Quality Core Tools II PDF
Document Details
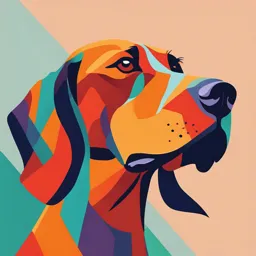
Uploaded by EventfulGorgon
Conestoga College
Prof. Rafat Tahboosh
Tags
Related
- Management Information Systems Managing The Digital Firm PDF
- Robbins - Circuit Analysis - Theory and Practice PDF 2003
- Complete Blood Count PDF
- Présentation Contrôle de Gestion Sociale PDF
- Chapter 6 Determination Of National Income PDF
- Week 5 Lectures on Macroeconomic Accounts and Policies (v.1) PDF
Summary
This Conestoga document is an introduction to quality core measurement systems. It describes the importance and application of MSA. Includes lesson outlines, objectives, and exercises.
Full Transcript
Quality Core Tools II Week – 2: Introduction to Measurement Systems B. Introduction to Measurement Systems – Main Material B. Introduction to Measurement Systems – In Class Lesson Outline 1. Feedback and Pre-assessment (Discussion of...
Quality Core Tools II Week – 2: Introduction to Measurement Systems B. Introduction to Measurement Systems – Main Material B. Introduction to Measurement Systems – In Class Lesson Outline 1. Feedback and Pre-assessment (Discussion of S.W.I.P.E Examples and MSA Review) 2. Introduction to Measurement System Errors ✓ 2.0. Basic Statistics – Additional Section ✓ 2.1 Resolution ✓ 2.2 Accuracy – Location Variation ✓ 2.3 Precision - Width Variation 3. Summary and Post Assessment 2 B. Introduction to Measurement Systems – In Class 2 Lesson Objectives 1. Explain the purpose of MSA using industry examples. 2. Describe the source of variations for the errors in the measurement system 3 1. Feedback and Pre-assessment ❑ Feedback of online discussion / assessment post (1) – 10 Min. S.W.I.P.E. Examples ❑ Pre-assessment (Not-graded): ▪ What is the definition of MSA? Purpose? 4 1. Feedback and Pre-assessment – Measurement System Review Standard Product specifications, Acceptance Criteria, Master Sample, Boundary Samples Work Piece (part) Part or product to be measured Instrument (or gauge) Tool or gauge used to measure the part or product Person (Appraiser) and Operator or Technology and Test Methods Procedures Environment Atmospheric or Controlled 5 2. Measurement System Errors Sources of Variation Total (obs) unit-to-unit Measurement piece-to-piece System Error True (actual) System variation variation Precision Accuracy Width/spread location Bias Repeatability Reproducibility (diff from actual) equipment operator equipment Uniformity Consistency Linearity Stability over range over time over range drift over time 6 Source: https://www.quality-assurance-solutions.com/Continuous-data.html 2.0. Basic Statistics - Basic Statistics - Minitab 7 2.0. Basic Statistics - Basic Statistics - Excel 8 2.0. Basic Statistics – Basic Statistics – Exercise (Perform basic 220 178 statistics using Minitab and Excel) 210 199 204 201 205 203 210 198 200 311 200 211 199 205 245 199 234 199 320 199 210 199 204 200 205 200 210 199 200 245 200 234 199 198 245 230 9 2.0. Basic Statistics Test number Crush Strength 1 40 In class Exercise 2 37.9 3 29 – Calculate: 4 31.7 5 39.3 Range 6 40 7 50.3 Average 8 33.8 Variance 9 10 39.3 42.1 Standard Deviation 11 12 45.5 41.4 – Histogram and Frequency Analysis 13 14 47.6 38.6 – Normality Test 15 35.9 16 41.4 17 44.1 P-value analysis 18 41.4 19 44.1 – Interpreting the Confidence Interval of the mean 20 40 21 40.7 22 42.1 23 38.6 24 36.5 25 40.7 10 2.0 Basic Statistics Histogram and Basic Statistics Stat>Basic Stat>Basic Statistics>Graphical Statistics>Display Summary Descriptive Statistics 11 2.0. Basic Statistics Normality Test Normality Test Stat>Basic Statistics>Normality test… Graphs>Probability plot… If p-value If p-value ≥0.05, then ≥0.05, then the data the data follows a follows a normal normal distribution distribution 12 2.0 Basic Statistics What is P-Value? In statistical hypothesis testing, the P-value (the green shaded area, value between 0 – 1) is the probability of an observed data point (or more extreme) result assuming that our null hypothesis is correct. If x is the observed value, then P-value is given by: https://upload.wikimedia.org/wikipedia/en/0/00/P-value_Graph.png P (X≥ x/H) – Right tail event P (X ≤ x /H) – Left tail event 2 * min. < P (X≥ x/H), P (X ≤ x/H)> - Double tail event. The null Hypothesis (Ho) is rejected if these probabilities (P-value) is less than or equal to the significance level α (a small, fixed but arbitrarily pre-defined threshold value set by the researcher before examining the data). Memory Aid: If P-value is LOW (Lower than α 0.05)…. Null Go / Reject the Null Hypothesis 13 2.0 Basic Statistics Statistical Inference 𝑥 1, 𝑥 2, 𝑥 3, … , 𝑥 n Draw a Population sample µ = population Sample is taken from the population mean We study properties of the sample and then σ= population we can make an inference of the population standard deviation properties (data distribution and parameters). Inference Example: Estimated mean: µ ෝ=𝒙 ഥ ෝ = σx Estimated Standard Deviation: 𝝈 We will never know the exact distribution of the population because we can only test a sample We can only make an inference based on the sample drawn from the population 14 2.0 Basic Statistics Interpreting a confidence interval of a mean A 95% confidence interval is a range of values that you can be 95% certain contains the true mean of the population. This is not the same as a range that contains 95% of the values. The graph below emphasizes this distinction. 15 2.0. Basic Statistics Interpreting a confidence interval of a mean The graph shows three samples (of different size) all sampled from the same population. With the small sample on the left, the 95% confidence interval is similar to the range of the data. But only a tiny fraction of the values in the large sample on the right lie within the confidence interval. The 95% confidence interval defines a range of values that you can be 95% certain contains the population mean. With large samples, you know that mean with much more precision than you do with a small sample, so the confidence interval is quite narrow when computed from a large 16 sample. 2. Measurement System Error – Experiment 15 Min. Sources of variation experiment 17 2. Measurement System Error - Experiment Source of Variation Exercise (this is an example of “Person/Procedure” variation) Purpose: To study the effect of training/measurement procedure (measurement procedure) on measurement variation Tools: One caliper (Measuring tape through mobile app could be used) and one block/part to be measurand (Thickness, Length, width,..etc.) Procedure: Half of the class measures the block thickness without measurement training The other half of the class measures the same block thickness with the caliper / measuring tape Mobile app with measurement training (professor trains this half class while the first half is taking measurements) Students record measurements in excel and send the file to the professor Analysis (by Faculty): Test of equal variances - are the variances different? (look at p-value) Capability study, Normal – review the histogram with specification limits of both groups, are they 18 different? 