Psychological Statistics BPSY 55 PDF
Document Details
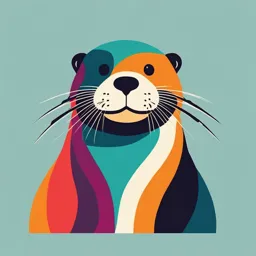
Uploaded by FavoriteHelium
St. Mary's School
Martonito, Justine Alliyah C.
Tags
Related
Summary
This document introduces psychological statistics, covering topics like variables, values, scores, data, populations, parameters, and samples. It also discusses the relationship between populations and samples.
Full Transcript
PSYCHOLOGICAL STATISTICS BPSY 55- Psychological Statistics Martonito, Justine Alliyah C. | BS- Psychology 1-1 | Sir Jhunar John Tauy, RPm., CCH 1.1 Introduction to VARIABLE Psychological Statistics...
PSYCHOLOGICAL STATISTICS BPSY 55- Psychological Statistics Martonito, Justine Alliyah C. | BS- Psychology 1-1 | Sir Jhunar John Tauy, RPm., CCH 1.1 Introduction to VARIABLE Psychological Statistics - Characteristic or condition that changes or has different values for different individuals Why Learn Statistics? (Aron et. al) VALUE - Crucial to being able to read psychology research articles - Possible number or a category that a score can - Crucial to doing research yourself have - Develops your analytic and critical thinking - E.g 0–20 in a Stress Scale, Male or Female SCORE WHAT IS PSYCHOLOGY - Or Raw Score, is a particular person’s value on a - Scientific study of human behavior and mental variable processes - GOALS: Describe, Predict, Explain, and Control DATA WHAT IS STATISTICS - Are measurements of observation - Datum- single measurement or observation and is - A branch of mathematics that deals with the commonly called a score or raw score organization, analysis, and interpretation of group of numbers - A set of mathematical procedures for organizing, DATA SET summarizing, and interpreting information - Collection of measurements or observations POPULATION 2 TYPES OF STATISTICS - Set of all the individuals of interest in a particular DESCRIPTIVE STATISTICS study - Are statistical measures used to summarize, organize, and simplify data PARAMETER - Respondents: Quantitative - Participant: Qualitative - A value– usually a numerical value– that describes a population INFERENTIAL STATISTICS - Consist of techniques that allows us to SAMPLE study samples and then make generalizations about populations from - Set of individuals selected from a population, which they were selected usually intended to represent the population in a - “The sample represents the population” research study STATISTIC - A value– usually a numerical value– that describes a sample SAMPLING ERROR Magnitude Equal Absolute Interval Zero - Naturally occurring discrepancy or error that exists between a sample statistic and the corresponding ✖ ✖ ✖ Nominal population parameter Ordinal ✔ ✖ ✖ VARIABLES AND MEASUREMENT Interval ✔ ✔ ✖ DISCRETE VARIABLE - Separate, indivisible categories Ratio ✔ ✔ ✔ - E.g Number of Students, Number of Correct Answers DIFFERENT TYPE OF DATA CONTINUOUS VARIABLE - Infinite number of possible values that fall QUALITATIVE between any two observed values - Nominal, Dichotomy, Ordinal, Discrete QUANTITATIVE DICHOTOMOUS VARIABLE - Interval, Continuous, Ratio - One that takes on only of two possible values when observed or measured - Artificial Dichotomous - derived from scores (passed or failed) 1.2 Three Data Structures and - True Dichotomous- naturally occurring Quantitative Research (Male/Female, Yes/No, Heads/Tails) Methods LEVELS OF MEASUREMENT DATA STRUCTURE 1: One Group with One or More Separate Variables Measured for Each Individual - Also called SCALES OF MEASUREMENT, is a classification that describes the nature of - One (or more) variables measured per individual information within the values assigned to variables - Variable(s) is/are described by descriptive statistics NOMINAL - May use category and/or numerical variables - Also known as Categorical Values - Variable with values that are categories DESCRIPTIVE RESEARCH - E.g Sex, Nationality, Religion, Civil Status - Involves measuring one or more separate variables ORDINAL for each individual with the intent of simply - Also known as Rank-Order Value describing the individual variables - E.g Highest Educational Attainment, S,M,L, Likert Scale SURVEY RESEARCH INTERVAL - A useful way of obtaining data about people’s - Variable that contains equal-interval opinions, attitudes, preferences, and experiences between numbers and contain no that are hard to observe directly absolute zero point - Data may be obtained using questionnaires and - Distance between value interviews - E.g Temperature, IQ, Stress RELATIONSHIP BETWEEN VARIABLES RATIO - An interval scale with the additional - Two (or more) variables observed and measured feature of an absolute zero point - One of two possible data structures used to - Variables that have a natural order, a determine what type of relationship exists quantifiable difference between values and a ‘true zero’ value. DATA STRUCTURE 2: One Group with Two Variable - E.g Time to Complete a task, number of Measured for Each Individual correct answers, weight gain in the past 6 months. - One group of respondents - Measurement of two variables for each respondent - Goal is to describe the type and magnitude of the relationship - Patterns in the data reveal relationships - Non-experimental method of study CORRELATIONAL METHOD QUASI-EXPERIMENTAL DESIGN - Two different variables are observed to determine - Often seem like (as the prefix quasi implies) real whether there is a relationship between them experiments, but they lack one or more of its - Two is observed, one is measured essential elements, such as manipulation of antecedents (independent variable) and random CORRELATIONAL METHOD LIMITATIONS assignment to treatment conditions - Can demonstrate the existence of a relationship - Does not provide an explanation for the relationship NONEQUIVALENT GROUP DESIGN - Does not demonstrate a cause-and-effect relationship between two variables - A design in which the researcher compares the effect of different treatment conditions on pre-existing groups of respondents/subjects DATA STRUCTURE 3: Comparing Two (or more) Groups of Scores PRETEST/POSTTEST DESIGN - One variable defines the group - Scores are measured on second variable - Design used to assess whether the occurrence of - Both experimental and non-experimental studies an event alters behavior; scores from use this structure measurements made before and after the event EXPERIMENTAL METHOD EX-POST FACTO STUDY - One (or