2011 NSDUH MRB Questionnaire PDF
Document Details
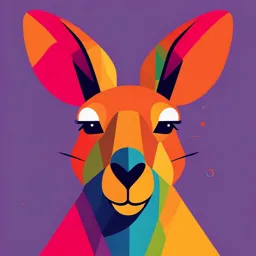
Uploaded by HappierSagacity4084
University of Engineering and Technology, Lahore
2011
Matthew Westlake,Patrick Chen,Harper Gordek,Thomas G. Virag
Tags
Summary
This document describes the weighting calibration method used for producing sampling weights for the 2011 National Survey on Drug Use and Health (NSDUH). It details procedures for questionnaire dwelling unit (QDU) and person pair weights, including selection probabilities, calibration using the generalized exponential model (GEM), extreme weight adjustments, and poststratification adjustments. The methods described have been used in NSDUH (previously NHSDA) since 1999
Full Transcript
2011 NATIONAL SURVEY ON DRUG USE AND HEALTH QUESTIONNAIRE DWELLING UNIT-LEVEL AND PERSON PAIR- LEVEL SAMPLING WEIGHT CALIBRATION Prepared for the 2011 Methodological Resource Book RTI Project No...
2011 NATIONAL SURVEY ON DRUG USE AND HEALTH QUESTIONNAIRE DWELLING UNIT-LEVEL AND PERSON PAIR- LEVEL SAMPLING WEIGHT CALIBRATION Prepared for the 2011 Methodological Resource Book RTI Project No. 0211838.207.005 Contract No. HHSS283200800004C Deliverable No. 39 Authors: Project Director: Thomas G. Virag Matthew Westlake Patrick Chen Harper Gordek Prepared for: Substance Abuse and Mental Health Services Administration Rockville, Maryland 20857 Prepared by: RTI International Research Triangle Park, North Carolina 27709 January 2013 DISCLAIMER SAMHSA provides links to other Internet sites as a service to its users and is not responsible for the availability or content of these external sites. SAMHSA, its employees, and contractors do not endorse, warrant, or guarantee the products, services, or information described or offered at these other Internet sites. Any reference to a commercial product, process, or service is not an endorsement or recommendation by SAMHSA, its employees, or contractors. For documents available from this server, the U.S. Government does not warrant or assume any legal liability or responsibility for the accuracy, completeness, or usefulness of any information, apparatus, product, or process disclosed. 2011 NATIONAL SURVEY ON DRUG USE AND HEALTH QUESTIONNAIRE DWELLING UNIT-LEVEL AND PERSON PAIR- LEVEL SAMPLING WEIGHT CALIBRATION Prepared for the 2011 Methodological Resource Book RTI Project No. 0211838.207.005 Contract No. HHSS283200800004C Deliverable No. 39 Authors: Project Director: Matthew Westlake Thomas G. Virag Patrick Chen Harper Gordek Prepared for: Substance Abuse and Mental Health Services Administration Rockville, Maryland 20857 Prepared by: RTI International Research Triangle Park, North Carolina 27709 January 2013 Preface This report documents the method of weight calibration used for producing the final set of questionnaire dwelling unit (QDU) and pair weights for the National Survey on Drug Use and Health (NSDUH) data from 2011. The weighting team faced several challenges in this task and was able to address them by resorting to innovative modifications of certain basic statistical ideas, which are listed below. Under Brewer's method, high weights may occur due to small pair selection probabilities. In any calibration exercise, some treatment of extreme value (ev) in weights is needed, but there is a danger of introducing too much bias by over- treatment. In the generalized exponential model (GEM), which is described in detail in Chen et al. (2013), extreme value control is built in, but one needs to define suitable ev domains so that not too many evs are defined. If too many design variables are used to define ev domains, then each domain will be very sparse and will not be of much use in defining thresholds for ev. As in past surveys, a hierarchy of domains was defined using pair age (each pair member being in one of the three categories: 12 to 25, 26 to 49, and 50+) and number of persons aged 12 to 25 in the household, State, and clusters of States (see Section 5.2 for details). Control of extreme values in weights helps reduce instability of estimates to some extent, but there is a need for methods that do not introduce much bias. Following the famous suggestion of Hajek (1971) in his comments on Basu's fabled example of circus elephants, we performed ratio adjustment (a form of poststratification) to estimated totals obtained from the household data on the number of persons belonging to the pair domain of interest. This was implemented in a multivariate manner to get one set of final weights. In the absence of a suitable source of poststratification controls for the person pair- level weights and the household-level weights, the inherent two-phase nature of the survey design was capitalized upon to estimate these controls from the first phase of the large screener sample. The first-phase sample weight was poststratified to person- level U.S. Census Bureau counts to get more efficient estimated counts for pair and household data. The problem of multiplicities complicated the issue of providing one set of final weights. When dealing with person-level parameters involving drug-related behaviors among members of the same household, it is possible for an individual to manifest himself or herself in the pair sample through different pairs. To avoid overcounting, the pair weights have to be divided by multiplicity factors, which tend to be domain specific. For this reason, multiplicity factors for a key set of pair analysis domains also are produced along with a set of final calibrated pair weights. Missing items in the respondent questionnaire led to imputation for deriving pair relationships, multiplicity factors, and household counts for Hajek adjustments. iii The calibration task described in this document has been in place, with minor modifications, since the 1999 version of NSDUH, which was then called the National Household Survey on Drug Abuse (NHSDA).1 Results from this calibration applied to an earlier survey year were presented at the 2001 Joint Statistical Meetings. The procedures described in the proceedings papers from these presentations can serve as useful supplemental reference material on estimation in the presence of multiplicities and extreme weights (Chromy & Singh, 2001) and on GEM calibration of pair weights (Penne, Chen, & Singh, 2001). The experience of using GEM with person weights is described in an earlier proceedings paper (Chen, Penne, & Singh, 2000). This work was completed for the Substance Abuse and Mental Health Services Administration (SAMHSA), Center for Behavioral Health Statistics and Quality (CBHSQ), by RTI International (a trade name of Research Triangle Institute), Research Triangle Park, North Carolina, under Contract No. HHSS283200800004C. The authors would like to take this opportunity to thank a number of individuals for useful discussions and suggestions: Joe Gfroerer and Art Hughes of SAMHSA and Jim Chromy of RTI. NSDUH Weighting Team Ralph Folsom, Senior Advisor Research Triangle Park, NC 1 The National Household Survey on Drug Abuse (NHSDA) was renamed the National Survey on Drug Use and Health (NSDUH) in the 2002 survey year. iv Table of Contents Chapter Page List of Terms and Abbreviations………………………………………………………………...xv 1. Introduction......................................................................................................................... 1 2. Questionnaire Dwelling Unit and Pair Selection Probabilities........................................... 7 2.1 Pair Selection Probability....................................................................................... 8 2.1.1 Case I: DUs with S ≥ 2............................................................................... 8 2.1.2 Case II: DUs with S < 2.............................................................................. 8 2.2 Questionnaire Dwelling Unit Selection Probability............................................. 10 3. Brief Description of the Generalized Exponential Model................................................ 13 4. Predictor Variables for the Questionnaire Dwelling Unit and Pair Weight Calibration via the Generalized Exponential Model............................................................................ 15 4.1 Questionnaire Dwelling Unit Weight Calibration................................................ 15 4.2 Pair Weight Calibration........................................................................................ 16 5. Definition of Extreme Weights......................................................................................... 21 5.1 Questionnaire Dwelling Unit Extreme Weight Definition................................... 21 5.2 Person Pair Extreme Weight Definition............................................................... 22 6. Weight Calibration at Questionnaire Dwelling Unit and Pair Levels............................... 25 6.1 Phase I SDU-Level Weight Components............................................................. 30 6.2 QDU Weight Components.................................................................................... 31 6.2.1 QDU Weight Component #11: Inverse of Selection Probability of at Least One Person in the Dwelling Unit............................................ 31 6.2.2 QDU Weight Component #12: Selected QDU Poststratification to SDU-Based Control Totals................................................................... 31 6.2.3 QDU Weight Component #13: Respondent QDU Nonresponse Adjustment................................................................................................ 31 6.2.4 QDU Weight Component #14: Respondent QDU Poststratification to SDU-Based Control Totals....................................................................... 32 6.2.5 QDU Weight Component #15: Respondent QDU Extreme Value Adjustment................................................................................................ 32 6.3 Pair-Level Weight Components............................................................................ 32 6.3.1 Pair Weight Component #11: Inverse of Selection Probability of a Person Pair in the Dwelling Unit....................................................... 32 6.3.2 Pair Weight Component #12: Selected Pair Poststratification to SDU-Based Control Totals................................................................... 32 6.3.3 Pair Weight Component #13: Respondent Pair Nonresponse Adjustment................................................................................................ 32 6.3.4 Pair Weight Component #14: Respondent Pair Poststratification to SDU-Based Control Totals................................................................... 