UNIT 1 Wind Resource and Data Analysis (1).pdf
Document Details
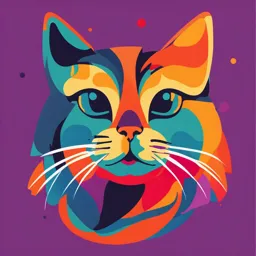
Uploaded by ReadableArcticTundra
Koforidua Technical University
Tags
Full Transcript
WIND ENERGY SYSTEMS II COURSE CODE ESE 510 SEMESTER 2 UNIT 1: Wind Resource CREDITS 3 LOCATION BM 102 Assessments (WRA) & Data PROGRAMME Analysis. BTECH 3 & 4 LECTURER: Isaac Kwasi Yanke...
WIND ENERGY SYSTEMS II COURSE CODE ESE 510 SEMESTER 2 UNIT 1: Wind Resource CREDITS 3 LOCATION BM 102 Assessments (WRA) & Data PROGRAMME Analysis. BTECH 3 & 4 LECTURER: Isaac Kwasi Yankey, DEPT.: Energy Systems Eng. 1 1.0 Introduction Wind Resource Assessment (WRA) is the discipline of estimating the strength of wind resources at a planned wind project site. The output of wind resource assessment in general is: 1. Wind conditions 2. Annual energy production at a project site. A financial model uses this data to compute the financial performance of the wind project. WRA is the core activity that determines viability of a wind project. 1.2 Why Assess Wind Resource 1. The Power in the wind is proportional to the Cube of the wind speed. This is the primary reason for wind resource assessment. 2. Also, Wind speed, Wind shear, Turbulence and gust intensity, all need to be specified when procuring a wind turbine and designing its foundation. 3. Wind Resources assessments are the cornerstone of identifying and mitigating risks and for realizing the potential rewards from a project. 1.3 Source of Wind Data There are three primary sources of wind data: 1. Onsite measurement is described in previous units with wind data measurement, analysis and reporting. 2. Network of Weather Stations all around the world that provide wind speed and direction data. Various organizations collect and provide this data. 3. Numerical Weather Models (long-term reanalysis data). The National Centers for Environmental Prediction (NCEP) and National Center for Atmospheric Research (NCAR) have cooperated in a project (denoted “reanalysis”). They produce a backdated record of more than 50 years of global analyses of atmospheric fields in support of the research and climate monitoring needs of communities and countries. 1.4 Resource Estimation Models Given the three types of data sources, a variety of models are used to estimate wind speed. The most commonly used models are: 1. Mesoscale Model 2. Computational Fluid Dynamics (CFD) 3. Microscale models. The purpose of these models is to create wind data for desired regions or sites. The created wind data contains: a. Wind speed b. Wind shear, c. Wind direction. 1.4.1 Mesoscale Models Mesoscale models describe weather phenomena that have a spatial resolution of 20 to 2,000 km and a temporal resolution of hours to days. These models take as input reanalysis data, elevation, and roughness data. The reanalysis data provides the external forcing of the model through boundary conditions. The most common mesoscale model are: KAMM (http://www.mesoscale.dk/) MM5 (www.mmm.uear.edu/mm5/mm5-home.html), MC2 (www.cmc.ec.gc.ca/rpn/modcom/en/index en.html). Mesoscale models are combined with microscale models to provide useful results for prospecting. The most common combination of mesoscale and microscale models are KAMM and WAsP. 1.4.2 CFD Models Computational Fluid Dynamics (CFD) models are used to model: 1. Airflow in complex terrains, for example, airflow around and over mountains and 2. thermal effects. These models provide a better understanding of turbulence when fine spatial resolution is used. CFD models are based on solving Reynolds- Averaged Navier-Stokes (RANS) equations along with turbulence models. Two popular 3-D CFD software programs are: 1. WindSim 2. 