more) variable is manipulated while - A study which a researcher systematically another variable is observed or measured examines the effects of pre-existing subject - Aims to establish a cause-and-effect relationship characteristics (or subject variables) by forming between two variables and attempts to control all group based on theses naturally occurring other variables to prevent them from influencing differences between subjects the results LONGITUDINAL DESIGN INDEPENDENT VARIABLE - A method in which the same group of subjects is - The variable that is manipulated by the researcher followed and measured at different points in time; a - Should consist of at least two (or more) levels method that looks for change across time (treatment conditions) to which subjects are exposed APA-STYLE RESEARCH WRITING - Style of documentation of sources used by the DEPENDENT VARIABLE American Psychological Association - The variable that is being observed or measured to - This form of writing research papers is used mainly assess the effect of the treatment in the social sciences like psychology, anthropology, sociology, as well as education fields EXPERIMENTAL CONDITION Sections of an APA-Style Research Paper - A condition in an experiment wherein the subjects - Title will receive the experimental treatment - Abstract - Experimental random assignment: manipulation of - Introduction independent variables, control of extraneous - Method variables and measurement of dependent variable - Results - Discussion - References CONTROL CONDITION - Appendices - A condition in an experiment wherein the subjects do not receive the experimental treatment A group of researchers conducted a study that will determine the effects of background noise in class performance. Students in one classroom worked on a mathematical task with calming music in the background. Students in the second classroom heard aggressive, exciting music, and students in the last room had no music at all. - INDEPENDENT VARIABLE - DEPENDENT VARIABLE - LEVELS OF INDEPENDENT VARIABLE HOW TO MAKE GROUPED FREQUENCY TABLES 1.3 Descriptive Statistics 1. Determine the: - Range (Highest Score – Lowest Score) - Class Size (K = 1 + 3.3 log (n)) DESCRIPTIVE STATISTICS - Class Width/Interval (CW = R/K). 2. There should be no overlapping elements in the class - Procedures for summarizing a group of scores or otherwise intervals. making them more understandable 3. Show or include all classes. 4. There should be enough classes to accommodate all data. FREQUENCY DISTRIBUTION 5. The classes must be equal in width. - An organized tabulation of the number of individual located in each category on the scale measurement WAYS OF PRESENTING DATA HISTOGRAM FREQUENCY DISTRIBUTION - barlike graph of a frequency distribution in which the - An organized tabulation of the number of individual values are plotted along the horizontal axis and the height located in each category on the scale of measurement of each bar is the frequency of that value; the bars are FREQUENCY TABLE usually placed next to each other without spaces, giving - Ordered listing of number of the appearance of a city skyline individuals/subjects/respondents having each of the different values for a particular variable Example: The following set of n = 20 scores was obtained from a 10-point statistics quiz. We organize these scores by constructing a frequency distribution table. 8, 9, 8, 7, 10, 9, 6, 4, 9, 8, FREQUENCY POLYGON 7, 8, 10, 9, 8, 6, 9, 7, 8, 8 - Continuous line that represents the frequencies of scores within a class interval, based on a histogram; used for continuous data HOW TO MAKE FREQUENCY TABLES 1. 1. Make a list down the page of each possible value, from highest to lowest. 2. Go one by one through scores, making a mark for each next to its value on your list. 3. Make a table showing how many times each value on your list is used. COLUMN CHART 4. Figure the percentage of scores for each value. - a data visualization where each category is represented by a rectangle, with the height of the rectangle being PROPORTION proportional to the values being plotted - Measures the fraction of the total group that is associated with each scores - Formula: p= f/n PERCENTAGE - Amount of something, often expressed as a number out of 100 - Formula: p(100)= f/n(100) BAR GRAPH - identical to column charts, but in this chart, categories are organized vertically on the y-axis, and values are shown horizontally on the x-axis. LINE GRAPH - (also known as a line plot or line chart) is a graph which GROUPED FREQUENCY TABLE uses lines to connect individual data points that display quantitative values over a specified time interval - Frequency table in which the number of individuals (frequency) is given for each interval of values - Interval- range of values in a grouped frequency table that are grouped together. (For example, if the interval size is 10, one of the intervals might be from 10 to 19.) MEDIAN (Mdn) 1.3 Descriptive Statistics - middle score when all the scores in a distribution are arranged from lowest to highest (Measures of Central Tendency and Shapes of Distributions) STEPS FOR FINDING THE MEDIAN: CENTRAL TENDENCY 1. Line up all the scores from lowest to highest. - Typical or most representative value of a group of scores 2. Figure how many scores there are to the middle score by - Mean, Median, Mode adding 1 to the number of scores and dividing by 2. 3. Count up to the middle score or scores MEAN (M) For the following sample, find the median: - Arithmetic average of a group of scores 5, 6, 9, 11, 5, 11, 8, 14, 2, 11 - Sum of scores divided by the number of scores - The balance point of the distribution 2, 5, 5, 6, 8, 9, 11, 11, 11, 14 Mdn= 8.5 µ= ∑x/n (population mean) M= ∑x/n (sample mean) WHEN TO USE MEDIAN? The following are the stress ratings of 30 students in the - with rank-ordered variables first week of their statistics class: - non-normal or skewed distributions - when a distribution has one or more outliers 8, 7, 4, 10, 8, 6, 8, 9, 9, 7, 3, 7 ,6, 5, 0, - Outliers – score with an extreme (very high or 9, 10, 7, 7, 3, 6, 7, 5, 2, 1, 6, 7, 10, 8, 8 very low) in relation to the other scores in the distribution. Calculate the M - rarely used in psychology research M = ∑x/n = 193/30 = 6.43 MODE - value with the greatest frequency in the distribution STEPS FOR FIGURING THE MEAN: The following are the stress ratings of 30 students in the 1. Add up all the scores first week of their statistics class: 2. Divide the sum by the number of scores. 