2. Measurement System Error - In Class Experiment Group 1-T Group 2 10.1 10.3 9.9 9.9 10 9.8 10.2 10.2 10.1 10.3 10 10.4 10.1 9.9 10.1 10.2 9.9 10 Ref 10mm LSL 9.8mm USL 10.2 mm 19 Measurement System Error – Test of Equal Variances (Steps) 20 Measurement System Error – Test of Equal Variances (Steps) 21 Measurement System Error – Test of Equal Variances (Steps) Note: We need to select for this test that the data based on normal distribution as this is the nature of this process of measurements – we assume the data is based on normal distribution to do this test (F Test) 22 Measurement System Error –Test of Equal Variances (Minitab Results) Conclusion: the P-value (0.048) is less than α = 0.05, so we reject the null hypotheses. So, the variances of the measurements of the two groups are statistically significant (Not equal) (P-value LOW = Null Go (Reject) 23 Measurement System Error –Process Capability Steps 24 Measurement System Error – Minitab Results Process Capability Conclusion: The process is not capable as Pp and Cp (Process capability indices) > 1.33 or 1 (The process variation is beyond the USL/LSL 25 2. Measurement System Error – MSA Steps Resolution Accuracy Precision Gauge reading Location Variation Width variation Smallest unit of (position of mean or (measurement measure required measurement error) error variation) Bias GRR Stability Repeatability Linearity Reproducibility 26 2.1 Instrument Resolution Smallest unit of measure: Resolution (Discrimination or Readability) Required Resolution = Smallest unit of measure that the system can Tolerance /10 recognize. If an instrument has “coarse” graduation, then half-graduation can be used If smallest unit of measure < Use the 10 to 1 rule: Divide tolerance by 10 or Required Resolution => more to obtain the resolution required Instrument is good to use the measurement equipment is able to discriminate at least one-tenth of the process variation The resolution is unacceptable for analysis if it cannot detect the variation of the process, and unacceptable for control if it cannot detect the 27 special cause variation 2.1 Instrument Resolution 2 Source: MSA Reference Manual, 4th edition 28 2.1 Instrument Resolution - Exercises Example: ring outside diameter specification is 5.50 mm +/- 0.1 mm. 1. What is the instrument resolution required? 2. What instrument would we recommend using? 10 Min. 29 2.1 Instrument Resolution - Exercises Part weight is 15.0 lbs +/- 0.5 lbs 1. What is the instrument resolution required? 2. What instrument would you recommend using? 30 2.2 Accuracy – Location Variation ❑ Accuracy – “Closeness” to the true value, or to an accepted reference ▪ Bias – Quantitative term (measures the system’s accuracy) – Position of the mean or measurement error Source: MSA Reference Manual, 4th edition – Difference between the observed average of measurements and the reference value – A systematic error component of the measurement system 31 2.2 Accuracy – Location Variation 2 ▪ Linearity – The change in bias over the operating range – A systematic error component of the measurement system – if it consistently changes in the same direction – Ex: Instrumental (Calibration), Observational (Parallax error), Environmental Source: MSA Reference Manual, 4th edition 32 2.2 Accuracy – Location Variation 3 ▪ Stability The change in bias over time A stable measurement process is in statistical control with respect to location Also known as “drift” 33 Source: MSA Reference Manual, 4th edition 2.3 Precision – Width Variation Precision – “Closeness” of repeated readings to each other – A random error component of the measurement system caused by unknown and unpredictable changes in the Source: MSA Reference Manual, 4th edition experiment. – EX: changes in measuring instruments or in the environmental conditions i.e. electronic noise in the circuit of an electrical instrument, Repeatability – Variation in measurements obtained with one measuring instrument when used several times by an appraiser while 34 measuring the identical characteristic on the same part 2.3 Precision – Width Variation 2 Reproducibility – Variation in the average of measurements made by different appraisers using the same gage when measuring a characteristic of a Source: MSA Reference Manual, 4th edition part Gage R&R – Gage repeatability and reproducibility: the combined estimate of measurement system repeatability and reproducibility Source: MSA Reference Manual, 4th edition 35 2.3 Precision – Width Variation 3 Consistency – The degree of change of repeatability over time – A consistent measurement process is in statistical control with respect to width (variability) Uniformity – The change in repeatability over the Source: MSA Reference Manual, 4th edition normal operating range 36 3. Summary and Post Assessment Location Variation Width Variation (Position of Mean or measurement instrument error) (Standard Deviation or Spread of the measurement system error) A measurement system is The measurement precise if the measurement Accuracy: system is accurate if Precision: system variation is small the bias is statistically “Closeness” to the true “Closeness” to the compared to the Total zero value (or reference value) repeated readings to each Variation (%GRR < 30%) other Bias: difference between the Repeatability: Variation in measurements observed average of using 1 gage, 1 operator while measuring the measurements and the same characteristic several times reference value EV = Equipment Variation Stability: the change in bias Reproducibility: Variation in the average of the measurements made by different over time appraisers using the same gage when measuring a characteristic on a part AV = Appraiser Variation Linearity: the change in bias GRR: The combined estimate of over the normal operating range measurement system Repeatability and of a gage Reproducibility 37 3. Summary and Post Assessment 2 Accuracy vs. Precision 38 Source: Introduction to Statistical Control, 7th Ed., by D. C. Montgomery – Dart Board 3. Summary and Post Assessment (Non-graded) ❑ What is the definition of the followings: ✓ Resolution? ✓ Accuracy (Bias, Linearity, Stability)? ✓ Precision (Repeatability, Reproducibility, Consistency, Uniformity)? ✓ GRR? 39 References ▪ AIAG. (2010). Measurement System Analysis (MSA) Reference Manual (4th Edition). 40 THANK YOU 41 Quality Core Tools II Week – 6: Attribute Measurement System Study B. Attribute GRR Study – Main Material Prepared By: Prof. Rafat Tahboosh B. Attribute GRR Study Lesson Outline 1. Feedback and Pre-assessment 2. Attribute GRR Study 3. Attribute GRR (Minitab) 4. Attribute Agreement Analysis Effectiveness 5. Agreement Analysis (Kappa) 6. Agreement Analysis (P-Value) 7. Attribute GRR Exercise (After Class) 2 8. Summary and Post Assessment B. Attribute GRR Study 2 Lesson Objective 1. Describe the importance and application of attribute GR&R (Gauge Repeatability and Reproducibility) and describe the agreement analysis (Kappa calculations). 3 1. Feedback and Pre-assessment ❑ Feedback and Pre-assessment of Attribute 10 Min. Measurement System 1. What is the definition of Attribute Data? 2. Provide three examples of attribute measurement systems? 3. Provide two examples of automated attribute inspection systems? ❑ Feedback of the Attribute GRR Minitab instruction? 