33 6.3.5 Pair Weight Component #15: Respondent Pair Extreme Weight Adjustment................................................................................................ 33 v 7. Evaluation of Calibration Weights.................................................................................... 35 7.1 Response Rates..................................................................................................... 35 7.2 Proportions of Extreme Values and Outwinsors................................................... 35 7.3 Slippage Rates....................................................................................................... 36 7.4 Weight Adjustment Summary Statistics............................................................... 36 7.5 Sensitivity Analysis of Drug Use Estimates......................................................... 37 References..................................................................................................................................... 49 Appendix Page A Technical Details about the Generalized Exponential Model......................................... A-1 B Derivation of Poststratification Control Totals............................................................... B-1 C GEM Modeling Summary for the Questionnaire Dwelling Unit Weights..................... C-1 C.1: Model Group 1: Northeast…................................................................................ C-11 C.2: Model Group 2: Midwest….................................................................................. C-19 C.3: Model Group 3: South…....................................................................................... C-27 C.4: Model Group 4: West…........................................................................................ C-35 D Evaluation of Calibration Weights: Questionnaire Dwelling Unit-Level Response Rates................................................................................................................................ D-1 E Evaluation of Calibration Weights: Questionnaire Dwelling Unit-Level Proportions of Extreme Values and Outwinsors................................................................................ E-1 F Evaluation of Calibration Weights: Questionnaire Dwelling Unit-Level Slippage Rates…............................................................................................................................. F-1 G Evaluation of Calibration Weights: Questionnaire Dwelling Unit-Level Weight Summary Statistics.......................................................................................................... G-1 H GEM Modeling Summary for the Pair Weights............................................................. H-1 H.1: Model Group 1: Northeast and South…................................................................. H-5 H.2: Model Group 2: Midwest and West….................................................................. H-13 I Evaluation of Calibration Weights: Pair-Level Response Rates......................................I-1 J Evaluation of Calibration Weights: Pair-Level Proportions of Extreme Values and Outwinsors....................................................................................................................... J-1 K Evaluation of Calibration Weights: Pair-Level Slippage Rates...................................... K-1 L Evaluation of Calibration Weights: Pair-Level Weight Summary Statistics.................. L-1 vi List of Tables Table Page 1.1 2007–2011 NSDUH Sample Sizes..................................................................................... 2 1.2 Pair Domains....................................................................................................................... 3 2.1 Building Blocks of the QDU and Person Pair Samples: Dwelling Units and Persons in the 2007–2011 NSDUHs.............................................................................................. 11 6.1 Sample Size, by Model Group at QDU and Pair Levels................................................... 30 7.1 Estimates of Totals and SEs for Domains of Interest Based on QDU Sample: 2011....... 38 7.2a Percentages of Youths (12 to 17) Reporting Lifetime, Past Year, and Past Month Use of Alcohol and Tobacco among Mother-Child (12 to 17) Pairs, by Mother Use: 2011.......................................................................................................................... 39 7.2b Percentages of Youths (12 to 17) Reporting Lifetime, Past Year, and Past Month Use of Alcohol and Tobacco among Father-Child (12 to 17) Pairs, by Father Use: 2011.......................................................................................................................... 40 7.3a Percentages of Youths (12 to 17) Reporting Lifetime, Past Year, and Past Month Use of Any Illicit Drug or Marijuana among Mother-Child (12 to 17) Pairs, by Mother Use: 2011............................................................................................................. 41 7.3b Percentages of Youths (12 to 17) Reporting Lifetime, Past Year, and Past Month Use of Any Illicit Drug or Marijuana among Father-Child (12 to 17) Pairs, by Father Use: 2011.......................................................................................................... 42 7.4 Percentages of Youths (12 to 17) Living with a Parent Reporting Lifetime, Past Year, and Past Month Use of Alcohol and Tobacco among Parent-Child (12 to 17) Pairs, Asked Whether Their Parents Had Spoken to Them about the Dangers of Tobacco, Alcohol, or Drug Use within the Past 12 Months: 2011................................... 43 7.5 Percentages of Youths (12 to 17) Living with a Parent Reporting Lifetime, Past Year, and Past Month Use of Any Illicit Drug and Marijuana among Parent-Child (12 to 17) Pairs, Asked Whether Their Parents Had Spoken to Them about the Dangers of Tobacco, Alcohol, or Drug Use within the Past 12 Months: 2011................ 44 7.6a Percentages of Youths (12 to 17) Reporting Lifetime, Past Year, and Past Month Use of Alcohol and Tobacco among Mother-Child (12 to 17) Pairs, for Mother in the Pair, Asked Whether She Had Spoken to Her Children about the Dangers of Tobacco, Alcohol, or Drug Use within the Past 12 Months: 2011................................... 45 7.6b Percentages of Youths (12 to 17) Reporting Lifetime, Past Year, and Past Month Use of Alcohol and Tobacco among Father-Child (12 to 17) Pairs, for Father in the Pair, Asked Whether He Had Spoken to His Child about the Dangers of Tobacco, Alcohol, or Drug Use within the Past 12 Months: 2011................................... 46 7.7a Percentages of Youths (12 to 17) Reporting Lifetime, Past Year, and Past Month Use of Any Illicit Drug and Marijuana among Mother-Child (12 to 17) Pairs, for Mother in the Pair, Asked Whether She Had Spoken to Her Child about the Dangers of Tobacco, Alcohol, or Drug Use within the Past 12 Months: 2011................ 47 7.7b Percentages of Youths (12 to 17) Reporting Lifetime, Past Year, and Past Month Use of Any Illicit Drug and Marijuana among Father-Child (12 to 17) Pairs, for Father in the Pair, Asked Whether He Had Spoken to His Child about the Dangers of Tobacco, Alcohol, or Drug Use within the Past 12 Months: 2011................ 48 vii C.1a 2011 QDU Weight GEM Modeling Summary (Model Group 1: Northeast)............... C-13 C.1b 2011 Distribution of Weight Adjustment Factors and Weight Products (Model Group 1: Northeast)...................................................................................................... C-14 C.2a 2011 QDU Weight GEM Modeling Summary (Model Group 2: Midwest)................. C-21 C.2b 2011 Distribution of Weight Adjustment Factors and Weight Products (Model Group 2: Midwest)........................................................................................................ C-22 C.3a 2011 QDU Weight GEM Modeling Summary (Model Group 3: South)..................... C-29 C.3b 2011 Distribution of Weight Adjustment Factors and Weight Products (Model Group 3: South)............................................................................................................. C-30 C.4a 2011 QDU Weight GEM Modeling Summary (Model Group 4: West)...................... C-37 C.4b 2011 Distribution of Weight Adjustment Factors and Weight Products (Model Group 4: West).............................................................................................................. C-38 D.1 2011 NSDUH QDU-Level Response Rates................................................................... D-1 E.1 2011 NSDUH Selected QDU-Level Proportions of Extreme Values and Outwinsors...................................................................................................................... E-1 E.2 2011 NSDUH Respondent QDU-Level Proportions of Extreme Values and Outwinsors...................................................................................................................... E-3 F.1 2011 NSDUH QDU-Level Slippage Rates...................................................................... F-1 G.1 2011 NSDUH Selected QDU-Level Weight Summary Statistics.................................. G-1 G.2 2011 NSDUH Respondent QDU-Level Weight Summary Statistics............................. G-3 H.1a 2011 Pair Weight GEM Modeling Summary (Model Group 1: Northeast and South).............................................................................................................................. H-6 H.1b 2011 Distribution of Weight Adjustment Factors and Weight Products (Model Group 1: Northeast and South)....................................................................................... H-7 H.2a 2011 Pair Weight GEM Modeling Summary (Model Group 2: Midwest and West)............................................................................................................................. H-14 H.2b 2011 Distribution of Weight Adjustment Factors and Weight Products (Model Group 2: Midwest and West)........................................................................................ H-15 I.1 2011 NSDUH Person Pair-Level Response Rates............................................................I-1 J.1 2011 NSDUH Selected Pair-Level Proportions of Extreme Values and Outwinsors....................................................................................................................... J-1 J.2 2011 NSDUH Respondent Pair-Level Proportions of Extreme Values and Outwinsors (res.pr.nr)...................................................................................................... J-3 J.3 2011 NSDUH Respondent Pair-Level Proportions of Extreme Values and Outwinsors (res.pr.ps and res.pr.ev)................................................................................ J-5 K.1 2011 NSDUH Respondent Pair-Level Slippage Rates................................................... K-1 L.1 2011 NSDUH Selected Pair-Level Weight Summary Statistics..................................... L-1 L.2 2011 NSDUH Respondent Pair-Level Weight Summary Statistics (res.pr.nr).............. L-4 L.3 2011 NSDUH Respondent Pair-Level Weight Summary Statistics (res.pr.ps and res.pr.ev)................................................................................................................... L-7 viii List of Exhibits Exhibit Page 1.1 QDU and Pair Sampling Weight Calibration Steps............................................................ 4 4.1 Definitions of Levels for QDU-Level Calibration Modeling Variables........................... 17 4.2 Definitions of Levels for Pair-Level Calibration Modeling Variables............................. 18 6.1 Summary of 2011 NSDUH QDU Sample Weight Components...................................... 27 6.2 Summary of 2011 NSDUH Person Pair Sample Weight Components............................. 28 6.3 U.S. Census Bureau Regions/Model Groups.................................................................... 29 C.1 Definitions of Levels for QDU-Level Calibration Modeling Variables......................... C-3 C.2 Covariates for 2011 NSDUH Questionnaire Dwelling Unit Weights.......................... C-10 C.1.1 Covariates for 2011 NSDUH Questionnaire Dwelling Unit Weights (sel.qdu.ps) Model Group 1: Northeast............................................................................................ C-16 C.1.2 Covariates for 2011 NSDUH Questionnaire Dwelling Unit Weights (res.qdu.nr) Model Group 1: Northeast............................................................................................ C-17 C.1.3 Covariates for 2011 NSDUH Questionnaire Dwelling Unit Weights (res.qdu.ps) Model Group 1: Northeast............................................................................................ C-18 C.2.1 Covariates for 2011 NSDUH Questionnaire Dwelling Unit Weights (sel.qdu.ps) Model Group 2: Midwest.............................................................................................. C-24 C.2.2 Covariates for 2011 NSDUH Questionnaire Dwelling Unit Weights (res.qdu.nr) Model Group 2: Midwest.............................................................................................. C-25 C.2.3 Covariates for 2011 NSDUH Questionnaire Dwelling Unit Weights (res.qdu.ps) Model Group 2: Midwest.............................................................................................. C-26 C.3.1 Covariates for 2011 NSDUH Questionnaire Dwelling Unit Weights (sel.qdu.ps) Model Group 3: South.................................................................................................. C-32 C.3.2 Covariates for 2011 NSDUH Questionnaire Dwelling Unit Weights (res.qdu.nr) Model Group 3: South.................................................................................................. C-33 C.3.3 Covariates for 2011 NSDUH Questionnaire Dwelling Unit Weights (res.qdu.ps) Model Group 3: South.................................................................................................. C-34 C.4.1 Covariates for 2011 NSDUH Questionnaire Dwelling Unit Weights (sel.qdu.ps) Model Group 4: West.................................................................................................... C-40 C.4.2 Covariates for 2011 NSDUH Questionnaire Dwelling Unit Weights (res.qdu.nr) Model Group 4: West.................................................................................................... C-41 C.4.3 Covariates for 2011 NSDUH Questionnaire Dwelling Unit Weights (res.qdu.ps) Model Group 4: West.................................................................................................... C-42 H.1 Definitions of Levels for Pair-Level Calibration Modeling Variables........................... H-2 H.2 Covariates for 2011 NSDUH Pair Weights.................................................................... H-4 H.1.1 Covariates for 2011 NSDUH Pair Weights (sel.pr.ps) Model Group 1: Northeast and South........................................................................................................................ H-9 H.1.2 Covariates for 2011 NSDUH Pair Weights (res.pr.nr) Model Group 1: Northeast and South...................................................................................................................... H-10 H.1.3 Covariates for 2011 NSDUH Pair Weights (res.pr.ps) Model Group 1: Northeast and South...................................................................................................................... H-11 H.1.4 Covariates for 2011 NSDUH Pair Weights (res.pr.ev) Model Group 1: Northeast and South...................................................................................................................... H-12 ix H.2.1 Covariates for 2011 NSDUH Pair Weights (sel.pr.ps) Model Group 2: Midwest and West........................................................................................................................ H-17 H.2.2 Covariates for 2011 NSDUH Pair Weights (res.pr.nr) Model Group 2: Midwest and West........................................................................................................................ H-18 H.2.3 Covariates for 2011 NSDUH Pair Weights (res.pr.ps) Model Group 2: Midwest and West........................................................................................................................ H-19 H.2.4 Covariates for 2011 NSDUH Pair Weights (res.pr.ev) Model Group 2: Midwest and West........................................................................................................................ H-20 x List of Terms and Abbreviations DU Dwelling unit. Ev Extreme value. See Sections 5.1 and 5.2 for more detail. GEM Generalized exponential model. See Chapter 3 for more detail. Household-level The number of pairs associated with a given domain in a given household. person count These counts are used as control totals in the poststratification step. See Chapter 10 in the imputation report (Frechtel et al., 2013) for details on how these counts are created, and Chapter 4 for details on their use in poststratification. IQR Interquartile range. Multiplicity factor The number of pairs associated with a given respondent in a given domain. See Frechtel et al. (2013) for more detail. Nr Nonresponse. Outwinsor The proportion of weights trimmed after extreme value adjustment via winsorization. Pair domain A pair relationship where the target population is defined by one of the pair members, conditional on the attributes of the other pair member. Pair relationship The relationship between selected pair members. Parent-child A pair relationship where either both pair members identify the other as part of a parent-child relationship, or both pair members otherwise are determined to form a parent-child pair (either through other evidence or through imputation). ps Poststratification. QDU Questionnaire dwelling unit: a household where at least one member responded to the questionnaire. res.pr.nr Respondent pair nonresponse adjustment step. See Section 6.3.3 for more detail. res.qdu.nr Respondent questionnaire dwelling unit nonresponse adjustment step. See Section 6.2.3 for more detail. res.pr.ev Respondent pair extreme value adjustment step. See Section 6.3.5 for more detail. res.qdu.ev Respondent questionnaire dwelling unit extreme value adjustment step. See Section 6.2.5 for more detail. xi res.pr.ps Respondent pair poststratification adjustment step. See Section 6.3.4 for more detail. res.qdu.ps Respondent questionnaire dwelling unit poststratification adjustment step. See Section 6.2.4 for more detail. SDU Screener dwelling unit: a household where screener information is available. sel.pr.ps Selected person pair poststratification adjustment step. See Section 6.3.2 for more detail. sel.qdu.ps Selected questionnaire dwelling unit poststratification adjustment step. See Section 6.2.2 for more detail. Sibling-sibling A pair relationship where the pair members are siblings (either reported to be so, or otherwise determined to be so). Spouse-spouse A pair relationship where the pair members are either married or living together as though married (either reported to be so, or otherwise determined to be so). SS State sampling. UWE Unequal weighting effect. It refers to the contribution in the design effect due to unequal selection probability and is defined as 1 + [(n - 1)/n]*CV2, where CV = coefficient of variation of weights and n is the sample size. Winsorization A method of extreme value adjustment that replaces extreme values with the critical values used for defining low and high extreme values. xii 1. Introduction Traditionally, most household surveys have been designed either to measure characteristics of the entire household or to focus on a randomly selected respondent from among those determined to be eligible for the survey. Selecting more than one person from the same household is generally avoided since persons from the same household often exhibit the same or similar characteristics and behavioral patterns. The intra-class correlation found among members of the same household leads to a clustering effect on the variance of estimates resulting in less precise estimates compared with estimates of the same sample size from a simple random sample. Selecting only one person per household avoids this clustering effect on the variance. The "one person per household" sampling approach, however, precludes the opportunity to gather information about the relationships among household members. In the National Survey on Drug Use and Health (NSDUH),2 we allow for a richer analytic capability of a survey designed to ensure a positive pairwise probability of selection among all eligible household members in each sample household. Achieving positive probabilities for all pairs within sampled households permits unbiased estimation of the within-dwelling-unit component of variance. Besides providing efficient data collection, this sampling method also facilitates the study of the relationships of social behaviors among members of the same household. This report documents the methodology and development of calibrated weights for the second objective, the study of behavioral relationships among persons residing in the same household. The report also describes the development of questionnaire dwelling unit (QDU) weights, which are of independent interest for studying household-level characteristics and also are needed for producing household count estimates of the number of persons belonging to pair relationship domains for use as poststratification controls for pair weights. NSDUH allows