Stat Manual PDF
Document Details
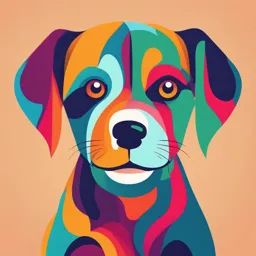
Uploaded by OticFourier
CCS HAU
Tags
Summary
This document is a manual on statistical methods, covering topics such as data classification, tabulation, graphical representations, frequency distributions, and measures of central tendency. It explains concepts like frequency, class limits, class intervals, and various graphical methods. It also discusses different types of classifications and ways to represent data graphically.
Full Transcript
STATISTICAL METHODS (STAT-511) Father of Statistics: R. A Fisher Statistics: According to Fisher ‘The science of statistics is essentially a branch of applied mathematics and may be regard as mathematics applied to observational data. Classification and tabulation of data: The process of arranging t...
STATISTICAL METHODS (STAT-511) Father of Statistics: R. A Fisher Statistics: According to Fisher ‘The science of statistics is essentially a branch of applied mathematics and may be regard as mathematics applied to observational data. Classification and tabulation of data: The process of arranging the items or thing or data in groups or classes according to similarities or dissimilarity is known as classification and the process by which the classified data are presented in an orderly manner by being placed in proper rows and columns of a table in order to bring out their essential features or characteristics is known as tabulation. Objectives of classification: 1. To reduce data in groups/classes according to similarity. 2. To facilitate comparison through statistical analysis. 3. To point out most significant features of the data at a glance. 4. To give importance to a particular item by dropping out the unnecessary elements. 5. To enable a statistical treatment of the material collected. Types of classification: 1. Geographical: When the classification is made on area basis e.g. district. taluka, city, 2. Chronological: When the classification is made on the basis of time e.g. production of wheat in past 10 years. 3. Qualitative: When classification is made on the basis of some attributes. This classification is further divided into four types. (A) Simple classification: Only one attribute is considered e.g. blindness or Gender. (B) Two way classification: Two attributes are considered e.g. blindness & deafness, colour & shape of flowers. (C) Three way classification: Three attributes are considered e.g. Gender, education level and residing location. (D) Manifold classification: More than three attributes are considered. e.g. Classification One way Two way Three way Gender Gender & Gender, Marital Marital status status & Education level High Married Medium Low Male High Unmarried Medium Low Population High Married Medium Low Female High Unmarried Medium Low 4. Quantitative: When the classification is made in the form of magnitude e.g. cows are classified according to milk yield. This classification is further divided into two types. (A) Discrete classification: Any value in the range of variation is considered e.g. length, width etc. (B) Continuous classification: Specific value in the range is considered e.g. no. of petal, no. of insects etc. Graphical Representation: Advantage of Graphical or Diagramatics representation: 1. Diagram give as bird’s-eye view of complex data. 2. They have long lasting impression 3. Easy to understand even by common man 4. They save time and Labour. 5. They facilitate comparison One dimensional diagram or graph Bar diagram and Line Diagram Different Types of Bar diagram are. 1. Simple bar chart 2. Multiple bar chart 3. Sub-divided bar chart Two dimensional diagram or graph Circle, Rectangle, Pie Chart Three Dimensional Diagram 1. Cubes, Cylinder and spheres Classification » Tabulation » Graphical Representation FREQUENCY DISTRIBUTION Objectives: 1. To condense the mass of data in such a manner that similarities and dissimilarities can be easily understand. 2. To enable statistical treatment to the data collected. Frequency: The no. or individual of items occurring in each class is termed as frequency. Frequency distribution: The manner in which the frequencies are distributed over the different class is called frequency distribution of the character under study and the table indicating frequency distribution is called frequency table. Class limit: It is the lowest and highest values of the distribution that can be included in the class e.g 10-20, 20-30 etc. Two boundaries of a class are known as the lower limit and upper limit of a class. Class interval: The width of a class that is the difference of upper and lower limit of the class is known as class interval. Class mid point: It is the value lying half way between the lower limit (LL) and upper limit (UL) of a class interval i.e. (LL + UL)/2. GRAPHICAL REPRESENTATION Graphical representation is used when we have to represent the data of a frequency distribution and of a time series. It is represented by points which are plotted on a graph paper. Advantages of graphical representation 1. Easy to understand and interpret data at a glance. 2. It facilitates comparisons. 3. It gives eye view of complex data. 4. It has long lasting impression. 5. It gives an attractive and interesting view. Limitations of graphical representation 1. It cannot show all those facts which are there in the tables. 2. It shows tendency and fluctuations, actual values are not known. 3. The charts take more time to be drawn than the tables. Graphs of Frequency Distribution Histogram: It is a bar diagram which is suitable for frequency distributions with continuous classes. The width of all bars is equal to class interval and heights of the bars are in proportion to the frequencies of the respective classes. In this diagram bars touch each other but one bar never overlaps the other. Frequency polygon: When the mid points of the tops of the adjacent bars of a histogram are joined in order by a straight line, then the graph of lines so obtained is called a frequency polygon. Frequency curve: A frequency curve is a graphical representation of frequencies corresponding to their variates values by a smooth curve. A smoothened frequency polygon represents a frequency curve. Ogive or Cumulative frequency curve: it is a graph plotted for the variates values and their corresponding cumulative frequencies and joined by a free hand smooth curve. The curve is ‘S’ shaped. There are two methods of constructing ogive viz. (i) “less than” method and (ii) “more than” method. In the “less than” method, we start with the upper limit of classes and go on adding the frequencies however, in the “more than” methods, we start with the lower limit of classes. The first method gives a rising curve whereas second method shows a declining curve. Pie chart A pie chart is a circular diagram which is usually used for depicting the computation of a single factor. The circle is divided into segment which are in proportion to the size of the components. They are show by the different pattern or colour to make them attractive. Sales 0THER USA 25% 34% PEPT COUNTRY 17% JAPAN 13% APEC USSR 5% 6% Fig : India’s import from various sources **Procedure to form frequency distribution: Step 1: Find range of the data. Range = Highest value – Lowest value. Step 2: Fix the number of classes. Number of classes should preferably between 5 to 15 and should not be less than 5 and more than 30. Approximate no. of classes = K =1 + 3.322 log N (Sturge’s rule) where N = no. of observations under study. Step 3: Fix the class interval = CI = Range/No. of classes or (L-S)/K where L = largest value and S = smallest value Step 4: Arrange different classes in ascending order of magnitude Step 5: Pick up the values of observation and make tally mark against respective classes. Step 6: Find total tally mark of each class which will give the no. of frequencies in the respective classes. box plot A box plot is a graphical rendition of statistical data based on the minimum, first quartile, median, third quartile, and maximum. The term "box plot" comes from the fact that the graph looks like a rectangle with lines extending from the top and bottom. Because of the extending lines, this type of graph is sometimes called a box-and-whisker plot. In a typical box plot, the top of the rectangle indicates the third quartile, a horizontal line near the middle of the rectangle indicates the median, and the bottom of the rectangle indicates the first quartile. A vertical line extends from the top of the rectangle to indicate the maximum value, and another vertical line extends from the bottom of the rectangle to indicate the minimum value. The illustration shows a generic example of a box plot with the maximum, third quartile, median, first