate, hormone levels, brain activity) 25. Construct validity: Types of reliability (inter-rater, test-retest, internal) Reliability (all do not apply in all cases) ○ Test-retest reliability: people get consistent scores every time they take the test Statistic: correlation between 2 numbers Test-retest often done within shorter time scale ○ Interrator reliability: 2 coders’ ratings of a set of targets are consistent with each other (consistent scores obtained no matter who measures the variable) Statistic: R-value is greater than 0.7 Most relevant for observational measures ○ Internal reliability: consistent pattern of answers no matter how the question is phrased Statistics: Lots of correlations need to be computed → average inter-item correlation (AIC) Crombach’s Alpha → takes into consideration the # of items 26. How is reliability quantified? Can use correlation coefficient r to quantify R = how close points are on a scatterplot to a line drawn to them ○ Represents strength (strong when abs. value of r is > 0.7) and direction (+/-) When measuring internal reliability: ○ Use average inter-item correlation (AIC) → does response on one question predict response on another? ○ AIC between.15 and.50 mean items go reasonably well together ○ Combine AIC & # of items on scale to get Cronbach’s alpha (closer to 1, the better) 27. Correlations, scatter plots, correlation coefficients Correlation coefficient (r)- the closer to absolute value of 1 → 2 variables are more correlated ○ > 0.7 is typically considered correlated ○ +/- measures directions of relationship 28. Cronbach’s alpha (for internal reliability) - When measuring internal reliability: - Use average inter-item correlation (AIC) → does response on one question predict response on another? - AIC between.15 and.50 mean items go reasonably well together - Combine AIC & # of items on scale to get Cronbach’s alpha (closer to 1, the better) - 29. Construct validity: Types of measurement validity (face, content, criterion, convergent, discriminant) Face validity - does it look like a good measure of the construct? Content validity - does it capture all parts of the construct? ^^ more subjective; ⌄⌄ more empirical Criterion validity - does it correlate with concrete behavioral outcomes it theoretically should be associated with? ○ Can use known-groups paradigm (researchers see whether scores on measure can discriminate among 2+ groups whose behavior is already confirmed) Convergent and discriminant validity ○ Convergent - tests should correlate with things you would expect it to ○ Discriminant - tests should not correlate with things you would not expect it to Reliability is necessary but not sufficient for validity!! 30. Reliability & validity - how are they related? Reliability of measurement - does it produce the same/similar results each time? Validity measurement - does it measure what it purports to measure? In-class practice: Construct validity (types of measurement validity): - Criterion validity: potential concrete behavioral outcomes - ADHD diagnosis - Visits to school counselor - School behavioral reports - Convergent validity: results of Strengths & Difficulties Questionnaire should correlate with similar types of questionnaire - Discriminant validity: In general, to have a causal relationship, variables must be manipulated in some way Data falsification vs data fabrication - Falsification = researchers influence study’s results, perhaps by deleting observations from data set - Fabrication = making up data STUDY PLAN: Use notes to create summary sheet Whiteboard out all knowledge already known Go through and answer study guide questions create quizlet + study Re-write figure 5.8 Chapter questions Textbook blog (“Everyday research methods blog”) Questions Examples sorted chapter-by-chapter Homework exercises Chapter quizzes 1 2 3 4 5 Test format: 25ish MCQ, 4 short answer questions (almost half of exam points), long answer, fill in the blank (no word bank) ⇒ look at exercises to review Unit 2: Ch 6-8 31. Correlations, scatterplots, correlation coefficients, and how to interpret them Correlation coefficient (r)- the closer to absolute value of 1 → variables are more correlated ○ > 0.7 is typically considered correlated ○ +/- measures directions of relationship ○ R value interpretation in psychology: 0.5 → very weak.10 → small or weak.20 →moderate.30 → fairly powerful.40 →unusually large; very powerful; too good to be true(?) based on small sample 32. Be able to define and identify observer bias, observer effects, reactivity Observer bias (in recording or interpreting participants’ behaviors) - observers see what they expect to see ○ Avoid by not telling the observer the hypothesis (double-blinding); clear coding manuals Reactivity (of the participants) - participants behave differently because they know they are being observed ○ A form of reactivity is behavior effects ○ Solution: Use unobstructive observation ○ Ex. - teachers’ expectations for students influence how well they perform Observer effects (AKA expectancy effects) (on the participants’ behaviors) - observers inadvertently change behavior of those they’re