for estimating characteristics at the person level, pair level, and household or QDU level. This report describes the weight calibration methods used for the pair- and QDU-level respondents. As described in the person-level report, NSDUH is an annual survey of about 67,500 persons selected from the civilian, noninstitutionalized population aged 12 or older from all 50 States and the District of Columbia. Unique to 2011 was a Gulf Coast Oversample (GCO) consisting of approximately 2,000 respondents in designated counties in Alabama, Florida, Louisiana, and Mississippi. This supplemental sample was used to study the impact of the April 2010 Deepwater Horizon oil spill on substance use and mental health and resulted in increasing the target national sample from 67,500 to 69,500. Based on a composite size measure, States were geographically partitioned into roughly equal-sized regions according to population. The 42 smaller States and the District of Columbia were partitioned into 12 State sampling (SS) regions, whereas the eight largest States were divided into 48 SS regions. Therefore, the partitioning of the United States resulted in the formation of a total of 900 SS regions. Under a stratified design with States serving as the primary strata and SS regions serving as the secondary strata, census tracts, segments within census tracts, and dwelling units (DUs) within segments were each selected using probability proportional to size sampling. NSDUH is sometimes referred to as a two-phase sample where the first phase consisted of a large number of screener dwelling units (SDUs, about 200,000) selected to ensure that various age groups (five in 2 This report presents information from the 2011 National Survey on Drug Use and Health (NSDUH). Prior to 2002, the survey was called the National Household Survey on Drug Abuse (NHSDA). 1 all: 12 to 17, 18 to 25, 26 to 34, 35 to 49, and 50+) of eligible individuals were represented adequately in the second phase. Information collected from SDUs also provided estimates of population controls (as in two-phase sampling) for calibration at levels (such as pair and QDU) for which suitable U.S. Census Bureau-based controls were not available. The second phase consisted of the selection of zero, one, or two persons from each selected SDU using a modification of Brewer's method such that prescribed sampling rates for the five age groups in each State were achieved with high selection rates for youths (12 to 17) and young adults (18 to 25). Table 1.1 shows the eligible number of selected and responding SDUs, QDUs, pairs, and persons for each of the 5 years (2007–2011). The distribution of pair data for different pairs of age groups may vary considerably (see Chapter 2 for details). It is seen that for certain age group domains, the realized sample size may not be sufficient to yield reliable estimates. Also, there may be problems of extreme weights due to small pair selection probabilities under Brewer's method that may cause instability of estimates. These and some other estimation issues related to pair data are discussed below, along with some adopted solutions. Table 1.1 2007–2011 NSDUH Sample Sizes Sample Unit 2007 2008 2009 2010 2011 Selected 158,377 160,114 161,377 166,532 179,293 SDU Completed 140,659 142,159 142,933 147,010 156,048 Selected 58,574 58,942 58,288 58,702 61,441 QDU Completed 47,709 48,180 48,088 48,113 50,133 Selected 26,696 26,769 26,497 26,295 27,095 Pair Completed 19,668 19,748 19,919 19,691 19,976 Selected 85,270 85,711 84,785 84,997 88,536 Person Completed 67,377 67,928 68,007 67,804 70,109 Note: The 2007–2010 sample sizes reflect the removal of falsified cases found in Pennsylvania and Maryland. The 2011 sample was not affected. For additional information, see Section B.3.5 in Appendix B of the Results from the 2011 National Survey on Drug Use and Health: Summary of National Findings (Center for Behavioral Health Statistics and Quality, 2012). First, we note that for studying drug-related behavioral relationships among members of the same household, pair data is required because the outcome variable generally is defined with respect to the specific other member selected from the household. However, the parameter of interest is generally at the person level and is not at the pair level. For example, in the parent- child pairs, one may be interested in the proportion of children that have used drugs in the past year who have parents that report talking to their child about drugs. Here the target population consists only of children, and not all possible pairs. Note that the pair-level (two persons per QDU) sample forms a subsample of the larger person-level (one or two persons per QDU) sample, with the QDUs themselves selected from the larger sample of SDUs. NSDUH has features of a two-phase design, which turns out to be useful for estimating calibration controls for poststratification of household-level weights and person pair-level weights. No other outside source is available for obtaining these controls. For this purpose, the screener-level household weights are poststratified to person-level census counts to obtain more efficient estimated controls for pair and household data. In estimation for pair domains, two major problems arise: one is that of multiplicities because, for a given domain defined by the pair relationship, when the parameter of interest is at 2 the person level, several pairs in the household could be associated with the same person, For example, analysts are interested in an outcome at the person level, the proportion of children who use drugs and whose parents report talking to them about drugs, where the focus is on the child in a parent-child pair. Several parent-child pairs in the household could be associated with the same child. If the household has two parents, the selected child has two inclusion possibilities (one with each parent) in the set of all such parent-child pairs (Frechtel et al., 2013). The other problem is that of extreme weights that may arise due to small selection probabilities for certain pair age groups, which may lead to unstable estimates. Each of these issues is discussed in turn. If several pairs in the household are associated with the same person, it is necessary to use the average measure of behavior relationships for each member, which gives rise to multiplicities. Thus, the pair weights need to be divided by the person-level multiplicity factors for each domain of interest, and, therefore, multiplicity factors need to be produced along with the final set of calibrated weights. Because it is not straightforward to create these multiplicities, analyses would have to be necessarily limited to pair relationships where the multiplicities were produced a priori. It was anticipated that analyses of interest would be limited to 14 pair domains, listed in Table 1.2. Since no multiplicity was necessary for the spouse-spouse/partner- partner pair relationships (by definition, each pair member could have only one partner or one spouse), multiplicity factors were produced for only 12 of these domains. Note that a single pair relationship might have two domains associated with it, since the parameter of interest might be associated with only one member of the pair (the "focus" member), and the multiplicity would differ depending upon which pair member was the focus member. Table 1.2 Pair Domains Pair Relationship Focus Parent-child: parent, child aged 12-14 Parent Parent-child: parent, child aged 12-14 Child Parent-child: parent, child aged 12-17 Parent Parent-child: parent, child aged 12-17 Child Parent-child: parent, child aged 12-20 Parent Parent-child: parent, child aged 12-20 Child Parent-child: parent, child aged 15-17 Parent Parent-child: parent, child aged 15-17 Child Sibling-sibling: older sibling 15-17, younger sibling 12-14 Older sibling Sibling-sibling: older sibling 15-17, younger sibling 12-14 Younger sibling Sibling-sibling: older sibling 18-25, younger sibling 12-17 Older sibling Sibling-sibling: older sibling 18-25, younger sibling 12-17 Younger sibling Spouse-spouse or partner-partner, with or without children No multiplicity necessary Spouse-spouse or partner-partner, with children aged 0-17 No multiplicity necessary Some of the multiplicities, including counts of all possible pairs in a household for a given domain, were used for poststratification. Details are provided in Chapter 4. Prior editions of this report contained a chapter on editing and imputation of pair relationships, multiplicity factors, and household-level person counts for poststratification, but this information has been 3 removed in the interest of consolidating the imputation documentation and can now be found in the imputation report (Frechtel et al., 2013). A resolution to the extreme weight problem is to use a Hajek-type modification (Hajek, 1971). This modification essentially entails calibration (like poststratification) to controls for the number of persons in households belonging to each domain of interest. These controls can be obtained from the larger sample of singles and pairs (i.e., one or two persons selected from DUs). Note, however, that the multiplicity factor, being domain specific, renders the calibration adjustment factor domain specific. This raises the question of finding one set of calibration weights for use with all domains or outcome variables. To get around this problem, we performed a multivariate calibration with respect to a key set of pair domains. This type of poststratification then was followed by a repeat poststratification to further control the extreme weights by imposing separate bound restrictions on the initially identified extreme weights. The generalized exponential model (GEM) method (Folsom & Singh, 2000) was used for calibration of both QDU- and pair-level design weights through several steps of adjustment as shown in Exhibit 1.1. In GEM, treatment of extreme value (ev) weights is built in via the definition of lower and upper bounds for the extreme weights. For pair data, there was a problem defining suitable domains for defining extreme weights, as explained in the following paragraphs. Exhibit 1.1 QDU and Pair Sampling Weight Calibration Steps In dealing with extreme weights, it is assumed that they arise due to design (due to an imperfect frame, assignment of very small selection probabilities to some units, or a small weight adjustment factor after calibration) so that they make the sample representative of the population 4 and, hence, do not introduce bias. The only problem is that they may lead to highly unstable estimates similar to the problem of Basu's circus elephants3 (Hajek, 1971). So, we need to perform some treatment (such as winsorization4) within suitably