quartile, and minimum values labeled. The relative vertical spacing between the labels reflects the values of the variable in proportion. First Quartile and Third Quartile Definitions: The lower half of a data set is the set of all values that are to the left of the median value when the data has been put into increasing order. The upper half of a data set is the set of all values that are to the right of the median value when the data has been put into increasing order. The first quartile, denoted by Q1 , is the median of the lower half of the data set. This means that about 25% of the numbers in the data set lie below Q1 and about 75% lie above Q1. The third quartile, denoted by Q3 , is the median of the upper half of the data set. This means that about 75% of the numbers in the data set lie below Q3 and about 25% lie above Q3. Example 1: Find the first and third quartiles of the data set {3, 7, 8, 5, 12, 14, 21, 13, 18}. First, we write data in increasing order: 3, 5, 7, 8, 12, 13, 14, 18, 21. As on the previous page, the median is 12. Therefore, the lower half of the data is: {3, 5, 7, 8}. The first quartile, Q1, is the median of {3, 5, 7, 8}. Since there is an even number of values, we need the mean of the middle two values to find the first quartile:. Similarly, the upper half of the data is: {13, 14, 18, 21}, so. Introduction MEASURES OF CENTRAL TENDENCY Different groups of data or statistical series or frequency distributions differ in four characteristics. 1) Central tendency or location 3) Skewness or symmetry 2) Dispersion or variation 4) Kurtosis or peaked ness Central tendency: A values of the variable tend to cluster around a central value or centrally located observation of the distribution. This characteristic is known as central tendency. This centrally located value which represents the group of values is termed as the measure of central tendency e.g. an average is called measure of central tendency. Objectives 1) To get one single value that describes the characteristics of the entire series/group. 2) To compare two or more distributions. Requisite/Characteristics of ideal measures of central tendency Since an average is a single value representing a group of values, it is expected that such a value should satisfied the following properties. 1) It should be rigidly defined. 2) It should be based on all the observations. 3) It should be easy to understand or comprehensible, otherwise its use will be limited 4) It should be easy to calculate. 5) It should be amenable to further mathematical treatment. 6) It should be least affected by fluctuation of sampling. 7) It should be least affected by the extreme values. Different measures of Central tendency 1) Arithmetic mean (A.M.) Algebraic average 2) Median Positional average 3) Mode 4) Geometric mean (GM) 5) Harmonic mean (H.M.) Algebraic average 6) Weighted mean (W.M.) (1) Arithmetic mean or Mean It is the most common and ideal measure of central tendency. It is defined as the sum of the observed values of the character (or variable) divided by the number of observations considered in obtained sum (total). _ Symbolically X: Sample mean : Population mean n X i X i1 n Sum Xi 3 4 5 4 3 5 4 28 Xi-Mean -1 0 1 0 -1 1 0 0 (Xi-5) -2 -1 0 -1 -2 0 -1 -7 (Xi-Mean)2 1 0 1 0 1 1 0 4 (X-5)2 4 1 0 1 4 0 1 11 x 3 4 5 4 3 5 4 Product=14400 log x 0.477 0.602 0.698 0.602 0.4771 0.698 0.602 Sum=4.158 1/x 0.333 0.25 0.2 0.25 0.333 0.2 0.25 Sum=1.8166 (3 4 ..... 4) 28 Mean ( X ) = 4 7 7 GM= (3x4……x4)1/7 = (14400)1/7 = 3.926 or n log xi Anti log( i 1 log 3 log 4...... log 4 GM= = Anti log( n n 0.477 0.602...... 0.602 Anti log Anti log(0.594) 3.926 7 (0.33 0.25........ 0.25) 1.816 1/HM= 0.2595 7 7 1 HM= 3.853 0.2595 AM˃GM˃HM Properties of Arithmetic mean 1) The algebraic sum of the deviations of a set of values (observed values) from their arithmetic mean is zero. 2) The sum of squares of the deviations of a set of values from their arithmetic mean is always minimum 3) Amenability of arithmetic mean to further mathematical calculation. _ _ (a) Let X1 be the mean of n1 observations, X2 be the mean of n2 observations, Xk be the mean of nk observations then the combined mean of N observations is given by (where N = n1 + n2 +...,+nk ) n1 X 1 n 2 X 2 ...... n k X k X n1 n 2 ...... n k This is also called weighted mean (W.M.) (b) Adding or subtracting a constant from each observation of a given series will add or subtract the same constant to the arithmetic mean. (c) Multiplying or diving each observation by a constant will multiply or divide the arithmetic mean by the same constant. Merits and demerits of Arithmetic mean Merits 1) It is rigidly defined. 2) It is based on all the observations. 3) It is readily comprehensible. 4) It is easy to calculate. 5) Its algebraic (Mathematical) treatment is especially easy and definitely possible. 6) It is also least affected by the fluctuation of sampling. Demerits 1) It is affected by the extreme values. 2) If there is large variation in the data then A.M. becomes some times meaning less. 3) It is not used to measure rate of growth or rate of speed directly. Uses: It is most popular and simple estimate and used widely in almost all the fields of studies such as social science, economics, business, agriculture, medical sciences, engineering and such other sciences. Weighted Mean When different observations are to be given different weights, arithmetic mean does not prove to be a good measure of central tendency. In such cases weighted mean is to be calculated. If X1, X2, X3,... Xn are different observation and W 1, W 2, W 3,... W n are their respective weights then, W.M. W1 X 1 W 2 X 2 ...... Wn X n = W X i i W1 W 2 ...... Wn W i Merits and demerits of Weighted mean (W. M.) W.M. is the A.M., hence merits and demerits are the same as there for the arithmetic mean. Uses 1) Used when the number of individuals in different classes of grouped widely varying. 2) Used when the importance of all the items in a series is not same. 3) Used when the ratios, percentages or rates e.g. rupees per kilogram, rupees per meter etc. are to be averaged. 4) Weighted mean is particularly used in calculating birth rates, death rates, index numbers, average yield etc. Geometric Mean AM gives equal weightage to all the items and has got a tendency towards the higher values. Sometimes it is necessary to get average having a tendency towards the lower values. In such case, geometric mean is helpful. It is defined as the n th root of the product of n items of a series with following relation. It is useful in ratio and Proportion data Raw data or ungrouped data n log X i GM X 1 , X 2,.....X n = X 1 , X 2 ,.....X n 1 n n = Antilog ( i 1 ) n Merits and demerits of Geometric mean Merits 1) It is rigidly defined. 2) It is based on all the observations. 3) It is not much affected by the fluctuation of sampling. 4) It gives less weightage to large items and more to small items. 5) It is suitable for averaging ratios, average rate of change, index nos. Demerits 1) It is difficult to understand. 2) It cannot be calculated when there are negative values. 3) If any item of the series is zero, it would be also zero. Harmonic Mean HM is the reciprocal of the arithmetic mean of the reciprocal of the values of a variable or series. Raw data or ungrouped data n n HM 1 1 1 1 ..... x x1 x 2 xn i Merits and demerits of Harmonic mean Merits 1) It is rigidly defined. 2) It is based on all the observations. 3) It is not much affected by the fluctuation of sampling. 4) It gives greater weightage to smaller values. 5) It is useful in average price, speed, time, distance, quantity etc. Demerits 1) It is not easy to calculate and understand. 2) It cannot be calculated if any value is zero or negative. 