3003PSY Mini-lecture Multiple Regression PDF
Document Details
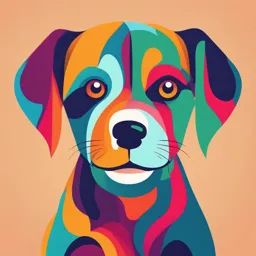
Uploaded by MesmerizedPeridot
Griffith University
Tags
Summary
This Griffith University document explains multiple regression, a statistical method in psychology for predicting outcomes from multiple variables. It also covers the concepts behind b-weights and standardized weights. The document illustrates the method with examples.
Full Transcript
3003PSY Survey Design and Analysis in Psychology MULTIPLE REGRESSION MULTIPLE REGRESSION uWhen we have > 1 predictor (X) variable, we simply need a way to combine them uThe regression procedure expands seamlessly to do this… uEssentially,we extend the least squares procedure to estimate b...
3003PSY Survey Design and Analysis in Psychology MULTIPLE REGRESSION MULTIPLE REGRESSION uWhen we have > 1 predictor (X) variable, we simply need a way to combine them uThe regression procedure expands seamlessly to do this… uEssentially,we extend the least squares procedure to estimate b0 and b1 and b2… bk to give us the best possible prediction of Y from all the variables jointly uThis type of regression might be referred to in some texts as OLS, Ordinary Least Squares Regression Back to b-weights: the b-weights are the numbers by which we multiply (i.e., weight) each X to make a composite X with all the information from the separate Xs in it B-WEIGHTS IN MULTIPLE b-weights are now partial slopes REGRESSION each b tells us how much change in pred. Y there will be for a change of 1 in that X when all the other Xs are held constant This gives us a clue as to how much each X is related to Y when considering the interrelationships among the Xs better than looking at correlations among all variables as these are not corrected X (GRE-Q) 620 GRE_Q example Y (stats exam) 65 600 73 590 85 590 80 580 64 560 69 550 78 540 70 530 74 530 70 500 77 480 69 480 64 460 76 440 54 430 44 340 75 380 69 370 54 280 43 uWe are going to try to understand the predictors of graduate statistics exam uWe are going to consider both previous predictors: STATS EXAM 1) GRE_Q score 2) Attendance (every lecture = 1, not EXAMPLE quite every lecture = 0) uWe have already found that both higher GRE-Q scores and attending every lecture are positively related to graduate exam performance. uNow we are interested in both variables as predictors of exam performance SPSS Syntax and Output for multiple regression This is the regression output regression var= Stats_Exam GRE_Q Attendance with both predictors in the /descriptive = def analysis /dep= Stats_Exam /enter. This is the correlation between the two predictor variables DECONSTRUCTING THE REGRESSION EQUATION uThis means, a person who scored 0 on the GRE_Q variable and 0 (zero) on attendance (not 100% lecture attendance) would have a predicted score on Stats_Exam of 36.130 (which is rather meaningless…) DECONSTRUCTING THE REGRESSION EQUATION uFor every point that a student scores on GRE_Q , their predicted score on stats_exam would increase by.053 when holding constant Attendance DECONSTRUCTING THE REGRESSION EQUATION uFor every point that a student “scores” on attendance (i.e., are someone who attended every lecture relative to someone who did not), their predicted score on stats_exam would increase by 12.157 when holding constant scores on GRE_Q STANDARDISED WEIGHTS uBeta (β) weights uAs for b weights but for standardised solution uIntercept = zero and drops out of equation uGive a measure of slope in standardised units uThis aids in comparison but is not importance as is misleadingly stated in some sources VARIANCE EXPLAINED uWe also get R = 0.78, and R2 = 0.608, so 60.8% of the variance in stats_exam is accounted for by the best linear composite of GRE_Q and Attendance. unote that it is not the sum of the two separate correlations. It is a joint quantity. SUMMARY u Bivariate regression is a special case of regression with a single predictor variable uThis is the basis of multiple regression, whereby additional predictors can be added uMultiple regression allows us to account for the correlation between predictor variables uThe intercept is the predicted score for scores of zero on each predictor variable uThe slope (or rather partial slopes) are the predicted value on Y for each 1 unit change on each X variable, while holding other other predictor variables constant uBeta weights can be used to compare the association between different predictor variables and the outcome variable uR and R2 refer to the amount of variance explained in the outcome variable by the linear composite (i.e., the regression equation)