3003PSY Mini Transcript (Part 1) - Multiple Regression PDF
Document Details
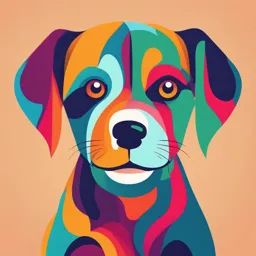
Uploaded by MesmerizedPeridot
Griffith University
Tags
Summary
This document contains a transcript of a lecture on multiple regression. The lecture covers topics such as variance explained by predictors, and sums of squares. It explains the concepts behind regression equations.
Full Transcript
SPEAKER 1 Welcome back to the mid Elector Syriza three by three SPEAKER 0 ps y I'm Dr Natalie Luxton and in his mini lecture we will look at variance explained in multiple regression. SPEAKER 1 Recall that in correlation that we square the correlation to give us an index of the variance in there....
SPEAKER 1 Welcome back to the mid Elector Syriza three by three SPEAKER 0 ps y I'm Dr Natalie Luxton and in his mini lecture we will look at variance explained in multiple regression. SPEAKER 1 Recall that in correlation that we square the correlation to give us an index of the variance in there. Why variable is explained by Xperia ble. SPEAKER 0 We call this our square and this represents proportion of SPEAKER 1 the variance shared by X y. In other words, how much the two variables overlooked as mentioned in a previous mini lecture In regression, we referred to us. Where is the amount of granted? Why accounted for or explained by either a single predictor, as in a Zeon, a vibrant regression or a set SPEAKER 0 of predictors as in multiple regression in multiple aggression are SPEAKER 1 squares the variant of white accounted for bio xperia Bols SPEAKER 0 X case simply indicates that we have up to K number of predictors we usually refer to This is big are square here ask where is not specific 21 single SPEAKER 1 predictor it is instead of them recalling the previous many SPEAKER 0 ledges that we have a linear complicit otherwise known as SPEAKER 1 our regression equation, they're correlates with the wide variable We SPEAKER 0 also referred to our regression equations as the model has SPEAKER 1 three years off model summary table. Just like in the Bible regression, we confined the value of our square in the model summary cable. You may note that our square is typically the square, SPEAKER 0 a path just like in correlation. But what does ask where really need recalling the Million SPEAKER 1 Lecture in Week six. SPEAKER 0 But we looked at how we partition the components of each person score in the UAE, variable into one. SPEAKER 1 The systematic component which is represented by the differences between a person's predicted score on why and the mean of why and record that this is the improvement in predicting their score based on our relationship with the ex variables and two is usually also a random or air component, which is the difference between the person's actual score. Why and they predicted school. I also noted that we need to do this for all the people in the diver sect and that we use a method called sons of Squares to calculate the SPEAKER 0 variability and why schools that can be predicted by the regression equation. SPEAKER 1 I also noted that we use some squares to calculate SPEAKER 0 our square. In the case of multiple regression are square. SPEAKER 1 Is the amount of various explained by the regression equations or our model here I spelled it in some two squares. Equation will fully remember that. I said in the previous many lectures that I would return to this. SPEAKER 0 So we have won some two squares. Why also referred to it some two squares taught or something squares total. This is the square differences of all the scores on SPEAKER 1 why from the mean apply two. SPEAKER 0 We have some squares, regression or something. Squares rig these air. The square difference is awful. The predicted schools on why from the minister's wife and SPEAKER 1 I've highlighted this in Peak three, we have some two SPEAKER 0 squares, residual or something. Squares risk the square differences of all the scores are SPEAKER 1 wife from their predictive scores, and I've highlighted this and blue. SPEAKER 0 Finally, we can express the formula are square as proportion off the total sum of squares explained by some two squares, regression and the equivalent formula with the Sons of squares component specified mournfully. SPEAKER 1 These are important concepts and formula to remember if you understand how the little formers air simply representing square differences SPEAKER 0 then they're really not that difficult to remember. So let's go back to our G R E Q output. SPEAKER 1 Luckily for us, we don't need to sit there and manually calculate all the differences, square them and then add them up. A sum of squares a calculated by S P SS. They're provided in the end of the table asked where is also conveniently calculated for us, but it's worth seeing where the numbers come from again. Here I've zoomed into the relevant parts of the output, and I've used my trusty calculator. We can see