3) It gives large weightage to smaller values. Uses: Time series data, units purchased per rupee, kilometers covered per hour, problems solved per time. Relation between AM, GM and HM: 1) AM > GM > HM 2) GM= AM HM Median: The median is the middle most items that divide the series into two equal parts when ith items are arranged in ascending or descending order. th n 1 In case of raw data, the median is the term where n (odd) is the total no. of 2 observations whereas in case of n even number medial is the average between th th n n and 1 terms, in case of grouped data it is given by the formula, 2 2 Uses: It is useful when the extreme values of the series are either not available or impossible to be obtained or abnormal. When in a group, the individual is denoted by better than half the individual’s, median is used. It is also useful when the items are not susceptible to measurement in definite units e.g. intelligence, ability, efficiency etc. Median is use full when the data are affected by outlier and more highly skewness. Mode: The value of the variable which occurs most frequently or whose frequency is maximum is known as mode. Uses: Business forecasting is particularly based on the modal values. Meteorological forecasting is also based on modal values. Relation between Mean, Median and Mode: Mode = 3Median – 2Mean (In normal distribution) 4. MEASURES OF DISPERSION Mean is though an important concept in statistics, it does not give a clear picture as to how the different observations are distributed in a given distribution or the series under study. Consider the following series. Series Observations Mean 1 2, 3, 4, 7 4 2 4, 4, 4, 4 4 3 1, 1, 2, 12 4 4 3, 4, 4, 5 4 In the above series, the mean is same i.e. 4 but the spread of the observations about the mean is in different manner. Hence after locating the measures of central tendency, the next point is to find out the center. This can be done by measuring the spread. The spread is also called a Scatter, Variation OR dispersion of the variate values. Definition Dispersion may be defined as the extend of the scatterness of observations around a measure of central tendency and a measure of such scatter is called measures of dispersion. Different measures of dispersion 1) Range. 2) Absolute mean deviation or Absolute deviation (A.M.D.) 3) Standard deviation (S) 4) Variance (S2) 5) Standard error of mean (S.Em.) 6) Coefficient of variation (C.V. %) Requisite/Characteristics of an ideal measure of dispersion Measures of dispersion should possess all those characteristics which are considered essential for measures of central tendency viz. 1) It should be based on all observations. 2) It should be readily comprehensible. 3) It should be fairly easily calculated. 4) It should be simple to understand. 5) It should not be affected by sampling fluctuations. 6) It should be amenable to algebraic treatment. Standard deviation (S) The standard deviation or "root of mean square deviation" is the most common and efficient estimator used in statistics. It is based on deviation from arithmetic mean and is denoted by S or . S = Standard deviation for sample. = Standard deviation for population. Definition "It is a square root of a ratio of sum of square of deviation calculated from arithmetic mean to the total number of observations minus one”. Method of computation Raw data or ungrouped data (1) Deviation method n (X i X) 2 S i 1 n 1 (2) Variable square method n n ( X i ) 2 X 2 i i 1 n S i 1 n 1 where: Xi = Variate value n = No. of observations (3) Assumed mean method n n ( d i ) 2 d 2 i i 1 n S i 1 di = Xi – A A = Assumed mean n 1 Grouped data or frequency distribution (1) Deviation method k f (X i i X) 2 k S i 1 where n f i ; fi= Frequency of ith class n 1 i 1 (2) Variable square method k k ( f i X i ) 2 f X i 2 i i 1 n S i 1 n 1 (3) Assumed mean method k k ( f i d i ) 2 f d i 2 i i 1 n S i 1 di = (Xi – A) n 1 (4) Step deviation method k k ( f i d Xi ) 2 f d i 2 Xi i 1 n S i 1 dxi = (Xi - A)/I n 1 Properties of Standard deviation (1) Combined standard deviation can be calculated using following formula when two series are given under study. It is symbolically denoted by S 12. N1S12 N 2 S 22 N1d12 N 2 d 22 S12 = N1 N 2 where, S12 = Combined standard deviation; S1 = Standard deviation of first group; S2 = Standard deviation of second group; d1 = ( X1 - X12 ) ; d2 = ( X 2 - X12 ) ; N1 and N2 are the numbers of observation for series one and two; X1 = A.M. for first series; X 2 = A.M. for second series; X12 = weighted or combined mean. (2) The sum of squares of the deviations of items in the series from their arithmetic mean is minimum. This is the reason why standard deviation is always computed from the arithmetic mean. (3) Addition or subtraction of a constant from the grouped of an observation will not change the value of S.D. (4) Multiplying or dividing each observation of a given series by a constant value will multiply or divide the std. deviation by the same constant. Variance: Variance is the square of standard deviation. It is also called the “Mean square deviation". It is being used very extensively in analysis of variance of results from field experiment. Symbolically denoted by S2 = Sample variance and 2 = Population variance. Method of computation Raw data or ungrouped data (1) Deviation method n (X i - X )2 S2 = i 1 ( n 1) (2) Variable square method X X i / n 2 2 2 i S.S. S = n 1 d. f. where : Xi = Variate value S S = Sum of square n = No. of observations. df = Degrees of freedom (3) Assumed mean method d d i / n 2 2 2 i S n 1 where di = Xi - A A = Assumed mean Grouped data or frequency distribution (1) Deviation method k f (X i i - X )2 S2 = i 1 (n 1) (2) Variable square method f X f i X i / n 2 2 2 i i S n 1 (3) Assumed mean method : fd f i d i / n 2 2 2 i i S where, di = (Xi - A) n 1 A = Assumed mean fI = Frequency of ith class (4) Step deviation method : f dx f dx 2 2 2 i i i i /n S xI 2 dxi = (Xi - A)/I n 1 Properties of variance 1) If V(x) represent the variance of X series and V (y) represent the variance of Y series then V (xy) = V(x) + V(y) i.e. V(x+y) = V(x) + V (y) and V(x-y) = V(x) + V(y) 2) Multiplying or dividing each observation by a constant will multiply or divide the variance by square of that constant. e.g. V(ax) = a2 V(x). 3) Addition or subtraction a constant from the groups of each observation will not change the value of variance. Standard error of mean ( S.Em.) The standard deviation is the standard error of a single variate where as standard error of mean is the standard deviation of sampling distribution of the sample mean OR it refers to the average magnitude of difference between the sample estimate and population parameter taken over all possible samples from the population. Definition : It is defined as square root of the ratio of the variance to the total no. of observations in a given set of data. Symbolically, it is written as S X for sample and X for population. S SX where, S = Standard deviation; n = No. of observations n For statistical analysis work, the use of S X is common. It is also used to provide confidence limit on population mean and for test of significance. Coefficient of variation (C.V. %) It is a relative measure of variation and widely used to compare two or more statistical series. The statistical series may differ from one another with respect to their mean or standard deviation or both. Some times they may also differ with respect to their units and then their comparison is not possible. To have a comparable idea about the variability present in them, C.V. % is used. It was developed by Karl Pearson". Definition : "It is a percentage ratio of standard deviation to the arithmetic mean of a given series". It is without unit or unit less. S CV% x100 X The series for which the C.V.% is greater is said to be more variable or we say less consistence, less homogeneous or less stable while the series having lower C.V.% is called more consistence or more homogeneous. WHAT IS EXPLORATORY DATA ANALYSIS (EDA)? Unlike hypothesis-driven data analyses which are motivated by a question, exploratory data analyses are not driven by a specific question. Exploratory data analyses search for patterns and relationships within data. At the easier levels an exploratory data analysis might reveal that the variable you are interested in is increasing or decreasing with the passage of time. Further exploratory data analysis might yield a basic description of how the pattern of this variable is distributed across geographic space or how it is related to another variable. Exploratory data analyses are typically used in the early stages of a study when you are trying to get a basic understanding of the data. The insights you achieve from such an analysis might lead you to formulate questions appropriate for a hypothesis-driven data analysis. A typical project might begin by simply looking at the data values, proceed to mapping the data, then conduct some basic pattern description exploratory data analysis, and finally progress to a more sophisticated hypothesis-driven analysis. EDA employed variety of technique to 1. Maximize insight into as data set 2. Overcome underlying structure 3. Extract important variables 4. Test underlying assumptions 5. Develop parsimonious models 6. And determine optimal factor selling Most EDA techniques are graphical in nature with a few quantitative techniques. Te particular graphical techniques employed in EDA are often quite simple, consisting of various technique of Plotting by row data (such as data traces, histograms, bihistograms, stem and leaf display, probability plot, lag plots, block plots, Youden plots, scatter plots, character plot, residual plots. Plotting simple statistics such as mean