4 2. Attribute GRR Study Attribute R&R: Attribute Agreement Analysis Use Attribute Agreement Analysis when you have attribute ratings from Appraisers to assess whether appraisers are consistent with: – themselves, (REPEATABILITY) – one another, and (REPRODUCIBILITY) PRECISION – known standards. (ACCURACY) For example, a quality engineer wants to assess the consistency and correctness of the appraisers' ratings who rate the print quality of cotton fabric. The appraisers for a clothing manufacturer can evaluate fabric samples several different ways, such as the following: – The appraisers can use binary ratings and rate the samples as pass or fail. – The appraisers can use ordinal ratings and rate the samples on a scale of 1 to 10 (or 1 to 5). – The appraisers can use nominal ratings and rate the samples as light blue, medium blue, or dark blue. Source: https://support.minitab.com/en-us/minitab/18/help-and-how-to/quality-and-process-improvement/measurement-system-analysis/how-to/attribute-agreement-analysis/attribute- 5 agreement-analysis/before-you-start/overview/ 2. Attribute GRR Study 2 ❑ Why to Perform Attribute GRR? Process Assessment – Assess the inspection or workmanship standards against customer’s needs – To determine if all operators across all shifts, machines, etc., are consistent with each other – To determine if each operator makes consistent inspection decisions – To identify how well the operators are conforming to a standard Process Improvement – Validate measurement system before data analysis – Identify where training is needed, procedures are lacking, or standards not defined 6 2. Attribute GRR Study - Procedure 3 Set up ✓ Select enough parts from the process (let’s use minimum of 10 in this class) ✓ The parts should be approximately 50% “Good”, 50% “Bad” ✓ If possible, select borderline samples ✓ Number the parts 1 through n so that the numbers are not visible to the appraisers ✓ Select 2 or 3 appraisers that normally do the inspections Execution ✓ Each appraiser inspects the parts in random order and identifies as Pass or Fail ✓ Each appraiser repeats the inspection of each part 2 or 3 times Analysis ✓ Enter the data into Excel or Minitab to determine the effectiveness of the measurement system Evaluation ✓ Document the results ✓ Implement appropriate actions ✓ Rerun the study to verify the fixes 7 2. Attribute GRR Study 4 Additional Resource - Attribute Agreement Analysis (After Class): https://www.youtube.com/watch?v=6hCzmbjxFEo&list=PLK1HYFVC26P4uXaaBbaeI56ZThczAMHIV&index=9&t=1265s ASQ Stats Division Webinar: How to Set Up, Perform, and Analyze an Attribute Agreement Analysis 78 Min. 8 2. Attribute GRR Study 5 Reproducibility (do operators agree with each other?) Screen % Effective Score Between Appraiser Percent Agreement Repeatability (do operators agree with themselves?) Appraiser Score or % Agreement within Appraisers 9 3. Attribute GRR (Minitab) Use the excel sheet provided to enter the data. See example: Select the cells with the bold tect, copy and paste in Minitab George Adam Kumar Sample A-1 A-2 B-1 B-2 C-1 C-2 Standard 1 p P p p p p p 2 f f f f f f f 3 p p p p p p p 4 f f f f f f f 5 p p p p p p p 6 f f f f f f f 7 p p p p p p p 8 f f f f f f f 9 p p p p p p p 10 f f f f f f f 10 3. Attribute GRR (Minitab) 2 Navigate to Stat > Quality Tools > Gage Study > Attribute Agreement Analysis Fill out the dialog box as follows: Press OK 11 3. Attribute GRR (Minitab) 3 12 4. Attribute Agreement Analysis Effectiveness Ideally, we would like 100% agreement in all cases (both within Appraisers and Appraisers vs. Standard); however, this is unlikely to be the case, so we use the following guidelines: Effectiveness Decision (Percent Agreement) Measurement System ≥ 90% Acceptable 80% to 90% Marginally acceptable – may need improvement ≤ 80% Unacceptable – needs improvement Source: AIAG MSA Reference Manual, 4th edition Note! If the decisions are critical – these standards may need to be much higher 13 4. Attribute Agreement Analysis Effectiveness 2 1. Within Appraiser (Percent Agreement) = Appraiser Score - the percentage the operator agrees with himself 2. Between Appraisers (Percent Agreement) = Screen % Effective Score – The percentage all operators agree within and between themselves 3. Each Appraiser vs. Standard (Percent Agreement) = Appraiser vs. Standard Score – the percentage each operator agrees with the known standard 4. All Appraisers vs. Standard (Percent Agreement) = Screen % Effective Score vs. Standard – The percentage all the appraisers agreed within, between themselves AND with the standard. Producer Bias - Operator has a tendency to pass defective product (in doubt, protects Producer) Customer Bias - Operator has a tendency to hold back good product (in doubt, protects 14 customer) 5. Agreement Analysis (Kappa) According to AIAG Guidelines (MSA, 4th edition, page 137): Kappa Decision Measurement System > 0.75 Good to Excellent Agreement 0.4 - 0.75 Marginal Agreement < 0.4 Poor Agreement Source: AIAG MSA Reference Manual, 4th edition What exactly is an “acceptable” level of agreement depending largely on your specific field. In other words, check with your supervisor, professor or previous research before concluding that a Fleiss’ kappa over 0.75 is acceptable. 15 5. Agreement Analysis (Kappa) 2 Fleiss’ Kappa is a way to measure agreement between three or more raters Fleiss' kappa can be used with binary or nominal-scale. It can also be applied to Ordinal data (ranked data) Kappa ranges from -1 to 1, where: -1 indicates agreement is worse than chance 0 indicates no agreement better than chance (or no better than chance), 1 Indicates perfect agreement. Fleiss’ Kappa is defined as: P-Pe = probability of agreement that can be reached above chance 1-Pe = probability of agreement that was reached above chance 16 Source: Kappa Wikipedia 5. Agreement Analysis (Kappa) 3 Cohen's kappa only work when assessing the agreement between not more than two raters Cohen’s kappa, is defined as: 𝑃𝑜 − 𝑃𝑒 𝑘𝑎𝑝𝑝𝑎 = 1 − 𝑃𝑒 Where: Po = the relative observed agreement among raters. Pe = the hypothetical probability of chance agreement 17 5. Agreement Analysis (Kappa) – In-Class Exercise 4 Kappa Calculations Hypothesis Test Analyses - Kappa Cross-Tab Method – Presented in a matrix format – Hand calculations presented in class 18 5. Agreement Analysis (Kappa) 4 ❑ Hypothesis Test Analyses 19 5. Agreement Analysis (Kappa) 5 ❑ Kappa – Between Appraisers 𝑝𝑜 − 𝑝𝑒 𝑘𝑎𝑝𝑝𝑎 = 1 − 𝑝𝑒 Where, 𝑝𝑜= the sum of the observed proportions in the diagonal agreement cells 𝑝𝑒=the sum of the expected proportion in the diagonal agreement cells 20 5. Agreement Analysis (Kappa) 6 ❑ Kappa – Appraiser vs. Standard Item A B C Kappa 0.88 0.92 0.77 21 5. Agreement Analysis (Kappa) 7 ❑ AIAG Guidelines for Kappa evaluation According to AIAG, MSA Reference Manual, 4th edition, page 137) Maximum Kappa = 1 22 6. Agreement Analysis (P-Value) Hypothesis Test: Ho: agreement is due to chance (appraiser guessed the answers) Ha: agreement is not due to chance (appraiser did not guess) p > 0.05 Accept Ho p ≤ 0.05 reject Ho P-value ≤ α: The appraiser agreement is not due to chance (Reject H0) If the p-value is less than or equal to the significance level, you reject the null hypothesis and conclude that the appraiser agreement is significantly different from what would be achieved by chance. P-value > α: The appraiser agreement is due to chance (Fail to reject H0) If the p-value is larger than the significance level, you fail to reject the null hypothesis because you do not have enough evidence to conclude that the appraiser agreement is different from what would be achieved by chance. 