Unit-1 Statistics 2024 PDF
Document Details
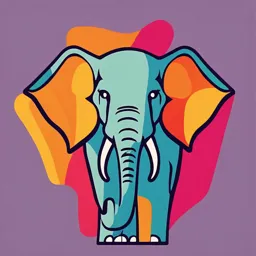
Uploaded by MotivatedOcarina
Christ University
2024
Dr. J. Ganesh Kumar
Tags
Related
- Psychological Statistics BPSY 55 PDF
- Psychological Statistics Lecture Notes PDF
- PSYC 204 Introduction to Psychological Statistics Lecture Notes PDF
- PSYC 204 Introduction to Psychological Statistics - Week 1 Lecture Notes (2024-09-03) PDF
- Psychological Statistics PDF
- Uni 1- Intro to Stats (PSYC1010) PDF
Summary
These lecture notes introduce statistics, covering definitions, functions in research, variables, levels of measurement, hypotheses, and the normal curve, its characteristics, application, skewness, kurtosis, population, and sampling. The presentation also details independent and dependent variables, discrete and continuous variables, and methods of statistical analysis.
Full Transcript
Dr. J. Ganesh Kumar, Assistant Professor, Department of Psychology, Christ (Deemed to be University), Kengeri Campus, Bangalore. Unit-1 Teaching Hours:10 Introduction to Statistics Statistics: definition, functions, and...
Dr. J. Ganesh Kumar, Assistant Professor, Department of Psychology, Christ (Deemed to be University), Kengeri Campus, Bangalore. Unit-1 Teaching Hours:10 Introduction to Statistics Statistics: definition, functions, and uses in research; Basic concepts: variables; levels of measurement, hypotheses; The Normal Curve: characteristics, applications, Skewness, Kurtosis; population, and sampling. Statistics: Derived from the Latin word ‘Status’ that means a group of numbers or figures; those represent some information of our human interest. A branch of mathematics that focuses on the organization, analysis, Statistics: and interpretation of a group of numbers. Definition Statistics consist of facts and figures such as average income, crime rate, birth rate, and so on. These statistics are usually informative and time saving because they condense large quantities of information into a few simple figures. ▪ Given the definitions, the following stages of Statistics emerge ▪ Collection of data- Decide how, where, when, what STATISTICS kind of data to be collected. ▪ Organization of data- Organize the collected data to make it comparable and simple. ▪ Analysis of data- To draw conclusions, analysis of data is required by different methods e.g. central tendency, correlation. ▪ Interpretation of data- Comparison and conclusions in simple and easy language. ▪ Presentation of data- Make the data meaningful, brief and attractive. ▪ To understand the fact ▪ To compare/ find out the difference Functions ▪ To find out the relationship and uses in ▪ To predict/ forecasting research ▪ Policy making ▪ Statistics are used to organize and summarize the information so that the researcher can see what happened in the research study and can communicate the results to others. ▪ Statistics help the researcher to answer the questions that initiated the research by determining exactly what general Functions and conclusions are justified based on the specific results that were uses in obtained. research ▪ Psychologists use statistical methods to help them make sense of the numbers they collect when conducting research. ▪ Psychologists usually use statistical software to carry out statistical procedure. 1. Descriptive statistics: Psychologists use descriptive statistics to summarize and describe a group of numbers Branches of from a research study. Statistical 2. Inferential statistics: Psychologists use inferential Methods statistics to draw conclusions and to make inferences that are based on the numbers from a research study but that go beyond the numbers. A population is the entire set of the individuals of interest for a particular research question. A sample is a set of individuals selected from a population, usually intended to represent the Population & population in a research study. Sample A variable is a characteristic or condition that changes or has different values for different individuals. ''A variable, as the name implies, is ” something that varies”. It may be weight, height, anxiety levels, income, body temperature and so on. Variable Each of these properties varies from one person to another and also has different values along a continuum. It could be physical or social and include religion, income, occupation, temperature, humidity, language, food, fashion, etc. Independent variable Dependent variable Discrete variable Continuous variable Types of Moderating variable variable Mediating variable Extraneous variable Independent variables (IV) are defined as those the values of which influence other variables. Dependent variables (DV) are defined as those the Independent values of which are influenced by other variables. A variable Vs dependent variable is the factor that appears, disappears, or varies as the experimenter introduces, Dependent removes or varies the independent variable variables The independent variable is the antecedent while the dependent variable is the consequent. If the independent variable is an active variable then we manipulate the values of the variable to study its affect on another variable. Independent vs Dependent variable A discrete variable consists of separate, indivisible categories. No values can exist between two neighboring categories. Discrete variables are commonly restricted to whole, Discrete countable numbers. variable Example: The number of children in a family or the number of students attending class. If you observe class attendance from day to day, you may count 65 students one day and 66 students the next day. Full mediation is when the entire relationship between the independent & dependent variables is through the mediator variable. If you take away the mediator, the relationship disappears. Since the real world is a complicated place with many interactions, this is less common than partial mediation. Partial