plots, standard deviation plots, box plot, and main effect plots of the raw data. Positioning such plot so as to maximize our natural pattern-recognition ability, such as using multiple plots per page. For classical analysis, the sequences is Problem » Data » Model » Analysis » Conclusion For EDA, the sequence is Problem » Data » Analysis » Model » Conclusion Graphical procedures are not just tools that we could use in an EDA context, they are tool that we must use. Such graphical tools are the shortest path to gain insight into a set in term of Testing assumption Model selection Model validation estimator selection Relationship identification Factor effect determination Outlier detection 35 y = 1.456x + 12.11 30 R² = 0.486 25 20 Series1 15 Linear (Series1) 10 5 0 0 5 10 15 Linear model 35 30 y = -0.417x2 + 7.295x - 2.482 R² = 0.926 25 20 Series1 15 Poly. (Series1) 10 5 0 0 5 10 15 5. P R O B A B I L I T Y Statistics concerns itself with inductive reasoning/inference based on the mathematics of probability. Sampling variation needs support in terms of probability / reliability of inference. Introduction: In the study of a population, one can not make any firm statements about the population concerned or its parameters, when only a sample investigation of the population is available for scrutiny. Due to the sampling variation there is doubt about sample investigation and hence it is practice to make statements of less definite nature in terms of probability or chance. The probability or chance for any statement depends on the number of favourable, unfavourable and total possible cases. e.g. in a tossing a coin for getting a head, one has to consider that there are two equally likely cases, head and tail, one is in favour of the statement and the other is against it. The theory of probability aims to generalize the laws of chance, to discover the regularities in the pattern in which events, depending on chance, repeat themselves. It may be the tossing of a coin, a game of cards or the genetical ratios which may be the object of our investigation. Jacob Bernoullis, an Italian mathematician was the first to give concept and definition of probability in 1713. The work of Gregor Mendel in Genetics showed that the theory of probability could be applied to biological investigations. Definition: Probability is a ratio of the number of “favourable” cases to the total number of equally likely cases. If probability is denoted by P then Number of favourable cases P Total number of equally likely cases Suppose a coin is tossed, the possible outcomes (events) are head and tail. These are equally likely and mutually exclusive events. The probability (P) of event head is 1/2. Number of favourable event(s) 1 i. e. P(Head) Total no. of events 2 If n is the number of equally likely and mutually exclusive events for an event A, of which m is the favourable to its occurrence, then the probability of A is the fraction m/n." P (A) = m/n This is “A PRIORI" or “CLASSICAL PROBABILITY” determined before trials are made actually. The probability is arrived at by examination of the nature of the event rather than from the results of experiment. Here frequencies of the events are known and exact. The estimation of "a priori" probability is logical. It may mislead, sometimes it fails to answer questions like; what is the probability that male die before the age of 60? What is the probability of rainfall on 15th August? One may reply as 1/2, which is not correct. One has to study here favourable events, the frequency of occurrence of rainfall on 15th August through past records. What is the probability of a student passing in an examination? Here we have two equally likely cases, passing or failing. The probability of passing the examination would be 1/2, then we might be ignoring the facts, the student might be a first class student who has studied well. In such cases the probability of passing the examination would be nearly 1 and not 1/2. Thus, in many agricultural problems, it may not be possible logically to define equally likely events, which may happen, before trials are made. In such situation, the probability is estimated from a set of observations, which is called “A POSTERIORI” or “EMPIRICAL PROBABILITY”. Such estimate is based on large number of observations. “a posteriori" probability is determined after the trial made i.e. the event has already occurred. Posteriori probability: In this case, the probability is determined after the event has already occurred. The post-facto analysis of the event occurred just to understand the probability and its application to the problem. Random Experiment: A happening with two or more outcomes is called an experiment. If the outcomes are associated with uncertainties, the experiment is called random. Trial and event: An experiment which repeated under essentially identical conditions, possible out comes is called events and experiment is known as trial. (Any out come or results of an experiment is termed as event) e.g. throwing a die is a trial and getting 1 or 2 … is an event. Simple event: The occurrence of a single event is known as simple event. Compound events: The occurrence of two or more in connection with each other, the joint occurrence is called the compound events. Exhaustive events: All possible out comes of any trial / experiment are known as exhaustive events. e.g. (i) tossing a coin there are two exhaustive events viz. head and tail. (Possibility of the coin standing on an edge being ignored) (ii) throwing of a die, there are six exhaustive events. Mutually exclusive events: Events are said to be mutually exclusive if happening of any one of them precludes the happening of other OR two events are said to be mutually exclusive when both can not happen simultaneously in a single trial. Independent events: Events are said to be independent if occurrence of any event is not affected by the occurrence the remaining events e.g. in tossing an unbiased coin event of getting head in the first toss is independent of getting a head in second, third and subsequent throws. Dependent events: Dependent events are those in which the occurrence or non- occurrence of one event in anyone trial affects the probability of other events in other trial. Equally likely events: Events are said to be equally likely when one does not occur more often than the others e.g. in throwing a die, all the six faces are equally likely to come. Law of addition: A: When events are mutually exclusive If two events A and B are mutually exclusive with probabilities P1 and P2 respectively, then the probability of occurrence of either of them (A or B) is equal to the sum of the individual probabilities (A and B). In symbols P(A or B) = P (A) + P(B) = P1 + P2 B: When events are not mutually exclusive If A and B are not mutually exclusive events, then the probability of either of them is equal to the sum of their probabilities less the probability of their simultaneous occurrence. Symbolically P(A or B) = P(A) + P(B) - P(AB) where P(AB) is the probability of joint occurrence of A and B Example: This will serve the proof also. If A is the event “drawing an ace” from a pack of cards, and B is the event “drawing a spade card”; then A and B are not mutually exclusive events, since the ace of spade can be drawn. Thus the probability of drawing either ace or a spade or both is P(ace or spade) = P(ace) + P(spade) – P(ace of spade) = 4/52 + 13/52 - 1/52 = 16/52 Similarly we can generalize the rule for more than two events also. i.e. P(A + B + C) = P(A) + P(B) + P(C) – P(AB) - P(AC) – P(BC) + P(ABC) Law of multiplication: Independent and dependent events: Events are said to be dependent or independent accordingly as the occurrence of one does or does not affect the occurrence of the others. Two events, drawing of a king and queen will be independent if the drawing of the card is replaced after the first draw but if the card after first draw is not replaced and another card is drawn for the second event, the probability of occurrence of the second event will depend on the probability of the occurrence of the first. Hence in the latter case the second event will be dependent on the first. Rule (iii) A: When events are independent If A and B are two independent events, with individual probabilities P1 and P2 respectively, then the probability of both happening at a time is the product of their respective probability (P1.P2) i.e. P(AB) = P(A). P(B) = Pl. P2 B: When events are dependent If two events A and B are dependent then the probability of both happening at a time is given as follows: P(AB) = P(A). P (B/A) This is the conditional probability or = P(B). P(A/B) Where P(B/A) means the probability of second event B dependent on the probability of first event A. In above, if P(B/A) = P(B) then A and B are independent events. Example: Suppose a box contains 3 white balls and 2 black balls. Let A be the event “first ball drawn is black” and B the event “second ball drawn is black”, where the balls are not replaced after being drawn. Here A and B are dependent events. 