toz268.pdf
Document Details
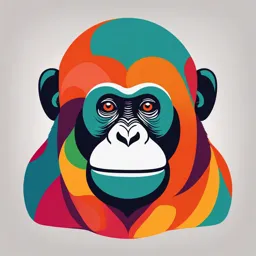
Uploaded by HardWorkingAgate2270
2020
Tags
Full Transcript
Journal of Economic Entomology, 113(1), 2020, 1–25 doi: 10.1093/jee/toz268...
Journal of Economic Entomology, 113(1), 2020, 1–25 doi: 10.1093/jee/toz268 Advance Access Publication Date: 7 December 2019 Review Review Drones: Innovative Technology for Use in Precision Pest Management Fernando H. Iost Filho,1, Wieke B. Heldens,2 Zhaodan Kong,3 and Elvira S. de Lange4, Downloaded from https://academic.oup.com/jee/article/113/1/1/5666881 by Anand Agricultural University user on 26 September 2024 1 Department of Entomology and Acarology, University of São Paulo, Piracicaba, São Paulo, Brazil, 2German Aerospace Center (DLR), Earth Observation Center, German Remote Sensing Data Center (DFD), Oberpfaffenhofen, D-82234 Wessling, Germany, 3Department of Mechanical and Aerospace Engineering, University of California Davis, 2094 Bainer Hall, Davis, CA 95616, 4Department of Entomology and Nematology, University of California Davis, 1 Shields Avenue, 367 Briggs Hall, Davis, CA 95616, and 5Corresponding author, e-mail: [email protected] Subject Editor: Silvia Rondon Received 4 February 2019; Editorial decision 10 September 2019 Abstract Arthropod pest outbreaks are unpredictable and not uniformly distributed within fields. Early outbreak detec- tion and treatment application are inherent to effective pest management, allowing management decisions to be implemented before pests are well-established and crop losses accrue. Pest monitoring is time-consuming and may be hampered by lack of reliable or cost-effective sampling techniques. Thus, we argue that an im- portant research challenge associated with enhanced sustainability of pest management in modern agricul- ture is developing and promoting improved crop monitoring procedures. Biotic stress, such as herbivory by arthropod pests, elicits physiological defense responses in plants, leading to changes in leaf reflectance. Advanced imaging technologies can detect such changes, and can, therefore, be used as noninvasive crop monitoring methods. Furthermore, novel methods of treatment precision application are required. Both sensing and actuation technologies can be mounted on equipment moving through fields (e.g., irrigation equipment), on (un)manned driving vehicles, and on small drones. In this review, we focus specifically on use of small unmanned aerial robots, or small drones, in agricultural systems. Acquired and processed canopy re- flectance data obtained with sensing drones could potentially be transmitted as a digital map to guide a second type of drone, actuation drones, to deliver solutions to the identified pest hotspots, such as precision releases of natural enemies and/or precision-sprays of pesticides. We emphasize how sustainable pest management in 21st-century agriculture will depend heavily on novel technologies, and how this trend will lead to a growing need for multi-disciplinary research collaborations between agronomists, ecologists, software programmers, and engineers. Key words: biological control, integrated pest management, precision agriculture, remote sensing, unmanned aerial system Arthropod pest outbreaks in field crops and orchards often show DiFonzo 2007, Amtmann et al. 2008, West and Nansen 2014). Thus, nonuniform spatial distributions. For some pests, such as cab- as pests are spatially aggregated, precision agriculture technologies bage aphids [Brevicoryne brassicae L. (Hemiptera: Aphididae)] in can offer important opportunities for integrated pest management canola fields (Brassica spp.), and Asian citrus psyllids [Diaphorina (IPM) (Lillesand et al. 2007). citri Kuwayama (Hemiptera: Liviidae)] in citrus orchards (Citrus Precision pest management is twofold: first, reflectance-based spp.) there is evidence of highest population densities along field crop monitoring (using ground-based, airborne, or orbital remote edges (Sétamou and Bartels 2015, Severtson et al. 2015, Nguyen sensing technologies) can be used to identify pest hotspots. Second, and Nansen 2018). For other pests, such as soybean aphids [Aphis precision control systems, such as distributors of natural enemies glycines Matsumura (Hemiptera: Aphididae)] in soybean (Glycine and pesticide spray rigs, can provide localized solutions. Both tech- max (L.) Merrill), and two-spotted spider mites [Tetranychus nologies can be mounted on equipment moving through fields (such urticae Koch (Acari: Tetranychidae)] in cowpea (Vigna unguiculata as irrigation equipment), on manned or unmanned vehicles driving (L.) Walp.), parts of fields that are exposed to abiotic stress, such around in fields, or on aerial drones. as drought or nutrient deficiencies, tend to be more susceptible In this review, we focus specifically on the use of small drones (Mattson and Haack 1987, Abdel-Galil et al. 2007, Walter and in IPM. Small drones are here defined as remotely controlled, © The Author(s) 2019. Published by Oxford University Press on behalf of Entomological Society of America. All rights reserved. 1 For permissions, please e-mail: [email protected]. 