defined extreme weight domains such that these domains contain units possibly from different strata but with similar sample selection probabilities to avoid the occurrence of extreme weights due to a mix of different designs. The domains must be large enough (e.g., at least size 30) to be able to define extreme values according to the domain-specific weight distribution. Any extreme value treatment to increase precision of estimates would introduce some bias. However, this bias can be reduced considerably if the ev treatment is performed under calibration controls. This is what the built-in ev control in GEM tries to accomplish. It follows that the definition of extreme weight domains should depend on factors that affect the selection probabilities of units in the sample, such as State- and age-specific sampling rates, segment selection probabilities, pair age-specific selection probabilities, and household composition. If one tries to define extreme weight domains by taking account of all these factors via cross-classification, it will lead to too many domains with insufficient observations. That is why it is difficult to define suitable extreme weight domains for pair data. In the case of person- level weights it was less difficult, since State by age group suitably captured the extreme weight domain requirements. The definition of extreme weight domains used in the 2011 survey was the same as the one used in the 1999–2010 surveys. The domains were defined as the cross- classification of State, pair age,5 and number of persons aged 12 to 25 in a household. In particular, the pair age was defined by the age groups of each pair member according to the age categories of 12 to 25, 26 to 49, and 50 or older (resulting in six pair age categories), and the number of persons aged 12 to 25 were categorically defined as zero, one, and two or more. For more details, see Chapter 5. 3 A circus owner had 50 elephants, and wanted to estimate the total weight to help him make arrangements for shipping. To save time, he only wanted to weigh Sambo (an average sized elephant), and use 50 times its weight as an estimate. However, the circus statistician, being highly conscious of the optimality and unbiasedness of the Horvitz-Thompson (HT) estimator, objected about the potential bias of his estimate because of the purposive selection. Instead, he suggested random selection of an elephant with a very high probability of 99/100 for Sambo, and the rest including Jumbo (the biggest in the herd) with probability 1/4900 each. The circus owner was very unhappy with the statistician's response of 100/99 times the Sambo's weight as the estimate if Sambo got selected in this random draw, and was outraged with the response of 4900 times the Jumbo's weight if Jumbo happened to get selected. It was obvious to the owner that this new estimator was extremely poor, although he didn't know anything about its unbiasedness. The story had an unhappy ending with the circus statistician losing his job. To alleviate the instability of the HT-estimator, Hajek suggested to multiply it by 50 divided by inverse of the selection probability, which reduces simply to 50 times the weight of the selected elephant. 4 Winsorization is a method of extreme value adjustment that replaces extreme values with the critical values used for defining low and high extreme values. 5 Pair age in this case should not be confused with the modeling term, which has a finer level breakdown. 5 6 2. Questionnaire Dwelling Unit and Pair Selection Probabilities Similar to the 1999–2001 National Household Surveys on Drug Abuse (NHSDAs) and the 2002–2010 National Surveys on Drug Use and Health (NSDUHs),6 the 2011 NSDUH had a two-phase design and used a computer-assisted interviewing (CAI) method. There were four stages of selection: census tracts, segments within census tracts, dwelling units (DUs) within segments, and persons within dwelling units. Any two survey eligible persons had some nonzero chance of being selected and, when both were selected, they formed a within household pair. This design feature is of interest to NSDUH researchers because, for example, it allows analysts to examine how the drug use propensity of an individual (in a family) relates to the drug use propensity of other members residing in the same dwelling unit (Morton, Martin, Shook-Sa, Chromy, & Hirsch, 2012). For the 1999–2001 surveys, the method used for selecting pairs was as follows. For a given DU, if the sum of the age-specific selection probabilities was larger than 2, then the individual person-selection probabilities were ratio adjusted downward to make the sum equal to 2. If the sum was less than 2, the difference between 2 and the sum of the probabilities was evenly distributed over three dummy persons so that the sum of the person probabilities was made to equal 2. Brewer's method was then applied to select a person pair. If the selected pair consisted of two real persons, then both persons were selected. If the selected pair consisted of one real person and one dummy person, then the real person was selected. If the selected pair consisted of two dummy persons, no one was selected from that DU. Starting with the 2002 NSDUH and continuing through 2011, the pair-sampling algorithm was modified to increase the number of pairs selected in the sample. Dwelling units with the sum of person selection probabilities greater than or equal to 2 were treated the same as in previous survey years. However, DUs where the sum of person-level selection probabilities was less than 2 received a slightly different treatment that increased the chance for selecting a pair of real persons. Section 2.1 describes the selection process for both types of DUs. Table 2.1 provides a summary of these NSDUH sampling units: eligible and completed screening dwelling units (SDUs), selected and completed questionnaire dwelling units (QDUs), selected and completed person interviews, and selected and completed person pairs, as well as their response rates. Using Brewer's method, zero, one, or two individuals were selected per household. Those SDUs where at least one person was selected were counted as the selected QDUs. A QDU where two persons were selected and both had completed interviews was considered to be a completed person pair. The table provides a breakdown by age group at the person level and age group by selection group (none, single, or pair) at the person pair level. 6 This report presents information from the 2011 National Survey on Drug Use and Health (NSDUH). Prior to 2002, the survey was called the National Household Survey on Drug Abuse (NHSDA). 7 2.1 Pair Selection Probability 2.1.1 Case I: DUs with S ≥ 2 For a given DU, if the sum of the age-specific person selection probabilities (S) was larger than 2, then the selection probability was ratio adjusted by a multiplicative adjustment factor so that all probabilities were scaled down to sum to exactly 2. Now, Brewer's method sets the pairwise selection probabilities at P P 1 1 Ph (ij ) = h (i ) h ( j ) + (2.1) K 1 − Ph (i ) 1 − Ph ( j ) by setting K at Ph( i ) K = 2+ , (2.2) 1 − Ph( i ) where i = ith person in household h (whose selection probability depends on his or her age category: 1, 2, 3, 4, or 5) and j = jth person in household h (whose selection probability depends on his or her age category: 1, 2, 3, 4, or 5), where age category 1 corresponds to persons aged 12 to 17, 2 to persons aged 18 to 25, 3 to persons aged 26 to 34, 4 to persons aged 35 to 49, and 5 to persons aged 50 or older. The sum of the pairwise selection probabilities taken over all unique pairs will be guaranteed to be exactly 1. P i j> i h (ij ) =1 (2.3) It also guarantees that the sum of the pairwise selection probabilities for an individual is equal to the individual's selection probability P h ( ij ) = Ph(i) j ≠i (2.4) for all values of i. Note the above scheme always selects a pair of two eligible persons. 2.1.2 Case II: DUs with S < 2 If the sum S of person-level selection probabilities was less than 2, the method used in survey years 1991 to 2001 consisted of dividing 2 − S equally among the three dummy persons 8 added to the household, and then used Brewer's method to select a pair, as in Case I. However, if the household had two or more persons, we preferred a pair of real persons to have a greater chance of being selected. To achieve this goal, the individual selection probabilities, Ph(i) , were scaled upward by the factor Fs such that their sum came close to but did not exceed 2 and such that each person selection probability did not exceed the maximum allowed probability of 0.99. Thus, denoting the revised person selection probabilities by P'h(i) , the factor Fs is given by T ( λ) 0.99 Fs = Min , , (2.5) S max { P } h( i) where T( λ ) = S + λ (2 – S) and λ is set to 0.5. Note that if λ is chosen as 0, then Fs = 1 and the selection scheme would follow that of Case I. The individual person probabilities are scaled upward by the factor Fs so they either sum to 2 or sum as close to 2 as possible. Denote S' as the sum of the selection probability after scale adjustment by Fs. If S' is exactly 2, then dummy persons are not needed. If S' is less than 2, then three dummy persons are added to the DU. Now, for Brewer's method, we set the pairwise selection probabilities similar to (2.1), as Ph′( i ) Ph′( j ) 1 1 Ph′(ij ) = + (2.6) K ′ 1 − Ph′(i ) 1 − Ph′( j ) by setting K' at Ph′(i ) K ′= 2 + , (2.7) i 1− Ph′( i) where P′h(i) and P′h(j) are the selection probabilities adjusted by the scaling factor Fs , where i = ith person in the household (whose selection probability depends on his or her age category: 0, 1, 2, 3, 4, or 5), j = jth person in the household (whose selection probability depends on his or her age category: 0, 1, 2, 3, 4, or 5), and where age category 0 corresponds to dummy persons, and categories 1 to 5 are defined as in Case I. Note that we now have P′ j ≠i h (ij ) = Ph′( i ). To maintain the original person selection probabilities despite the scale adjustment by Fs , we modified Brewer's method as follows. First, draw a random number, R, from a uniform (0,1) distribution. If R ≤ 1/Fs, then select a pair using Brewer's method based on formula (2.6). However, if R > 1/Fs, then no persons are selected from 9 the household. In this way, the probability for selecting a pair (i,j) in household h becomes P*h(ij) = P′h(ij)/Fs, which, in turn, gives the original person selection probabilities, Ph(i). Unlike Case I, where a pair of eligible persons was always selected, this adjusted selection scheme allows for zero, one, or two persons to be selected from a DU. 2.2 Questionnaire Dwelling Unit Selection Probability A dwelling unit was considered a selected QDU if it had