2 2 P(A) probability of drawing first black ball. 32 5 1 1 P(B) P(B/A ) the probability of second black ball given the first ball 3 1 4 drawn is black Then P(A.B) = P(both black) = 2/5. 1/4 = 2/20 = 1/10 Similarly we can generalize the rule for more than one dependent event. P(A.B.C) = P(A).P(B/A).P(C/AB) 6. PROBABILITY DISTRIBUTION It is also called parent population distribution, theoretical distributions or theoretical frequency distribution. In previous chapter, the probability of the occurrence of a single event is obtained. In scientific research using statistical methodology, it is often required to obtain the probabilities of occurrence of all possible events. A table of the possible values (Xi) which a chance event may assume with a corresponding probability distribution for each value is called a probability distribution for the population. Following table gives the probability distribution of sum of two unbiased dice. Table: Probability distribution of sum of two dice. _________________________________________________________ Xi 2 3 4 5 6 7 8 9 10 11 12 _________________________________________________________ fi 1 2 3 4 5 6 5 4 3 2 1 _________________________________________________________ pi 1/36 2/36 3/36 4/36 5/36 6/36 5/36 4/36 3/36 2/36 1/36 _________________________________________________________ k pi = 1 pi = f(x) = f(xi) i=1 Instead of a table of values such as above, one can represent the outcomes (pi) by proper mathematical function over a range of X i. In this chapter we would like to describe three theoretical distribution (i) Binomial distribution - James Bernoulli (1700) (ii) Poisson distribution - S.D. Poisson (1857) and (iii) Normal distribution – De moivre (1733). BINOMIAL DISTRIBUTION Binomial distribution was discovered by James Bernoulli in 1713. This is very important distribution dealing with discrete variable. The binomial distribution has two parameters viz. n and p. In other words, it is completely determined by the values of n and p. Let a random experiment be performed repeatedly and let the occurrence of an event in any trial be called a success and its non-occurrence a failure. Consider a series of n independent Burnoullian traials(n being finite, in which the probability ‘p’ of success in any trial is constant for each trial. Then q=1-p is the probability of failure in any trial. The probability of x successes and consequently (n-x) failures in n independent trials, in a specified order (say) SSFSFFFS ….. FSF (where S represent success and F failure) is given by the compound probability theorem by the expression: P(SSFSFFFS ….. FSF)=P(S)P(S)P(F)P(S)P(F)P(F)P(F)P(S)…..P(F) P(S)P(F) =p.p.q.p.q.q.q.p…..q.p.q = p.p…..p… …...q.q.q…….q x factors (n-x) factors But successes in n trials can occur in nCx ways and probability for each of these ways is px qn-x. Hence the probability of x successes in n trials in any order whatsoever is given by the addition theorem of probability by the expression: p(X=x)= p(x)= nCx px qn-x Where p(x) denotes the probability of getting exactly x successes. Binomioal Distribution. It sate that, the experiment of n trial having constant p probability of success, then the probability of x success out of the n trial is given by n P(X=x) = cx P x q n x , where q is the probability of failure i.e p+q+2 Properties of Binomial distribution (1) The shape of the distribution depends on the values of q and p. If p = q, the shape if it is symmetrical. If p = q the shape of it is, asymmetrical but the asymmetry decreases as n increases. (2) Arithmetic mean = np (3) Standard deviation = npq (4) Variance = npq Conditions for using Binomial distribution (1) The outcome or results of each trial in the process are characterized as one of two types of possible outcomes. (2) The possibility of outcome of any trial does not change and is independence of the results of previous trials. Use It is useful in describing an enormous variety of real life events. POISSON DISTRIBUTION The Poisson distribution is the limiting form of the binomial probability distributions n become infinitely large and p approaches 0 in such a way that np = m remained constant. Such situation are fairly common. That is to say, a Poisson distribution may be expected in cases were the chance of any individual event being a success is small. e.g. occurrence of comparatively rare event, such as serious floods, percentage infestation of any diseases etc. Like binomial distribution, the variate of the Poisson distribution is also a discrete one. The probability functions is e-m mx P(x) = --------- x! Where P(x) represents the number of successes m represents the average number of successes (m = np) e is a constant (e = 2.7183) Properties of Poisson distribution (1) Arithmetic mean =m (2) Variance =m (3) Standard deviation = m (4) Central moment value : (1) First moment = 1 = 0 (2) Second moment = 2 = m (3) Third moment = 3 = m2 (4) Fourth moment = 4 = m+3m2 Use Poisson distribution is used in practice in wide variety of problems where there are infrequently occurring events with respect to time, area, volume or similar unit. For example it is used in quality control statistics to count the number of defects of an item, or in biology to count number of bacteria, insects etc. Following are some instances where poison distribution are successfully employed: 1. Number of suicide reported in a particular city. 2. No of printing mistake in a book 3. The number of infested plants in a plot 4. The number of insects caught in traps 5. Number of weeds per plot 6. Parthenocarpy in some varieties of mango Q. If the mean and variance of binomial distribution are 4 and 4/3 respectively. Fine the parameter (or p and n) of the distribution. Ans: n=6 and p=2/3 8. CORRELATION ANALYSIS So far we have studied problems relating to one variable only. In practice we come across a large number of problems involving the use of two or more than two variables. Univariate population A population that is characterized by a single variable is termed as univariate population e.g. population of height of students, weight, yield etc. Bivariate population When two variables are simultaneously studied in a single population is termed as bivariate population e.g. the height and weight of the students, rainfall and yield, the amount of fertilizer used and the crop yield. If two quantities vary in such a way that movement in one are accompanied by movements in the other, these quantities are said to be correlated e.g. price of commodities and amount demanded, increase in rainfall up to a point and production of crop. The degree of relationship between the variables under consideration is measured through the correlation analysis. Correlation It indicates the association between the two or more variables in a bivariate distribution or an analysis of the covariation of two or more variables is usually called correlation. Types of correlation Correlation is described or classified in several different ways. Three of the most important ways of classifying correlation are: i) Positive or negative ii) Simple, partial and multiple iii) Linear and non-linear Positive and negative correlation Whether correlation is positive or negative would depend upon the direction of change of the variable. If both the variables are varying in the same direction i.e. if as one variable is increasing the other on an average is also increasing, correlation is said to be positive. Eg. Height and weight, Plant nutrient available and yield, Number of tiller and yield, income and expenditure, Demand and price, etc.If, on the other hand the variable is varying in opposite directions, i.e. as one variable is increasing the other is decreasing or vice-versa, correlation is said to be negative. Eg. Yield and disease incidence, Supply and price, etc Positive correlation X: 10 12 15 18 20 X: 80 70 60 40 30 Y: 15 20 22 25 37 Y: 50 45 30 20 10 Negative correlation X: 20 30 40 60 80 X: 100 90 60 40 30 Y: 40 30 22 15 10 Y: 10 20 30 40 50 Simple, Partial and Multiple correlation When only two variables are studied it is a problem of simple correlation. When three or more variables are studied it is a problem of either multiple or partial correlation. In multiple correlation, three or more variables are studied simultaneously. In partial correlation, we recognize more than two variables, but consider only two variables to be influencing each other, the effect of other