2 Journal of Economic Entomology, 2020, Vol. 113, No. 1 unmanned flying robots that weigh more than 250 g but less than although there is a big margin among predictions of future drone 25 kg, including payload (FAA 2018a). These types of drones typic- use, an increasing number of growers is expected to use and/or own ally have flight-times of a few minutes to hours and limited ranges a drone within the next decade. (Hardin and Jensen 2011). We will also briefly discuss the larger There are various ways to classify drones (Watts et al. 2012). drones that are typically used for pesticide sprays. Discussion of For our purpose, we currently distinguish two major types of smaller and larger drones is beyond the scope of this review, but small drones: rotary wing and fixed wing. Each of these has its see Watts et al. (2012), and Anderson and Gaston (2013) for more own advantages and limitations (Hogan et al. 2017). Multi-rotor information. Drones used for detection of pest hotspots are here and single-rotor (helicopter) drones do not require specific struc- referred to as sensing drones, while drones used for precision distri- tures for take-off and landing. Moreover, they can hover and per- bution of solutions are referred to as actuation drones. Both types form agile maneuvering, making them suitable for applications of drones could communicate to establish a closed-loop IPM solu- (e.g., inspection of crops and orchards or pesticide applications) Downloaded from https://academic.oup.com/jee/article/113/1/1/5666881 by Anand Agricultural University user on 26 September 2024 tion (Fig. 1). Importantly, use of drones in precision pest manage- where precise maneuvering or the ability to maintain a visual of a ment could be cost-effective and reduce harm to the environment. target for an extended period of time is required. Especially multi- Sensing drones could reduce the time required to scout for pests, rotor drones tend to be easy to use, and relatively cheap to obtain. while actuation drones could reduce the area where pesticide ap- Fixed-wing systems are usually faster than rotor-based systems, plications are necessary, and reduce the costs of dispensing natural and generally larger in size, allowing for higher payloads (Stark enemies. et al. 2013b, Dalamagkidis 2015). Both have been used for preci- Reports of drones in agriculture started appearing around 1998 sion agriculture (Barbedo 2019). Since drone technology quickly and increased dramatically in the last decade (Fig. 2). According to improves, we will refrain from discussing drone types in further the abstract of a licensed report, the worldwide drone market value detail, but see Dalamagkidis (2015) and Stark et al. (2013b) for is currently estimated about $6.8 billion and is anticipated to reach more information. $36.9 billion by 2022 (WinterGreen Research 2016b). Another A number of reviews discuss the use of drones in precision paid report predicts that drones will reach a value of $14.3 billion agriculture, focusing on airborne remote sensing for various ap- by 2028 (Teal Group 2019). Agricultural small drones currently plications, such as predicting yield and characterizing soil proper- account for about $500 million, and their value is expected to ties (Hardin and Jensen 2011, Prabhakar et al. 2012, Zhang and reach $3.7 billion by 2022 (WinterGreen Research 2016a). A dif- Kovacs 2012, Mulla 2013, Gago et al. 2015, Nansen and Elliott ferent paid report predicts similar values (ABI Research 2018), 2016, Pádua et al. 2017, Hunt and Daughtry 2018, Aasen et al. while a freely available resource predicts the value of drone-based 2018, Gonzalez et al. 2018, Barbedo 2019, Maes and Steppe 2019). solutions for agriculture at $32 billion (PwC 2016). Recently, the In this review, we focus on precision management of arthropod pests United Nations published a report on the use of drones for agri- and describe the use of both sensing and actuation drones. First, we culture, stressing its potential benefits for food security (Sylvester provide an update about airborne remote sensing-based detection 2018). A text message poll among ca. 900 growers based in the of pest problems. Then, we evaluate the possibilities of actuation United States showed that around 30% use drone-based tech- drones for precision distribution of pesticides and natural enemies. nology for farming practices (Farm Journal Pulse 2019). Thus, Also, we discuss the possibilities of sensing and actuation drones for Fig. 1. (a) State-of-the-art open-loop remote sensing paradigm and (b) closed-loop IPM paradigm envisioned in this article. Sensing drones could be used for detection of pest hotspots, while actuation drones could be used for precision distribution of solutions. Adapted from Teske et al. (2019). Journal of Economic Entomology, 2020, Vol. 113, No. 1 3 light, and infrared light) (Usha and Singh 2013). It is a non-invasive, relatively labor-extensive method that could be used to detect plant stress before changes are visible by eye. For crops, remote sensing equipment generally assesses the spectral range of visible light or photosynthetically active radiation (PAR, 400–700 nm) and near- infrared light (NIR, 700–1,400 nm), with most studies referring to the 400–1,000 nm range (Nansen 2016). Particular stressors, such as arthropod infestations, induce physiological plant responses, causing changes in the plants’ ability to perform photosynthesis, which leads to changes in leaf reflectance in parts of this spectral range. For aerial remote sensing, a drone can be equipped with an RGB (red green Downloaded from https://academic.oup.com/jee/article/113/1/1/5666881 by Anand Agricultural University user on 26 September 2024 blue) sensor, a multispectral sensor with between 3 and 12 broad spectral bands, or a hyperspectral sensor with hundreds of narrow spectral bands. An RGB sensor is low-cost, but results in limited spectral infor- mation. A multispectral sensor results in more spectral information, but a hyperspectral sensor is generally much better at differentiating subtle differences in canopy reflectance than a multispectral sensor Fig. 2. Number of articles published between 1998 and 2018 on the use of drones in agriculture. Shown is the number of publications for each year (Yang et al. 2009a). However, since hyperspectral sensors are gen- mentioning ‘drone’, ‘UAV’ (Unmanned Aerial Vehicle), or ‘UAS’ (Unmanned erally larger, they would require mounting on drones adapted for Aerial System) and ‘agriculture’. The words ‘bee’, ‘honey bee’, and ‘hive’ heavier payloads. Also, they are generally more expensive, and data were explicitly excluded from the search, to avoid including publications on analysis requires more time and experience, limiting use for indi- drones defined as male bees. Source: Web of Science. vidual growers. A comprehensive review of the sensor types compat- ible with drones has been written by Aasen et al. (2018). novel functions in pest management. Lastly, we discuss challenges and opportunities in the adoption of drone technology in modern Remote Sensing and Arthropod Pests agriculture. Remote sensing technologies have been used in precision agricul- ture for the last few decades, with various applications, such as yield predictions and evaluation of crop phenology (Mulla 2013). Sensing Drones to Monitor Crop Health Also, these techniques are being used to monitor different abi- Traditional field scouting for pest infestations is often expensive otic plant stressors, such as drought (Gago et al. 2015, Katsoulas and time-consuming (Hodgson et al. 2004, Severtson et al. 2016b, et al. 2016, Zhao et al. 2017, Jorge et al. 2019) and nutritional Dara 2019). It may be practically challenging, such as when a large deficiencies (Quemada et al. 2014), and biotic plant stressors, such acreage is involved, when the arthropod pests are too small to see as pathogens (Calderón et al. 2013, Mahlein et al. 2013, Zarco- with the naked eye, or when they reside in the soil or in tall trees. Tejada et al. 2018), nematodes (Nutter et al. 2002), and weeds In some cropping systems, effective scouting is hampered by lack of (Rasmussen et al. 2013, Peña et al. 2015). Likewise, remote sensing reliable pest sampling techniques. Hence, one of the main drivers for technologies have been successfully used to detect stress caused the implementation of drone-based remote sensing technologies into by various arthropod pests on a wide variety of field and orchard agriculture is the potential time saved by automatizing crop moni- crops (Riley 1989, Nansen 2016, Nansen and Elliott 2016; Tables toring, making the technology cost-effective for growers (Carrière 1–4). A limited amount of studies concerning arthropod-induced et al. 2006, Backoulou et al. 2011a, Dara 2019). stress detection used drone-based aerial remote sensing (Table 1), Compared to conventional platforms for remote sensing, such as manned aircraft-based aerial remote sensing (Table 2), or orbital ground-based, aerial (with manned aircraft) and orbital (with sat- remote sensing (Table 3), while most studies used ground-based ellites such as Landsat [30 m spatial resolution], Sentinel 2 [10 m] remote sensing (Table 4). or RapidEye [5 m]; Mulla 2013), sensing drones present several ad- In these tables, optical sensors are grouped, in addition to the vantages that make them attractive for use in precision agriculture. platform, they are mounted on, into RGB, multispectral, and Sensing drones potentially allow for coverage of larger areas than hyperspectral sensors. As stated above, generally, multispectral ground-based, handheld devices. They can fly at lower altitudes than sensors have 3–12 broad spectral bands at selected wavelength manned aircraft and orbital systems, increasing images’ spatial reso- ranges, whereas hyperspectral sensors have many (usually >20, lution and reducing the number of mixed pixels (pixels representing but up to several hundreds) narrow, contiguous spectral bands, ac- reflectance of both plant and soil, discussed in more detail below). quiring the spectrum within the selected spectral region with many Also, they cost less to obtain and deploy than manned aircraft and measurement points. However, there is no clear agreed on definition. satellites and do not have long revisiting times like satellites, al- Therefore, the tables include multispectral sensors acquiring more lowing for higher monitoring frequencies (Zhang and Kovacs 2012, than 12 spectral bands. While grouping the sensors, we adhered to Mulla 2013, Matese et al. 2015, Aasen and Bolten 2018, Barbedo the authors’ classifications (Tables 1–4). 