3D Wind. 1.4.3 WAsP, a Microscale Model The scale of applicability of microscale models is of the order of 100 km. Although the name may suggest otherwise, microscale is used to perform wind assessments for large wind farms that cover hundreds of square kilometers. Wind Atlas Analysis and Application Program (WAsP) is a microscale model developed by Riso in the late 1980s (http://www.wasp.dk/). Several of the most prominent wind assessment tools like: WindPRO and WindFarmer use the WAsP engine to perform wind resource assessments 1.5 Wind Resource Assessment Parameters Information in the resource assessment will include :- 1. Frequency distribution 2. Wind power density 3. Turbulence intensity 4. Daily average wind speeds 5. Monthly average wind speeds 6. Annual Average wind speeds 7. Wind Rose 1.5.1 Frequency Distribution The basic tool for estimate energy production. It shows the % of time that the wind blows at a certain speed. The wind speed are binned, meaning that speed between 0 and 1 m/s are binned as 1 m/s, wind speeds between 1 and 2 m/s are binned as 2 m/s, and so on. 1.5.2 Wind Power density (W/m2) It is defined as the wind power available per unit area swept by the turbine blades. It is a true indication of wind energy potential at the site than wind speed alone. Class Resource Wind Power Wind 2 Potential density w/m speed m/s 1 Poor < 200 < 5.6 Table 2.1: Wind power class 2 Marginal 200 – 300 5.6 – 6.4 3 Moderate 300 – 400 6.7 – 7 4 Good 400 – 500 7 – 7.5 5 Very Good 500 – 600 7.5 – 8 6 Excellent 600 – 800 8 – 8.8 7 Outstanding > 800 > 8.8 1.5.3 Turbulence Intensity It is the rapid disturbances in the wind speed and direction. Low < 0.1 Medium 0.1 ~ 0.25 Large > 0.25 High turbulence level cause extreme loading on wind turbine components. Turbulent locations: 1. severely limit the lifetime of wind turbines 2. increases the chance of their catastrophic failures. 𝑺𝒕𝒂𝒏𝒅𝒂𝒓𝒅 𝑫𝒆𝒗𝒊𝒂𝒕𝒊𝒐𝒏 𝒐𝒇 𝑾𝒊𝒏𝒅 𝒔𝒑𝒆𝒆𝒅 𝑻𝒖𝒓𝒃𝒖𝒍𝒆𝒏𝒄 𝑰𝒏𝒕𝒆𝒏𝒔𝒊𝒕𝒚 = 𝑴𝒆𝒂𝒏 𝑾𝒊𝒏𝒅 𝑺𝒑𝒆𝒆𝒅 Standard deviation of wind speed calculation (σ): A number that indicates how much wind speed changes above or below the mean. Example: For set of data v1 = 6 m/s n1= 19 times v2 =7m/s n2= 54 times v3=8 m/s n3= 42 times Total Number of times occurrence (n) = 115 Mean Wind Speed = (n1v1 + n2v2 + n3v3)/n = (19x6 +54x7 + 42x8)/ 115 = 7.2 m/s σ2 =1/(n-1){(n1v12 + n2v22 + n3v32) – 1/n(n1v1 +n2v2 +n3v3)2} =1/114 {(19x(6)2+54x(7)2 +42(8)2 – (1/115)(19x6 + 54x7 +42x8)2} = 0.495 m2/s2 σ = 0.703 m/s 𝑆𝑡𝑎𝑛𝑑𝑎𝑟𝑑 𝑑𝑣𝑖𝑎𝑡𝑖𝑜𝑛 𝑜𝑓 𝑤𝑖𝑛𝑑 𝑠𝑝𝑒𝑒𝑑 Turbulence intensity = mean wind speed = 0.703 / 7.2 = 0.097 1.5.4 Wind Rose Wind Rose is one of the ways that wind data is presented. It is a convenient tool for displaying wind speed and direction for site analysis. The figure shows an example of a Wind Rose. The Wind Rose shows strong winds from SW direction of the compass. It is a valuable tool for project layout and micro-siting. It is good to talk about the direction from which the wind blows. Fig 1.1 Wind Rose at Schiphol Airport, The Netherlands 1.6 Wind Resource Assessment Step 1: Process WRA starts with a preliminary assessment or prospecting. In this step check for publicly available wind resource maps and wind data. Step 2: Then an onsite wind measurement campaign is conducted. After wind data has been collected for sufficient period, typically one year or more, then a process of detailed WRA begins. Step 3: Begins with spatial extrapolation, in which measured data at multiple locations within the project area are used to estimate wind speeds over the entire project area. Step 4 : The next step is to extrapolate along the temporal dimension. (Long-Term Hindcast). A process called Measure-Correlate-Predict (MCP) is used with multiple reference datasets as input. Reference datasets are long-term wind data from a variety of sources Step 5: Annual Energy Production (AEP) is computed with several power production curves from different turbines. Step 6: The last step is to compute uncertainty of AEP, which consolidates the uncertainty in each factor