observing ○ Teachers’ expectations influence student performance 33. Methods to prevent observer bias, observer effects, reactivity How to avoid these problems: unobstructive observation can help prevent reactivity; double-blinding can help prevent observer bias, observer effects; clear coding manuals to prevent bias (clear operational definitions & training in identifying target behavior) ○ Use multiple observers →check for inter-rator reliability; doesn’t eliminate bias but helps you be aware of it ○ Almost always need to ensure masked (blind) designs - participants shouldn’t know study’s purpose or their condition Double blind design - researcher/observer also “blind” to condition/study’s purpose 34. Surveys, polls: ways questions can be formatted, worded, and administered, and pros/cons Question format does not make or break construct validity Ways questions can be formatted: ○ Open-ended questions Pro: spontaneous, rich info Con: responses must be coded + categorized (takes time) Not used often in psych because of inefficiency ○ Forced-choice questions Pick best of 2 options, often used to measure personality, yes/no question ○ Likert scale Must contain options of strongly agree, agree, neither agree nor disagree, disagree, and strongly disagree (5 options!) If not EXACTLY these options, then it’s a Likert-like scale ○ Semantic differential format Respondents asked to rate target object using numeric scale anchored with adjectives Example - “profs get F’s too” 1 2 3 4 5 “A real gem” 35. Factors that affect the accuracy of survey responses and methods to help ensure accuracy (construct validity) ○ Well-worded questions (avoid leading questions, double-barreled questions, negative wording) i. Leading questions - biased language that leads toward a certain response ii. Double-barreled - 2 questions in one iii. Negative wording - non-, im-, not-, un- double negatives ○ Order matters…prepare different versions of survey with difference sequences →one question can impact responses to later questions by putting you in a particular mindset ○ Flip questions (reverse-worded) to check for internal reliability, whether acquiescence is going on (someone is agreeing to everything) ○ Provide no middle group - use forced choice, even # of options to avoid fence-sitting (which is common w/ controversial topics) ○ Avoid socially desirable responding by making polls anonymous, ask friends to respond instead, force quick implicit responding ○ Can respondents accurately report what we’re asking them? i. No, usually not for self-reflection questions or questions about motivation of behavior, because confidence about memories does not = accuracy ii. People are unconsciously swayed in product reviews by outside factors like price, others’ opinions, etc. 36. Population vs. sample - Population - entire set of people/products in which you’re interested - Sample - smaller set taken from population - Just because a sample comes from a population does not mean that it generalizes to that pop 37. Representative (unbiased, random, probability) vs unrepresentative (biased, non-random, non-probability) sampling: what it is, methods (know the different types of samples), pros/cons - Representative sample: leads to better external validity → external validity is most important for frequency claims - Use probability sampling/random sampling - Simple random sampling (most basic) - Systematic sampling (2 counts, start at first, count by second) - Cluster sampling (divided into arbitrary groups, all individuals in randomly selected group are selected) - Related technique - multistage sampling - random sample of clusters - Stratified random sampling - intentional groups - Oversampling - intentionally overrepresenting minority group to get accurate data - Researchers often use a combination of sampling techniques! - Unbiased - all members have equal chance of being included in sample - Unrepresentative sample: - Biased - some members of a population of interest have much higher probability than other members of being included in sample - When is a sample biased? If it contains too many “unusual”people (ex. - students who leave reviews on prof’s website may have stronger opinions than those who didn’t) - Convenience sampling - sampling those easy to reach - Purposive sampling - when only certain kinds of people are recruited - snowball sampling - participants are asked to recommend a few acquaintances for the study (people recruited via social networks) - Quota sampling - researcher identifies subsets of population of interest & then sets target # for each category; continues sampling until quota is reached - Self-selection - participants select themselves to participate; can be avoided with random selection 38. Stages of data analysis Stage 1: Get to know your data (“cleaning” your data) Stage 2: Summarize the data ○ Visual methods, Central Tendency (mean, median, mode), Variation/Dispersion (range, standard deviation, standard error of the mean) Central tendency and variation are not necessarily correlated with each other ○ Correlational Data, Scatterplots, Stage 3: 39. “Data” as plural, how to use it grammatically “Data were collected” NOT “data was collected” 40. Measures of central tendency and variation, what larger vs. smaller values mean, how they are affected by outliers Central tendency measures ○ Mean (average value) ←very affected by outliers ○ Median (middle value) ○ Mode (most frequent value) Measures of variability: range (highest-lowest, affected by outliers), st. deviation, standard error of deviation ○ Larger value = more variable data ○ Range depends on sample size; smaller n, less stable the estimate? 