2019, Maes and Steppe 2019). Tables 1–4 focus on detection of arthropod pests; we did not ad- dress diseases caused by arthropod vectors (e.g., Garcia-Ruiz et al. Remote Sensing in Precision Agriculture 2013). Also, these tables only contain studies related to crops and Remote sensing is the detection of energy emitted or reflected by orchards. We did not address forestry studies, as the body of lit- various objects, either in the form of acoustical energy or in the form erature on pest detection involves multi-species forests, adding an of electromagnetic energy (including ultraviolet [UV] light, visible additional layer of complexity as opposed to crops and orchards 4 Table 1. Studies on drone-based hyperspectral, multispectral, and RGB remote sensing to detect arthropod-induced stress in crops and orchards Plant Platform Spectral No. of spec- Field common Arthropod Arthropod details Type resolutiona Sensor details tral bands observations name Plant species common name species Order: Family References md4-1000, Four rotors RGB α ILCE-5100L with 3 Visual in- Grape Vitis vinifera L. Cotton jassid Jacobiasca lybica Hemiptera: Del-Campo- Microdrones an E 20 mm F2.8 spection of Bergevin and Cicadellidae Sanchez lens, Sony images Zanon et al. 2019 Aeryon Scout, Four rotors RGB + M Photo3S, Aeryon 3+3 Outbreak Wheat Triticum aestivum Fall armyworm Spodoptera Lepidoptera: Zhang et al. Aeryon Labs Inc. Labs Inc. + ADC- reported by frugiperda Smith Noctuidae 2014 Lite, Tetracam Inc. grower S800 EVO, SZ DJI Six rotors RGB + M + H 5DsR, Canon Inc. 3 + 5 + 274 Ground traps Grape Vitis vinifera Grape phylloxera Daktulosphaira Hemiptera: Vanegas et al. Technology Co. + RedEdge, and root vitifoliae Fitch Phylloxeridae 2018a MicaSense Inc. + digging, Nano- visual vigor Hyperspec, assessments Headwall Pho- tonics Inc. S800 EVO, SZ DJI Six rotors / RGB + M + 5DsR, Canon Inc. 3 + 5 + 274 Ground traps Grape Vitis vinifera Grape phylloxera Daktulosphaira Hemiptera: Vanegas et al. Technology Co. four ro- H / RGB + RedEdge, /3 and root vitifoliae Phylloxeridae 2018b / Phantom3 Pro, tors MicaSense Inc. + digging, SZ DJI Tech- Nano- visual vigor nology Co. Hyperspec, assessments Headwall Pho- tonics Inc. / Phantom3 Pro as- sociated camerab eBee, senseFly Fixed wing M S110 NIRb, Canon 3 NA Onion Allium cepa L. Thrips NA Thysanoptera: Nebiker et al. Thripidae 2016 Cinestar-8 MK Eight rotors M Mini-MCA6, 6 Arthropod Canola Brassica spp. Green peach aphid Myzus persicae Hemiptera: Severtson Heavy Lift, Tetracam Inc. counts, Aphididae et al. 2016a Freefly Systems soil and plant tissue nutrient analysesc Matrice 100, SZ Four rotors M ADC-Lite, Tetracam 3 Damage as- Cotton Gossypium Two-spotted Tetranychus urticae Acari: Tetranychidae Huang et al. DJI Technology Inc. sessments hirsutum L. spider mite 2018 Co. Spreading Wings Six rotors M Mini-MCA6, 6 Damage as- Potato Solanum Colorado potato Leptinotarsa Coleoptera: Hunt and S800, SZ DJI Tetracam Inc. sessments tuberosum beetle decemlineata Chrysomelidae Rondon Technology Co. 2017, Hunt et al. 2017 eBee, senseFly Fixed wing M S110 NIRb, Canon 3 Arthropod Sorghum Sorghum bicolor Sugarcane aphid Melanaphis sacchari Hemiptera: Stanton et al. counts Aphididae 2017 a RGB = red green blue, M = multispectral, H = hyperspectral. b NIR = near infrared. c Remote sensing was used to detect nutrient deficiencies, which were correlated to arthropod presence. NA = information not provided. Journal of Economic Entomology, 2020, Vol. 113, No. 1 Downloaded from https://academic.oup.com/jee/article/113/1/1/5666881 by Anand Agricultural University user on 26 September 2024 Table 2. Studies on aerial (manned aircraft) hyperspectral and multispectral remote sensing to detect arthropod-induced stress in crops and orchards Spectral No. of spectral Plant common Arthropod common resolutiona Sensor details bands Field observations name Plant species name Arthropod species Order: Family References M K-17, Fairchild Camera NA Arthropod counts, sooty Citrus Citrus spp. Citrus blackfly Aleurocanthus woglumi Hemiptera: Aleyrodidae Hart et al. 1973 and Instrument Corp. + mold assessmentsb Ashby Hasselblad camera M System composed of 3 video 3 Visual inspections, sooty Citrus Citrus spp. Citrus blackfly Aleurocanthus woglumi Hemiptera: Aleyrodidae Everitt et al. 1994 cameras mold assessmentsb M K-17, Fairchild Camera and NA Arthropod counts, sooty Citrus Citrus spp. Brown soft scale Coccus hesperidum L. Hemiptera: Coccidae Hart and Meyers Instrument Corp. mold assessmentsb 1968 M System composed of 3 video 3 Visual inspections, sooty Cotton Gossypium hirsutum Silverleaf whitefly Bemisia tabaci Hemiptera: Aleyrodidae Everitt et al. 1996 cameras mold assessmentsb M MS2100, DuncanTech 3 Arthropod counts Cotton Gossypium hirsutum Beet armyworm Spodoptera exigua Hübner Lepidoptera: Noctuidae Sudbrink et al. 2003 M CRSP, NASA 3 Sweep net sampling, drop Cotton Gossypium hirsutum Tarnished plant Lygus lineolaris Palisot de Hemiptera: Miridae Willers et al. 1999 cloth sampling bug Beauvois M RDACS, ITDc, Stennis Space 3 Sweep net sampling Cotton Gossypium hirsutum Tarnished plant bug Lygus lineolaris Hemiptera: Miridae Willers et al. 2005 Journal of Economic Entomology, 2020, Vol. 113, No. 1 Center M MS3100, DuncanTech 3 Damage assessments Sorghum Sorghum bicolor Sugarcane aphid Melanaphis sacchari Hemiptera: Aphididae Elliott et al. 2015; Backoulou et al. 2018a, b M MS3100, DuncanTech 3 Visual inspections Wheat Triticum aestivum Russian wheat Diuraphis noxia Hemiptera: Aphididae Backoulou et al. aphid 2011a,b, 2013, 2016 M SSTCRIS, SST Development 3 Proportion of infested Wheat Triticum aestivum Russian