that influences AEP. Step 7: The output of the WRA is input to the financial analysis step, in which the financial viability of the project is assessed. Fig.1.2 Overall process of (WRA) and financial analysis. ( Source :Jain 2011) 1.6.1 Phases of Wind Resource Assessment (WRA) Figure 1.2 describes the step-wise processes in WRA. Step 1 is preliminary assessment step comprises three steps. The duration of this step is 1–3 months. Step 2 is the onsite wind measurement task, which was covered in Wind Energy systems I. It is the longest duration task that may take 1–3 years. Steps 3–5 are performed in sequence and the total duration of the three steps is about 1–3 months. Fig 1.3 Phases of Wind Resource Assessment (Source: Jain 2011). 1.6.1.1 Preliminary Wind Resource Assessment Most projects start with no onsite measurement of wind data. A preliminary wind resource assessment is performed during the prospecting phase. It is a multistage process with the following steps: 1. Wind resource map lookup 2. Preliminary analysis of data from neighboring airports and other met-towers 3. Detailed analysis of wind data from neighboring airports and other met-towers 1. Wind Resource Map Lookup In the prospecting phase of a wind project, a developer starts with a high-level color wind resource map like the one shown in Fig. 1.3. 1. Most areas of the world have a wind resource map; a list of sources for maps is given below: a. Coarse world wind resource maps may be found at http://na.unep.net/swera ims/map/ b. US wind resource maps and a few international maps may be found at http://nrel.gov/wind/resource assessment.html c. European maps and international wind atlas may be found at: http://www.windatlas.dk. Fig. 1.4 Wind Resource Map of Ghana These maps provide annual average wind speed categories or wind speed ranges over large areas. These maps normally display wind speed at 50 m height. As expected, these maps display large-scale patterns with no temporal variation. The maps do not provide information about wind direction and shear. Therefore, the maps should be used as a tool to identify regions with good wind resources, and not for serious viability of a wind project. 2. Preliminary Analysis of Data from Neighboring Airports and Other Met- Towers If the wind resource maps show promise, then the next task is to find neighboring airports within 100-km radius that have topography that is similar to the site under consideration. The simplest form of preliminary airport data analysis is available in RetScreen (www.RetScreen.net), a free MS-Excel based application. It contains a database of monthly average wind speed data from major airports at an elevation of 10 m above the ground level. Information about wind direction and shear is not available from this data source. 3. Detailed Analysis of Wind Data from Neighboring Airports and Other Met- Towers The WAsP methodology provides a more sophisticated level of prospecting analysis. It also takes as input elevation and roughness to estimate shear. Applications like WindPRO, WindFarmer, or WAsP may be used to perform this analysis. The output of the analysis is: a. wind speed time series data at the desired site and at the desired height, b. wind rose (relationship between wind speed and wind direction derived from wind data), c. approximation of wind shear (based on surface roughness), d. estimate of average annual energy production. This preliminary assessment involves: 1. Analysis of hourly wind speed data available at 10 m height from local airport. 2. Creation of a GIS-based model of the airport site with contour elevation and terrain roughness. 3. Computation of a generalized Regional Wind Climate (RWC) from the airport data. 4. GIS-based model of site with modeling of elevation contour, roughness, and obstacles. 