completed the screening interview and had at least one person selected for the questionnaire interview. QDUs with at least one respondent were considered respondent QDUs. The QDU selection probability was defined as (2.8) ( ) Ph* = 1 − Ph*(00) , where P*h(00) is the probability of not selecting any person. For the DUs with an unadjusted sum of age-specific selection probabilities larger than or equal to 2 (Case I), P*h(00) is 0. It follows from Section 2.1, under Case II, P*h(00) can be calculated as 1 3 Ph′( 0) Ph′( 0) 1 1 Ph*( 00) = 1 − + + , (2.9) Fs Fs K ′ 1 − Ph′(0) 1 − Ph′( 0) where P′h(0) is the selection probability of a dummy person when person selection probabilities are adjusted by Fs. 10 Table 2.1 Building Blocks of the QDU and Person Pair Samples: Dwelling Units and Persons in the 2007–2011 NSDUHs 2007 2008 2009 2010 2011 1 2 3 1 2 3 1 2 3 1 2 3 1 Domain Sel. Resp. % Rate Sel. Resp. % Rate Sel. Resp. % Rate Sel. Resp. % Rate Sel. Resp.2 % Rate3 DUs Total DUs Screened 158,377 140,659 88.81 160,114 142,159 88.79 161,377 142,933 88.57 166,532 147,010 88.28 179,293 156,048 87.04 QDUs Total QDUs 58,574 47,709 81.45 58,942 48,180 81.74 58,288 48,088 82.50 58,702 48,113 81.96 61,441 50,133 81.60 Persons Total Persons 85,270 67,377 79.02 85,711 67,928 79.25 84,785 68,007 80.21 84,997 67,804 79.77 88,536 70,109 79.19 12-17 26,039 22,286 85.59 26,228 22,250 84.83 26,157 22,398 85.63 25,908 21,960 84.76 27,911 23,510 84.23 18-25 27,910 22,006 78.85 28,793 22,875 79.45 28,158 22,686 80.57 28,164 22,793 80.93 28,589 22,876 80.02 26-34 8,865 6,861 77.39 8,337 6,560 78.69 8,242 6,591 79.97 8,545 6,780 79.34 8,323 6,543 78.61 35-49 13,411 9,906 73.86 12,995 9,674 74.44 12,855 9,616 74.80 12,979 9,668 74.49 12,220 9,149 74.87 50+ 9,045 6,318 69.85 9,358 6,569 70.20 9,373 6,716 71.65 9,401 6,603 70.24 11,493 8,031 69.88 Pairs Total Pairs4 26,696 19,668 73.67 26,769 19,748 73.77 26,497 19,919 75.17 26,295 19,691 74.88 27,095 19,976 73.73 0,05 82,085 N/A N/A 83,217 N/A N/A 84,645 N/A N/A 88,308 N/A N/A 94,607 N/A N/A 0, 12-17 8,416 7,966 94.65 8,634 8,094 93.75 8,432 7,936 94.12 8,595 7,906 91.98 9,402 8,651 92.01 0, 18-25 9,738 8,870 91.09 9,932 9,213 92.76 9,870 9,081 92.01 10,093 9,270 91.85 10,306 9,497 92.15 0, 26-34 3,972 3,412 85.90 3,771 3,303 87.59 3,798 3,318 87.36 3,914 3,418 87.33 3,930 3,386 86.16 0, 35-49 4,796 3,956 82.49 4,601 3,835 83.35 4,565 3,810 83.46 4,659 3,843 82.49 4,431 3,704 83.59 11 0, 50+ 4,956 3,836 77.40 5,235 4,013 76.66 5,126 4,042 78.85 5,146 3,998 77.69 6,277 4,919 78.37 12-17, 12-17 4,347 3,691 84.91 4,319 3,654 84.60 4,288 3,648 85.07 4,160 3,525 84.74 4,649 3,885 83.57 12-17, 18-25 3,353 2,596 77.42 3,449 2,668 77.36 3,595 2,852 79.33 3,444 2,718 78.92 3,756 2,921 77.77 12-17, 26-34 917 743 81.03 897 690 76.92 872 724 83.03 922 752 81.56 834 685 82.13 12-17, 35-49 3,996 3,106 77.73 3,944 3,012 76.37 3,979 3,061 76.93 3,948 3,044 77.10 3,855 2,918 75.69 12-17, 50+ 663 492 74.21 666 482 72.37 703 532 75.68 679 493 72.61 766 565 73.76 18-25, 18-25 5,602 4,082 72.87 5,872 4,261 72.56 5,588 4,168 74.59 5,502 4,165 75.70 5,476 4,015 73.32 18-25, 26-34 1,207 865 71.67 1,103 821 74.43 1,102 820 74.41 1,140 851 74.65 1,049 768 73.21 18-25, 35-49 1,666 1,058 63.51 1,706 1,112 65.18 1,576 1,059 67.20 1,639 1,098 66.99 1,469 994 67.67 18-25, 50+ 742 454 61.19 859 546 63.56 839 547 65.20 844 537 63.63 1,057 666 63.01 26-34, 26-34 1,017 692 68.04 890 614 68.99 886 635 71.67 903 621 68.77 858 599 69.81 26-34, 35-49 517 344 66.54 545 380 69.72 447 312 69.80 512 354 69.14 492 314 63.82 26-34, 50+ 218 113 51.83 241 145 60.17 251 149 59.36 251 162 64.54 302 192 63.58 35-49, 35-49 1,009 599 59.37 873 535 61.28 917 569 62.05 886 542 61.17 748 474 63.37 35-49, 50+ 418 244 58.37 453 269 59.38 454 239 52.64 449 245 54.57 477 271 56.81 50+, 50+ 1,024 589 57.52 952 559 58.72 1,000 604 60.40 1,016 584 57.48 1,307 709 54.25 DU = dwelling unit; N/A = not applicable; QDU = questionnaire dwelling unit. Note: The 2007–2010 sample sizes reflect the removal of falsified cases found in Pennsylvania and Maryland. The 2011 sample was not affected. For additional information, see Section B.3.5 in Appendix B of the Results from the 2011 National Survey on Drug Use and Health: Summary of National Findings (Center for Behavioral Health Statistics and Quality, 2012). 1 Selected pairs are based on the screener age. 2 Respondent pairs are based on the questionnaire age and are comprised only of respondent persons. 3 These rates are unweighted and based only on the total selected and total responding counts of pairs. 4 Total pairs excludes dummy person pairs. 5 Among the completed screening dwelling units, no person was selected in this dwelling unit. 12 3. Brief Description of the Generalized Exponential Model In survey practice, design-based weights are typically adjusted in three steps: (1) for extreme values (ev) via winsorization, (2) for nonresponse (nr) via weighting classes, and (3) for poststratification (ps) via raking ratio adjustments. If weights are not treated for extreme values, the resulting estimates, although unbiased, will tend to have low precision. The bias introduced by winsorization is alleviated to some extent through ps. The nr adjustment is a correction for bias introduced in estimates based only on responding units, and ps is an adjustment for coverage (typically undercoverage) bias and variance reduction due to correlation between the study and control (usually demographic) variables. There are limitations in the existing methods of weight adjustment for ev, nr, and ps. It would be desirable to adjust for bias introduced in the ev step (when extreme weights are treated via winsorization) in that the sample distribution for various demographic characteristics is preserved. For the nr step, there are general raking type methods, such as the scaled constrained exponential model developed by Folsom and Witt (1994), where the lower and upper bounds can be suitably chosen by use of a separate scaling factor. The factor is set as the inverse of the overall response propensity. It would be desirable to have a model for the nr adjustment factor so that the desired lower and upper bounds on the factor are part of the model. Note that the lower bound on the nr adjustment factor should be one, as it is interpreted as the inverse of the probability of response for a particular unit. For the ps step, on the other hand, the general calibration methods of Deville and Särndal (1992), such as the logit method, allow for built-in lower (L) and upper (U) bounds (for ps, typically L < 1 < U). However, it would be desirable to have nonuniform bounds ( Lk ,U k ) depending on the unit k such that the final adjusted weight, wk , could be controlled within certain limits. An important application of this feature would be weight adjustments in the presence of ev to allow some control on the final adjustment of the initially identified extreme values. A modification of the earlier method of the scaled constrained exponential model of Folsom and Witt (1994), termed as the method of the generalized exponential model (GEM) and proposed by Folsom and Singh (2000), provides a unified approach to the three weight adjustments for ev, nr, and ps, and it has the desired features mentioned above. The functional form of the GEM adjustment factor is provided in Appendix A. It generalizes the logit model of Deville and Särndal (1992), typically used for ps, such that the bounds (L, U) may depend on k. Thus, it provides a built-in control on ev during both ps and nr adjustments. In addition, the bounds are internal to the model and can be set to chosen values (e.g., Lk = 1 in the nr step). If there is a low frequency of ev in the final ps, then a separate ev step may not be necessary. In fitting GEM to a particular problem, the choice of a large number of predictor variables along with tight bounds will have an impact on the resulting unequal weighting effect (UWE) and the proportion of extreme values. In practice, this leads to somewhat subjective considerations of trade-off between the target set of bounds for a given set of factor effects and the target UWE and the target proportion of extreme values. It also may be beneficial to look at 13 the proportion of "outwinsors" (a term coined to signify the extent of residual weights after winsorization), which is probably more realistic in determining the robustness of estimates in the presence of extreme values. A large increase in the number of predictor variables in GEM typically would result in a higher UWE, thus indicating a possible loss in precision. This was checked by comparing SUDAAN-based standard errors of a key set of estimates computed from two sets of calibration models, one baseline using only the main effects and the other using the final model. The results are presented in Chapter 7. To implement GEM, several steps need to be followed: (1) define and create all the covariates; (2) define the extreme weights; (3) fit the GEM model. The details of practical aspects of GEM implementation can be found in Chapters 4 and 5 of this report and Chapter 4 of Chen et al. (2013). 14 4. Predictor Variables for the Questionnaire Dwelling Unit and Pair Weight Calibration via the Generalized Exponential Model We note that unlike the person-level weight calibration, the control totals for the questionnaire dwelling unit (QDU)-level and person pair-level poststratification are not available from the U.S. Census Bureau. A way around this problem is to take advantage of the two-phase nature of the design, in which the screener data provides a large sample containing demographic information that can be used to derive control totals for the QDU-level and person pair-level sampling weight calibrations, as well as for the selected person poststratification adjustment. The stability of control totals from the screener dwelling unit (SDU)-level data can be improved by poststratification of the SDU sample using person-level counts from the census. This was indeed done and is documented in the person-level weight calibration report (Chen et al., 2013). 