wheat Diuraphis noxia Hemiptera: Aphididae Elliott et al. 2007 Group Inc. plants aphid M TerrAvion 2 Arthropod counts Wheat Triticum aestivum Hessian fly Mayetiola destructor Say Diptera: Cecidomyiidae Bhattarai et al. 2019 M MS3100, DuncanTech 3 Arthropod counts or Wheat Triticum aestivum Greenbug Schizaphis graminum Hemiptera: Aphididae Elliott et al. 2009; visual inspection Backoulou et al. 2015, 2016 M CASI, Borstad Associates + 4-8d Root digging Grape Vitis vinifera Grape phylloxera Daktulosphaira vitifoliae Hemiptera: Lobits et al. 1997 EO Camera, NASA ARCd Phylloxeridae M+H SAMRSS + AVNIR, Opto- 4 + 60 Arthropod counts Cotton Gossypium hirsutum Cotton aphid Aphis gossypii Hemiptera: Aphididae Reisig and Godfrey Knowledge Systems 2006, 2010 M+H SAMRSS + AVNIR, Opto- 4 + 60 Arthropod counts Cotton Gossypium hirsutum Spider mite Tetranychus spp. Acari: Tetranychidae Reisig and Godfrey Knowledge Systems 2006 H AVIRIS, NASA 224 Arthropod counts Cotton Gossypium hirsutum Strawberry spider Tetranychus turkestani Acari: Tetranychidae Fitzgerald et al. 2004 mite Ugarov and Nikolskii H AISA, Specim Spectral Im- 50 Visual inspection of Wheat Triticum aestivum Russian wheat Diuraphis noxia Hemiptera: Aphididae Mirik et al. 2014 aging Ltd. images aphid H RDACS-H4, ITDc, Stennis 120– Damage assessments Corn Zea mays European corn Ostrinia nubilalis Lepidoptera: Carroll et al. 2008 Space Center 240 borer Crambidae a M = multispectral, H = hyperspectral. b A fungus not infesting the plant, but growing on the arthropod’s sugary honeydew secretions. c Institute of Technology and Development. 5 d Primary project sensors; five additional sensors were used with 3–8 spectral bands. NA = information not provided. Downloaded from https://academic.oup.com/jee/article/113/1/1/5666881 by Anand Agricultural University user on 26 September 2024 6 Journal of Economic Entomology, 2020, Vol. 113, No. 1 Table 3. Studies on orbital multispectral remote sensing to detect arthropod-induced stress in crops No. of Plant Spectral spectral Field common Arthropod Arthropod resolutiona Sensor details bands observations name Plant species common name species Order: Family References M QuickBird, 3 Arthropod Cotton Gossypium Cotton aphid Aphis gossypii Hemiptera: Reisig and DigitalGlobe counts hirsutum Aphididae Godfrey 2006, 2010 M QuickBird, 3 Arthropod Cotton Gossypium Spider mite Tetranychus Acari: Reisig and DigitalGlobe counts hirsutum spp. Tetranychidae Godfrey 2006 Downloaded from https://academic.oup.com/jee/article/113/1/1/5666881 by Anand Agricultural University user on 26 September 2024 M Terra, MODIS, 36 Arthropod Wheat Triticum aestivum Wheat stem Cephus cinctus Hymenoptera: Lestina NASA counts sawfly Norton Cephidae et al. 2016 M Sentinel-2, S2A- 13 Arthropod Wheat Triticum aestivum Hessian fly Mayetiola de- Diptera: Bhattarai L1C, ESAb counts structor Cecidomyiidae et al. 2019 M HJ-1A/B, CCD 4 Arthropod Wheat Triticum aestivum Wheat aphid Sitobion avenae Hemiptera: Luo et al. sensor, NDRCC/ counts, Aphididae 2014 SEPAc, damage as- sessments M Landsat-8, NASA 9 Arthropod Wheat Triticum aestivum Wheat aphid Sitobion avenae Hemiptera: Ma et al. counts Aphididae 2019 M Landsat-5 TM, 7 Arthropod Wheat Triticum aestivum Aphid NA Hemiptera: Huang NASA counts Aphididae et al. 2011 M RapidEye, Planet 5 Arthropod Corn Zea mays Stem borer Busseola spp. Lepidoptera: Abdel- Labs counts Noctuidae Rahman et al. 2017 M HJ-1A/B, CCD 4 Damage assess-Corn Zea mays Oriental Mythimna Lepidoptera: Zhang sensor, NDRCC/ ments armywormd separata Noctuidae et al. SEPAc, Walkerd 2016 a M = multispectral. b European Space Agency. c National Committee for Disaster Reduction and State Environmental Protection Administration of China. d The arthropod species was originally misidentified as Spodoptera frugiperda; a correction was issued. NA = information not provided. in monoculture. More information about remote sensing in forestry by building vegetation indices (VIs). These two techniques are ad- settings can be found elsewhere (Dash et al. 2016, Pádua et al. 2017, dressed below exemplarily. An overview of techniques to quantify Stone and Mohammed 2017, Dash et al. 2018). vegetation biophysical variables using imaging spectroscopy is given It is important to note that with remote sensing, not the pests in Verrelst et al. (2019). themselves are detected, but patterns of canopy reflectance that are indicative of arthropod-induced plant stress. Field observations to Spectral Features and VIs confirm the presence of specific stressors remain necessary, but field An important spectral feature light region is the red edge, i.e., the scouting can be more efficiently focused with the a priori knowledge slope between the red and near infrared region of the spectrum, from remote sensing. around 700 nm. This spectral region relates to the chlorophyll con- centration (Horler et al. 1983, Delegido et al. 2011, Huang et al. Analysis of Reflectance Spectra 2015b) and the Leaf Area Index (LAI), the area of green leaves per For the detection of plant stress using remote sensing, the spectral unit of ground area (Delegido et al. 2013). The red edge position reflectance (the spectral signature or spectrum) of the vegetation is (REP), the point of maximum slope in the red edge region, is a valu- analyzed. Figure 3 shows a spectrum of healthy soybean leaves as