41. Be able to identify all elements of IVs & DVs (construct? manipulated or measured? levels? operational definition?) from a description of research 42. Be able to identify the type of claim (frequency, association, causal) based on a description of an original study or popular media story 43. Four validities (construct, internal, external, and statistical validities): definitions, how to interrogate (evaluate) them for observational studies and association/causal claims, from a description of a study Observational studies ○ Construct validity - interrater reliability, clear coding manuals and operational definitions, ○ Internal validity - N/A ○ External - random sampling? ○ Statistical - consider strength, precision, replication, outliers, restriction of range association/causal claims: ○ Construct validity 44. Effect size: what it is, how to identify and interpret it for correlational research Effect size - correlation between 2 variables in correlational research, refers to r 45. 95% CIs, what they are, how to interpret and apply them Confidence interval - captures degree of uncertainty ○ Very large ranges are imprecise (likely due to lower sample size - less stable) ○ 95% CI: “There is a 95% probability that this CI includes/captures the true population correlation” 46. Third variable problem / spurious correlations: what it is, be able to identify it in a description of research Occurs when trying to make a causal inference from an association (cannot be done) ○ Third-variable problem - to be a plausible third variable, must relate to both variables under consideration (ex. - ice cream sales and graffiti) → association does not imply causation ○ Spurious association - bivariate correlation is there only because of a third variable, such as gender (if you take this third variable out, association disappears) 47. Be able to identify all elements of IVs & DVs (construct? levels? operational definition?) from a description of research In experiments, levels = conditions of the independent variable Independent variable - what’s manipulated 48. Control variables Vary only one thing at a time; factors that are purposely held constant across all conditions (ex. - time of day that experiment is being done, scale being used to categorize behavior, etc.) Not the same thing as control condition/group →control group = ‘no treatment’ condition (for ex, placebo group) 49. Confounds and internal validity Confounds = alternative explanations for the results of an experiment other than the manipulated variable Threatens internal validity (whether alternate explanations are controlled for) 50. Design confounds Design confound - experimenter’s mistake in designing the independent variable; occurs when a second variable happens to vary systematically along with the intended independent variable Accidental second variable = alternative explanation for results Classic threat to internal validity → if an experiment has design confounds, it lacks internal validity and cannot support a causal claim Examples of design confounds: ○ if adult models in baby effort condition had been systematically more cheerful than the adult models in the no effort condition, this second variable (model’s cheerfulness) would have systematically varied along with independent variable (effort vs. no effort) ○ If test for laptop group was harder than the test for the longhand notes group, test difficulty would be a design confound that explains results of study Control for design confounds by varying only 1 factor at a time (control variables) 51. Systematic vs unsystematic variability To be a design confound, a variable must show systematic variability with the independent variable ○ Unsystematic variability (random) across both groups is not a confound ○ Unsystematic variability can lead to other problems, but it’s not considered a design confound 52. Selection effects, how they can be avoided Selection effects - occur when kinds of participants in 1 level/condition of independent variable are systematically different from those in the other; contributes to systematic variability ○ Ex. - more motivated participants sign up for the test prep course Can be avoided by: ○ Random assignment - each participant has equal chance of being selected for each condition Deliberately unsystematic! Creates situation in which experimental groups will become virtually equal before independent variable is applied ○ Matched group - more hands on; participants are sorted from lower to higher on some variable & groups into sets of 2 Individuals within each set are randomly assigned to 2 experimental groups Ensures