23 6. Agreement Analysis (P-Value) 2 Repeatability Remember: Repeatability checks if 1 appraiser inspecting the same sample gets the same result each time. (Does each operator agree with himself/herself on both trials) Note: This doesn’t check if they are right – only if they are repeatable. Within Appraisers (checking the repeatability of each appraiser) In this example, 3 different appraisers checked 14 samples Appraiser A agreed with herself on both trials every time: Appraiser A is 100% repeatable Appraiser B agreed with himself only 11 times (he didn’t classify 3 parts the same way): 78.57% repeatability ( Appraiser B repeatability is not acceptable) Appraiser C also agreed with himself on both trials every time: Appraiser C is 100% 24 repeatable 6. Agreement Analysis (P-Value) 3 Fleiss’ Kappa Statistics Appraiser Response Kappa SE Kappa Z Repeatability Kappa P(vs > 0) A f 1.00000 0.267261 3.74166 The Kappa statistic determines if 0.0001 the appraiser results are better or p 1.00000 0.267261 3.74166 worse than random or chance 0.0001 B f 0.55080 0.267261 2.06091 results. 0.0197 Random chance = Kappa 0.0 p 0.55080 0.267261 2.06091 If someone guessed at a T/F test with 0.0197 C f 1.00000 0.267261 3.74166 30 questions, and got 15 right, Kappa 0.0001 would be 0. p 1.00000 0.267261 3.74166 Better than chance, k > 0 0.0001 Worse than chance, kANOVA>Test for equal variances Fill out the dialog box Press OK 4. Comparing Variable GRR Systems 16 Variance Analysis - Results 4. Comparing Variable GRR Systems 17 Minitab Result Analysis - Variance 1. Determine whether the differences between group Variances are statistically significant In these results, the null hypothesis states that the variance of 8 different gages are equal. Because the p-value If p-value > 0.05 => Variances are statistically is less than the significance level of equal 0.05, we reject the null hypothesis and If p-value < 0.05 => Variances are statistically conclude that at least one gage has different statistically different variance. 4. Comparing Variable GRR Systems 18 Minitab Results Analysis - Variance 2: Examine the group variances The plot shows the multiple comparison intervals for the standard deviations of each group. Group intervals that do not share any values are significantly different In this example, the variance of Gauge 1 and Gauge 8 have significantly different variances because their intervals don’t overlap. 4. Comparing Variable GRR Systems – In-Class Exercise Download the excel file called “Gauge Comparison Exercise” from the course shell Copy the GRR data to Minitab Perform the comparison of means and 10 Min. variances for both gauges using Minitab 5. Summary and Post Assessment (Non-graded) 1. Crossed Vs. Nested GRR? 2. Non-Replicable GRR procedures? 3. The procedure to comparing Variable GRR Systems? 10 Min. 4. The statistical methods to compare Variable GRR Systems? 5. Analysis of the comparison using Minitab? 45 References ▪ AIAG. (2010). MSA Reference Manual (4th Edition). ▪ Levine, D, Ramsey, P, Smidt R. Applied Statistics For Engineers and Scientists. 2001 NJ ▪ Doublas D. Montgomery, Introduction to Statistical Quality Control, P 120. 46 THANK YOU 47 Quality Core Tools II Week – 4: Variable Gauge Repeatability and Reproducibility GRR B. GRR– In Class Prepared By: Prof. Rafat Tahboosh B. GRR – In Class Lesson Outline 1. Feedback and Pre-assessment 2. Introduction to Variable GRR 3. Gage R&R Calculation Methods 4. Gage R&R In-Class Exercise 5. Summary and Post Assessment 2 B. GRR– In Class Lesson Objective 1. Describe the importance and application of variable GRR (gauge, repeatability, and reproducibility). 3 1. Feedback and Pre-assessment ❑ Feedback of Minitab GRR Instructions 15 Min. ❑ Pre-assessment (Not-graded): 1. What does “R&R” mean? 2. What does the Variable Gage R&R measure? 3. What does Repeatability mean? 4. What does Reproducibility mean? 5. What does EV, AV, PV, P/T mean? 6. What are the AIAG- Automotive Industry Action Group guidelines for Gage R&R? (how do we know the measurement system is acceptable or not) 7. What are the first 2 things we need to check before doing a Gage R&R? 4 2. Introduction to GRR - Variable MSA Steps Resolution Accuracy Precision Gauge reading Location Variation Width variation Smallest unit of (position of mean or (measurement measure required measurement error) error variation) Stability GRR Bias Repeatability Linearity Reproducibility 5 2. Introduction to GRR - Variable MSA Steps - Measurement System Error Variable Gauge R&R: calculates the measurement system variation related to Sources of Repeatability and Reproducibility. Variation total unit-to-unit Measurement Repeatability = Equipment Variation (EV) piece-to-piece System Error – will the same appraiser measuring the same True (actual) variation System variation part multiple times with the same measurement device get the same value. Precision Accuracy Width/spread location Reproducibility = Appraiser Variation (AV) GRR – will different appraisers measuring the same Bias Repeatability Reproducibility (diff from part with the same measurement device get equipment operator actual) equipment the same value. Uniformity Consistency Linearity Stability Once we can establish the gauge, or over range over time over range drift over time measurement system is both accurate and precise, we can trust it to make good decisions about our product. 6 2. Introduction to GRR - Variable MSA Steps - Measurement System Error 2 Width Variation Location Variation (Standard Deviation or Spread of the measurement system error) (Position of the Mean or measurement system error) A measurement system is Precision: precise if the measurement Accuracy: system variation is small The measurement “Closeness” to the compared to the Total “Closeness” to system is accurate if the repeated readings to Variation (%GRR < 30%) the true value bias is statistically zero each other (or reference value) Repeatability: Variation in measurements using 1 gage, 1 operator while measuring the same characteristic several Bias: difference between the times observed average of measurements and the reference value EV = Equipment Variation Reproducibility: Variation in the average of the measurements Stability: the change in bias over made by different appraisers using the same gage when time measuring a characteristic on a part AV = Appraiser Variation Linearity: the change in bias over the normal operating GRR: The combined estimate of measurement range of a gage system Repeatability and Reproducibility 7 2. Introduction to GRR - Variable MSA Steps - Measurement System Error CHAPTER III Recommended Practices for Replicable Measurement Systems......................................81 Section A Example Test Procedures...............................................................................................................83 Section B Variable Measurement System Study Guidelines..........................................................................85 Guidelines for Determining Repeatability and Reproducibility..............................................................101 Range