re- able indicator of stress and senescence (Das et al. 2014, Verrelst et al. corded by a ground-based hyperspectral field spectrometer, together 2019), possibly because various stressors decrease leaf chlorophyll with the same spectrum resampled to the spectral resolution of a concentrations (Carter and Knapp 2001). For instance, an increased hyperspectral imaging spectrometer for drones, and a multispectral reflectance around 740 nm is associated with spider mite suscepti- sensor for drones. The figure shows the large loss of information be- bility in corn (Zea mays L.) (Nansen et al. 2013). Also, the overall tween a hyperspectral sensor and a multispectral sensor. With higher reflection level of the spectrum might be characteristic. spectral resolutions (i.e., more spectral bands), detailed spectral It should be noted that a spectrum of an imaging spectrometer, characteristics become visible and can be used to analyze vegetation such as one mounted on drones, always describes an area, not a spectra. This analysis can be done in various ways, e.g., by analyzing point. This area, or pixel size, depends on the flight height of the spectral reflectance features (e.g., absorption bands or reflectance drone and can range from less than 1 cm2 to more than 10 cm2. With peaks) that can be directly related to plant physiology, or indirectly larger pixels, the recorded spectrum consists of reflectance of both Table 4. Studies on ground-based hyperspectral and multispectral remote sensing to detect arthropod-induced stress in crops and orchards Spectral No. of spectral Field Plant common Arthropod common resolutiona Sensor details bands observations name Plant species name Arthropod species Order: Family References M 12–1000 modular-multiband 3 Visual inspections, Cotton Gossypium hirsutum Silverleaf whitefly Bemisia tabaci Hemiptera: Aleyrodidae Everitt et al. radiometer, Barnes Engin- sooty mold 1996 eering Co. assessmentsb M System composed of visible 68 Arthropod counts Cotton Gossypium hirsutum Strawberry spider Tetranychus turkestani Acari: Tetranychidae Fitzgerald et al. and NIR ‘Varispec’ liquid- mite 2004 crystal tunable-filters, Cambridge Research In- strumentation Inc. + Pluto digital camera, PixelVision Inc. M Model 505 GreenSeeker 2 Controlled in- Cotton Gossypium spp. Two-spotted spider Tetranychus urticae Acari: Tetranychidae Martin et al. optical sensor, Trimble festations or mite 2015, Martin Navigation arthropod counts and Latheef 2017, 2018 M ADC, Tetracam Inc. 3 Controlled infest- Cotton Gossypium hirsutum Two-spotted spider Tetranychus urticae Acari: Tetranychidae Lan et al. 2013 ations mite Journal of Economic Entomology, 2020, Vol. 113, No. 1 M Model 505 GreenSeeker 2 Controlled infest- Pinto bean Phaseolus vulgaris L. Two-spotted spider Tetranychus urticae Acari: Tetranychidae Martin and optical sensor, Trimble ations mite Latheef 2018 Navigation M MSR 16 radiometer, 16 Visual inspections or Wheat Triticum aestivum Russian wheat Diuraphis noxia Hemiptera: Aphididae Mirik et al. Cropscan Inc. controlled infest- aphid 2012 ations M MSR 16R radiometer, 16 Arthropod counts Wheat Triticum aestivum Russian wheat Diuraphis noxia Hemiptera: Aphididae Yang et al. Cropscan Inc. aphid 2009b M MSR 16R radiometer, 16 Arthropod counts Wheat Triticum aestivum Greenbug Schizaphis graminum Hemiptera: Aphididae Yang et al. 2005, Cropscan Inc. 2009b M GreenSeeker optical sensor, 2 Damage assessments Corn Zea mays Banks grass mite Oligonychus Acari: Tetranychidae Martin and Trimble Navigation + two-spotted pratensis Banks + Latheef 2019 spider mite Tetranychus urticae H MS-720 spectroradiometer, 213 Visual inspections Pepper Capsicum annuum L. Chilli thrips Scirtothrips dorsalis Thysanoptera: Mohite et al. EKO Instruments Co., Ltd. Hood Thripidae 2018 H FieldSpec Pro FR 2,151 Damage assessments Pepper Capsicum annuum Two-spotted spider Tetranychus urticae Acari: Tetranychidae Herrmann et al. spectroradiometer, ASD mite 2012, 2015, 2017 H FieldSpec FR 2,151 Arthropod counts Strawberry Fragaria × ananassa Two-spotted spider Tetranychus urticae Acari: Tetranychidae Fraulo et al. spectroradiometer, ASD mite 2009 H FieldSpec 4 Hi-Res 2,151 Arthropod counts Soybean Glycine max Soybean aphid Aphis glycines Hemiptera: Aphididae Alves et al. spectroradiometer, ASD 2015, 2019 H FieldSpec 3, ASD 2,151 Damage assessments Soybean Glycine max Silverleaf whitefly Bemisia tabaci Hemiptera: Aleyrodidae Iost Filho 2019 H FieldSpec Pro FR spectrom- 2,151 Arthropod counts Cotton Gossypium hirsutum Cotton aphid Aphis gossypii Hemiptera: Aphididae Reisig and God- eter, ASD frey 2006 H FieldSpec Pro FR spectrom- 2,151 + Arthropod counts Cotton Gossypium hirsutum Cotton aphid Aphis gossypii Hemiptera: Aphididae Reisig and God- eter, ASD + GER 1500 512 frey 2007 spectroradiometer, Spectra 7 Vista Corp. Downloaded from https://academic.oup.com/jee/article/113/1/1/5666881 by Anand Agricultural University user on 26 September 2024 8 Table 4. Continued Spectral No. of spectral Field Plant common Arthropod common resolutiona Sensor details bands observations name Plant species name Arthropod species Order: Family References H FieldSpec 3 Hi-Res 2,151 Damage assessments Cotton Gossypium hirsutum Cotton aphid Aphis gossypii Hemiptera: Aphididae Chen et al. 2018 spectroradiometer, ASD H FieldSpec 3 Hi-Res 2,151 Damage assessments