11_Pearson correlation and ttest.pdf
Document Details
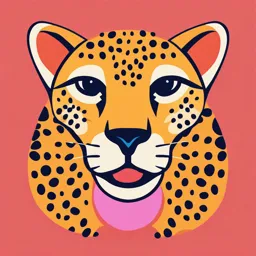
Uploaded by LuckiestForethought
University of Oxford
2019
Tags
Full Transcript
BEHL 2005 / BEHL 2019 (UO) Introductory Research Methods PEARSON CORRELATION AND T-TESTS Professor Hannah Keage What are we going to cover? New concepts: homoscedasticity and independence Pearson correlation T-tests Content from this lecture references: Homoscedasticity Homoscedasticity Homo...
BEHL 2005 / BEHL 2019 (UO) Introductory Research Methods PEARSON CORRELATION AND T-TESTS Professor Hannah Keage What are we going to cover? New concepts: homoscedasticity and independence Pearson correlation T-tests Content from this lecture references: Homoscedasticity Homoscedasticity Homoscedastic Even distribution/variance across correlation line Homogenous or equal variance Heteroscedastic Uneven distribution/variance across correlation line Heterogenous or unequal variance Homoscedasticity Homoscedastic Heteroscedastic Homoscedasticity • Refers to homogeneity of variance. • A common assumption for parametric statistical models. • Most commonly tested using Levene’s test (F): • p<.05 violated homogeneity of variance. • p≥.05 all is fine, you have not violated homogeneity of variance. • When you violate the assumption of homogeneity of variance, use Welch’s (t-test) Test. Independence (in context of parametric tests) • Observations are independent, i.e. no two observations in a dataset are related to each other or affect each other in any way. • A common assumption for parametric statistical models. • You need to check this using logic. There’s no test for it. Independence (in context of parametric tests) A gym manager wanted to compare the fitness levels of people who attend the gym on Mondays and Wednesdays. She collected fitness levels from 20 people on Monday and 20 people on Wednesday. Three participants were represented in both days. A gym manager wanted to investigate if a new weight class approach improves strength. One instructor teaches two weight classes using the old approach; one instructor teaches one weight class using the old approach and two using the new approach. A gym manager wanted to determine how many gym members would use an outdoor pool, if they added one on. She asks each member if they would, wouldn’t, or can’t decide. Independence (in context of parametric tests) A gym manager wanted to compare the fitness levels of people who attend the gym on Mondays and Wednesdays. She collected fitness levels from 20 people on Monday and 20 people on Wednesday. Three participants were represented in both days. The two groups are not independent, they share participants. A gym manager wanted to investigate if a new weight class approach improves strength. One instructor teaches two weight classes using the old approach; one instructor teaches one weight class using the old approach and two using the new approach. The two groups are not independent, the instructor is shared. A gym manager wanted to determine how many gym members would use an outdoor pool, if they added one on. She asks each member if they would, wouldn’t, or can’t decide. Great! Pearson correlation • Pearson correlation coefficient is referred to a as r. • Assesses linear (straight line) relationships between two variables. • Assumptions that apply to Pearson correlation: • • • • • • Linear relationship (straight line). Parametric data/normality. Homogeneity of variance (homoscedasticity). Independence. At least one variable needs to be continuous. The other variable can be continuous or dichotomous. Pearson correlation r = 0 no relationship r = 1 perfect positive relationship r = -1 perfect negative relationship Pearson correlation All r = 0.82. Need to conduct a scatterplot before running a correlation to ensure linear relationship. Linear Non-linear Non-linear Non-linear Pearson correlation Correlations are also measures of effect size! There are a few ways to (arbitrarily) employ cut-offs. Here is one. Pearson correlation Reporting examples: r(38) = .34, p=.009 There was a statistically significant and weak positive relationship between hours of TV watched and fatigue r(196) = -.75, p<.001 There was a statistically significant and strong negative relationship between hours of TV watched and sleep duration r(degrees of freedom) = esimate, p=x T-tests • When you want to compare two means. • If “group 1” is larger than “group 2” the t statistic will be positive; if “group 2” is larger then the t statistic will be negative. • Assumptions that apply to t-tests: • • • • • Parametric data/normality. Independence. Homogeneity of variance (homoscedasticity). DV needs to be continuous. IV needs to be dichotomous (groups or time points). T-tests Between groups (independent) t-test: if those two means are from different people Group 1 versus Group 2 Within groups (dependent or paired) t-tests: if those two means are from the same people Time 1 versus Time 2 T-tests • T-tests are not measures of effect size. • We traditionally use Cohen’s d to measure effect size for t-tests. T-tests Reporting examples: t(24) = 5.23, p=.002, d=1.09 Those in the heavy TV watching group were statistically significantly more fatigued than the low TV watching group, with a large effect size. t(187) = -2.31, p=.017, d=0.04 Those in the heavy TV watching group had statistically significantly less sleep than those in the low TV watching group, with a small effect size. t(degrees of freedom) = esimate, p=x, d=x BEHL 2005 / BEHL 2019 (UO) Introductory Research Methods PEARSON CORRELATION AND T-TESTS Professor Hannah Keage