EEP/IAS 118 Introductory Applied Econometrics, Section 3 PDF
Document Details
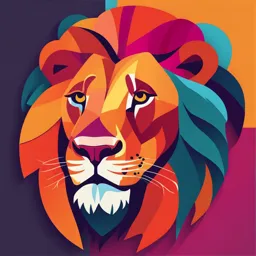
Uploaded by SwiftPrimrose2997
University of California, Berkeley
2024
Leila Njee Bugha and Nicolas Polasek
Tags
Related
- 1 Introduction Linear regression model.pdf
- 2 Regression diagnostics complications.pdf
- 3 Endogeneity Instrumental variables.pdf
- 11_Regression with a binary dependent variable.pptx (1).pdf
- EEP/IAS 118 Introductory Applied Econometrics, Section 1 PDF
- EEP/IAS 118 - Introductory Applied Econometrics, Section 2 PDF
Summary
These lecture notes cover introductory applied econometrics, specifically focusing on population parameters, sample estimators, and multiple linear regression. The document also discusses various concepts including variance, covariance, and the characteristics of estimators.
Full Transcript
EEP/IAS 118 - Introductory Applied Econometrics, Section 3 Leila Njee Bugha and Nicolas Polasek Week of September 16th, 2024 1 / 21 Overview and announcements Solutions for Small Assignment #1 released (Big) Assignment #1 due F...
EEP/IAS 118 - Introductory Applied Econometrics, Section 3 Leila Njee Bugha and Nicolas Polasek Week of September 16th, 2024 1 / 21 Overview and announcements Solutions for Small Assignment #1 released (Big) Assignment #1 due Friday at 11.59pm on Gradescope Today: Population parameters and sample estimators Multiple linear regression 2 / 21 Quick review Variance and covariance 1 (Population) Variance: 2 (Population) Covariance: Punchline of SLRs 1 through 4: 1 E( β̂ 0 )= 2 E( β̂ 1 )= Goodness of fit (R2 ): 1 R2 = 2 SSE, SSR, SST : 3 / 21 Population Parameters If X is a random variable: the expected value (or expectation) of X = weighted average of all possible values of X. k E( X ) = µX = ∑ xj f (xj ) j =1 the variance tells us the expected distance from X to its mean: Var ( X ) = σX2 = E[( X − E( X ))2 ] Both of these are population parameters. 4 / 21 Sample Estimator(s) We don’t observe the population, but we can calculate the mean and variance in a sample ⇒ This is the best estimate for the mean and variance in the population. Definition: An estimator is a pre-specified rule (function) that assigns a value to some unknown population parameter θ for any possible outcome of the sample. 5 / 21 Mean and Variance Sample Estimators Recall our population has mean µ X and variance σX2. Then An estimator of µ X is the sample mean X̄ = An estimator of σX2 is s2X = When we collect a specific sample from this population, we can get a particular estimate for X̄ and s2X. Note: σ̂X2 or s2X mean the same thing. 6 / 21 Distributions of Estimators The estimators themselves are random variables because they depend on a random sample. This means 2 things: 1 As we obtain different random samples from the population, the values of X̄ can change. 2 Hence they have a certain probability distribution, with a certain mean and a certain variance/standard deviation. 7 / 21 Characteristics of X̄ Estimator Just like our underlying variable X has an expected value and variance, so does the estimator X̄. 1 1 1 1 E[ X̄ ] = E[ n ∑ Xi ] = n E[∑ Xi ] = n nE[Xi ] = n n(µX ) = µX i i " # " # 1 1 1 σX2 Var [ X̄ ] = Var n ∑ Xi = 2 Var n ∑ Xi = n2 nVar [ X i ] = n i i q Sd[ X̄ ] = (Var [ X̄ ]) = BUT we don’t know σX because this is a population parameter! So how can get the standard deviation of our estimator? 8 / 21 Standard Error of X̄ Estimator 1 We use our estimator for σX2 , s2X = ∑n ( xi − x̄ )2 , since this n−1 i estimator is unbiased. We call s x the standard error - essentially the standard deviation of our estimator once we replaced the population σX with the sample estimator s X sX Se[ X̄ ] = √ n Note: It may seem unintuitive to divide by n − 1 rather than n to calculate s2X but basically this is a way of accounting for fact that the sample mean in this formula is also an estimator with its own variance 9 / 21 Summary: X as continuous variable Symbol Formula Population parameters µ ∑kj=1 xj f (xj ) σX2 E[( X − E( X ))2 ] p σX E[( X − E( X ))2 ] 1 Sample estimators X̄ ∑ i Xi n s2X 1 ( X − X̄ )2 qn−1 ∑i i 1 sX n−1 ∑i ( Xi − X̄ ) 2 Estimator parameters E( X̄ ) µX σX2 Var ( X̄ ) n σX Sd( X̄ ) √ n sX SE of estimator Se( X̄ ) √ n 10 / 21 The estimator β̂ Transitioning back to the population model we discussed previously: y = β0 + β1 x + u β̂ 0 and β̂ 1 are estimators for the parameters β 0 and β 1. The formula for our βs was a rule that assigns each possible outcome of the sample a value of β. Then, for the given sample of data we work with, we obtain particular intercept and slope estimates, β̂ 0 and β̂ 1. Because β̂ 1 ≡ cov( x, y)/var( x ) is an estimator based off a random sample, it has a standard error of its own. 11 / 21 Summary: Regression Estimates Symbol Formula Population parameters β0 β1 Sample estimators β̂ 0 ȳ − β̂ 1 x̄ n ∑i=1 ( xi − x̄ )(yi −ȳ) β̂ 1 n ∑i=1 ( xi − x̄ )2 Estimator parameters* E( β̂ 0 ) β0 E( β̂ 1 ) β1 σu2 Var ( β̂ 1 ) SSTx Sd( β̂ 1 ) √ σu SSTx SE of estimator Se( β̂ 1 ) √ σ̂u SSTx *We don’t show Var ( β̂ 0 ), Sd( β̂ 0 ), or Se( β̂ 0 ) because we rarely care. Note σ̂u2 = nSSR −2 under SLR 1-5 (see L5 slides). 