Cotton Gossypium hirsutum Leafhopper NA Hemiptera: Cicadellidae Prabhakar et al. spectroradiometer, ASD 2011 H FieldSpec spectroradiometer, 2,151 Visual inspections Cotton Gossypium hirsutum Whitefly NA Hemiptera: Aleyrodidae Nigam et al. ASD 2016 H FieldSpec 3 Hi-Res 2,151 Damage assess- Cotton Gossypium hirsutum Solenopsis Phenacoccus Hemiptera: Prabhakar et al. spectroradiometer, ASD ments, sooty mold mealybug solenopsis Tinsley Pseudococcidae 2013 assessmentsb H GER 1500 spectroradiometer, 512 Arthropod counts Cotton Gossypium hirsutum Beet armyworm Spodoptera exigua Lepidoptera: Noctuidae Sudbrink et al. Spectra Vista Corp. 2003 H GER 1500 spectroradiometer, 512 Arthropod counts Cotton Gossypium hirsutum Cabbage looper Trichoplusia ni Lepidoptera: Noctuidae Sudbrink et al. Spectra Vista Corp. Hübner 2003 H FieldSpec Pro FR spectrom- 2,151 + Arthropod counts or Cotton Gossypium hirsutum Two-spotted spider Tetranychus urticae Acari: Tetranychidae Reisig and God- eter, ASD + GER 1500 512 presence/ absence mite frey 2007 spectroradiometer, Spectra assessments Vista Corp. H FieldSpec Pro FR spectrom- 2,151 Arthropod counts Cotton Gossypium hirsutum Spider mite Tetranychus spp. Acari: Tetranychidae Reisig and God- eter, ASD frey 2006 H SE590 spectroradiometer, 252 Arthropod counts Apple Malus domestica European red mite Panonychus ulmi Acari: Tetranychidae Peñuelas et al. Spectron Engineering, Inc. Koch 1995 H ImSpector V10E imaging 512 Damage assessments Rice Oryza sativa Striped stem borer Chilo suppressalis Lepidoptera: Crambidae Fan et al. 2017 spectograph, Specim Spec- Walker tral Imaging Ltd. H Fieldspec Full Range, ASD 2,151 Damage assessments, Rice Oryza sativa Rice leaf folder Cnaphalocrocis Lepidoptera: Crambidae Liu et al. 2012, visual inspections medinalis Guenee 2018 or microscope analyses H FieldSpec Handheld 512 Damage assessments Rice Oryza sativa Rice leaf folder Cnaphalocrocis Lepidoptera: Crambidae Huang et al. spectroradiometer, ASD medinalis 2012a H GER 2600 spectroradiometer, 640 Damage assessments Rice Oryza sativa Rice leaf folder Cnaphalocrocis Lepidoptera: Crambidae Yang et al. 2007 Spectral Vista Corp. medinalis H FieldSpec Handheld 512 Arthropod counts or Rice Oryza sativa Brown planthopper Nilaparvata lugens Hemiptera: Delphacidae Huang et al. spectroradiometer, ASD controlled infest- Stål 2015a, Liu ations and Sun 2016, Tan et al. 2019 H FieldSpec 3 Hi-Res 2,151 Controlled infest- Rice Oryza sativa Brown planthopper Nilaparvata lugens Hemiptera: Delphacidae Prasannakumar spectroradiometer, ASD ations et al. 2013, 2014, Zhou et al. 2010 H GER 2600 spectroradiometer, 640 Damage assessments Rice Oryza sativa Brown planthopper Nilaparvata lugens Hemiptera: Delphacidae Yang et al. 2007 Spectra Vista Corp. Journal of Economic Entomology, 2020, Vol. 113, No. 1 H FieldSpec Pro FR 2,151 Damage assessments Bean Phaseolus vulgaris Two-spotted spider Tetranychus urticae Acari: Tetranychidae Herrmann et al. spectroradiometer, ASD mite 2017 Downloaded from https://academic.oup.com/jee/article/113/1/1/5666881 by Anand Agricultural University user on 26 September 2024 Table 4. Continued Spectral No. of spectral Field Plant common Arthropod common resolutiona Sensor details bands observations name Plant species name Arthropod species Order: Family References H FieldSpec Pro 2,151 Arthropod counts or Peach Prunus persica (L.) Spider mite Tetranychus spp. Acari: Tetranychidae Zhang et al. spectroradiometer, ASD damage assess- Batsch 2008, ments Luedeling et al. 2009 H FieldSpec 3 2,151 Arthropod counts Sugarcane Saccharum spp. Sugarcane thrips Fulmekiola serrata Thysanoptera: Thripidae Abdel-Rahman spectroradiometer, ASD or damage assess- Kobus et al. 2009, ments 2010, 2013 H Nexus FT-NIR spectrometer, 531 Damage assessments Tomato Solanum lycopersicum Leafminer NA NA Xu et al. 2007 Thermo Nicolet Corp. L. H HR2000 spectroradiometer, 62 Arthropod counts Sorghum Sorghum bicolor Corn leaf aphid Rhopalosiphum Hemiptera: Aphididae Li et al. 2008 Ocean Optics Inc. maidis Fitch H HR2000 spectroradiometer, 62 Arthropod counts Sorghum Sorghum bicolor Greenbug Schizaphis graminum Hemiptera: Aphididae Li et al. 2008 Ocean Optics Inc. H Hyperspectral camera, 213 Controlled in- Wheat Triticum aestivum Wheat stem sawfly Cephus cinctus Hymenoptera: Cephidae Nansen et al. Resonon festations and 2009 Journal of Economic Entomology, 2020, Vol. 113, No. 1 arthropod pres- ence confirmations H FieldSpec Handheld 512 Arthropod counts Wheat Triticum aestivum Sunn pest Eurygaster integriceps Hemiptera: Scutelleridae Genc et al. 2008 Spectroradiometer, ASD Puton H Personal Spectrometer II, 512 Controlled infest- Wheat Triticum aestivum Greenbug Schizaphis graminum Hemiptera: Aphididae Riedell and ASD ations Blackmer 1999 H S2000 spectrometer, Ocean 2,048 Arthropod counts or Wheat Triticum aestivum Greenbug Schizaphis graminum Hemiptera: Aphididae Mirik et al. Optics Inc. controlled infest- 2006a, b ations H Pushbroom imaging spec- 1,024 Arthropod counts or Wheat Triticum aestivum Wheat aphid Sitobion avenae Hemiptera: Aphididae Zhao et al. trometer (PIS), Beijing damage assess- 2012, Luo Research Center for ments et al. 2013a Information Technology in Agriculture and University of Science and Technology of China H FieldSpec Pro 2,151 Damage assessments Wheat Triticum aestivum Wheat aphid Sitobion