mediation happens when the mediating variable is only responsible for a part of the relationship between independent & dependent variables. If the mediating variable is eliminated, there will still be a relationship between the independent and dependent variables; it just won’t be as strong. For a continuous variable, there are an infinite number of possible values that fall between any Continuous two observed values. A continuous variable is divisible into an infinite number of fractional parts. variable Example: Age, height, weight, time Moderator variable social support and quality of life depends on an individual’s stress level Socioeconomic status predicts parental education levels, Parental education levels predicts child reading ability, The correlation between socioeconomic status and child reading ability is greater when parental education levels are taken into account in your model. A hypothesis is an assumption that is made based on some evidence Must be specific Clear and precise to consider it to be reliable. If the hypothesis is a relational hypothesis, then it should be stating Hypothesis the relationship between variables. The way of explanation of the hypothesis must be very simple and it should also be understood Null hypotheses –H0 Alternative hypotheses-H1: Directional and Non Directional Nominal Scale Levels of Ordinal Scale Measurement Interval Scale s Ratio Scale A nominal scale is the 1st level of measurement scale in which the numbers serve as “tags” or “labels” to classify or identify the objects. A nominal scale usually deals with the non-numeric variables or the numbers that do not have any value. Characteristics of Nominal Scale Nominal It is qualitative. The numbers are used here to identify the objects. Scale Example: What is your gender? M- Male F- Female 2nd level of measurement that reports the ordering and ranking of data without establishing the degree of variation between them. Ordinal represents the “order.” Ordinal data is known as qualitative data or categorical data. It can be grouped, named and also ranked. Characteristics of the Ordinal Scale Shows the relative ranking of the variables It identifies and describes the magnitude of a variable Along with the information provided by the nominal scale, ordinal Ordinal Scale scales give the rankings of those variables Example: Ranking of school students – 1st, 2nd, 3rd, etc. Ratings in restaurants Evaluating the frequency of occurrences Very often Often Not often Not at all The 3rd level of measurement scale. It is defined as a quantitative measurement scale in which the difference between the two variables is meaningful. In other words, the variables are measured in an exact manner, not as in a relative way in which the presence of zero is arbitrary. Characteristics of Interval Scale Interval Scale The interval scale is quantitative as it can quantify the difference between the values It allows calculating the mean and median of the variables To understand the difference between the variables, you can subtract the values between the variables 4th level of measurement scale, which is quantitative. It possesses the character of the origin or zero points. Ratio scale has a feature of absolute zero Ratio Scale It doesn’t have negative numbers, because of its zero-point feature Ratio scale has unique and useful properties. One such feature is that it allows unit conversions like kilogram – calories, gram – calories, etc. ▪ Data – observations or measurements, usually quantified and obtained in the course of research. ▪Quantitative data – information expressed numerically, such as test scores or measurements of length or width. Organization ▪ Qualitative data – information that is not of data expressed numerically, such as descriptions of behavior, thoughts, attitudes, and experiences. If desired, qualitative data can often be expressed quantitatively through coding. ▪ Ungrouped data – are a set of scores that are distributed individually, where the frequency for each individual score is counted. Ungrouped Data & ▪ Grouped data – information that is grouped into one or Grouped Data more sets in order to analyze, describe, or compare outcomes at a combined level rather than at an individual level. For example, data from a frequency distribution may be arranged into class intervals. Frequency :The frequency of any value is the number of times that value appears in a data set. A frequency distribution is an organized tabulation of the number of individuals located in each category on the scale of Measurement. A table in which all of the scores are listed along with the frequency with which each occurs It shows whether the scores are generally high or low, whether Frequency they are concentrated in one area or spread out across the entire distribution scale, and generally provides an organized picture of the data. A frequency distribution can be structured either as a table or as a graph, but in either case, the distribution presents the same two elements: 1. The set of categories that make up the original measurement scale. 