3003PSY Week 10 Mini-Transcript (PDF)
Document Details
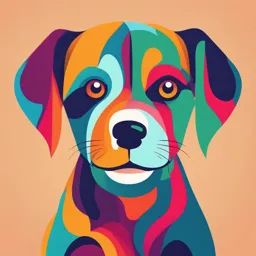
Uploaded by MesmerizedPeridot
Griffith University
Tags
Summary
This document details a lecture on statistical tests, specifically the chi-square test. Examples of two-way Chi-square tests are presented in the context of student attendance and marks. The document discusses how to calculate expected frequencies, degrees of freedom and how to interpret the results of a chi-square test.
Full Transcript
SPEAKER Welcome back to the Mini Lecter syriza $3. 3 ps Y I'm Dr Natalie Locks in. In. In this movie lecture, we will look at the two Waco Square. There's also notice the test of Independence Co. Square and the previous mini lecture. You were introduced to the one way Kaif Square, also known as the...
SPEAKER Welcome back to the Mini Lecter syriza $3. 3 ps Y I'm Dr Natalie Locks in. In. In this movie lecture, we will look at the two Waco Square. There's also notice the test of Independence Co. Square and the previous mini lecture. You were introduced to the one way Kaif Square, also known as the Goodness of Fit Quite square. In that case, there was only one variable. In that case, we use an example of the third flavour of chocolate chips, and we looked at the differences in the frequencies of people's preferences. Are the equity probable distribution null hypothesis In the two way version? There are two variables, and we can now look at the relationship between these variables. The good news is that we use exactly the same formula as the one way chi square They're not. So great news is that we don't get to pick and choose their expected frequency. We have to use a special formula, but don't worry is it's pretty simple. We also don't get to choose our null hypothesis. In the case of the two wake, I swear, the not high offices is that the two variables are distributed independently. This is why we refer to this test is the test of independence. We also referred to this as a contingency case where, as you'll see on the next on the next flight, basically, the no hypothesis states that one variable must not depend upon the other. When we present to wake, I swear data we as a contingency or a cross tabulation table just like this one table pictured here. The cells of the table contains the frequencies of the individuals in that particular sample and that particular category. So the cell which corresponds to blue Eminem preference and mail, contains the frequency of 18. This means that in this started, there are 18 men that had a blue eminent preference. Note that a person can I be male or female and choose either red, blue or green Eminem's. They can't choose more than one category. If they did, then that would be a violation of one of the assumptions of kites were discussed in the next minute lecture. So how does the Tu Wei Chi Square work? Conceptually, as we're dealing with frequency data, we don't have means of sampling distributions. Here we look at the shape of the frequency distribution two variables casted to be independent when the frequency distribution of one variable has the same shape at all levels. Off the second variable. Let's use seller in gender as an example. We just use men and women as we have more information on men and women and salaries than other gender identities. Another prosthesis is that artillery is independent of gender. In this case is another hypothesis is supported in the shape of the frequencies across low, medium high. So it would look the same for men and women, most people in the media matter, and fewer at the high and low in, regardless of gender. There would be independent, however, if Sally Wass dependent on gender. In other words, how much you earn depended on whether you're male, female and, as you can see, the shape of the frequency. Distribution is different for men and women, many earning more at the high salary category. Well, women only more. At the low and medium categories, they have a different shape. So when the shape is a saying that no hypothesis of independence is yet there is no association between gender and salary or when the shape is different, the know how officers rejected and we conclude there's two variables are dependent. In other words, there is an association between gender and salary. Here is a summary of the two types of Chi Square. The two both use frequency data that test somewhat quest. Different questions. The one way asked, How well does Thie observed data fit the model? The model is the expected frequencies, while the two way asked whether the two variables are independent. The two is also asking about model fit, but is more about testing that dependency between the two variables. Okay, let's return to our beloved Jari Key data this time. Assume that we only know if students are the passed or failed the graduates. That's exact in this hypothetical exam, not the one you'll sit. The mark for a passing grade is 65%. Can we see if it were students pass or fail, depending upon whether there had perfect attendance or not record that we've We've already previously used the attendance status variable to state this more formally, is a relationship between class attendance and passing fell in the Examiner sample in the language of Christ Square, our attendance and pass fail status distributed independently or not in the contingency table I've added in the cell numbers for those students were left them perfect. Attendance. £6.5 passed the exam for those streams with perfect attendance, none of the foul and nine past these air out the frequencies. Next, like the one way quite square we need to calculate the expected frequencies has already mentioned for the two week twice where you don't get to choose to show you how this works will work through this conceptually toe workout are expected frequencies. We need to calculate the marginal totals and the grand means, similar to the calculation of analysis of variance where you were interested in the marginal means and the grand means. Since in closely we have frequencies, we look, it totals, hear about it in extra column and rode with the total with the marginal totals