avenae Hemiptera: Aphididae Luo et al. 2011; spectroradiometer, ASD Huang et al. 2012b, 2013, 2014 H FieldSpec UV/VNIR 2,151 Damage assessments Wheat Triticum aestivum Wheat aphid Sitobion avenae Hemiptera: Aphididae Yuan et al. spectroradiometer, ASD or visual inspec- 2014, 2017; tions Zhang et al. 2017 H FieldSpec FR 2,151 Arthropod counts Wheat Triticum aestivum Wheat aphid Sitobion avenae Hemiptera: Aphididae Luo et al. spectroradiometer, ASD 2013b,c H FieldSpec spectroradiometer, 2,151 Damage assessments Wheat Triticum aestivum Wheat aphid Sitobion avenae Hemiptera: Aphididae Huang et al. 9 ASD 2014, Shi et al. 2017 Downloaded from https://academic.oup.com/jee/article/113/1/1/5666881 by Anand Agricultural University user on 26 September 2024 10 Journal of Economic Entomology, 2020, Vol. 113, No. 1 the plant and the soil (mixed pixels). This should be considered when 2010, Nansen Ribeiro et al. analyzing the spectrum. Wherever possible, pixels that represent soil Nansen et al. Blackmer Mirik et al. Riedell and References or other types of non-canopy area are excluded from data analysis. Do Prado 1999 2018 2012 2007 Various VIs assist in interpreting remote sensing data (Roberts et al. 2001, Xue and Su 2017, Verrelst et al. 2019). These are mainly ratios between multiple spectral bands (Glenn et al. 2008). An often- used index is the Normalized Difference Vegetation Index (NDVI), Hemiptera: Aphididae Hemiptera: Aphididae Acari: Tetranychidae which incorporates the ratio of NIR and visible red light. Compared melacanthus Dallas Pentatomidae to a healthy plant, an unhealthy plant will generally reflect more Order: Family visible light and less NIR light. In farming, the NDVI can be used as Hemiptera: a predictor of plant physiological status, as well as potential yield Downloaded from https://academic.oup.com/jee/article/113/1/1/5666881 by Anand Agricultural University user on 26 September 2024 (Peñuelas and Filella 1998). NDVI has its limitations, e.g., when there is a lot of soil in the background. To solve that issue, other VIs have been developed, such as the Soil Adjusted Vegetation Index (SAVI) Two-spotted spider Tetranychus urticae Arthropod species (Huete et al. 1988). Where these two indices are broadband indices Diuraphis noxia Diuraphis noxia (i.e., they can be calculated with multispectral data), hyperspectral data allows for narrowband VIs that can more precisely focus on a Dichelops specific aspect. An example is the Modified Chlorophyll Absorption in Reflectance Index (MCARI), which is defined to be maximally sensitive to chlorophyll content (Daughtry et al. 2000). Xue and Su Arthropod common (2017) provide a review of over 100 VIs for vegetation analysis. Green belly stink Russian wheat Russian wheat Classification Accuracy aphid aphid mite Classification algorithms, which could be based on the red edge and/ bug name or VIs, can be developed to group plants based on spectral data by relating field observations to spectral measurements (e.g., ‘healthy’ A fungus not infesting the plant, but growing on the arthropod’s sugary honeydew secretions. NA = information not provided. and ‘pest-infested’ plants). The algorithms can be based on various Triticum aestivum Triticum aestivum statistical approaches (Lowe et al. 2017). Classification accuracy is high if data has high robustness or repeatability. Different remote Plant species sensing studies report different classification accuracies (Lowe et al. Zea mays Zea mays 2017). A recent study with drone-based remote sensing to detect susceptibility against green peach aphid [Myzus persicae Sulzer (Hemiptera: Aphididae)] in canola, using a multispectral sensor Plant common mounted on an octocopter, a drone with eight rotors, reported a classification accuracy of 69–100%. These values depended on ex- Wheat Wheat name Corn Corn perimental day, drone height above the canopy, and whether or not non-leaf pixels were removed from the dataset. In this study, aphid infestations happened naturally, and aphids were counted on Arthropod counts Arthropod counts Controlled infest- Controlled infest- selected plants for ground verification of infestations (Severtson et al. observations 2016a). A study involving two-spotted spider mite-induced stress in cotton (Gossypium spp.), using a multispectral sensor mounted on ations ations a quadcopter, a drone with four rotors, reported a classification ac- No. of spectral Field curacy of 74–95%. These values depended on classification methods. Spider mite infestation levels were estimated based on plant damage (Huang et al. 2018). As it is hard to reach 100% accuracy, especially bands 2,048 512 240 160 when data are obtained on different days, in most studies, there are certain numbers of false positives (plants are classified as infested while they are healthy) and/or false negatives (plants are classified as Pika II hyperspectral imaging Pika II hyperspectral imaging healthy while they are infested) (Congalton 1991, Lowe et al. 2017). S2000 spectrometer, Ocean Personal Spectrometer II, Nevertheless, multiple robust classifications have been developed to detect pest problems in different agro-ecosystems, which provide M = multispectral, H = hyperspectral. camera, Resonon camera, Resonon good indicators for field scouting (Tables 1–4). Sensor details Optics Inc. Drones, Remote Sensing, and Arthropod Pests