influencing variables being kept constant. Linear and Non-linear (curvilinear) correlation If the amount of change in one variable tends to bear a constant ratio to the amount of change in the other variable than the correlation is said to be linear e.g. X: 10, 20, 30, 40, 50 Y: 70,140, 210, 280, 350 Correlation would be called non-linear if the amount of change in one variable does not bear a constant ratio to the amount of change in the other variable. Methods of studying correlation There are four major approaches of ascertaining whether two variables are correlated or not: 1. Scatter diagram method 2. Graphic method 3. Algebraic method: Karl Pearson’s coefficient of correlation 4. Rank method 1. Scatter diagram method The simplest device for deciding whether two variables are related or not is to prepare a dot chart called scatter diagram. When this method is used the given data are plotted on a graph paper in the form of dots i.e. for each pair of X and Y values we put a dot and thus obtain as many points as the number of observations. By looking to this scatter of the various points we can form an idea as to whether the variables are related or not. The greater the scatter of the plotted points on the chart, the lesser is the relationship between two variables. The more closely the points come to a straight line, the higher the degree of relationship. If all the points lie on a straight line falling from the lower left hand corner to the upper right hand corner, correlation is said to be perfectly positive e.g. volume and weight of metal. r = 1 If all the points are lying on a straight line rising from the upper left hand corner to the lower right hand corner of the diagram, correlation is said to be perfectly negative e.g. pressure and volume of gas. r = -1 If the plotted points fall in a narrow band there would be a high degree of correlation between the variables. Y x Y x x x x x x x xx xx x x x x x x x x xx X X High degree +ve r High degree -ve r Rainfall and yield Intensity of diseases and yield If the points are widely scattered over the diagram, it is the indication of very little relationship between the variables. Y x x Y x x x x x x x xx x x x x x x x x x x x x x x x x x x x x x x X X Imperfect +ve Imperfect -ve (Low degree of positive correlation) (Low degree of negative correlation) If the points lie on a straight line parallel to the X-axis or in a haphazard manner it shows absence of any relationship between the variables e.g. height of students and marks. 2. Graphic method When this method is used the individual values of the two variables are plotted on the graph paper. We thus obtain two curves, one for X-variable and another for Y variable. By examining the direction and closeness of the two curves so drawn we can infer whether or not the variables are related. If both the curves drawn on the graph is moving in the same direction (either upper or downward) correlation is said to be positive. On the other hand, if the curves are moving in the opposite directions correlation is said to be negative. 3. Algebraic method (Karl Pearson Coefficient of correlation) (population) and its estimate as ‘r’ (sample) indicate Karl Pearson coefficient of correlation. Definition: It is a measure of intensity of association between two variables in a bivariate population. Computational formula: Cov( XY ) = XY r Cov( XY = xy = SP( xy ) S X SY x. y 2 2 SS X.SS Y where, X Y ( X i X )(Yi Y ) xy XY or n 1 n 1 Y 2 (Y Y )2 y Y n 1 2 2 i or n 1 X 2 (X X )2 x X 2 2 i or n 1 n 1 Properties of correlation coefficient: 1. A change in an origin does not affect the value of the correlation coefficient. 2. A change in a scale does not affect the value of correlation coefficient. 3. The value of correlation coefficient lies between -1 to +1. 4. Correlation coefficient is unit free. 5. Geometric mean of two-regression coefficient is equal to correlation coefficient. Test of significance of correlation coefficient Comparison of sample 'r' with population value Ho: = 0 (both the variables are not linearly associated) Ha: 0 1 r2 t(n-2) = r - / SE of r SE of r = n2 r n 2 under Ho : = 0 1 r2 If cal. t table t0.05, (n-2) d.f. Ho: rejected Rejection of Ho: Means there is an association between two variables under study. If cal. t < table t0.05 (n-2) d.f. Ho: accepted Acceptance of Ho: indicates that there is no association between two variables in the population. REGRESSION ANALYSIS REGRESSION Deals with Cause & Effect/Independent & Dependent Relationship Fertilizer Irrigation Diseases & Pests Weather parameters Rainfall, Temp.,WS, RH etc 15 y = 2x + 2 10 R² = 1 5 x = 0.5y - 1 0 0 2 4 6 -5 Y Y = a + bYX X bYX = tan X ON tan ( Slope B yx ) O MN Y Y Y X X M a N Y Y β YX X X X O X X β XY Y Y Regression lines In a scatter diagram if the points are scattered around a line than the relationship between two variables can be considered as linear. The resulting line is termed as regression line or line of best fit. For any pair of two variables that are related with each other linearly a set of two regression lines could be observed and they can be represented by two equations which are called regression equations. Let X and Y are the two variables. Then the two regression lines can be given by the two equations. The word regression was first used by Sir Fransis Galton and he introduces functional relationships between two variables. Many a times it is observed that change in one variable from a bivariate population causes change in the other variable, indicating a cause and effect relationship between the two variables. The former variable is termed as independent variable whereas, the later as dependent variable. Quantity of fertilizer and the crop will have this type of cause and effect relationship, where as quantity of fertilizer could be termed as independent variable and crop yield as dependent variable. The functional relationship between this independent and dependent variable is known as regression relationship. Definition: Regression is a study of average relationship between two or more variables in terms of original units of the data. Regression lines In a scatter diagram if the points are scattered around a line than the relationship between two variables can be considered as linear. The resulting line is termed as regression line or line of best fit. For any pair of two variables that are related with each other linearly a set of two regression lines could be observed and they can be represented by two equations which are called regression equations. Let X and Y are the two variables. Then the two regression lines can be given by the following two equations. Y Y β YX X X ...... (i) Increase the height also increase the weight but decrease the weight may or may not be decrease the weight. X X β XY Y Y ...... (ii) (Regg and coirre) Where, _ _ X = Mean of X variable; Y = Mean of Y variable yx = Reg. coefficient of Y on X ; xy = Reg. coefficient of X on Y We may observe that in first regression equation Y is considered as the dependent and X as independent where as in the second it is the reverse case. These lines have been shown in the following diagram. PQ bYX tan , QM RS b XY tan SM Fitting of the regression lines A regression equation which represents a straight line is of the following form. Y YX X Y = Here Y is the dependent variable and X is the independent variable. yx is population regression coefficient of Y on X Intercept Y YX X In case of the sample data the estimates of yx i.e. byx and the estimate of ‘’ as a are obtained and placed in the equation. a Y bYX X In a similar fashion the regression equation of the straight line where X is considered as dependent variable and Y as the independent variable the form of the equation would be X ' XY Y The estimate of xy is bxy and ' is a ' X b XY Y Regression coefficient Regression coefficient can be defined as the average increase or decrease in the dependent variable for a unit change in the independent variable or it is the average rate of change in dependent variable with a unit change in independent variable. It is represented by yx and xy for the population regression coefficient. In practice they are estimated with the help of the sample from the bivariate population under consideration and these estimates are generally represented as b yx and bxy respectively. Method of computation YX X X Y Y Cov( XY ) X X V (X ) 2 bYX X X Y Y xy XY X Y n X X x X X n 2 2 2 2 Similarly, XY X Y X Y Cov( XY ) Y V (Y ) 2 Y bYX X X Y Y xy XY X Y n Y Y y Y Y n 2 2 2 2 Test of significance of regression coefficient 1) When our interest is to ascertain whether the effect of the independent variable on the dependent variable is appreciable or not, we employ 't' test. Ho : yx = 0 Ha : yx = 0 y xy x 2 b YX 2 2 t SE of b YX SE of b YX n 2 x 2 Where, n = size of the sample The calculated t value is to be compared with the table t value at the desired level of significance with (n-2) d.f. and conclusion is to be drawn. Types of Regression models: 1. Linear : Y=a+bX 2. Quadratic: Y=a+bX2 or Y=a+bX+cX2 3. Cubic: Y=a+bX3 or Y=a+bX2+cX3 or Y =a+bX+cX2+dX3 4. Non-Linear: Y= abx Here, Y= dependent Variable, X= Independent Variable and a= Constant and b,c and d = regression coefficients Properties of regression coefficient 1) Geometric mean between regression coefficients is correlation coefficient i.e. r = b yx. b xy a) Arithmetic mean of byx & bxy is equal to or greater than correlation coefficient b b XY i.e. YX r 2 b) If one regression coefficient is greater than unity than other regression coefficient must be less than unity. 