Method.............................................................................................................................................102 Average and Range Method......................................................................................................................103 Analysis of Variance (ANOVA) Method.....................................................................................................123 Source: AIAG MSA Reference Manual 8 2. Introduction to GRR - Types of Data VARIABLE – Continuous Data that is measured (real numbers). Examples: Length, Thickness, Weight, Pressure, Temperature, etc. ATTRIBUTE – Categorical data that can be counted. Examples: Pass/Fail, Good/Bad 9 2. Introduction to GRR Gage R&R is an industry standard method to verify a measurement system is: Repeatable: consistent reading with same part, gauge and operator Reproduceable: consistent reading from operator to operator on same part and gauge Gage R&R validates the Precision of the gauge Bias & Linearity validates the Accuracy of the gauge 10 2. Introduction to GRR - AIAG General Guidelines for Evaluating a Variable GRR – Gauge Evaluation (Study Var%) 11 Source: AIAG MSA Reference Manual (4th Edition) 2. Introduction to GRR - Before running a Variable Gage R&R Study 1) Check the measurement device resolution Instrument Smallest Can use for no Reading tighter tolerance than… Digital Vernier 0.01mm xx +0.1/-0 mm Digital Micrometer 0.001mm xx +0.01/-0 mm Pressure Gauge 50psi Xx +500/-0 psi 12 2. Introduction to GRR - Before running a Variable Gage R&R Study 2 2) Ensure the measurement device is Accurate Measurement system needs to be stable – Stability analysis Bias needs to be statistically zero – Bias analysis Linearity needs to be acceptable – Linearity and Bias analysis 13 2. Introduction to GRR - Before running a Gage R&R Study 3) Collect parts to be measured It’s important to collect samples (parts, materials, widgets) that represent the majority of the variation present in the process (at the high and low ends of the tolerance, and even parts that are outside of tolerance). Sometimes it is helpful to have inspectors set aside a group of parts that represent the full spectrum of measurements. 20 samples are considered a good number for a Gage R&R study in a manufacturing environment, but smaller quantities can be used for low volume environments. 14 2. Introduction to GRR - Before running a Gage R&R Study 3 4) Ensure parts are not identifiable to the operators In order to avoid any human influence, only the person conducting the Gage R&R study should know which part is being measured. It must be a blind study, so they cannot subconsciously achieve results based on what they remember as the last reading. It is common to have an outside observer conduct and watch the entire Gage R&R study, to avoid these potential issues, which could make the measurement system look better than actually it is. 15 2. Introduction to GRR - Before running a Gage R&R Study 4 5) Determine who does the gage R&R Choose 2 or 3 appraisers (depending on the Gage R&R method) who do the measurements in production. These might be production people, quality inspectors, or lab technicians, depending on the situation. (During the tryout phase of a new part, you may have to use substitutes for the people who will actually do the measurements in the future.) It doesn’t matter who collects the data. A calibration technician, intern or engineer in training are good options. He or she would serve as a resource to answer questions and would have access to Gage R&R software. Source: https://www.qualitydigest.com/inside/metrology-article/basics-gauge-rr.html 16 2. Introduction to GRR - Before running a Gage R&R Study 5 6) Address all known issues with the gage: If you have prior knowledge that there are worn out cables, bent pins, untrained operators, outdated software, or any other problems with the gage, get those resolved first. You should have the philosophy that the Gage R&R will achieve acceptable results. Don’t spend the time and effort to perform a Gage R&R to prove what you already know or suspect. Inspect the gauge! (use a magnifying glass if necessary) 7) Calibrate the gage if required: Ensure that the gage is calibrated through its operating range. Recall that Gage RR and gage accuracy (bias) are two different things. 17 Source: http://leansixsigmadefinition.com/glossary/gage-rr/ 2. Introduction to GRR – Source of Variation 7 Min. Rockwell Hardness Tester (1) For what kind of tests we use (demo video) this machine? (2) What sources of potential variation do you see? 18 Source: https://youtu.be/NlWVmp_q_XE 2. Introduction to GRR – Source of Variation 2 What sources of potential variation do you see? Parallax error reading the dial or reading before settled Mounting the Selecting the indenter: too loose or wrong bed wrong indenter plate Raising the Selecting the platform too much wrong gauge or too little load 19 Source: https://youtu.be/NlWVmp_q_XE 2. Introduction to GRR – Source of variation 3 Verify Do ALL inspections and verifications Select the proper platform is scale before proceeding with a Gage R&R! leveled Select the correct tip Set correct pre-load Make sure tip and base are clean, no debris, and in good condition Set correct load Make sure unit is leveled Source: https://youtu.be/NlWVmp_q_XE 20 2. Introduction to GRR – Basic Variable Gage R&R Rules 1. Use only 1 gauge. (unless you are intentionally conducting an “expanded gage R&R) 2. Everyone uses the same measuring method. Measurement procedure should be available and appraisers should be trained. (Prevent variation due to the appraisers using different methods.) 3. Measure the same dimension on each part each time. 4. Measure each part in the same place to eliminate the possibility of within-part variation. 5. Conduct the study under the same type of conditions that exist when the parts are normally measured. (Prevent variation introduced by the location or environmental concerns of where the measurements are taken.) 21 2. Introduction to GRR – GRR Results - Overview GRR is 27.86%, since it is between 10% and 30%, it is marginally acceptable. 22 2. Introduction to GRR – GRR Results Overview The %Contribution guidelines are: Less than 1% - the measurement system is acceptable. Between 1% and 9% - the measurement system is acceptable depending on the application, the cost of the measuring device, cost of repair, or other factors. Greater than 9% - the measurement system is unacceptable and should be improved. AIAG: Automotive Industry Action Group 23 2. Introduction to GRR – GRR Results Overview 3 ❑ The %Study Var guidelines are: Less than 10% - the measurement system is acceptable. Between 10% and 30% - the measurement system is marginally acceptable, depending on the application, the cost of the measuring device, cost of repair, or other factors. Greater than 30% - the measurement system is unacceptable and should be improved. 