8, 7, 4, 10, 8, 6, 8, 9, 9, 7, 3, 7, 6 ,5, 0, 9, 10, 7, 7, 3, 6, 7, 5, 2, 1, 6, 7, 10, 8, 8 WEIGHTED MEAN Find the Mode - an average in which each observation in the data set is MODE: 7 assigned or multiplied by a weight before summing to a single average value - Weighted Mean= ∑xw/∑w WHEN TO USE MODE? - with categorical variables (nominal) CHARACTERISTICS OF MEAN - rarely used in psychology research - Changing a score in the distribution can affect the value of the mean - Introducing a new score or removing a score can affect the SHAPES OF A FREQUENCY DISTRIBUTION value of the mean. UNIMODAL DISTRIBUTION - Adding or subtracting a constant from each score will - frequency distribution with one value clearly change the value of the mean. having a larger frequency than any other - Multiplying or dividing each score by a constant will change the value of the mean WHEN TO USE MEAN? - very commonly used in quantitative research, especially in psychological studies BIMODAL DISTRIBUTION - approximately normally distributed data - frequency distribution with two approximately - with equal-interval variables equal frequencies, each clearly larger than any ✔Continuous Data/Variable the others ✔Interval/Ratio REPORTING THE MEAN IN RESEARCH ARTICLES (APA FORMAT) The students who were exposed to classical music during the experiment have significantly higher test scores (M = 23.50, SD = 1.24) than those students who were exposed to rock music (M = 19.24, SD = 2.32). MULTIMODAL DISTRIBUTION NORMAL CURVE - frequency distribution with two or more high - specific, mathematically defined, bell-shaped frequencies separated by a lower frequency frequency distribution that is symmetrical and unimodal - distributions observed in nature and in research commonly approximates it RECTANGULAR DISTRIBUTION - frequency distribution in which all values have approximately the same frequency KURTOSIS - extent to which a frequency distribution deviates from a normal curve in terms of whether its curve in the middle is more peaked or flat than the normal curve - Leptokurtic- the scores are concentrated towards the mean - Mesokurtic – normal curve - Platykurtic– the scores have an SYMMETRICAL DISTRIBUTION extremely large deviation from the - distribution in which the pattern of frequencies mean on the left and right side are mirror images of each other SKEWED DISTRIBUTION IF n 300 - The Skewness must be between +2 and -2 - The Kurtosis must be between +7 and -7 TEST FOR NORMALITY SHAPIRO-WILK - n105 If a person has an IQ of 105, ,What percentage of people have an IQ 2.50) = 0.062 5. Reject the null hypothesis. The training significantly increases friendliness. WHEN TO USE ONE-TAILED OR TWO-TAILED TEST DIRECTIONAL HYPOTHESIS - Research hypothesis predicting a particular direction of difference between population ONE-TAILED TEST - Is a situation in which the region of the comparison distribution in which the H0 would be rejected is all on one side (tail) of the distribution TWO-TAILED TEST EXAMPLE A researcher predicts that making people hungry will affect how well they do on a coordination test. A randomly selected person is asked not to eat for 24 hours before taking a standard coordination test and gets a score of 400. For people in general of this age group and gender, tested under normal conditions, coordination scores are normally distributed with a mean of 500 and a standard deviation of 40. Using the.01 significance level, what should the researcher conclude? NON-DIRECTIONAL HYPOTHESIS - Research hypothesis that does not predict a H0: Hunger has no significant effect on coordination test particular direction of difference between the scores. population α= 0.01 TWO-TAILED TEST - Is a situation in which the region of the comparison distribution in which the H0 would be rejected is divided into two sides (tails) of the distribution. z= (x-µ)/σ z= (400-500)/40 z= -2.50 “If the p is low, the H0 must go” Making people hungry does not have a significant effect on the scores in a coordination test, z = -2.50, p >.01 DETERMINING CUTOFF SCORES WITH TWO-TAILED TEST CONVENTIONAL LEVEL OF SIGNIFICANCE.01 significance level p <.05 p <.01 Level of significance widely used in psychology STATISTICAL SIGNIFICANCE ON TABLES CENTRAL LIMIT THEOREM - For any population with the mean and the standard * - significant at 0.05 level deviation, the distribution of sample means for ** - significant at 0.01 level sample size n will have a mean of µ and σ of *** - significant at 0.001 level and will approach a normal distribution as n approaches infinity LAW OF LARGE NUMBERS 7. Hypothesis Tests with Means of - It states that the larger the sample size (n), the Samples more probable it is that the sample mean will be close to the population mean. DISTRIBUTION OF SAMPLES MEANS HYPOTHESIS-TESTING WITH A DISTRIBUTION OF MEANS - Distribution of means of samples of a given size (n) (z Test) from a population - hypothesis-testing procedure in which there is a - Also called sampling distribution of mean single sample, and the population variance/SD is - Comparison distribution when testing hypotheses known. involving a single sample of more than one individual SAMPLING DISTRIBUTION - A distribution of statistics obtained by selecting all the possible samples of a specific size from a REPORTING z TEST RESULTS IN RESEARCH ARTICLES population The study suggests that traumatic events have a significant effect on the number of dreams, z = 4.48, p <.05. Specifically, DETERMINING THE CHARACTERISTICS OF A DISTRIBUTION Those subjects who experienced traumatic events have a OF MEANS greater number of dreams than those who did not experience traumatic events. RULE 1: The mean of a distribution of means is the same as the mean of the population of individuals. CONFIDENCE INTERVAL (CI) - roughly speaking, the range of scores (that is, the scores between an upper and lower value) that is likely to include the true population mean; RULE 2a: The variance of a distribution of means is the - more precisely, the range of possible population variance of the population of individuals divided by the means from which it is not highly unlikely that you number of individuals in each sample. could have obtained your sample mean - 95% OR 99% Confidence Intervals STEPS IN FIGURING CONFIDENCE LIMITS 1. Figure the Standard Error RULE 2b: The SD of a distribution of