2) Regression coefficient is independent of origin but not scale 2) Regression coefficient lies between - to + 3) Regression coefficient has unit 5) Regression coefficient has one way relationship Uses of regression 1) To predict the value of Y for a given value of X with the help of regression equation. 2) To know the rate of change in Y for a unit change in X with the help of regression coefficient. Relations among r, byx, bxy, Sx and Sy SY SX (i) r b YX.b XY (ii) b YX r (iii) b XY r SX SY Differences between Correlation and Regression Correlation Regression 1 It deals with mutual It deals with cause and effect association relationship 2 It is two way relationship It is one way relationship 3 Correlation coefficient is unit Regression coefficient is in the units of free dependent variable 4 Correlation coefficient lies Regression coefficient lies between - between - 1 to + 1 and + 5 For a given value of one For a given value of independent variable other variable can not variable the value of the dependent be predicted variable can be predicted. STATISTICAL INFERENCE AND TESTING OF HYPOTHESIS (Test of Significance) Statistical inference is that branch of statistics, which is concerned with using probability concept to deal with uncertainty in decision making. It refers to the process of selecting and using a sample statistic or estimate to draw inferences about population parameters based on the sample drawn from the population. The subject of statistics deals with statistical estimation and testing of statistical hypothesis. These are the two important functions for drawing inference about the population parameters. Statistical estimation is the technique of estimating the population parameter values on the basis of information obtained from the sample. Suppose we wish to know the yield of a crop. To know this figure, it is not necessary to harvest entire field of that crop or all the fields of that crop grown in the region. One may collect the sample from the fields by appropriate sampling procedure and on the basis of sample information, one may estimate the average yield of the crop of entire area. The estimate thus obtained is not the final form for drawing valid conclusion regarding population from which the samples are drawn. It needs to be tested by applying an appropriate test or method. Such test is known as the test of significance. Thus, test of significance can be defined as “The statistical procedure for deciding whether the observed difference between sample estimate and population parametric value is significant or not at specified level of significance". Hypothesis: It is the statement specifying the parametric value of a distribution from which the sample/s is/are drawn. Null Hypothesis: It is a hypothesis of no difference between different populations parametric values from which samples are drawn OR It is the hypothesis of equality of population parametric values from which sample/s is/are drawn. Procedure for testing a hypothesis Step I : Set appropriate null hypothesis Let us consider that there are two methods for preparing compost. Method A : standard method and Method B : new method Now to test which method is better, the hypothesis can be 1) B is better than A B > A 2) A is better than B A > B 3) B is not different from A A = B The first two statements indicate a preferential attitude to one or the other of the two methods. Hence, it is better to adopt the third statement and make the test. This third statement is called the null hypothesis, which is denoted as H o: symbolically Ho : 1 = 2 or 1 - 2 = 0 where 1 and 2 are the population parametric values. In the above examples, suppose in first method the average nitrogen content is 1 and in the second method the average nitrogen is 2. Ho : 1 = 2 can be tested by the appropriate test. As against the null hypothesis, the alternative hypothesis should also be set up, which specifies those values, the researcher believes to hold true. Since one is going to accept or reject the null hypothesis one has to set the alternative hypothesis also. It is denoted by Ha : 1 2 or Ha : 1 < 2, 1 > 2. Step II : Fix appropriate level of significance The confidence with which an experimenter reject or accept the null hypothesis depends upon the significance level adopted. It is expressed in percentage such as 5 per cent, 1 per cent etc. When the hypothesis in question, is accepted at 5 per cent level of significance, the experimenter is running the risk that in the repeated cases (experiments/trials), he will be making the wrong decision in about 5 per cent of the cases. By rejecting the hypothesis at the same level, he runs the risk of rejecting a true hypothesis in 5 out of every 100 occasions. Thus, level of significance is defined as: "It is the maximum probability at which one would like to reject the null hypothesis when it is true OR The level of significance is the average proportion of incorrect statements made when the null hypothesis is true." Step III : Set suitable test criterion To construct a test criterion, one has to select the appropriate probability distribution for the particular test viz. Z, t, F, 2 etc. Step IV : Computation This step involves the calculations of various statistics from sample data such as mean and standard error of mean. Step V : Conclusion After doing the necessary calculations one has to decide whether to accept or reject the null hypothesis at a certain level of significance. Therefore, the computed value of the test criterion is compared with the table value. If the computed value is greater table value, the observed difference is significant and H o is not accepted. If calculated value is less than or equal to table value the H o is accepted at a given level of significance. Not acceptance of Ho means the difference between sample estimate and the hypothetical parametric value is a real difference, while acceptance of H o means the difference between sample estimate and population/hypothetical parametric value can be explained due to chance variation (sampling variation). Type - I and Type - II errors While testing the hypothesis one is liable to commit two kinds of errors. An error of first kind is made by rejecting the true null hypothesis. The probability of committing a Type-I error is denoted by (alpha). Type-II error is committed by accepting the null hypothesis when it is false. The probability of Type-II error is denoted by (Beta). Type-I error depends on the level of significance. When 5 per cent level of significance is fixed, we fixed the probability of committing Type-I error at 5 per cent. It is possible to control Type-I error by shifting the level of significance. Type-II error increases as the Type-I error decreases. Therefore, the common practice is to keep the Type-I error at five percent or one percent fixed and try to decrease Type-II error by increasing sample size and following refined technique of conducting experiment. Degrees of freedom For testing any hypothesis the estimated statistic is compared with table value. The knowledge of degrees of freedom is essential for referring the table value. With X 1, X2,.....Xn having constant sum, (n -1)X values can be given freely, but the nth X value will be determined by the condition that the sum of all `X` is equal to the given constant quantity i.e. one degree of freedom is lost. So in one way classification, number of observations - 1 is called degrees of freedom and in general number of observation minus number of independent constraints or restrictions is called degrees of freedom. Small sample or Student's ‘t’ test When the sample is large and if r is not known, we estimate the same and can be used in Z test. But if 'n' is small error will be more for replacing r by S and under that situation the Z remain no longer normal, but changes to another distribution named "t". The "t" distribution was found out by W.S. Gossett in the name of 'Student' in 1908. Values of 't' depends on degree of freedom and is always greater than its limiting value of Z for any unit degree of freedom. When d.f. is large tZ. Difference between t and Z becomes more and more marked as n become smaller and smaller. Definition: It is the ratio of the deviation between sample mean and hypothetical mean to the standard error of mean estimated from the small sample. Conditions for applying 't' test 1) Data follow normal distribution. 