groups are equal on some important variable Disadvantage: extra step, more time/resources 53. Order effects, how they can be avoided Order effects - happen when being exposed to 1 condition first changes how participants react to later condition (i.e., get better or get worse because of practice/exhaustion) ○ Example of within groups design confound Types of order effects: ○ Practice effects (AKA fatigue effects) Long sequence might lead participants to get better at task or get tired/bored towards end ○ Carryover effects - some form of “contamination” carries over from one condition to the next (ex. - during OJ after brushing teeth affects perception of OJ’s taste) Avoid order effects by counterbalancing - researchers present levels of IV to participants in different sequences ○ Any order effects should theoretically cancel each other out when all data are combined ○ Cannot eliminate order effects altogether ○ Different types of counterbalancing depending on whether its a complete or incomplete RMD (repeated measures design) 54. Random assignment, matched groups - how? when? does matched groups involve random assignment? Random assignment used to counteract group differences Matched groups is preferable when there’s a small sample size (ex. - with age, match 2 oldest and 2 youngest and randomly assign one from each pair to each condition) →matched group involves random assignment 55. Three criteria for making a causal claim: be able to evaluate them from a description of research 3 criteria for making a causal claim: ○ Covariance (statistically, one 1 variable must correlate with another) ○ Internal validity (are confounds ruled out? Random assignment/matching necessary to control for selection effects. Control for order effects by counterbalancing if using within groups design) ○ Temporal precedence (treatment must come before the cause) 56. Three stages of data analysis Stage 1: get to know your data Stage 2: summarize the data → descriptive statistics, measures of central tendency Stage 3: confirm what the data reveal → inferential statistics: confidence intervals and/or Null Hypothesis Significance Testing (NHST) (p-values - must be less than 0.05 to be considered significant) 57. Confirming what data reveal using confidence intervals and NHST Confidence intervals: range of values, specifying how confident we want to be that CI includes true population mean (i.e., 95% confident) ○ Based on sample means, standard deviation, and sample size (higher sample size →narrower CI) ○ “New statistics”: research questions are estimates based on “how much?”, “to what extent?” ○ F-value NHST = Null Hypothesis Significance Testing ○ Binary, yes/no terms (is there a significant difference between groups or not?) ○ p-value 58. 95% CIs: how to interpret them and how to use them in data analysis Interpreting 95% CI: there is a.95 probability that CI includes (captures) the true population mean ○ We cannot claim that population mean falls in CI (unmoveable, we need to capture it) Comparing 2 means tells us whether they seem different; need to compare CIs to determine extent to which they differ ○ If CIs for 2 samples overlap, we cannot be confident that groups differ ○ If CIs do overlap, we can be 95% confident that groups do differ (i.e., sample means are estimating from different populations) ○ 2 means on their own CANNOT be used to determine whether 2 groups differ reliably (there could be too much variability) In results section, write results statistically and behaviorally and reference the hypothesis 59. Null hypothesis testing (know the three sub-steps) Ho = Null hypothesis = there is no effect of writing condition in the population Ha = Alternative hypothesis = there is an effect of writing condition in population (often includes direction of difference as well) NHST Steps: 1. Assume there is no effect of my IV on the population (Ho) How big of a difference might I expect from chance alone? (‘expected’ test statistics → based on laws of probability) 2. What’s the probability of getting my result (‘observed’ test statistic) or one even more extreme if the null is true (i.e., no effect of IV)? This is your p-value! 3. Based on the p-value, make a conclusion about IV a. If p>= 0.05, fail to reject the null hypothesis b. If p 1 ○ Higher F means groups’ means are fairly different from each other (>2.0 or 3.0) 61. Statistically significant effects: be able to identify and describe them, including your evidence (know how to interpret the F-statistic and p-value from an ANOVA) Higher F means groups’ means are fairly different from each other (>2.0 or 3.0) P-value < 0.05 → statistically significant difference between groups (less than.05 chance that we would get these results and there is no statistically significant difference between the groups) ○ P-value = probability of getting result of that magnitude (or more extreme) if null hypothesis is true ○ Rejecting null hypothesis means the result is statistically significant 62. Effect size; how to interpret the eta squared from an ANOVA Following up on significant NHST: How large was the effect of IV? (effect size) Which condition