mean is the square root 2. For the 95% confidence interval, figure the raw of the variance of the distribution of means. scores for 1.96 standard errors above and below the sample mean; for the 99% confidence interval, figure the raw scores for 2.58 standard errors above and below the sample mean STANDARD ERROR OF THE MEAN (SEM) - Same as the standard deviation of a distribution means - Also called Standard Error (SE) RULE 3: The shape of a distribution of means is approximately normal if either (a) each sample is of 30- or more individuals; (b) the distribution of the population of individuals is normal. FIGURING EFFECT SIZE 8. MAKING SENSE OF STATISTICAL SIGNIFICANCE (DECISION ERRORS, EFFECT SIZE, AND STATISTICAL POWER) DECISION ERRORS - incorrect conclusions in hypothesis-testing in EFFECT SIZE CONVENTIONS relation to the real (but unknown) situation - standard rules about what to consider a small, medium, and large effect size, based on what is TYPE I ERROR typical in psychology research; also known as (FALSE POSITIVE) Cohen’s conventions - occurs when a researcher rejects a null hypothesis that is actually true - in an experimental research, it means that the researcher concludes that a treatment does have an effect when in fact it has no effect TYPE II ERROR META ANALYSIS (FALSE NEGATIVE) - statistical method for combining effect sizes from - occurs when a researcher fails to reject a null different studies hypothesis that is in fact false - in an experimental research, it means that the hypothesis test has failed to detect a real treatment POWER effect - also called statistical power, is the probability of a statistical test to correctly reject a false null hypothesis - probability that the test will identify a treatment effect if one really exists DETERMINING STATISTICAL POWER POWER TABLES - table for a hypothesis-testing procedure showing the statistical power of a study for various effect sizes and sample sizes EFFECT SIZE - standardized measure of difference (lack of WHAT DETERMINES THE POWER OF A STUDY overlap) between populations - it is intended to provide a measurement of the EFFECT SIZE absolute magnitude of a treatment effect, - Determining Power from The Predicted Effect Sizes independent of the size of the sample(s) being - Predicted µ1 = µ2 + (d)(𝜎) used SAMPLE SIZE Figuring the Sample Size based on Power - Begin with the level of power - Figure how many participants you need to get that level of power using the formula in determining the power. (better use a power table) OTHER INFLUENCES ON POWER 1. Significance Level (a) 2. One-versus Two-tailed Test 3. Type of Hypothesis-Testing Procedure ROLE WHEN PLANNING A STUDY t TEST FOR A SINGLE SAMPLE 1. Increase effect size by increasing the predicted - also known as one sample t test , is a difference between population means. hypothesis-testing procedure in which a sample 2. Increase effect size by decreasing the population mean is being compared to a known population standard deviation. mean and the population variance is unknown 3. Increase the sample size 4. Use a less extreme level of significance 5. Use a one-tailed test. BASIC PRINCIPLE OF THE t TEST 6. Use a more sensitive hypothesis-testing procedure (Estimating the Population Variance from the Sample Scores) BIASED ESTIMATE - estimate of a population parameter that is likely systematically to overestimate or underestimate the true value of the population parameter. (e.g., s2 would be a biased estimate of the population variance (it would systematically underestimate it). UNBIASED ESTIMATE OF THE POPULATION VARIANCE (S2) - estimate of a population variance, based on sample scores, which has been corrected so that it is unlikely to overestimate or underestimate the ROLE OF POWER WHEN INTERPRETING THE RESULTS OF A true population variance STUDY - The correction used is dividing the sum of squared deviations by the sample size minus 1 - Is it statistically significant or practically significant? - Practical Significance - shows that the effect is large enough to be meaningful in the real world. DEGREES OF FREEDOM (df) ROLE OF POWER WHEN A RESULT IS NOT STATISTICALLY SIGNIFICANT - number of scores free to vary when estimating a population parameter - A nonsignificant result from a study with low power - usually part of a formula for making that estimate is truly inconclusive. (e.g. when calculating the estimated population - A nonsignificant result from a study with high power variance from a single sample, the degrees of does suggest either that the research hypothesis is freedom is the number of scores minus 1) false or that there is less of an effect than was predicted when figuring power. ROLE OF POWER WHEN EVALUATING RESULTS OF A STUDY THE STANDARD DEVIATION OF THE DISTRIBUTION OF MEANS (Based on Unbiased Estimate of Population Variance) 9. INTRODUCTION TO T TEST (ONE SAMPLE AND DEPENDENT SAMPLES) t TEST - hypothesis-testing procedure in which the population variance is unknown - it compares t scores from a sample to a comparison distribution called a t distribution ASSUMPTIONS OF THE t TEST FOR SINGLE SAMPLE ESTIMATED STANDARD ERROR (SM) 1. The dependent variable must be continuous - it is used as an estimate of the real standard error (interval/ratio). σM when the value of σ is unknown 2. The observations are independent of one another - it is computed from the sample variance or sample (independence of observation) standard deviation and provides an estimate of the 3. The dependent variable should be approximately standard distance between a sample M and the normally distributed. population µ 4. The dependent variable should not contain any outliers. t DISTRIBUTION Note: To be able to use the One Sample t Test, you should - it is the complete set of t values computed for every have a population mean (µ) possible random sample for a specific sample size (n) or a specific degrees of freedom (df) EFFECT SIZE FOR ONE SAMPLE t TEST MEASURING THE PERCENTAGE VARIANCE EXPLAINED (r2) t TABLE - table of cutoff scores on the t distribution for r2= 0.01 - Small Effect 2 various degrees of freedom, significance levels, and r = 0.09 - Medium Effect one- and two-tailed tests. r2= 0.25 - Large Effect t SCORE CONFIDENCE INTERVALS FOR ESTIMATING μ IN t TEST FOR SINGLE SAMPLE - also known as the t value, is the number of standard deviations from the mean (like Z score, but on a t distribution) Where: t = critical values for a given confidence