24 2. Introduction to GRR - AIAG Guidelines for Evaluating GRR % Contribution %StudyVar Less then Measurement System is Less then Measurement System is Acceptable Acceptable 1% 10% 1% to MS is Marginally Acceptable 10% to MS is Marginally Acceptable Depends on the application 30% Depends on the application 9% Greater Measurement System is not Greater Measurement System is not than 9% Acceptable than 30% Acceptable 25 3. Variable GRR Calculation Methods Three common methods to calculate GRR: 1. Range Method 2. Average and Range Method 3. ANOVA Method – preferred method (WHY?) 26 3. Variable GRR Calculation Methods 2 Choosing a Gage R&R method ANOVA is usually the best choice. Source: http://www.rubymetrology.com/add_help_doc/MSA_Reference_Manual_4th_Edition.pdf 27 RANGE METHOD 28 3.1 Range Method Provides a quick approximation of measurement variability Provides overall picture only – Does not decompose variability into repeatability and reproducibility – Does not estimate any interaction effects (part*operator) – Typically used as a quick check to verify gage R&R has not changed Typically uses 2 appraisers, 5 parts, 1 gauge, 1 measurement per part Example – Conducted as an in-class exercise – see next page for formulas 29 3.1 Range Method 2 Percentage of the process standard deviation that the measurement variation consumes Source: http://www.rubymetrology.com/add_help_doc/MSA_Reference_Manual_4th_Edition.pdf 30 3.1 Range Method 3 Source: AIAG MSA Reference Manual 31 3.1 Range Method 4 In-Class Group Exercise: 10 Min. Open the excel file “Variable GRR exercises” from eConestoga and solve the Range Method GRR 32 Average & Range Method Using Minitab 33 3.2 Average and Range Method Provides an estimate of both repeatability and reproducibility Does not estimate any interaction effects (part*operator) Relatively straightforward method 34 3.2 Average & Range Method This is an older method, also called “long AIAG.” This method was intended for spreadsheets or pocket calculators, but has been replaced with the use of professional software such as Minitab. The average and range method assumes that an error term called “appraiser × part interaction” equals zero. If this assumption is not true (and it sometimes isn’t), then the calculations will not be reliable. When this method is used with spreadsheets, it requires the use of constants which must be found in tables. Performing this GRR method using Excel becomes more complicated, but if the companies don’t have Minitab Software, Measurement systems can be evaluated using the Average and Range Method. A worked example using this method can be found here: Source: https://www.spcforexcel.com/knowledge/measurement-systems-analysis/three-methods-analyze-gage-rr-studies 35 3.2 Average and Range Method 2 Conducting the study: Obtain at least 10 parts that represent the range of process variation – Number the parts 1 through n so that the numbers are not visible to the appraisers Calibrate the gauge if required Select 2 or 3 appraisers that normally measure the parts Have the first appraiser measure the parts in random order Have the other 1 or 2 appraisers measure the same parts Repeat the cycle for a second and third set of measurements 10 parts, 2 to 3 appraisers, 2 to 3 measurements per part, 1 gauge 36 3.2 Average and Range Method - Example Download the excel file “Variable GRR Exercises” Open Minitab Copy the “data for Minitab” from excel to Minitab Follow the Minitab Instructions on the next page 37 3.2 Average and Range Method - Minitab Instructions Stat > Quality Tools > Gage Study > Gage R&R Study (crossed) Complete the dialog box Select Xbar/R Method Click OK 38 3.2 Average and Range Method - Minitab Results Numerical Analysis Graphical Analysis ? 39 3.2 Average and Range Method - Numerical Analysis Recall: Total Gage R&R: The sum of the repeatability and the reproducibility variance components. 𝜎2𝑀𝑆 = 𝜎2𝑅𝑒𝑝𝑒𝑎𝑡𝑎𝑏𝑖𝑙𝑖𝑡𝑦 + 𝜎2𝑅𝑒𝑝𝑟𝑜𝑑𝑢𝑐𝑖𝑏𝑖𝑙𝑖𝑡𝑦 Repeatability: The variability in measurements when the same operator measures the same part multiple times. Reproducibility: The variability in measurements when different operators measure the same part at the various conditions defined by the other factors in the model. The Reproducibility term can be divided further into Operator, Operator*Part, and other main effects and interaction effects. Part-to-Part: The variability in measurements due to different parts. In addition to Part, other factors might be used to calculate part-to-part variation. Total Variation: The sum of part-to-part plus measurement system variance components. 40 𝝈𝟐 𝑻𝒐𝒕𝒂𝒍 = 𝝈𝟐 𝒑𝒂𝒓𝒕 − 𝒕𝒐 − 𝒑𝒂𝒓𝒕 + 𝝈𝟐 MS 3.2 Average and Range Method - Numerical Analysis 2 Key Results: %Contribution (%Tolerance, %Process) %Contribution is based on the estimates of the variance components. Each value in VarComp is divided by the Total Variation, and then multiplied by 100. – Ex: Part-to-Part variation = (1.21982/1.31332)*100 = 92.88% Therefore, 92.98% of the total variation in the measurements is due to the differences between parts. This high %Contribution is considered very good. When %Contribution for Part-to-Part is high, the system can distinguish between parts. The %Contribution for the total gage R&R is 7.12%, the measurement system is marginally acceptable. For more information, go to Is my measurement system acceptable?. 41 3.2 Average and Range Method - Numerical Analysis 3 2 Key Results: %Study Var (%SV) Use %Study Var to compare the measurement system variation to the total variation. Minitab calculates %StudyVar by dividing each value in StudyVar by Total Variation and then multiplying by 100. – Ex: %Study Variation for Total Gage R&R is (1.83469/6.87601) * 100 ≈ 26.68%. ? Minitab displays the %Tolerance column when you enter a tolerance value. Minitab displays the %Process column when you Precision of Measurement System: enter a historical standard deviation value. In this example the Gauge R&R is 26.68%, therefore the measurement system is marginally P = 6*SDGRR acceptable. 42 3.2 Average and Range Method - Numerical Analysis 4 Key Results: Number of Distinct Categories The Number of Distinct Categories value estimates how many separate groups of parts the Number of Distinct Categories = 5 system can distinguish. Minitab rounds down the value to the integer except when the value calculated is less than 1. #Distinct Categories = 1.41*(σparts/ In that case, Minitab sets the number of distinct σMS) categories equal to 1. Ndc=1.41*(PV/GRR) In this example, the number of distinct categories is 5, which indicates the system has good Measurement System is acceptable if resolution, it can distinguish between parts well. NDC: ≥5 43 3.3 Graphical Analysis - Components of Variation Graph This graph shows the variation from the sources of measurement error and part-to-part variation. Minitab displays bars for %Tolerance when you enter a tolerance value, and Minitab displays bars for %Process when you enter a historical standard deviation. This graph shows that part-to-part variability is much larger than the variability from repeatability and reproducibility. The total gage R&R variation is lower than 30% and is marginally acceptable 44 3.3 Graphical Analysis - Range Chart ▪ Shows whether any points fall above the upper control limit. ▪ If the operators measure consistently, the points will fall within the control limits. ▪ If one operator has points above the control limits – their method differs 45 3.3 Graphical Analysis - Range Chart 2 Shows whether most points fall beyond the control limits. We want the points to be outside the control limits – The area within the control limits is the “noise” or measurement sensitivity – If less than half of the points are outside the control limits then either the system lacks resolution or the samples do not represent the process variation 46 3.3 Graphical Analysis - Run Chart ▪ Shows whether multiple measurements for each part are close together. ▪ Multiple measurements for each part that are close together indicate small variation between the measurements of the same part. ▪ Outliers are indication of measurements not close together 47 Shows whether differences between operators are small compared to the differences between parts. A straight horizontal line across operators indicates that the mean measurements for each operator are similar. Ideally, the measurements for each operator vary an equal amount. 