differed from which? What was the direction? Effect size - strength of association btw. IV and DV ○ Independent of sample size ○ r - correlation coefficient → IV & DV are quantitative variables ○ Cohen’s d - IV is categorical, 2 levels (i.e., comparing means of 2 groups) ○ Eta squared - IV is categorical, more than 2 groups (i.e., comparing means of 3+ groups); used with ANOVA Ranges from 0 to 1 small :.01 Medium:.06 (must surpass.06 to be considered medium) Large:.14+ 63. Type I (false positive) and type II (miss) errors Type I error = false positive; claiming an outcome is statistically significant when null hypothesis is actually true Type II error = miss; claiming an outcome is not statistically significant when null hypothesis is actually false 64. Individual differences, effect they can have on type I/type II errors Large variability within groups could obscure any true effects of IV, leading to Type II error Large variability in individual differences between groups might lead us to make a Type I error (false positive) → control for by using random assignment/matched pair 65. Limits (“fine print”) of statistical analysis Statistical analyses are very strong, but they cannot tell us ○ Whether confounding variables are present ○ Whether results are meaningful / important (statistically vs practically significant) ○ Whether IV did not have an effect (cannot confirm that there’s no significant effect – not enough evidence) ○ Whether we can be certain when we draw a conclusion Best test is replication 66. Between-groups (independent) vs within-groups designs Between-groups (independent) design: participants experience only ONE condition (level) of the IV ○ 2 types: post test-only & posttest-pretest ○ IV = “between subjects factor” Within-groups design: participants experience ALL levels of an IV ○ Concurrent measures (exposed to each condition at the same time; single attitudinal or behavioral preference is the DV - rare) ○ Repeated measures design (RMD) - participants are measured on a DV more than once, after exposure to EACH level of IV 67. Types of IGDs: Posttest only, pretest-posttest 68. Types of within-groups designs: Concurrent, RMDs (see above) 69. Sources of variation in IGD vs RMD - More within subjects variation in IGD than RMD (same participants in RMD) 70. Advantages & disadvantages of RMDs (vs. IGDs) Advantages of RMDs: ○ Subtracting the within ‘subjects’ variation from calculations of the F statistic makes dependent variable more sensitive to IV ○ More powerful → better able to detect statistically significant effect of IV on DV (if effect exists) ○ No confounding by individual differences Participants in your groups are equivalent because they are the same participants and serve as their own controls ○ More efficient → requires less participants overall (especially helpful when there’s 2+ conditions) ○ More sensitive - gives researchers more power to notice differences between conditions Disadvantages of RMDs: ○ Potential for order effects (solution = counterbalancing) ○ Might not be practical or possible (ex. - learn a skill like riding a bike from Method A vs Method B) ○ Experiencing all levels of an IV may change the way that participants act Demand characteristics - participants guess the hypothesis (similar to observer effects) Do NOT use within groups design if this is likely 71. Statistical power in RMD vs IGD RMD give researchers more statistical power (ability to detect statistical significance if an effect exists) than IGD because more precise estimates can be made between conditions →participants are the same in each condition, act as their own controls ○ Treating each participant as his/her own control (also means matched group design can be considered within group design) 72. Internal validity threats with IGDs, RMDs Internal validity threats with IGDs ○ Design confounds ○ Selection effects Internal validity threats with RMDs: ○ Design confounds (NOT selection effects) ○ Order effects (practice effects, carryover effects) 73. Complete vs incomplete RMDs Complete RMDs: participants experience each level more than once Incomplete RMDs: participants experience each level only 1 time 74. Counterbalancing methods and when to use each Counterbalancing only used in within groups designs, when each participant is exposed to more than 1 condition Used to counteract order effects Complete RMD - participants are exposed to each condition more than once ○ ABBA counterbalancing →present the conditions in one random sequence (ABCD) followed by the opposite/mirror/reverse of that sequence (DCBA) Participant 1: ABCDDCBA Participant 2: DBACCABD Avoid using this method if it’s easy to pick up on pattern in the trials (anticipation effects); need even(?) number of repetitions to do it ○ Block randomization → only possible in complete design because you need more than 1 block Each block = all conditions once, in random order Block size = number of conditions # of blocks = # of times participants receives each condition Each block is 4 trials; within each block, you randomly shuffle the order If you have enough blocks and enough participants, the randomization