4.1 Questionnaire Dwelling Unit Weight Calibration After the nonresponse and poststratification adjustments at the SDU level, which are common to the person-level weight calibration, the QDU sample weights were adjusted in three steps: poststratification of selected QDUs, nonresponse adjustment of respondent QDUs, and poststratification of respondent QDUs. The set of initially proposed predictor variables for these adjustments using generalized exponential model (GEM) were set to be common and to correspond to those used for the SDU nonresponse and poststratification adjustments. The variables are of two types: Those used for SDU nonresponse adjustment are 0/1 indicators, while those used for SDU poststratification adjustment are counting variables. The variables of the first type (0/1 indicators) are population density, group quarters, race/ethnicity of householder, percentage of persons in segment who are black or African American, percentage of persons in segment who are Hispanic or Latino, percentage of owner-occupied dwelling units (DUs) in segment, segment-combined median rent and housing value, and household type. Variables of the second type (counting variables) represent the number of eligible persons within each DU who fall into the various demographic categories of race, age group, Hispanicity, and gender. Note that the State and quarter variables are represented as both binary and counting variables. Thus, not only are DU counts within a specific State or quarter in the QDU sample controlled to the corresponding totals obtained from the SDU sample, but also counts of persons living in the DUs in the QDU sample are controlled to totals from the SDU sample. These person-level totals match the census estimates because of the SDU-level poststratification to census counts. It may be noted that in the poststratification of selected QDUs and the nonresponse adjustment of the respondent QDUs steps, demographic information from screener data was used in defining covariates, whereas in the poststratification of the selected QDUs step, questionnaire demographic information was used. Exhibit 4.1 lists all predictor variables proposed for QDU-level calibration and identifies them as counting, binary, or both. Various main effects and higher level factor effects based on 15 the predictor variables were included in the GEM modeling. As stated previously, all adjustment steps at the QDU level used a common set of proposed predictor variables. 4.2 Pair Weight Calibration Like QDU, the initial set of weight components in pair weight calibration are the same as the set obtained from the SDU-level weight calibration. The SDU-calibrated weight is multiplied by the pair-level design weight, which in turn was adjusted in four steps: poststratification of selected pairs, nonresponse adjustment of respondent pairs, poststratification of respondent pairs, and the extreme weight adjustment of respondent pairs. All the adjustment steps for pair weights utilized the same set of initially proposed predictor variables, which included a subset of those used for the person-level nonresponse adjustment. This included segment characteristic variables, such as population density, percentage of persons in segment who are black or African American, percentage of persons in segment who are Hispanic or Latino, percentage of owner- occupied DUs in segment, and segment-combined median rent and housing value. Also included were pair-specific covariates, such as the demographic characteristics of pair age, pair race/ethnicity, and pair gender, as well as dwelling unit characteristics, such as race/ethnicity of householder, household type, household size, and group quarters indicators. State and quarter indicators were included as well. However, for two-factor effects, instead of individual State, State/region was used due to insufficient sample size. This resulted in a 12-level variable where the eight large sample States were kept separate, and the remainder of States were grouped according to the four census regions. All variables were defined as 0/1 indicators. These proposed predictor variables and their levels are shown in Exhibit 4.2. In the poststratification of selected pairs and the nonresponse adjustment of respondent pairs, screener data were used in the definition of the pair-specific variables such as pair age, pair race/ethnicity, and pair gender, whereas in the poststratification and extreme weight adjustment of respondent pairs, these variables were obtained from the questionnaire. For the latter case, in addition to the variables described above, indicator covariates corresponding to selected pair domains were included to perform Hajek-type ratio adjustments via weight calibration, as mentioned in Chapter 1. The selected pair domains were limited to 10 of the 14 pair domains listed in Chapter 1. (Parent-child pairs where the child was in the 15- to 17-year-old age range and sibling-sibling-younger sibling focus pairs were not included in the poststratification.) The inclusion of these pair domain covariates led to the use of two sets of control totals in the modeling. Details of the construction of these control totals can be found in Appendix B. 16 Exhibit 4.1 Definitions of Levels for QDU-Level Calibration Modeling Variables Ageb 1: 12-17, 2: 18-25, 3: 26-34, 4: 35-49, 5: 50+1 Genderb 1: Male, 2: Female1 Group Quarter Indicatora 1: College Dorm, 2: Other Group Quarter, 3: Non-Group Quarter1 Hispanicityb 1: Hispanic or Latino, 2: Non-Hispanic or Latino1 Household Sizeb Continuous Variable Count of Individuals Rostered with DU Household Type (Ages of Persons Rostered within DU)a 1: 12-17, 18-25, 26+; 2: 12-17, 18-25; 3: 12-17, 26+; 4: 18-25, 26+; 5: 12-17, 6: 18-25; 7: 26+1 Percentage of Owner-Occupied Dwelling Units in Segment (% Owner-Occupied)a 1: 50-100%,1 2: 10->50%, 3: 0->10% Percentage of Segments That Are Black or African Americana 1: 50-100%, 2: 10->50%, 3: 0->10%1 Percentage of Segments That Are Hispanic or Latinoa 1: 50-100%, 2: 10->50%, 3: 0->10%1 Population Densitya 1: MSA 1,000,000 or More, 2: MSA Less than 1,000,000, 3: Non-MSA Urban, 4: Non-MSA Rural1 Quartera,b 1: Quarter 1, 2: Quarter 2, 3: Quarter 3, 4: Quarter 41 Race (3 Levels)b 1: White,1 2: Black or African American, 3: Other Race (5 Levels)b 1: White,1 2: Black or African American, 3: American Indian or Alaska Native, 4: Asian, 5: Two or More Races Race/Ethnicity of Householdera 1: Hispanic or Latino White,1 2: Hispanic or Latino Black or African American, 3: Hispanic or Latino Other, 4: Non-Hispanic or Latino White, 5: Non-Hispanic or Latino Black or African American, 6: Non-Hispanic or Latino Other Relation to Householdera 1: Householder or Spouse, 2: Child, 3: Other Relative, 4: Nonrelative1 Segment-Combined Median Rent and Housing Value (Rent/Housing)a,2 1: First Quintile, 2: Second Quintile, 3: Third Quintile, 4: Fourth Quintile, 5: Fifth Quintile1 Statesa,b,3 Model Group 1: 1: Connecticut, 2: Maine, 3: Massachusetts,1 4: New Hampshire, 5: New Jersey, 6: New York, 7: Pennsylvania, 8: Rhode Island, 9: Vermont Model Group 2: 1: Illinois, 2: Indiana, 3: Iowa, 4: Kansas, 5: Michigan, 6: Minnesota, 7: Missouri, 8: Nebraska, 9: North Dakota, 10: Ohio, 11: South Dakota, 12: Wisconsin1 Model Group 3: 1: Alabama, 2: Arkansas, 3: Delaware, 4: District of Columbia, 5: Florida, 6: Georgia, 7: Kentucky, 8: Louisiana, 9: Maryland, 10: Mississippi, 11: North Carolina,1 12: Oklahoma, 13: South Carolina, 14: Tennessee, 15: Texas, 16: Virginia, 17: West Virginia Model Group 4: 1: Alaska, 2: Arizona,1 3: California, 4: Colorado, 5: Idaho, 6: Hawaii, 7: Montana, 8: Nevada, 9: New Mexico, 10: Oregon, 11: Utah, 12: Washington, 13: Wyoming State/Regiona,3 Model Group 1: 1: New York, 2: Pennsylvania, 3: Other1 Model Group 2: 1: Illinois, 2: Michigan, 3: Ohio, 4: Other1 Model Group 3: 1: Florida, 2: Texas, 3: Other1 Model Group 4: 1: California, 2: Other1 DU = dwelling unit; MSA = metropolitan statistical area; QDU = questionnaire dwelling unit. 1 The reference level for this variable. This is the level against which effects of other factor levels are measured. 2 Segment-Combined Median Rent and Housing Value is a composite measure based on rent, housing value, and percentage owner-occupied. 3 The States or district assigned to a particular model is based on census regions. a Binary variable. b Counting variable. 17 Exhibit 4.2 Definitions of Levels for Pair-Level Calibration Modeling Variables Group Quarter Indicator 1: College Dorm, 2: Other Group Quarter, 3: Non-Group Quarter1 Household Size 1: DU with 2 Persons,1 2: DU with 3 Persons, 3: DU with > 4 Persons Pair Age (15 Levels) 1: 12-17 and 12-17,1 2: 12-17 and 18-25, 3: 12-17 and 26-34, 4: 12-17 and 35-49, 5: 12-17 and 50+, 6: 18-25 and 18-25, 7: 18-25 and 26-34, 8: 18-25 and 35-49, 9: 18-25 and 50+, 10: 26-34 and 26-34, 11: 26-34 and 35-49, 12: 26-34 and 50+, 13: 35-49 and 35-49, 14: 35-49 and 50+, 15: 50+ and 50+ Pair Age (6 Levels) 1: 12-17 and 12-17,1 2: 12-17 and 18-25, 3: 12-17 and 26+, 4: 18-25 and 18-25, 5: 18-25 and 26+, 6: 26+ and 26+ Pair Age (3 Levels) 1: 12-17 and 12-17,1 2: 12-17 and 18+, 3: 18+ and 18+ Pair Gender 1: Male and Female,1 2: Female and Female, 3: Male and Male Pair Race/Ethnicity (10 Levels) 1: White and White,1 2: White and Black or African American, 3: White and Hispanic or Latino, 4: White and Other, 5: Black or African American and Black or African American, 6: Black or African American and Hispanic or Latino, 7: Black or African American and Other, 8: Hispanic or Latino and Hispanic or Latino, 9: Hispanic or Latino and Other, 10: Other and Other Pair Race/Ethnicity (5 Levels) 1: Two or More Races Pair, 2: Hispanic or Latino Pair, 3: Black or African-American Pair, 4: White Pair,1 5: Other Pair Pair Race/Ethnicity (4 Levels) 1: Two or More Races Pair or Other and Other, 2: Hispanic or Latino Pair, 3: Black or African-American Pair, 4: White Pair1 Percentage of Owner-Occupied Dwelling Units in Segment (% Owner-Occupied) 1: 50-100%,1 2: 10->50%, 3: 0->10% Percentage of Segments That Are Black or African American 1: 50-100%, 2: 10->50%, 3: 0->10%1 Percentage of Segments That Are Hispanic or Latino 1: 50-100%, 2: 10->50%, 3: 0->10%1 Segment-Combined Median Rent and Housing Value (Rent/Housing)2 1: First Quintile, 2: Second Quintile, 3: Third Quintile, 4: Fourth Quintile, 5: Fifth Quintile1 Population Density 1: MSA 1,000,000 or More, 2: MSA Less than 1,000,000, 3: Non-MSA Urban, 4: Non-MSA Rural1 Quarter 1: Quarter 1, 2: Quarter 2, 3: Quarter 3, 4: Quarter 41 Race/Ethnicity of Householder 1: Hispanic or Latino White,1 2: Hispanic or Latino Black or African American, 3: Hispanic or Latino Other, 4: Non-Hispanic or Latino White, 5: Non-Hispanic or Latino Black or African American, 6: Non-Hispanic or Latino Other 18 Exhibit 4.2 Definitions of Levels for Pair-Level Calibration Modeling Variables (continued) State/Region Model Group 1:1: Connecticut, Maine, Massachusetts, New Hampshire, New Jersey, Rhode Island, Vermont; 2: Alabama, Arkansas, Delaware, District of Columbia, Georgia, Kentucky, Louisiana, Maryland, Mississippi, North Carolina, Oklahoma, South Carolina, Tennessee, Virginia, West Virginia;1 3: New York; 4: Pennsylvania; 5: Florida; 6: Texas Model Group 2: 1: Indiana, Iowa, Kansas, Minnesota, Missouri, Nebraska, North Dakota, South Dakota, Wisconsin;1 2: Alaska, Arizona, Colorado, Idaho, Hawaii, Montana, Nevada, New Mexico, Oregon, Utah, Washington, Wyoming; 3: Michigan; 4: Illinois; 5: Ohio; 6: California States3 Model Group 1: 1: Alabama, 2: Arkansas, 3: Connecticut, 4: Delaware, 5: District of Columbia, 6: Florida, 7: Georgia, 8: Kentucky, 9: Louisiana, 10: Maine, 11: Maryland,1 12: Massachusetts, 13: Mississippi, 14: New Hampshire, 15: New Jersey, 16: New York, 17: North Carolina, 18: Oklahoma, 19: Pennsylvania, 20: Rhode Island, 21: South Carolina, 22: Tennessee, 23: Texas, 24: Vermont, 25: Virginia, 26: West Virginia Model Group 2: 1: Alaska, 2: Arizona,1 3: California, 4: Colorado, 5: Idaho, 6: Illinois, 7: Indiana, 8: Iowa, 9: Hawaii, 10: Kansas, 11: Michigan, 12: Minnesota, 13: Missouri, 14: Montana, 15: Nebraska, 16: Nevada, 17: New Mexico, 18: North Dakota, 19: Ohio, 20: Oregon, 21: South Dakota, 22: Utah, 23: Washington, 24: Wisconsin, 25: Wyoming Pair Relationship Associated with Multiplicity 1: Parent-Child (12-14)* 2: Parent-Child (12-17)* 3: Parent-Child (12-10)* 4: Parent*-Child (12-14) 5: Parent*-Child (12-17) 6: Parent*-Child (12-20) 7: Sibling (12-14)-Sibling (15-17) 8: Sibling (12-17)-Sibling (18-25) 9: Spouse-Spouse/Partner-Partner 10: Spouse-Spouse/Partner-Partner with Children (younger than 18) DU = dwelling unit; MSA = metropolitan statistical area; QDU = questionnaire dwelling unit. 1 The reference level for this variable. This is the level against which effects of other factor levels are measured. 