5. Translation of the RWC to the desired wind project site. 6. Customization of RWC to site. 1.6.1.2 Onsite Wind Measurement This was covered in Wind Energy Systems I. 1.7 Wind Data Analysis For estimating the wind energy potential of a site, the wind data collected from the location should be properly analyzed and interpreted. Modern wind measurement systems give us the mean wind speed at the site, averaged over a pre- fixed time period. Ten minutes average is very common as most of the standard wind analysis softwares are tuned to handle data over ten minutes. The data may also be categorized on: daily, monthly or yearly basis. 1.7 Distribution of Wind speeds The speed of the wind is continuously changing. This makes it desirable to use statistical methods to describe the wind. The following four (4) approaches will be considered: 1. direct use of data averaged over a short time interval; 2. the method of bins; 3. development of velocity and power curves from data; 4. statistical analysis using summary measures. 1.7.1 Direct use of Data Suppose one is given a series of N wind speed observations Ui, each averaged over the time interval Δt. These data can be used to calculate the following useful parameters: 1. The long-term average (Mean) and Median wind speed, 2. The Variance and standard deviation of the individual wind speed averages. 3. The average wind power density 4. The average wind machine Power, 5. The energy from the wind machines, E 1.7.1.1 The Mean and Median Suppose we have a set of measured wind speed Vi then the mean of the wind speed is defined as Vm: 𝒏 𝟏 𝑽𝒎 = 𝑽 𝒊 eq. 1.1 𝒏 𝒊=𝟏 Where the sample size or number of measured values is n The Median We occasionally use median in statistics. Considering the first set of data if n is odd then the median is the middle number after all the numbers have been arranged in order of size. If n is even the median is halfway between the two middle numbers when we rank the numbers. 1.7.1.2 The Standard Deviation One measure for the variability of velocities in a given set of wind data is the standard deviation (𝝈V). Standard deviation tells us the deviation of individual velocities from the mean value. Thus σ𝒏 𝒊=𝟏 𝑽𝒊 −𝑽𝒎 𝟐 𝝈𝑽 = eq.1.2 𝒏−𝟏 Lower values of 𝜎𝑉 indicate the uniformity of the data. Example 1 Five measured wind speeds are 2,4,7,8, and 9 m/s. Find the mean, the variance, and the standard deviation. u ̄ = 1/5{(2+4+7+8+9)}=6.00 m/s 𝝈 = √𝟖. 𝟓=2.92 m/s 1.7.1.2.1 Observed Specific Wind Speeds Wind speeds are measured in integer values are observed many times in the year. If we define the number of observation of a specific wind speed ui as mi then the mean wind speed is given as: Eq 1.3 w is the number of different values of wind speed observed and n is the total number of observations. The variance of such data is given as: eq. 1.4 Example 2 A wind data acquisition system located in the trade winds on the northeast coast of Ghana measures 6 m/s 19 times, 7 m/s 54 times, and 8 m/s 42 times during a given period. Find the i. mean, ii. variance, iii. standard deviation. Let us now define the probability p of the discrete wind speed ui being observed as: eq 1.5 Therefore considering obexample 2, the probability of an 8 m/s wind speed being served would be: 42/115 = 0.365 Hence the sum of all the probabilities will be: eq 1.6 Also we define a cumulative distribution function 𝑭 𝒖𝒊 as the probability that a measured wind speed will be less than or equal to 𝒖𝒊 Eqn. 1.7 t ot al number of observat ions is n = 211. Assignment 1 Table 2.2. W in A set of measured wind speeds is given in the Table. i ui mi p Find : 1 0 0 0 2 1 0 0 (i) p(ui) 3 2 15 0 (ii) F(ui) for each speed. 4 3 42 0 The total number of observations 5 4 76 0 6 5 51 0 is n = 211. 