2) The sample is small (n < 30) and the standard deviation of the population is estimated from the sample. Uses 1) Comparing sample mean with hypothetical mean or population mean. 2) Comparing two sample means. (a) When the number of observation of both the samples are unequal (n1 n2). (b) When number of observations of both the samples are equal (n 1 = n2). (c) When the observations are paired. 3) Comparing the regression coefficient of sample with the hypothetical or population regression coefficient. 4) Comparing the correlation coefficient with the correlation coefficient of population. 5) Comparing two regression coefficients. Characteristics of "t" distribution 1) It is the exact distribution and not approximate. 2) t value ranges from - to + 3) The distribution is symmetrical one. 4) It is flatter than the normal distribution i.e. the area near the tail is large for t distribution compared to normal distribution. Value of coefficient of kurtosis is less than 3. 5) As sample size increases, the t distribution approaches to normal distribution. 6) There is need to know the d.f. to obtained the probability value from the table. One Sample 't' test Objective: To test whether the given small sample (n < 30) has come from the population having mean . Procedure : If (i) X1, X2, X3,... , Xn is the given sample (n < 30) or (ii) class value : X1, X2,... ,Xk with corresponding frequencies : f1, f2,... ,fk (fi = n) of a given sample, Step I : Set the null hypothesis : Ho : = o or Ho : - o = 0 Ha : o (two tailed test) or < o, > o (one tailed test) Where, is the population mean from which the random sample has been drawn and o is the mean of the hypothetical population. Step II : Fix the level of significance.Usually 5 and 1 per cent levels of significance are fixed. Step III : Calculate the following estimates. If (i) X1, X2, X3,... , Xn is the given sample (n < 30) or (ii) class value : X1, X2,...,Xk with corresponding frequencies f1, f2,... ,fk (fi = n) of a given sample, Calculate, n Xi Sample mean X i1 n n ( X i - X) 2 Variance : S2 = i1 (n 1) Standard error of mean: S SX = (If population standard deviation is known) n n Step IV : Compute the student 't' with (n-1) degree of freedom X O t SX Step V : Conclusion If calculated t < table t0.05,(n-1) d.f. observed difference is not significant. Ho: = o is accepted. Acceptance of Ho: = o means the given small random sample has come from the hypothetical population having mean o. If calculated t table t0.05,(n-1) d.f. observed difference is significant. Ho: = o is rejected. If calculated t table t0.01, (n-1) d.f., observed difference is highly significant. Ho: = o is rejected. Rejection of Ho: = o means the given random sample does not come from the hypothetical population having mean o. Two sample 't' test (Independent sample) Objective : To test whether the given two small random samples have come from the same population having mean o. Let sample - I : X1, X2,... ,Xn1 sample - II : Y1, Y2,... ,Yn2 are two random samples drawn from a population. Procedure: Step I: Set the null hypothesis that both the samples have come from the same population having mean and standard deviation S. i.e. Ho : 1 = 2 = against Ha : 1 2 or 1 > 2 or 1 < 2 Where 1 is the population mean from which sample one is drawn and 2 is the population mean from which the second sample is drawn. Step II: Fix the level of significance. Usually 5 per cent and 1 per cent levels of significance are fixed. Step III: Calculate the following estimates. Sample - I Sample - II n1 n2 Xi Yi i) Mean X i1 Y i1 n1 n2 ii) Variance n1 n2 ( X i - X) 2 (Yi - Y) 2 S 2x = i1 S 2y = i1 (n1 1) (n2 1) (iii) Pooled sample variance n1 n2 n1 n2 ( X i - X) 2 (Yi - Y) 2 fi (X i - X) 2 fi (Yi - Y) 2 Sp2 i1 i1 or Sp2 i1 i1 n1 n 2 - 2 n1 n 2 - 2 (iv) Standard error of mean of differences 1 1 Sp2 ( ) S n1 n 2 (XY) Step IV: Calculate student 't' with n1 + n2 - 2 d.f. ( X 1) (Y 2 ) t S( X Y ) Step V: If cal t < Table t 0.05, (n1+n2-2) d.f. difference is non significant at 5% level of significance Ho: 1 = 2 accepted. Acceptance of Ho: 1 = 2 means both the samples have came from the same population If cal t table t0.05,(n1+n2-2) d.f. difference is significant at 5 % level of significant Ho : 1 = 2 rejected at 5 % level of significance. If cal t table t 0.01,(n1+n2-2) d.f. difference is highly significant 1 % level of significance. Ho:1 = 2 rejected at 1% level of significance. Rejection of H o : 1 = 2 means both the sample are drawn from two different populations. Two sample 't' test ( Dependent sample) : Paired 't' test Objective: To test whether the two small related random samples have come from the same population. Let Sample-I : X1, X2,... ,Xn and Sample-II: Y1, Y2,... ,Yn be two related sample such that (X1, Y1), (X2, Y2),... , ( Xn, Yn) are the pairs of related observations. Procedure : Step I : Set the null hypothesis : Ho : d = 0 ; Ha : d 0 Where, d is the average difference between Xi - Yi in the population. Step II: Fix the level of significance. Usually 5 per cent and 1 per cent levels of significance are fixed. Step III: Calculate the following estimates. (i) di = Xi - Yi i = 1,... ,n (ii) d di n d 2 2 i d (iii) S n 1 d 2 S2 i d (iv) S.E. of d n nn 1 Step IV: Calculate the student t with n-1 d.f. d d t (Under Ho : d = 0 ) Sd Step V: Conclusion If cal. t < table t 0.05, (n-1) d.f., observed difference is non-significant at 5% level of significance and null hypothesis (Ho) is accepted. Acceptance of null hypothesis (Ho: d = 0) means the given two related small samples have come from the same population. If cal. t table t 0.05, (n-1) d.f., observed difference is significant at 5% level of significance and null hypothesis (Ho) is rejected at 5% level of significance. If cal. t table t 0.01, (n-1) d.f., observed difference is highly significant at 1% level of significance and null hypothesis (Ho) is rejected at 1% level of significance. Rejection of null hypothesis (Ho : d = 0) means the given two related samples does not come from the same population. Large sample test: Z - test It is a large sample test and can be utilized for testing the hypothesis if the following conditions are satisfied. (1) Data follow normal distribution. (2) Sample size should be large ( n > 30 ) or (3) The standard deviation of population should be known if sample is not large. Z test can be defined as "It is the ratio of the difference between the estimated population mean and hypothetical mean to the standard error of mean based on population standard deviation or its estimate from large sample. Confidence limits Usually parametric values of various characteristics are not known but their estimates are obtained from the samples. Such estimates are known as point estimates of the corresponding parameters. The reliability of such point estimates varies. Some time the mathematical distribution of such estimates is known. On the basis of the nature of distribution and the null hypothesis for a given probability, interval estimates can be worked out which specify that the parametric value may lie between these two values, with a given probability level of confidence. These two values of such interval estimates are known as confidence limits. Thus, in case of test of significance of the differences between the sample mean X and population mean , one has to determine with reasonable degree of confidence, the range within which the true mean may lie. The limit of this range is usually expressed as confidence limits and the range of these limits is called confidence interval. Confidence limits depends on (1) Size of the sample (2) Level of significance and (3) Inherent variation exists in the population. The confidence limit for various test criterions can be worked out as under. (A) Large sample (I) One sample Lower limit: X - Z .S X l1 Upper limit: X Z .S X l2 l1 < o < l2 (ii) Two sample Lower limit: X - Y - Z . S( X Y ) l1 Upper limit: X - Y Z . S( X Y) l2 l1 < < l2 (B) Small sample: (i) One sample Lower limit: X - t , (n - 1). S X l1 Upper limit: X t , (n - 1). S X l2 l1 < < l2 (ii) Two sample Lower limit: X - Y - t ,(n1 n2 - 2). S( X Y) l1 Upper limit: X - Y t ,(n1 n2 - 2). S( X Y) l 2 l1 < < l2 (iii) Paired observation Lower limit: | d | - t , (n - 1). S d l1 Upper limit: | d | t , (n - 1). S d l2