Introductory Research Methods PDF
Document Details
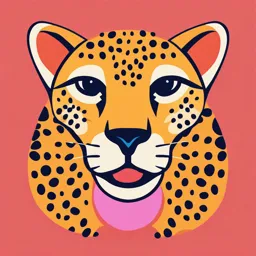
Uploaded by LuckiestForethought
Hannah Keage
Tags
Summary
This document covers important aspects of statistical models and why they are used in research. It discusses null hypothesis testing, p-values, statistical significance, effect size, and power. The lecture is part of an Introductory Research Methods course (BEHL 2005 / BEHL 2019).
Full Transcript
BEHL 2005 / BEHL 2019 (UO) Introductory Research Methods Important aspects of statistical models and why we use them Professor Hannah Keage What are we going to cover? Null hypothesis testing What is the p value? What is statistical significance? Effect size Power Content from this lecture refer...
BEHL 2005 / BEHL 2019 (UO) Introductory Research Methods Important aspects of statistical models and why we use them Professor Hannah Keage What are we going to cover? Null hypothesis testing What is the p value? What is statistical significance? Effect size Power Content from this lecture references: Null hypothesis testing • Step 1: Formulate a hypothesis that embodies our prediction (before seeing the data). • Step 2: Specify null and alternative/experimental hypotheses. • Step 3: Collect some data relevant to the hypothesis. • Step 4: Fit a model to the data that represents the alternative hypothesis and compute the test statistics. • Step 5: Compute the probability of the observed value of that statistic assuming that the null hypothesis is true. • Step 6: Assess the “statistical significance” (p value) of the result. Null hypothesis testing • Step 1: Formulate a hypothesis that embodies our prediction (before seeing the data). Hypothesis: those who sleep 7 hours or more per night will show better memory performance than those who sleep less than 7 hours. Null hypothesis testing • Step 2: Specify null and alternative (experimental) hypotheses. Alternative hypothesis: those who sleep 7 hours or more per night will show better memory performance than those who sleep less than 7 hours. HA = memory performance in those who sleep ≥7h > memory performance in those who sleep <7h. Null hypothesis: no difference in memory performance as a function of sleep duration. H0 = memory performance in those who sleep ≥7h = memory performance in those who sleep <7h. Null hypothesis testing • Step 3: Collect some data relevant to the hypothesis. Null hypothesis testing • Step 4: Fit a model to the data that represents the alternative hypothesis and compute the test statistics. Null hypothesis testing • Step 5: Compute the probability of the observed value of that statistic assuming that the null hypothesis is true. 1 – the p-value i.e. 1 – 0.007 =0.993 Null hypothesis testing • Step 6: Assess the “statistical significance” (p value) of the result. p=0.007 The p value Under the assumption that the null hypothesis is true, the p value is the probability of getting a sample as or more extreme as our own. • • • • Is a probability. It is not the probability of the null hypothesis being true. It is not the probability that you are making the wrong decision. It is not the probably that if you ran the study again, you would obtain the same result that % of the time. • It does not mean you found an important effect. • It does not reflect the size of the effect. Statistical significance • • • • Dichotomous assessment. Arbitrary with historical reference point. Dependent on your alpha value and your p value. Please never use terms such as nearly significant, trend for significance, close to significant. • Please always report the actual p value of your model, not just p<.05 or p<.01. • Please never report p=.000, as it’s a probability, this means p<.001. The alpha value • This is predefined before you run your analyses. • Determines what you define as a “significant” or “not significant” effect by comparing with p value from statistical model. • Typically alpha is set at 0.05, meaning that p<.05 is deemed statistically significant. But this is entirely arbitrary! • In setting your alpha value you are balancing Type I and Type II errors. Type I v Type II errors Type I error False positive We conclude that those who sleep 7 hours or more per night show better memory performance. In fact, those who sleep 7 hours or more per night do not show better memory performance. Type II error False negative We conclude that those who sleep 7 hours or more per night do not show better memory performance. In fact, those who sleep 7 hours or more per night do show better memory performance. Effect size • As (more?) important as the p value, but often over looked. • Measure of the strength of the effect. • For example, sleep can have a large effect on memory performance or a small effect. • This is really important if we are looking at interventions or findings that may inform policy. • There are different ways to measure effect size, e.g. r2, Cohen’s d. Statistical power • Power = 1 − the probability of making a Type II error • Three factors affect power: • Sample size: larger samples provide greater statistical power. • Effect size: a given design will always have greater power to find a large effect than a small effect (because finding large effects is easier). • Type I error rate: there is a relationship between Type I error and power such that (all else being equal) decreasing Type I error will also decrease power. When running a statistical model, we need to consider the key ingredients… • Our approach of null hypothesis testing. • Setting our alpha value before running statistical models. • The effect size and p value of models. • Keeping in mind Type I and II errors, and our power to detect effects. BEHL 2005 / BEHL 2019 (UO) Introductory Research Methods Important aspects of statistical models and why we use them Professor Hannah Keage