3.3 Graphical Analysis - Value by Appraiser ▪ Shows whether differences between operators are small compared to the differences between parts. ▪ A straight horizontal line across operators indicates that the mean measurements for each operator are similar. Ideally, the measurements for each operator vary an equal amount. 48 ANOVA METHOD Using Minitab 49 3.4 ANOVA Method Compared with the Average and Range method ANOVA – Is capable of handling any experimental set-up – Can estimate the variances more accurately – Extracts more information Interaction between parts and operators The main disadvantage is the complexity of the calculations 50 3.4 ANOVA Method – Additional Analysis If the p-value for the operator and part interaction is 0.05 or higher, Minitab removes the interaction because it is not significant and generates a second ANOVA table without the interaction. In this case, “Part Number * Appraiser” p-value > 0.05, meaning that “Part Number * Appraiser” interaction is not a significant source of variation, therefore Minitab removes this interaction from the analysis model. 51 3.4 ANOVA Method – ANOVA Output - Minitab 52 Shows whether the lines that connect the measurements from each operator are similar or whether the lines cross each other. Lines that are coincident indicate that the operators measure similarly. Lines that are not parallel or that cross indicate that an operator's ability to measure a part consistently depends on which part is being meas 3.4 ANOVA Method – ANOVA – Additional Graphical Shows whether the lines that connect the measurements from each operator are parallel or coincident or whether the lines cross each other. Lines that are coincident indicate that the operators measure similarly. Lines that are not parallel or that cross indicate that an operator's ability to measure a part consistently depends on which part is being measured. A line that is consistently higher or lower than the others indicates that an operator adds bias to the measurement by consistently measuring high or low 53 3.4 ANOVA Method – Analysis of GRR Studies If repeatability is large compared to reproducibility, the reasons may be: The gage may need to be redesigned to be more rigid. The location for gaging needs to be improved. There is excessive within-part variation. The instrument needs maintenance. If reproducibility is large compared to repeatability, the reasons may be: The appraiser needs to be better trained in how to use and read the gage instrument. Calibrations on the gage dial are not clear. 54 Assessing GRR Results 55 3.5 Assessing GRR Results There are 4 different approaches to determine the process variation (standard deviation): 1. Using the process variation When the selected parts represents the expected process variation (preferred) 2. Historical process variation When sufficient parts to represent the process are not available, but the existing process with similar process variation is available 3. Pp (or Ppk) target value When sufficient parts to represent the process are available, similar process variation is not available or the new process is expected to have less variability. 4. Specification Tolerance When the measurement system is to be used to sort the process, and the process has a 56 Pp reject Ho => Bias is statistically significant Which means that the measurement system is not measuring accurately, there is a significant difference between the average measurement and the true value, and therefore it cannot be used as it is, it needs to be corrected. 9 3. Accuracy Analysis: Bias 2 Procedure for conducting a bias study: 1. Obtain one part and establish its true value or reference value (obtain it from the lab for example). 2. Measure one part at least 10 times consecutively with one gauge by a single appraiser. Source: AIAG MSA Ref. Manual 10 3. Accuracy Analysis: Bias 3 Analysis of Results – Graphical 1. Calculate the bias for each reading: Biasi = Xi – True Value 2. Plot the histogram for bias 3. Check the data for normality 11 3. Accuracy Analysis: Bias 4 1. Check the histogram for abnormalities or 1. If data points lie along the straight line, data may follow a normal distribution => check p- outliers requiring additional analysis. value. 2. If Zero (bias = 0) lies inside the 95% confidence 12 2. If the normality p-value > 0.05 => Data follows interval then bias is statistically zero a normal distribution. Hence, we can continue with the statistical analysis for bias. 3. Accuracy Analysis: Bias 5 Analysis of Results – Numerical 1. Use 1 sample t-test using Minitab to calculate the t- statistic and corresponding p-value 2. See additional detailed analysis at the end of this presentation Stat>Basic Statistics>1-Sample t… Bias is acceptable (statistically zero) if: P-value >0.05 T- P-value Statistic 13 3. Accuracy Analysis: Bias Analysis Example Data – from excel file Stat>Basic Statistics>1-Sample t… Observed Trial Value Bias 1 6 -0.1 2 5.8 -0.3 3 6 -0.1 4 5.7 -0.4 5 6 -0.1 6 6.2 0.1 7 6.1 0 8 6.2 0.1 9 6.3 0.2 10 6 -0.1 11 6.1 0 12 6.1 0 13 5.9 -0.2 14 5.7 -0.4 15 6.1 0 14 3. Accuracy Analysis: Bias Analysis Example 2 Histogram Normality Test Bias P-Value Hypothesis test (using 1 sample T- test) Ho: bias = 0 Ha: bias ≠ 0 1. The histogram of the values did not show 1. The test for normality using the any abnormalities or outliers requiring probability plot shows that the data The p-value>0.05, therefore we additional analysis. The data appears to points lie along the straight line accept the null hypothesis, which be normal (it has a bell shape curve). 2. The P-Value is 0.695, it is greater means that the bias is acceptable Let’s confirm normality with the Normality than the alpha value of 0.05, (statistically zero). test. therefore there is no problem with 2. Zero lies inside the 95% confidence normality. Hence, we can continue interval, therefore bias is acceptable with the statistical analysis for bias. (statistically zero). 15 3. Accuracy Analysis: Bias Analysis Group Exercise Minitab => Stat>Basic Statistics>1-Sample t… 5 Min. Observed Trial Value-mm Bias ❑ As a group (2-3 students), use Minitab to conduct a 1 10 0 2 9.8 -0.2 bias analysis study for the provided measurements? 3 9.7 -0.3 4 10.1 0.1 5 10 0 6 10.1 0.1 ❑ Analyze the results indicating if the bias is acceptable 7 10.2 0.2 8 9.9 -0.1 or not and justify your answer? 