interval sM = estimated standard error M = sample mean t SCORE 1. State the Null and Alternative Hypothesis REPORTING RESULTS OF A t TEST IN RESEARCH ARTICLES 2. Set a Criteria for Decision 3. Determine the Characteristics of Comparison The participants had an average of M = 46.00 with SD = 4.50 Distribution and Cutoff Sample Score/s. on a standardized alertness test the morning following - Mean of the Sample, Mean of Population is bedtime reading from a light emitting screen. Statistical Known, Degrees of Freedom, Estimated analysis indicates that the mean level of alertness was Population Variance and Standard significantly lower than scores for the general population, Deviation, Estimated Standard Error, t(8) = -2.67, p <.05, d = -0.89, r 2 = 0.47. Critical Value/s in the t Table 4. Collect the data and Compute for the Sample Statistic. t TEST FOR DEPENDENT SAMPLES 5. Make a Decision REPEATED-MEASURES DESIGN - also known as within-subjects design, is a research design that uses the same group of individuals in all of the different treatment conditions 4. Collect the data and Compute for the Sample t TEST FOR DEPENDENT SAMPLES Statistic. - also known as paired samples t test, a 5. Make a Decision hypothesis-testing procedure in which there are two scores for each person and the population ASSUMPTIONS OF THE t TEST FOR DEPENDENT SAMPLES variance is not known - it determines the significance of a hypothesis that 1. The dependent variable must be continuous is being tested using difference or change scores (interval/ratio). from a single group of people 2. Your independent variable should consist of two categorical, "related groups" or "matched pairs". 3. The observations within each treatment condition must be independent. 4. There should be no significant outliers in the differences between the two related groups. 5. The distribution of the differences in the dependent variable between the two related groups should be DIFFERENCE SCORES approximately normally distributed. - difference between a person’s score on one testing and the same person’s score on another testing ASSUMPTIONS - often an after-score minus before-score, in which case it is also called change score - condition, such as a population’s having a normal distribution, required for carrying out a particular hypothesis-testing procedure - a part of the mathematical foundation for the accuracy of the tables used in determining cutoff POPULATION OF DIFFERENCE SCORES values - The mean of the population of the difference scores µD is 0. ROBUSTNESS - extent to which a particular hypothesis testing COHEN’S d AND r2 FOR THE t TEST FOR DEPENDENT procedure is reasonably accurate even when its SAMPLES assumptions are violated - e.g. t test can still produce a valid result even if the normality assumption is not met (robust to normality) CPOWER TABLE FOR t TEST FOR DEPENDENT SAMPLES CONFIDENCE INTERVALS FOR ESTIMATING μ IN t TEST FOR DEPENDENT SAMPLES where: MD = Mean of the difference scores t = critical values for a given confidence interval SMD = estimated standard error for MD SUMMARY FOR HYPOTHESIS-TESTING PROCEDURE FOR t TEST FOR DEPENDENT SAMPLES 1. State the Null and Alternative Hypothesis 2. Set a Criteria for Decision PLANNING A SAMPLE SIZE FOR t TEST FOR DEPENDENT 3. Determine the Characteristics of Comparison SAMPLES (80% STATISTICAL POWER) Distribution and Cutoff Sample Score/s. - Mean of the Difference Scores, Degrees of Freedom, Population Mean is 0, Estimated Standard Error for MD, Critical Value/s in the t Table REPORTING RESULTS OF A t TEST FOR DEPENDENT SAMPLES DISTRIBUTION OF DIFFERENCES BETWEEN MEANS IN RESEARCH ARTICLES - distribution of differences between means of pairs A t Test for dependent samples was used to determine the of samples such that, for each pair of means, one is effects of counseling on anxiety among college students. from one population and the other is from a second After several counseling sessions, the students showed a population change in anxiety scores (M = 4.00, SD = 0.71). Specifically, - the comparison distribution in a t test for the anxiety scores were significantly lower after several independent means counseling sessions, t(4) = -7.76, p <.05, 95% CI [UL,LL]. The effect size is large (d = -3.45) and 94% of the variability in MEAN OF THE DISTRIBUTION OF DIFFERENCES BETWEEN anxiety scores can be explained by counseling. MEANS - The mean of the distribution of differences of WILCOXON SIGNED-RANK TEST means is 0. - the nonparametric test equivalent to the paired t test ESTIMATING THE POPULATION VARIANCE - It does not assume normality in the data, it can be used when this assumption has been violated and POOLED ESTIMATE OF THE POPULATION VARIANCE (s2p) the use of the paired t test is inappropriate - in a t test for independent samples, weighted average of the estimates of the population variance from two samples (each estimate ASSUMPTIONS OF THE WILCOXON SIGNED-RANK TEST weighted by the proportion consisting of sample’s 1. The dependent variable should be measured at the degrees of freedom divided by the total degrees of ordinal or continuous level freedom for both samples) 2. The independent variable should consist of two categorical, “related groups” or “matched pairs”. 3. The distribution is not normal REPORTING RESULTS OF A WILCOXON SIGNED-RANK TEST ESTIMATING THE STANDARD ERROR OF IN RESEARCH ARTICLES M1-M2 (S(M1-M2)) A Wilcoxon’s signed-rank test showed that hypnotherapy significantly reduces anxiety scores (Mdn = 15) compared to pre-therapy (Mdn =22) scores, W = 322, p <.001. 