that I've divided the value of the SPEAKER 0 sum squares total, but the values something squares regression to SPEAKER 1 give the value of 0.61 You can see that with rounding. This is the same as they are squid calculate by SPEAKER 0 its process. So you see how our squares calculated using something squares. We're just using something. Squares toe work at the proportion of variance in the SPEAKER 1 UAE scores that explained by the improvement of prediction. By using the regression equation, I agree that you can also simply have just square the value of bigger. But there are advantages to understanding how some two squares SPEAKER 0 plays a role for practise. SPEAKER 1 I placed a worksheet on Lenny aggressive. SPEAKER 0 Okay, so that was looking at the various explained for SPEAKER 1 the overall regression equation are good on linear complicit but SPEAKER 0 with multiple regression. We want to also see how much of the various SPEAKER 1 an outcome score is explained by each of the predictive SPEAKER 0 variables. After controlling for or partially out the various explained by the other. Predict the variables. SPEAKER 1 Here we use a new coefficient, quarter semi partial correlation and a certain cultural correlation squared. These, like our square that Timmy partially is also squared SPEAKER 0 to provide the amount of variants that is uniquely explained by each variable. SPEAKER 1 So it's only partials are actually quite simple to calculate. In our regression syntax, we simply adds e p p to the end of the syntax, asking for the stats that we wanted the output. Now, the trick for young players is that in the output we have three extra columns in the coefficient table. Now, this table may actually be an extension of the SPEAKER 0 coefficients table above or meet maybe under there it depends SPEAKER 1 sometimes on the formatting that you're getting from your version of this Pegasus. The club that we're interested in is actually annoyingly labelled part. So we actually used the park all not the partial call. And this is not an error. You don't really need to know why you need to SPEAKER 0 use the part correlations. SPEAKER 1 Let's just accept that and move on. What we need to do is we need to square SPEAKER 0 each of our part correlations to calculate the squared Timmy partials for each predict a variable. SPEAKER 1 Okay, before we go on with our calculation of semi SPEAKER 0 partials. Recall that previously we looked at calculating various explained by SPEAKER 1 very regression. Here have summarised some squares calculation for the key score SPEAKER 0 and the stats exam performance here. Gr eek! You account for approximately 32 percent of the variance. That's that's exam performance. I represented this previously using a pie graph. The Biograph is perfectly fine for the Bybee regression analysis. SPEAKER 1 The problem is, once we have multiple predictors, we actually need a different visual approach. How I visualised parents explained in multiple regression. We used then diagrams. This is a diagram that is based on a figure in a rather large text that many people who do a lot of regression analysis have a notebook shells. The authors are two women to bash Nick and Fidel. You may hear people refer to bash you give the SPEAKER 0 dealt in your travels. Many of the assumption cheques that we're going to cover next week's topics are actually from this text. I've used the Venn diagram from this text and colour coded to highlight the important areas. I apologise in advance to anyone who's colour blind. The wife's circle represents the outcome variable. The X one circle represents the first predictor and the X to the second predictor, and you could have more SPEAKER 1 of these. Have you had more predicted? But the figure becomes quite complicated. SPEAKER 0 The more that the expert circles over let the white circle the greater amount of that variance of wife that SPEAKER 1 is accounted for by each X variable this figure and SPEAKER 0 allows for the correlation between the predictive variables to be SPEAKER 1 taken into account. SPEAKER 0 The wrist section represents the effect of the predictor one SPEAKER 1 on the Y variable that is independent of any correlation SPEAKER 0 with predicted, too. We refer to this as unique variant of X one. SPEAKER 1 The green section represents the effect of predicted two on the Y variable that is independent of any correlation with predict one, and we refer to This is the unique parents of X two. SPEAKER 0 The youngest section represents the effect of the predictors on the Y variable that they both share and we call SPEAKER 1 the shared variance. We also have a blue section, and this represents the correlation between the two predictors that is not related to SPEAKER 0 the wide variable. We really don't have a great deal of interest in SPEAKER 1 that. SPEAKER 0 Together, the red, yellow and blue portions represent our square, so that's pretty