9 9.8 -0.2 10 10.3 0.3 11 10.2 0.2 12 10 0 13 10.2 0.2 14 9.8 -0.2 15 9.9 -0.1 16 3. Accuracy Analysis - Bias Possible causes for significant Bias (or Linearity) – Instrument needs calibration, reduce the calibration interval – Worn instrument, equipment or fixture Top reasons that your data – Poor maintenance – air, power, hydraulic, filters, corrosion, rust, is not normally Distributed: – cleanliness – Outliers – Worn or damaged master(s), error in master(s) – – Equipment poor resolution minimum/maximum – Not enough data – Repeatability – Reference Value – Data follows a different – Improper calibration (not covering the operating range) or use of the distribution: – setting master(s) Poison distribution – Poor quality instrument – design or conformance Uniform distribution – Instrument design or method lacks robustness Weibull distribution – Wrong gage for the application Gamma distribution – Different measurement method – setup, loading, clamping, technique Beta distribution – Distortion (gage or part) changes with part size – Etc. – Environment – temperature, humidity, vibration, cleanliness – Violation of an assumption, error in an applied constant – Application – part size, position, operator skill, fatigue, observation 17 – error (readability, parallax) 4. Accuracy Analysis - Linearity Linearity tests Bias over the operating range of the instrument 18 4. Accuracy Analysis – Linearity 2 Source: Symphony Technologies, 2014. (5:44) 19 4. Accuracy Analysis – Linearity 3 Source: AIAG MSA Ref. Manual 20 4. Accuracy Analysis – Linearity 4 Procedure to conduct a Linearity Study: Parts Trials 1 2 3 4 5 1. Obtain at least 5 parts whose measurements cover 1 2.5 5 7.5 10 12.5 2 2.6 5.2 7.8 10.4 13 the operating range of the gage. 3 2.4 4.8 7.2 9.6 12 4 2.2 4.4 6.6 8.8 11 2. Have each part measured in the lab to obtain the 5 2.5 5 7.5 10 12.5 6 2.6 5.2 7.8 10.4 13 true values of each part. 7 2.7 5.4 8.1 10.8 13.5 8 2.3 4.6 6.9 9.2 11.5 3. A single appraiser measures each part at least 10 9 2.6 5.2 7.8 10.4 13 10 2.7 5.4 8.1 10.8 13.5 times consecutively. 4. Perform a Linearity Study in Minitab with the data Stat>Quality Tools>Gauge Study>Gage Linearity and Bias Study 21 4. Accuracy Analysis: Linearity Source: Symphony Technologies, 2014. (5:44) 22 4. Accuracy Analysis – Linearity Minitab Instructions Reference Data>Stack>Blocks of Stat>Quality Value 0.5 0.75 1 1.25 1.5 1 0.491 0.747 0.998 1.249 1.511 columns Tools>Gauge 2 3 0.502 0.5 0.749 0.75 1 1.035 1.248 1.253 1.508 1.5 Study>Gage Linearity 4 0.497 0.751 1.003 1.25 1.5 and Bias Study 5 0.497 0.753 1.001 1.25 1.502 6 0.501 0.753 0.999 1.253 1.501 7 0.5 0.75 0.999 1.251 1.5 8 0.497 0.754 1 1.25 1.5 9 0.499 0.749 1.023 1.26 1.501 10 0.502 0.748 1.003 1.25 1.501 Change column to numeric 23 4. Accuracy Analysis - In Class Experiment (Groups, Non-graded) Purpose: Linearity Study 20 Min. Tools: 1 large lego / any other product, 1 caliper / Measuring Tape Mobile App Procedure: 1. Groups of 4 or 5 2. Obtain one large Lego / any other product and Measuring Tape Mobile App 3. Assign an appraiser to measure the part 4. Professor trains all appraisers measuring the parts 5. Measure 5 different dimensions 10 times each in the Lego/product to test linearity 6. Enter the data in Minitab and perform the linearity analysis 7. Email results to your Professor (two power point slides: 1 slide with the measurements, 1 slide with the Linearity Chart and the Graphical and Numerical Analysis 24 4. Accuracy Analysis: Linearity 5 A measurement system Linearity is acceptable if the Fitted Line… slope ≈ 0 AND intercept ≈ 0 25 4. Accuracy Analysis - Linearity : Graphical Analysis 5 If “Bias = 0” line is entirely inside the 95% confidence interval of the fitted line, then: Bias 1. Fitted line slope is statistically 0, which =0 line means that: The fitted line is close to being flat The bias is not changing significantly across the operating range of the gauge Blue dots: individual bias points at each reference 2. Fitted line intercept is statistically 0, which value means that: Red dots: average bias points for each reference value The fitted line is close to zero) The bias is statistically zero across the operating range of the gauge 26 4. Accuracy Analysis - Linearity Numerical Analysis Slope: Null Hypothesis: Ho: Slope = 0 Alternative Hypothesis: Ha: Slope ≠ 0 if slope P-Value > 0.05, accept the null hypothesis. Bias is not changing significantly across the operating range of the gauge. => check intercept if slope P-Value < 0.05, reject the null hypothesis. Bias is changing significantly across the operating range of the gauge Linearity is not acceptable Measurement System is not acceptable (no need to check intercept) 27 4. Accuracy Analysis - Linearity Numerical Analysis 2 Intercept : Null Hypothesis: Ho: Intercept = 0 Alternative Hypothesis: Ha: Intercept ≠ 0 if intercept P-Value > 0.05, accept the null hypothesis. Bias is statistically zero across the gauge Linearity is acceptable (given that the slope is acceptable) if intercept P-Value < 0.05, reject the null hypothesis. Bias is not statistically zero across the gauge Linearity is not acceptable. Measurement System is not acceptable 28 4. Accuracy Analysis - Linearity Analysis Example 1 Graphical Analysis Bias = 0 line does not lie entirely inside the 95% confidence interval => Linearity is not acceptable Bias =0 Numerical Analysis Line Slope p-value = 0 Intercept p-value = 0 Since p-value Linearity is acceptable Bias =0 Numerical Analysis Line Slope p-value = 0.277 Intercept p-value = 0.208 Since p-value>0.05 the linearity is acceptable. 30 4. Accuracy Analysis - Linearity Example 3 Think, Pair and Share 5 Min. Graphical Analysis Numerical Analysis 31 4. Accuracy Analysis - Possible causes for significant Bias (or Linearity) – Instrument needs calibration, reduce the calibration interval Top reasons that your data is not – Worn instrument, equipment or fixture normally Distributed: – Poor maintenance – air, power, hydraulic, filters, corrosion, rust, – Outliers – cleanliness – Equipment poor resolution – Worn or damaged master(s), error in master(s) – minimum/maximum – Not enough data – Repeatability – Data follows a different – Reference Value distribution: – Improper calibration (not covering the operating range) or use of the Poison distribution – setting master(s) Uniform distribution – Poor quality instrument – design or conformance Weibull distribution – Instrument design or method lacks robustness Gamma distribution – Wrong gage for the application Beta distribution – Different measurement method – setup, loading, clamping, technique – Etc. – Distortion (gage or part) changes with part size – Environment – temperature, humidity, vibration, cleanliness – Violation of an assumption, error in an applied constant – Application – part size, position, operator skill, fatigue, observation – error (readability, parallax) 32 5. Bias - Additional Details 33 5. Bias – Detailed Analysis Source: AIAG MSA Ref. Manual 34 5. Bias – Detailed Analysis 2 Source: AIAG MSA Ref. Manual http://statcalculators.com/wp-content/uploads/2018/02/StudentTTable.png 35 6. Linearity - Additional Details 36 6. Linearity - Additional Details -Guidelines for Determining Linearity Parts Trials 1 2 3 4 5 1 2.7 5.1 5.8 7.6 9.1 2 2.5 3.9 5.7 7.7 9.3 3 2.4 4.2 5.9 7.8 9.5 4 2.5 5 5.9 7.7 9.3 5 2.7 3.8 6 7.8 9.4 6 2.3 3.9 6.1 7.8 9.5 7 2.5 3.9 6 7.8 9.5 8 2.5 3.9 6.1 7.7 9.5 9 2.4 3.9 6.4 7.8 9.6 10 2.4 4 6.3 7.5 9.2 11 2.6 4.1 6 7.6 9.3 12 2.4 3.8 6.1 7.7 9.4 Part Average