7 6 27 0 T he values of p(u i ) and F (u i ) are comput ed f 1.7.1.3 The Average Wind Power Density The average wind power density, P/A, is the average available wind power per unit area and is given by: eq.1.8 1.7.2 Method of Bins and Velocity Distributions The method of bins also provides a way to summarize wind data and to determine expected turbine productivity. The data must first be separated into the wind speed intervals or bins in which they occur. It is most convenient to use the same size bins. For developing the frequency distribution, the wind speed domain is divided into equal intervals (say 0-1, 1-2, 2-3, etc.) and the number of times the wind record is within this interval is counted. An example for the monthly frequency distribution of wind speed is given in Table 1.1. If the velocity is presented in the form of frequency distribution, the average and standard deviation can be calculated as: Table 1.1 Frequency distribution of monthly wind velocity Fig. 1.5. Probability distribution of monthly wind velocity Fig.1.6. Cumulative distribution of wind velocity The average and standard deviation of wind data presented in Table 1.1 are 8.34 m/s and 0.81 m/s respectively. Frequency histogram based on the above data is shown in Fig. 1.5 and is interesting to note that, the average wind speed does not coincide with the most frequent wind speed. Generally, the average wind speed is higher than the most frequent wind. The cumulative distribution of the above data is shown in Fig. 1.6. Assignment 2 Wind Speed m/s Hours per month 0-1 12 For the given table below 1-2 18 compute: 2-3 30 a. The Mean and standard 3-4 42 deviation and 4-5 47 b. Draw the monthly wind 5-6 57 frequency histogram and 6-7 60 the cumulative 7-8 34 distribution of wind speed 8-9 71 c. Calculate the: 9-10 65 i. Average wind power 10-11 57 density 11-12 40 ii. Average wind machine 12-13 35 power 13-14 28 iii. Energy from the wind 14-15 19 machine 15-16 9 1.7.3 Statistical Models for Wind Data Analysis The two major distribution functions that are used to describe the wind variations in a regime with an acceptable accuracy level are: 1. Weibull distribution 2. Rayleigh distribution Statistical analysis can be used to: a. determine the wind energy potential of a given site b. estimate the energy output of an installed wind turbine. If time series measured data are available at the desired location and height, there may be little need for a data analysis in terms of probability distributions and statistical techniques. That is, the previously described techniques may be all that are needed. For statistical analysis, a probability distribution is a term that describes the likelihood that certain values of a random variable (such as wind speed) will occur. Probability distributions are typically characterized by a probability density function or a cumulative 1.7.3.1 Weibull Distribution In Weibull distribution, the variations in wind velocity are characterized by the two functions; 1. The probability density function and 2. The cumulative distribution function. The probability density function f (V) indicates the fraction of time (or probability) for which the wind is at a given velocity V. It is given by: 𝒌 𝑽 𝒌−𝟏 − 𝑽Τ𝒄 𝒌 𝒇 𝑽 = 𝒆 (k>0, V>0,c>1) eq.1.11 𝒄 𝒄 Here, k is the Weibull shape factor and c is scale factor The cumulative distribution function of the velocity V gives us the fraction of time (or probability) that the wind velocity is equal or lower than V. Thus the cumulative distribution F(V) is the integral of the probability density function. Thus, ∞ 𝐹 𝑉 = න 𝑓 𝑉 𝑑𝑉 0 𝑽Τ 𝒌 = 1-𝒆− 𝒄 eq.1.12 1.7.3.1.1 The Mean /Average Velocity The average velocity (Mean) can be expressed as: 𝟏 𝑽𝒎 = 𝒄 𝜞 𝟏 + 𝒌 The standard deviation of wind speed, following the Weibull distribution is: 𝟏ൗ 𝟐 𝟏 𝟐 𝟐 𝝈𝑽 = 𝒄 𝜞 𝟏 + −𝜞 𝟏+ 𝒌 𝒌 1.7.3.1.3 Wind Speed Between V1 and V2 Probability of wind velocity being between V1 and V2 is given by the difference of cumulative probabilities corresponding to V2 and V1. Thus: P(V1