10. T TEST FOR INDEPENDENT THE DEGREES OF FREEDOM FOR t TEST FOR INDEPENDENT SAMPLES SAMPLES BETWEEN-SUBJECTS DESIGN - also known as independent-measures design - is a research design that uses a separate group of participants for each treatment condition (or for EXAMPLE each population) Twenty students were recruited to take part in the study. The 10 students randomly assigned to the expressive writing t TEST FOR INDEPENDENT SAMPLES group wrote about their thoughts and feelings associated with their most traumatic life events. The 10 students - also known as t test for independent means or randomly assigned to the control group wrote about their independent measures t Test plans for the day. One month later, all of the students rated - is a hypothesis-testing procedure in which there their overall level of physical health on a scale from 0 = very are two separate groups of people tested and in poor health to 100 = perfect health. (Although this example is which the population variance is not known based on actual studies, we made up the details we use here to be an easier example to follow for learning. Mainly, actual studies usually have large samples. Real studies on this kind of topic also often use more objective measures of health, such as number of physician visits or days missed from school.) EXPRESSIVE GROUP CONTROL GROUP CONFIDENCE INTERVAL 2 2 M1-M2 = M1-M2 ± 2.101 (4.54) X (X-M1) X (X-M2) LL= 1.46 UL= 20.54 77 4 87 361 REPORTING: 88 81 77 81 A t test for independent samples was used to 77 4 71 9 determine the effect of expressive writing on physical health. Subjects in the expressive writing group (M = 79 , SD = 9.72) 90 121 70 4 showed higher physical health than the subjects in the control group (M = 68, SD = 10.55), and it is statistically 68 121 63 25 significant t(18) = 2.42, p <.05, 95% CI [1.46,20.54]. Furthermore, the effect size is large (d=1.08) and 25% of the 74 25 50 324 variability can be explained by expressive writing. In addition, the effect was large (d = 1.08) and 25% 62 289 58 100 of the portion of variance in physical health can be explained by expressive writing. 93 196 63 25 ASSUMPTIONS OF THE t TEST FOR INDEPENDENT SAMPLES 82 9 76 64 1. The dependent variable should be measured on a 79 0 65 9 continuous scale (interval/ratio). 2. The independent variable should consist of two categorical, independent groups. EFFECT SIZE: 3. There should be an independence of observations, S12 = SS1 / df1 which means that there is no relationship between = 850/9 the observations in each group or between the = 94.44 groups themselves. 4. There should be no significant outliers. S22 = SS2 / df2 5. The dependent variable should be approximately = 1002/9 normally distributed for each group of the = 111.33 independent variable. 6. There needs to be homogeneity of variances ESTIMATING POPULATION VARIANCE Sp2 = (df1 S12 + df2 S22)/ df1 + df2 = (9*94.44)+(9*111.33) / 9+9 MANN-WHITNEY U TEST = (894.96 +1001.97)/18 - used to compare differences between two = 1851.93 / 18 independent groups when the dependent variable = 102. 98 is either ordinal or continuous, but not normally distributed STANDARD ERROR S(M1-M2) = √(sp2 / n1) +(sp2 / n2) = √10.29 + 10.29 ASSUMPTIONS OF THE MANN-WHITNEY U TEST =√20.58 1. The dependent variable should be measured at the = 4.54 ordinal or continuous level. 2. The independent variable should consist of two HYPOTHESIS TESTING categorical, independent groups. 1. Ho = expressive group writing has no significant 3. You should have independence of observations effect on physical health. 4. The two variables are not normally distributed. 2. a= 0.05 3. Critical value: ± 2.101 Reporting in Mann-Whitney U Test: 4. t= (M1 - M2) /S(M1-M2) A Mann-Whitney U Test was used to determine the = (79-68)/4.54 difference between the experimental group (expressive = 2.42 writing) and control group (writing their plans for the day) in 5. Reject the null hypothesis terms of physical health. Based on the gathered data, it suggests that the expressive writing group has significantly EFFECT SIZE AND PROPORTION VARIANCE higher physical health measurement than the control group d= M1 - M2 / √sp2 (U = 21.00, p =.028). = (79-68) /√102.89 = 1.08 (large) r2= t2 / (t2 + df) = 2.422 / [(2.422) + 18] = 0.25 or 25% (large) STEP 7: - calculate the Between-Treatments Variance 11. INTRODUCTION TO ANALYSIS OF (MSbetween) VARIANCE (ANOVA) STEP 8: ANALYSIS OF VARIANCE (ANOVA) - calculate the Within-Treatments Variance (MSwithin) - a hypothesis-testing procedure that is used to evaluate mean differences between two or more STEP 9: treatments/groups/populations - Calculate the F ratio F RATIO MEASURING THE EFFECT SIZE FOR ANOVA - Ratio of the between-groups population variance Percentage of Variance Accounted For (η2) estimate to the within-groups population variance - pronounced as eta squared, a percentage that estimate measures how much of the variability in the scores is accounted for by the differences between F TABLE treatments INTERPRETATION: - Table at cutoff scores on the F distribution - 0.01 = small effect - 0.06 = medium effect BASIC LOGIC OF ANOVA - 0.14 = large effect - The null hypothesis is an anova is that the population being compared all have the same ASSUMPTIONS mean 1. Your dependent variable should be measured at the interval or ratio level (i.e., they are continuous). CARRYING OUT AN ANALYSIS OF VARIANCE (SUM OF 2. Your independent variable should consist of two or SQUARES) more categorical, independent groups. 3. You should have independence of observations, STEP 1: which means that there is no relationship between - Calculate the total sum of squares (SStotal) the observations in each group or between the groups themselves. 4. There should be no significant outliers. Where: 5. Your dependent variable should be approximately X = score of each subject/respondent G = ΣT (T = sum of scores in a group) normally distributed for each category of the N = kn (k = number of groups; n = number of independent variable. Alternatively, the residuals of scores for each group) the dependent variable is approximately normally STEP 2: distributed - Calculate the Within-Treatments Sum of Squares (SSwithin) 6. There needs to be homogeneity of variances. STEP 3: POST HOC COMPARISONS - Calculate the Between-Treatments Sum of Squares - also known as Post Hoc Tests or Posttests, are (SSbetween) additional hypothesis tests that are done after an ANOVA to determine exactly which mean differences are significant and which are not STEP 4: - Calculate the total degrees of freedom (dftotal) TUKEY’S HONESTLY SIGNIFICANT DIFFERENCE (HSD) TEST - or Tukey Test, is a single-step multiple comparison STEP 5: procedure and statistical test. It can be used on raw - Calculate the Within-Treatments Degrees of data or in conjunction with an ANOVA to find means Freedom (dfwithin) that are significantly different from each other - most commonly used for equal sample sizes STEP 6: - Calculate the Between-Treatments Degrees of Freedom (dfbetween) GAMES-HOWELL ANALYSIS OF COVARIANCE (ANCOVA) - a nonparametric approach in comparing - analysis of variance that controls for the effect of combinations of groups or treatments one or more additional variables - it is like the Tukey’s test, but it does not assume - Covariate - variable controlled for in