2 Segment-Combined Median Rent and Housing Value is a composite measure based on rent, housing value, and percentage owner-occupied. 3 The States or district assigned to a particular model is based on combined census regions. * The pair member focused on. 19 20 5. Definition of Extreme Weights An important feature of the generalized exponential model (GEM) is the built-in provision of extreme value (ev) treatment. Sampling weights are often classified as extreme (high or low) if they fall outside the interval, median ± 3 × interquartile range (IQR). The interval is set for prespecified domains defined usually by design variables corresponding to deep stratification.7 Similar to previous NSDUHs, for the GEM modeling used in the 2011 NSDUH, a more conservative (narrower) interval was defined, median ± 2.5 × IQR. The narrower interval better prevents the adjusted weights from crossing the standard interval boundaries by treating weights near but not outside the commonly used boundaries (i.e., those that have the most potential to become extreme) as extreme as well. Denote the interval boundaries (or critical values) for low and high extreme values by bk(l) and bk(u) , respectively. For implementing extreme value control via GEM, the variable mk was defined as the minimum of bk(u) / wk and one for high extreme weights, and the maximum of bk(l) / wk and one for low extreme weights, where wk represents the sampling weight before adjustment, and bk(u) and bk(l) denote the critical values for the extreme weights. Note that under this definition, for high extreme weights, the more extreme the weight is, the smaller mk will be, and, conversely, for low extreme weights, the more extreme the weight is, the bigger mk will be. Nonextreme weights had a value of one for mk. The upper and lower bounds for the adjustment factors were defined, respectively, as the product of mk and the upper and lower boundary parameters of GEM. GEM allows inputs of up to three different upper and lower boundary parameters (L1 and U1, L2 and U2, L3 and U3) for high, non-, and low extreme weights. By applying a small upper boundary parameter for high extreme weights and a large lower boundary parameter for low extreme weights, the extreme weights can be controlled in the modeling process. 5.1 Questionnaire Dwelling Unit Extreme Weight Definition For the questionnaire dwelling unit (QDU)-level weight adjustment, extreme weights were defined using a nested hierarchy of six domains: 1. State; 2. State sampling region; 3. State by household type; Levels of household type indicate whether the household has members who are youths, young adults, or adults, where youth signifies 12- to 17-year-olds, young adult 18- to 25- year-olds, and adult 26 years or older. 7 Deep stratification refers to the stratification that was used in the sample design. In the case of the 2011 survey, deep stratification refers to the cross-classification of State sampling region by age group. 21 a. Youth, Young Adult, Adult; b. Youth, Young Adult; c. Youth, Adult; d. Young Adult, Adult; e. Youth Only; f. Young Adult Only; and g. Adult Only. 4. State sampling region by household type; 5. State by household type by household size (1, 2, 3, 4+); and 6. State sampling region by household type by household size. The hierarchy is used to satisfy the minimum of 30 observations for defining the boundaries for extreme values. If this sample size requirement is not met at the lower level, then the next level up in the hierarchy is used. 5.2 Person Pair Extreme Weight Definition The pair selection probability is a function of the selection probability of each person in the pair given by formula (2.1) or (2.6), depending on the sum of the person selection probabilities within the household as discussed in Section 2.1. This probability can be very small if the selection probabilities of individual members are small. For example, consider a particular selected dwelling unit (DU) from the 2011 survey. This DU gave rise to a selected pair of respondents, one aged between 35 and 49 and the other aged 50 or older. The selection probability in this DU was 0.14811 for a respondent aged between 35 and 49, and it was 0.08509 for a respondent aged 50 or older. Using the formula (2.6) in Chapter 2, the pair selection probability was computed to be 0.000487359. Therefore, the inverse of the selection probability, the pair-level design weight, was 2051.88. Thus, a small pair selection probability can create a high initial weight, which is the product of the screener dwelling unit (SDU) weight and the person pair design-based weight. As mentioned in the introduction, it turns out to be difficult to select suitable domains for defining extreme weights for pair-level data. However, as was done for the 1999–2011 surveys, the extreme weight definition was based on the following hierarchy of domains: 1. Pair age group8 (with three age categories, 12 to 25, 26 to 49, and 50+) by number (0, 1, 2+) of persons aged 12 to 25 in the household; 2. State cluster (with five levels [explained below]) by pair age group by number (0, 1, 2+) of persons aged 12 to 25 in the household; 3. State cluster (with three levels [explained below]) by pair age group by number (0, 1, 2+) of persons aged 12 to 25 in the household; and 4. State by pair age group by number of persons aged 12 to 25 (0, 1, 2+) in the household. 8 Pair age in this case should not be confused with the modeling term, which has a finer level breakdown. 22 The hierarchy was used to satisfy the minimum of 30 observations for defining the boundaries for extreme values. If this sample size requirement was not met at the lower level, then the next level up in the hierarchy was used. We now briefly introduce the considerations behind the above definition for extreme weight domains. The sample design prespecified the person-level selection probability within State by five age groups (12 to 17, 18 to 25, 26 to 34, 35 to 49, 50+). Age groups 12 to 17 and 18 to 25 have a relatively similar selection probability, and the same is true for age groups 26 to 34 and 35 to 49. The 50+ group, however, has a quite different selection probability from the other groups. Furthermore, since the 12 to 17 and 18 to 25 age groups have large selection probabilities, they have a very high chance of being selected if the household has persons in these age groups. Therefore, the number of persons aged 12 to 25 in the household has a significant impact on the type of pair selected and the pair selection probability. Taking into consideration these design-related features, a suitable domain to define the pair-level extreme weight seems to be given by State by pair age group by number of persons aged 12 to 25 in the household. The hierarchy of domains mentioned above was used to satisfy the minimum of 30 observations. However, it was found that for many ev domains the minimum sample size requirement was not met. To alleviate this problem, States were grouped into a small number of clusters, such as three or five. The assignment of States to clusters was determined by the clustering algorithm in PROC CLUSTER in SAS, where the clustering variable was defined as the average person-level weight (ANALWT) for each of the five age groups within each State. The choice of the average person-level weight for each group for each State was motivated from the objective of finding a single variable that would reflect the design-based difference in pair selection probabilities across States. Even with clustering of States, the ev domain sample size was insufficient in some cases, so the most general level of the hierarchy, the national level, was required. Furthermore, at the national level, we had to collapse some pair age categories in forming domains of reasonable sample size to define extreme weights. More specifically, for the national level, we collapsed all levels of number of persons aged 12 to 25 for the pair age groups of 50+, 50+ and 26 to 49, 50+. In addition, levels 1 and 2+ of number of persons aged 12 to 25 were combined for the pair age group of 26 to 49, 26 to 49. 23 24 6. Weight Calibration at Questionnaire Dwelling Unit and Pair Levels The 2011 National Survey on Drug Use and Health (NSDUH) was based on probability sampling so that valid inferences can be made from survey findings about the target population. Probability sampling refers to sampling in which every unit on the frame is given a known, nonzero probability for inclusion in the survey. This is required for unbiased estimation of the population total. The assumption of nonzero inclusion probability for every pair of units in the frame also is required for unbiased variance estimation. The basic sampling plan involved four stages of selection across two phases of design: within Phase I, (1) the selection of census tracts within each State sampling (SS) region, (2) the selection of subareas or segments (comprised of U.S. Census Bureau blocks) within SS regions; (3) the selection of dwelling units (DUs) within these subareas; and, finally, within Phase II, (4) the selection of eligible individuals within DUs. Specific details of the sample design and selection procedures for the sample can be found in the 2011 NSDUH sample design report (Morton, Martin, Shook-Sa, Chromy, & Hirsch, 2012). As part of the postsurvey dat