and the grand total. To make it a bit easier. Let's remove the cell frequency and just look at the margins, as you can see in the in. The column margins there. Off the 20 students, 11 students had less than perfect attendance and 19 perfect attendance looking at the rose off the 20 students, six failed the exam and 14 students passed. This is how the test of independence works. By ignoring the frequencies within the body of the table and just focusing on the marginals we have you in the data. From the perspective of the now hypothesis is another prosthesis Truth. Anna Marginals Are that what we need in order to understand how the variables would be distributed if they were independent of each other, how do we obtain the necessary expected frequencies? By using the marginal totals, we use the frequencies expected under the no hypothesis of independence. Remember, you can't pick and choose here just like we could with the one week I square. Let's use the marginal totals to calculate how many past and how many foul deeds. Regardless ofthe tenants status, we see that six out of 20 or 30% failed the exam, while 14 out of 20 or 70% Parsi death. This was regardless of their attendance status with the variables are independent. The relative proportion of those who passed failed thie Similarly, city would be mirrored. Each level of the attends terrible, that is 70% of those with perfect attendance would pass and 30% would fail, and 70% of those with less than for the tenants were passed and 30% would fail. From this, we can work out our expected frequencies for the students with less than perfect attendance. There was no association relation between the attendance status and passing or not. Then we would expect 30% of the 11 students with less than perfect attendance to fail. So 3.3 students and 70% pass so 7.7 students they're looking at those with perfect attendance again. If there was no association between passing on that and the tennis status, then we would expect 30% of the nine students with perfect attendance to fail. So 2.7 students and 70% to pass So 6.3 students. We could continue to do it this way, but there's actually a formula that makes it easier. FreeCell expected frequency would multiply the column total by the total and divide this by the grand total. Here I've duplicated the total replaced the obtained frequencies that is our actual data with the expected frequencies to represent the weather data, Oughta book under the No high Offices, assuming a null hypothesis is true. In other words, our calculation off the expected frequencies for itself. On the free These slides Yeah, I've put in the calculate expected frequency into the table. See how these are the same as the expected frequencies calculated before. Also know how these add up to the 20 students in the study. For now, I've had the actual data thie observe schools and blue and expected schools have stated under the no of independence and pink. Now that we have the required information, we can use the case with formula to calculate outcry Square values just is in the one week I square OK, I've moved the observed in expected frequencies title a bit to look at the first part of the calculation in a larger table. I've added in the difference between the absurd frequencies and the obtained frequency squared, divided by expectancies, part of the formula, the part that was highlighted in blue, for example, this is the less than perfect attendance part self. You can see that he's in the former 0.95 this calculate for that particular cell, and I would do this for every other cell. Now, for the final part of the formula, we need to add up all the cell values. So we end up with the chi square value of 7.2. As with with the one way the chi square, what does is actually me yet again, we consult a good old guy square table for our critical value. The calculation off the degrees of freedom is different to the calculation of degree of freedom. With one way we need to take into account the two variables. So the formula for the two way degree the freedom is the number of cells minus one multiplied by the number of columns minus one. And this particular example we would have a two by two Chi square, and so our degrees of freedom would be two minds, one multiplied by two most one one more. One more blood by one is one. We have one degree of freedom zooming in on the critical value of one degree of freedom. With probability point of five, we get a cut off 3.84 as are obtained. Chi Square is 10.2 and is therefore larger than 3.84 We reject the null hypothesis of independence and conclude that our sample represents population in which class attendance and grave passing a fairly our associate ID in other words, not independent. So what do we conclude? If we had predicted that attendant status and example formats were related, will be very pleased to found evidence in support of this prediction. Sometimes, though, we may be hoping to find it. They were actually we're independent. This type of hypothesis is a bit complex, but it does happen. Remember, the statistical output has always had meaning taken out of it. Before we get it, we need to supply the meaning to our results. In this new lecture, we've used hand calculations, and often this is easier, especially when you don't have the raw data and you only have the self frequencies and the truths they you'll actually practise. Using FBS using the cross taps function, I've added a worksheet to Lenny Griffith, and it's also a spark page that works through this particular example. In summary, the two way Chi Square test the association between two categorical variables. The two way car squares referred to us the test of independence. The expected frequencies of the two way, it said, is set to test the independence of the two variables. The calculation of expected frequencies involves the marginal totals and the grand total. The obtained Chi Square is tested against the Chrysler distribution. The calculation of degrees of freedom involves a number of categories for both variables, not sample size and calculated by hand is better than S P SS when you only have cell means.