abstract. SPEAKER 1 Let's make this more concrete by returning to our JI. Our example. SPEAKER 0 Remember how we looked at the significance of the overall model, our aggression equation, and also the significance in each of our two predicted variables while controlling for the other in the previous mini lecture. SPEAKER 1 We also want to know how much of the variance into that's exempt form is accounted for uniquely by each predictor here. The inexperience of stats example formers explained by gr Eek SPEAKER 0 ee school is represented by the bread section. SPEAKER 1 We've taken out any variances explained by attendance, so to calculate this, we need to square the park correlation. Once again, I pulled out my trusty calculator and square SPEAKER 0 the park correlation of 0.433 This gives us a value of 0.187 If we rounded to decimal points, then we SPEAKER 1 can say that we have 90% of the variance. That's exam performance accounted for by Geo. SPEAKER 0 We can state this more folly as after various after very insecure counter for where the tenders has been removed SPEAKER 1 for Y. G R E Q accounts for 90% of additional very intense That's exam performance. This is the unique various accountable by G. R. E. Q. You may have noticed that this is smaller than the various accounted indeed, by very regression, which is 32%. SPEAKER 0 This is because of Barrett, shared with attendance, has now SPEAKER 1 being removed. So what about attendance again? We need to square the park correlation when we swear SPEAKER 0 apart correlation, we get 0.286 or 29% of the very SPEAKER 1 six million technique, uniquely by attendance or more fully after very into shared with gr eek has been removed from why attendance accounts for 29% of the additional variance in that's example. For months, this is a unique variance accounted for by attendants. Again, this is smaller than the by very regression. The variance explained off 42% and again is due to the removal of shared variance with J. R. E. Q. Just make a note that the drawing here is not to scale. Finally, we need to calculate the shared variance. Is this the parents of the UAE variable that is SPEAKER 0 explained by the predictors but also shared by the predictors as ask where is the title of a ll the SPEAKER 1 unique variances and the share parents? Then we simply need to minus the unique they combined unique variances from our square. Once again I call it my trusty calculator and scrap of paper. I know that my are square or 0.608 semi partial are predicted one is 10.187 and my semi partial squared SPEAKER 0 off predicted Tio 2.286 I can't commend the squares of eggs in the previous steps. SPEAKER 1 Then I simply minus the two semi partials which together SPEAKER 0 are 0.473 from my are square. This leaves us which she had various appoint 136135 So we can state that the shared varies between the two predict the variables on states exam performance was 13.5%. SPEAKER 1 Again, I've redrawn this to note the unique and shared variants conceptually, with the sections now more to scale. SPEAKER 0 And here are our results, I thought, the easier to SPEAKER 1 visual ageing again. Shared variances using Ben diagrams with two predictors models with SPEAKER 0 more predictive variables become quite confusing. SPEAKER 1 They What this figure shows us is that it had SPEAKER 0 that it appears that attendance accounts for a large proportion offsets exam performance than J. R. E. Q. When we take it, the variance is shared between them. This was also reflected in the beat awaits. As a psychologist, I would use this information to suggest that class attendance for the post graduates that's course is a better predictor of Sam performance. An aptitude as such. If I was to suggest an intervention and it would be to encourage class attendance, this is also behaviour that is changeable, whereas it would be difficult to chance someone aptitude. SPEAKER 1 Another thing worth noting is that sometimes there may be a significant correlation between a predictor in an outcome, but this becomes not significant in a multiple regression. SPEAKER 0 This is usually because the correlation between the predict the variables maybe so high that there's relatively little unique various left again. SPEAKER 1 I had provided what shit for you to practise, in summary asked were first to the variance in the wide SPEAKER 0 variable explained by the linear compass, it also known as a regression inflation or the model in most for aggression. SPEAKER 1 We want the variance explained by its predictive variable after SPEAKER 0 controlling for it for the other predict the variables we SPEAKER 1 call this unique variance is represented by squared, semi partial correlations. The difference between total various explained our square and unique variant is shared. Various. We can look atyou Nick variances to better understand the strength of the relationships between a predictive variables and the outcomes.