2. A record of the frequency, or number of individuals in each category. Cumulative Frequency Distribution In cumulative frequency distribution, the frequencies are Cumulative shown in the cumulative manner. Frequency The cumulative frequency for each class interval is the frequency for that class interval added to the preceding Distribution cumulative total. Cumulative frequency can also defined as the sum of all previous frequencies up to the current point. A table in which the scores are grouped into intervals and listed along with the frequency of scores in each interval. Class interval frequency distribution Inclusive class interval: The Exclusive class interval: The lower limit of a class does not get upper limit of one class is the repeated in the upper limit of the same as the lower limit of the preceding class succeeding class Class frequency cf Class Adjusted freque cf interval interv CI ncy al 20-30 5 5 Inclusive vs 30-40 7 12 20-29 19.5-29.5 5 5 30-39 29.5-39.5 7 12 Exclusive 40-50 4 16 40-49 39.5-49.5 4 16 50-60 3 19 50-59 49.5-59.5 3 19 A graphical representation is the geometrical image of a set of data. It is a mathematical picture and it enables us to think about a statistical problem in visual terms. ADVANTAGES ▪ The data can be presented in a more attractive and an appealing form Graphical ▪ Provides a more lasting effect on the brain representatio ▪ Comparative analysis and interpretation may be effectively and easily made n ▪ May help in the proper estimation, evaluation, and interpretation ▪ Helps in the forecasting, as it indicates the trend of the data in the past A graphical representation of a frequency distribution in which vertical bars are centered above each category along the x-axis and are separated from each other by a space, indicating that the levels of the variable represent distinct, unrelated categories. If the data collected are on a nominal scale (a categorical variable for which each value represents a discrete category), then a bar graph is most appropriate. Bar graph A barlike graph of a frequency distribution in which the values are plotted along the horizontal axis and the height of each bar is the frequency of that value; the bars are usually placed next to each other without spaces. It is used to display the distribution of a single continuous variable (e.g. Intelligence, motivation, stress ). Histogram A graphic display in which a circle is cut into wedges, with the area of each wedge being proportional to the percentage of cases in the category represented by that wedge. A pie chart generally works best when there are not many categories being shown. Pie chart/Pie Diagram Step 1: First, Enter the data into the table. Step 2 :Add all the values in the table to get the total. Step 3: Next, divide each value by the total and multiply by 100 to get a percent. Step 4: Next to know how many degrees for each “pie sector” How to Create we need, we will take a full circle of 360° and follow the calculations below: a Pie Chart? Step 5: The central angle of each component = (Value of each component/sum of values of all the components)✕360° Step 6: Draw a circle and use the protractor to measure the degree of each sector. FVOURI N % pie sector TE MOVIE COMIC 15 (15/60)x100=25% (15/60)x360*=90* ROMAN 25 (25/60)x100=42% (25/60)x360*=150* CE ACTION 20 (20/60)x100=33% (20/60)x360*=120* Pie chart A frequency polygon is almost identical to a histogram, which is used to compare sets of data. It uses a line graph to represent quantitative data. To construct a polygon, you begin by listing the numerical scores (the categories of measurement) along the X-axis. Then, A dot is centered above each score so that the vertical position of the dot corresponds to the frequency for the Frequency category. Calculate the classmark for each class interval. The formula Polygon for class mark is: Classmark = (Upper limit + Lower limit) / 2 Mark all the class marks on the horizontal axis. It is also known as the mid-value of every class. A continuous line is drawn from dot to dot to connect the series of dots. The graph is completed by drawing a line down to the X-axis (zero frequency) at each end of the range of scores. Frequency Polygon Frequency Polygons Histograms A histogram is a graph that depicts data A frequency polygon graph is a curve that is through rectangular-shaped bars with no depicted by a line segment spaces between them. In a frequency polygon graph, the midpoint In a histogram, the frequencies are evenly Frequency of the frequencies is used. spread over the class intervals. polygons vs Histograms The accurate points in a frequency polygon graph represent the data of the particular The height of the bars in a histogram only depicts the quantity of the data. class interval. Comparison of data is visually more Comparison of data is not visually appealing accurate in a frequency polygon graph. in a histogram graph. The construction of frequency curve is similar to that of a frequency polygon. The frequency curve is drawn freehand by joining the points of frequency polygon as closely as possible. Advantage : More smooth appearance of data than frequency polygon. The only difference between a frequency curve and a frequency polygon is that: Frequency polygon is drawn by Frequency joining points by a straight line. Frequency curve is drawn by a curve smooth hand. When frequency polygon is smoothed out then it is known as frequency curve. curves or curved shapes Ogives are graphs that are used to estimate how many numbers lie below or above a particular variable or value in data. To construct an Ogive, firstly, the cumulative frequency of the Cumulative variables is calculated using a frequency table. It is done by adding the frequencies of all the previous variables frequency in the given data set. The result or the last number in the curve /Ogive cumulative frequency table is always equal to the total frequencies of the variables. First we prepare the cumulative frequency table, then the cumulative frequencies are plotted against the upper or lower limits of the corresponding class intervals. By joining the points the curve so obtained is called a cumulative frequency curve or ogive. To find the median of the given set of data. Uses of Ogive Less than and greater than, cumulative frequency curve is drawn Curve on the same graph, we can easily find the median value. The point in which, both the curve intersects, corresponding to the x-axis, gives the median value. Less than Ogive Greater than or more than Ogive Marks Frequency More than Less than Cumulative Cumulative Frequency Frequency Methods of 0-5 3 60 3 Ogives 5-10 10-15 8 12 57 49 11 23 15-20 14 37 37 20-25 10 23 47 25-30 6 13 53 30-35 5 7 58 35-40 2 2 60 Ogive THANK YOU