should counteract any order effects (which condition occurs first versus last) Incomplete repeated measures design (each participant experiences each condition only once): ○ Full counterbalancing: All possible orders are represented Simple when only 2-3 conditions ex. : 2*1 = 2 possible orders 3 * 2 * 1 = 6 possible orders (ABC, BAC, CAB, ACB, BCA, CBA) >3 requires a lot of participants Need # of participants to be the same as * of orders ○ Partial counterbalancing → Latin Squares Preferred for 4 or more conditions Each condition appears in each condition at least once Each condition precedes and follows every other participants Method of selecting Selected orders (partial counterbalancing): randomize order of conditions for every subject Preferred when there are very many conditions! 75. Full vs partial counterbalancing Full counterbalancing: All possible orders are represented (ABBA or randomly assign to each possible order) Partial counterbalancing → only some of possible condition orders are represented (ex. - present conditions in randomized order for every subject) or use Latin Squares or block randomization (block randomization doesn’t ensure that all possible orders are necessarily represented, because orders are random within each block) 76. What is randomly assigned in an IGD vs a RMD? In an IGD: participants are randomly assigned to a level of the IV (condition) In a RMD: participants are randomly assigned to an order of receiving each condition 77. Three possible interpretations of null effects Perhaps there is not enough between groups difference ○ Possibilities for not enough between groups difference: Weak manipulation - maybe there’s not a big enough difference between green and red; perhaps $1 vs $5 is not enough Insensitive measures: ‘are you happy?’ → yes/no might not be a precise enough way to measure happiness ceiling/floor effects - poorly designed IV or DV If everyone in both conditions is scoring very high (ceiling) or very low (floor), maybe the scale is not detecting any difference. Design confound working in reverse An unintended variable goes along with the IV: maybe increased anxiety is associated with taking the test prep course, so this obscures any improvements that taking the course does have. Perhaps within-group variability obscured the significant difference (means are very different, but CIs are very wide and don’t overlap) ○ Possible causes of too much within-group variability: measurement error, individual differences, situation noise Perhaps there really is no difference 78. What could cause a lack of between-groups difference that might produce a null effect? Solutions? Potential causes for lack of between-groups difference: Weak manipulation → poorly designed IV (e.g., ‘some’ = $1, ‘a lot’ =$5 vs ‘some’ = $5 vs ‘a lot’ = $50 Insensitive measures → poorly designed DV (binary questions, no opportunity for nuance) Ceiling effects (all scores squeezed together at high end) and floor effects (all scores cluster at low end) ○ Result of either poorly designed IV or DV ○ Example of ceiling effect of IV: researchers threaten participants with either 10, 30, or 50 volts of electricity → all lead to very high anxiety scores ○ Example of floor effect on DV: researchers measured logical reasoning ability with very hard test (all scores were low) Design confound acting in reverse ○ Some other variable may be working in opposite direction that counteracts effects of IV Solutions: Make sure the manipulation is strong; manipulation checks Are there meaningful differences between the levels of the DV and the IV? 79. How could within-groups variability obscure group differences and produce a null effect? Solutions? Possible causes of too much variability within groups: ○ Measurement error (e.g., heigh, angle of vision, slouching) Solution: use measurement tools that are reliable and precise Measure MORE instances so random errors cancel out ○ Individual differences (spread out scores within each group → some people are naturally more happy or more sad) Solution: change decision to within-groups or matched groups to decrease CI and SD (e.g., pair people with similar levels of happiness and split pairs between groups) ○ Situation noise - any kind of external distraction that could cause variability within groups that obscures between groups differences Example - outside noise, sights, odors Solution: minimize by controlling the experiments' surroundings (quiet room, no interesting posters on wall) ____ One of the reasons you might choose to do within-groups design is that repeated group designs have more power to notice differences - Power = ability to detect a statistically significant difference (if an effect exists) - 2 sources of variation in DV: - Variation within groups (within groups = same individuals = less variation within) - Variation between groups Within groups design - includes concurrent measures design (experiencing more than 1 condition all at one time) and repeated measures design First unit - validities, observation Second unit - Association, Correlation Third unit - Experimentation ←questions are more weighted towards third unit