12 / 21 Review: Assumptions of (Simple) Linear Regression We make these assumptions about the “true data generating process” Model Simple SLR.1 The population model is linear in parameters y = β 0 + β 1 x1 + u SLR.2 {( xi , yi ), i = 1 · · · N } is a random sample from the population SLR.3 The observed explanatory variable ( x ) is not constant: Var ( x ) ̸= 0 SLR.4 No matter what we observe x to be, we expect the unob- served u to be zero E[u| x ] = 0 (mean independence) SLR.5 The “error term” has the same variance for any value of x : Var (u| x ) = σ2 (homoskedasticity) 13 / 21 Why Multiple Linear Regression? If we think other variables besides our variable of interest x1 belong in our model, then we want to include them in our model. Otherwise they’d enter in u, potentially violating SLR4. The following two equations illustrate these benefits: wage = β 0 + β 1 educ + β 2 exper + u (1) 2 consumption = β 0 + β 1 inc + β 2 inc + u (2) In equation (1) we want to know the direct effect of education on wages. Here we explicitly control for experience. Compared to SLR, we have effectively taken experience out of the error term and put it explicitly in the equation. Otherwise we would have had to unrealistically assume that experience is uncorrelated with education to make SLR 4 hold. In equation (2) the model falls outside simple regression because it contains two functions of income, income and income2. 14 / 21 What Changes With Multiple Linear Regression Our model is now y = β 0 + β 1 x1 + · · · + β k xk + µ. How do we interpret β 1 now? We now say it is the effect of x1 on y holding all else fixed/equal Can also rephrase this as ceteris paribus or conditional on or controlling for x2... xk We will also need to adjust our assumptions to accommodate the additional x variables. 15 / 21 Assumptions for Multiple Linear Regression How do the necessary assumptions change when we have multiple xs ? Model Multiple MLR.1 The population model is linear in parameters y = β 0 + β 1 x1 + · · · + β k x k + µ MLR.2 {( xi1 , · · · , xik , yi ), i = 1 · · · N } is a random sample from the population MLR.3 No perfect colinearity among observed variables and Var ( x j ) ̸= 0, j = 1 · · · k MLR.4 No matter what we observe ( xi1 , · · · , xik ) to be, we ex- pect the unobserved u to be zero E[u| x1 , · · · , xk ] = 0 MLR.5 The “error term” has the same variance for any value of( x1 , · · · xk ) : Var (u| x1 , · · · xk ) = σ2 16 / 21 What do we get from these assumptions? 1 E( β̂ j ) = β j , using only assumptions 1 - 4 The mean of our estimators β̂ j are our true population parameters βj. σu2 2 Var ( β̂ j ) = SSTj (1− R2j ) , adding assumption 5 Note here: - SSTj = ∑i ( xij − x̄ )2 is the total sample variation in x j - R2j is the R2 from regressing x j on all other independent variables Xs ∑i û2i SSR - σ̂u2 = n − k −1 = n − k −1 17 / 21 Exercise Suppose we estimated the following equation: d = 10.36 − 0.094sibs + 0.131meduc + 0.210 f educ educ where educ is years of schooling, sibs is number of siblings, meduc is mother’s years of schooling, and f educ is father’s years of schooling. 1 Does sibs have the expected effect? Interpret and answer. 2 Holding meduc and f educ fixed, by how much does sibs have to increase to reduce predicted years of education by one year? 3 Discuss the interpretation of the coefficient on meduc 4 Suppose that Alice has no siblings, and her mother and father each have 12 years of education. Bob has no siblings, and his mother and father each have 16 years of education. What is the predicted difference in years of education between Alice and Bob? 18 / 21 Exercise solving: 1, 2 Suppose we estimated the following equation: 1 Does sibs have the expected effect? Interpret and answer. 2 Holding meduc and f educ fixed, by how much does sibs have to increase to reduce predicted years of education by one year? 19 / 21 Exercise solving: 3, 4 Suppose we estimated the following equation: 1 Discuss the interpretation of the coefficient on meduc 2 Suppose that Alice has no siblings, and her mother and father each have 12 years of education. Bob has no siblings, and his mother and father each have 16 years of education. What is the predicted difference in years of education between Alice and Bob? 20 / 21 Running multivariate linear regression models in R What happens if you want to run a model with multiple Xs in R? It’s very similar to single regression, we still use lm() Just now we have: for a dataset object named “mydataset” that contains dependent variable ‘y’ and independent variables ‘x 1’, ‘x 2’ and ‘x 3’ Click here for an example! 21 / 21