an analysis of equal variances and sample sizes variance SCHEFFE’S TEST MULTIVARIATE ANALYSIS OF VARIANCE (MANOVA) - method of figuring the significance of post hoc - analysis of variance with more than one dependent comparisons that takes into account all possible variable comparisons that could be made1 - It is customarily used for unequal sample sizes2 MULTIVARIATE ANALYSIS OF COVARIANCE (MANCOVA) - analysis of covariance with more than one BONFERRONI PROCEDURE dependent variable - is a multiple-comparison procedure in which the total alpha percentage is divided among the set of comparisons so that each is tested at a more 12. CORRELATION AND PREDICTION stringent significance level CORRELATIONAL DESIGN KRUSKAL-WALLIS H TEST - a nonexperimental quantitative research design - (sometimes also called the "one-way ANOVA on where two different variables are observed to ranks") is a rank based nonparametric test that can determine whether there is a relationship between be used to determine if there are statistically them significant differences between two or more groups of an independent variable on a continuous or ordinal dependent variable CORRELATION - also known as bivariate correlation, is a statistical ASSUMPTIONS OF THE KRUSKAL-WALLIS H TEST technique that is used to measure and describe the relationship 1. The dependent variable should be measured at the ordinal or continuous level. 2. The independent variable should consist of two or SCATTER DIAGRAM more categorical, independent groups. - also known as scatterplot, is a graph showing the 3. You should have independence of observations relationship between two variables 4. The two or more dependent variables are not - the values of one variable are along the horizontal normally distributed. axis and the values of the other variable are along the vertical axis VARIATIONS OF ANOVA - Each score is shown as a dot in this two dimensional space. REPEATED MEASURES ANOVA - an ANOVA for a repeated-measures design--a design with one group of individuals participating in PATTERNS OF CORRELATION three (3) or more treatment conditions LINEAR CORRELATION - Relation between two variables that shows up on a TWO-WAY ANOVA scatter diagram as the dots roughly following a - an ANOVA used for a factorial design--a design straight line with more than one independent variable and one dependent variable MAIN EFFECT - The action of a single independent variable in an experiment; the change in the dependent variable CURVILINEAR CORRELATION produced by the various levels of a single factor - Relation between two variables that shows up on a scatter diagram as dots following a systematic INTERACTION pattern that is not a straight line - the effect of one independent variable changes across the levels of another independent variable; can only be detected in a factorial design NO CORRELATION - No systematic relationship between two variables DIRECTIONS OF CORRELATION POSITIVE CORRELATION - Also known as direct correlation - Is when the two variables tend to change in the same direction - As the value of X variable increases from one individual to another, the Y variable also tends to increase; when the X variable decreases, the Y variable also decreases NEGATIVE CORRELATION - Also known as the inverse correlation - When the two variables tend to go in opposite CORRELATION COEFFICIENT (r) directions - measure of degree of linear correlation between - As the X variable increases, the Y variable two variables ranging from -1 (a perfect negative decreases linear correlation) through 0 (no correlation) to +1 (a perfect positive correlation) STRENGTH OF CORRELATION LOGIC OF FIGURING THE LINEAR CORRELATION CROSS-PRODUCT OF Z SCORES - the result of multiplying a person’s z score on one variable by the person’s z score on another variable PEARSON PRODUCT-MOMENT CORRELATION - also called Pearson’s r, devised by Karl Pearson, is a measure of the strength of a linear association between two variables STEPS IN CONFIGURING THE CORRELATION COEFFICIENT ASSUMPTIONS 1. Change all the scores to z scores 1. Your two variables should be measured at the 2. Figure the cross-product of the z scores for each interval or ratio level (i.e., they are continuous). person 2. Your two continuous variables should be paired. 3. Add up the cross-products of the z scores 3. There should be independence of cases. 4. Divide by the sample size minus 1 (n – 1) 4. There is a linear relationship between your two continuous variables. 5. Both continuous variables should follow a bivariate SIGNIFICANCE OF THE r normal distribution. - To determine the statistical significance of the r, 6. There should be homoscedasticity. convert it into t score and check the critical value 7. No univariate or multivariate outliers. from the t table - Alternatively, you can also use the r table ISSUES INTERPRETING THE r Restriction in Range - also called range truncation or restricted range, is a situation in which you figure a correlation but only a limited range of the possible values on one of the variables is included in the group studied Influence of the Outliers INTERPRETING THE r - presence of an outlier can affect the value of r DIRECTIONS OF CAUSALITY Three possible directions of causality in correlation: 1. X could be causing Y 2. Y could be causing X 3. Some third factor could be causing X and Y SPEARMAN’S RHO (rs) DETERMINING THE EFFECT SIZE - a nonparametric measure of the strength and - 0.10 = Small Effect direction of association that exists between two - 0.30 = Medium Effect variables measured on at least an ordinal scale - 0.50 = Large Effect ASSUMPTIONS OF THE SPEARMAN’S RHO STRENGTH OF CORRELATION 1. The two variables should be measured on an ordinal, interval, or ratio scale 2. The two variables represent paired observations. 3. There is a monotonic relationship between the two variables. KENDALL’S TAU-B (rT) - a nonparametric measure of the strength and direction of association that exists between two variables measured on at least an ordinal scale ASSUMPTIONS OF THE KENDALL’S TAU-B 1. Your two variables should be measured on an COEFFICIENT OF DETERMINATION (r2) ordinal or continuous scale. - also known as proportionate reduction in error, it 2. There is a monotonic relationship between your two measures the proportion of variability in one variables. variable that can be determined from the relationship with the other variable SPEARMAN’S RHO OR KENDALL’S TAU? - “The Spearman and Kendall correlation have a MULTIPLE REGRESSION bounded and smooth influence function, and reasonably small values for the gross-error - an extension of simple linear regression which can sensitivity. The gross-error sensitivity, as well as the be used if we want to predict the value of a variable efficiencies, are depending on the true value of the (i.e., outcome) based on the value of two or more correlation in a nonlinear way. Kendall's correlation other variables (predictors) measure is more robust and slightly more efficient than Spearman’s rank correlation, making it the ASSUMPTIONS OF MULTIPLE REGRESSION preferable estimator from both perspectives.” PARTIAL CORRELATION - the amount of association between two variables, over and above the influence of one or more other variables SIMPLE LINEAR REGRESSION GUIDE FOR REGRESSION DIAGNOSTICS - also called bivariate regression or bivariate prediction, is a statistical technique where the NO SIGNIFICANT OUTLIERS prediction of scores on one variable is based on - Cook’s distance greater than 1 indicate a scores of one other variable multivariate outlier - X -> Y LINEARITY - The assumption of linearity means that the relationship between each predictor and outcome MULTIPLE REGRESSION variable is linear. - Procedure for predicting scores on a criterion - Create a scatterplot using JASP or Jamovi variable from scores on two or more predictor AUTOCORRELATION TEST (Ho: THE RESIDUALS ARE variables INDEPENDENT) - If the p >.05, Fail Reject the Null Hypothesis - If the p <.05, Reject the Null Hypothesis - If the D-W Statistic is near to 2, the residuals are 13. PREDICTION independent. If the D-W Statistic is < 1 or > 3, the residuals are not independent HOMOSCEDASTICITY REGRESSION - If the p >.05 for the Breusch-Pagan Test, Fail Reject - commonly used for predictive analysis such as to the Null Hypothesis predict an outcome variable from one (simple - If the p <.05 for the Breusch-Pagan Test, Reject the regression) or more (multiple regression) predictor Null Hypothesis variables - the next step after correlation REGRESSION DIAGNOSTICS - term that refers to the art of checking that the NORMALITY OF RESIDUALS (Ho: THE DISTRIBUTION IS assumptions of your regression model have been APPROXIMATELY NORMAL) met - If the p >.05 for the Normality Test, Fail Reject the Null Hypothesis SIMPLE LINEAR REGRESSION - If the p <.05 for Normality Test, Reject the Null Hypothesis - used when we want to predict the value of a - Q-Q Plot of Residuals should follow a straight line variable (i.e., outcome) based on the value of MINIMAL OR NO MULTICOLLINEARITY another variable (i.e., predictor) - The VIF should be < 10 for each predictor. The Tolerance should be > 0.1 ASSUMPTIONS OF SIMPLE LINEAR REGRESSION 5. Divide each squared difference by the expected frequency for its category. 14. CHI-SQUARE STATISTICS 6. Add up the results of Step 5 for all the categories. (GOODNESS OF FIT AND INDEPENDENCE) CHI-SQUARE DISTRIBUTION PARAMETRIC TEST - mathematically defined curve used as the - a statistical test that makes an assumption about comparison distribution in chi-square tests the population parameters and the distributions - distribution of the chi-square statistic that the data came from (e.g. normality and DEGREES OF FREEDOM FOR CHI-SQUARE GOODNESS TO FIT homogeneity of variance) TEST: NONPARAMETRIC TEST CHI-SQUARE TABLE - a statistical test that doesn’t assume anything about the population parameters - table of cutoff scores on the chi-square distribution - also called the distribution-free test or rank-order for various degrees of freedom and significance test levels CHI-SQUARE STATISTIC ASSUMPTIONS OF THE CHI-SQUARE GOODNESS OF FIT - also known as chi-square test, 1. One categorical variable (e.g. dichotomous, - is a hypothesis-testing procedure used when the nominal, ordinal) variables of interest are nominal variables 2. Independence of observations 3. The groups of the categorical variable must be mutually exclusive. CHI-SQUARE GOODNESS OF FIT TEST 4. There should be at least 5 expected frequencies in - it uses a sample data to test hypotheses about each group of your categorical variables proportions for a population distribution - it is used to determine how well the obtained CHI-SQUARE TEST OF INDEPENDENCE sample proportions fit the population proportions specified by the null hypothesis - hypothesis testing procedure that examines whether the distribution of frequencies over the categories of one nominal variable is unrelated to the distribution of frequencies over the categories of a second nominal variable OBSERVED FREQUENCY (fo) CONTINGENCY TABLE - in chi-square test, number of individuals actually found in the study to be in a category or cell - two-dimensional chart showing frequencies in each combination of categories of two nominal variables EXPECTED FREQUENCY (fe) - in chi-square test, number of people in a category DETERMINING EXPECTED FREQUENCIES FOR EACH CELL or cell expected if the null hypothesis is true CHI-SQUARE STATISTIC(𝛘2) - statistic that reflects the overall lack of fit between Where: the expected and observed frequencies R = row total - sum of the squared difference between observed C = column total and expected frequencies divided by the n = total number of respondents STEPS IN CONFIGURING (𝛘2) DEGREES OF FREEDOM FOR CHI-SQUARE TEST OF INDEPENDENCE 1. Determine the actual, observed frequencies in each category. 2. Determine the expected frequencies in each category. 3. In each category, take the observed minus Where: expected frequencies. NC = number of columns 4. Square each of these differences. NR = number of rows ASSUMPTIONS OF THE CHI-SQUARE TEST OF INDEPENDENCE 1. Two variables should be measured at an ordinal or nominal level 2. Two variables consist of two or more categorical, independent groups. 3. Less than 20% of the cells should have an expected count/frequency of less than 5 EFFECT SIZE FOR CHI-SQUARE TEST OF INDEPENDENCE PHI COEFFICIENT(φ) - effect-size measure for a Chi-Square Test of Independence with a 2x2 contingency table - Square root of division of chi-square statistic by n - 0.10 = small effect size; 0.30 = medium effect size; 0.50 = large effect size CRAMER’S PHI (Cramer’s ϕ) - measure of effect size for Chi-Square Test of Independence with a contingency table that is larger than 2x2 - also known as Cramer’s V and sometimes ϕC or VC COHEN’S CONVENTION FOR CRAMER’S V