New Mansoura University Statistics (MAT131) Lecture 01 PDF
Document Details
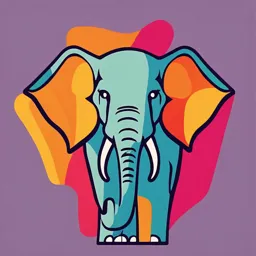
Uploaded by AudibleAnaphora
New Mansoura University
Tags
Summary
This document is a lecture on statistics, covering basic concepts and terminology, variable types (qualitative and quantitative), populations versus samples, sampling methods (simple random, stratified, cluster, systematic), and descriptive versus inferential statistics.
Full Transcript
Statistics (MAT131) LECTURE 01 Introduction and Basic Terminology Why Statistics? Deal with uncertainty in repeated scientific measurements. Draw reliable conclusions from data. Design valid experiments and draw reliable conclusions. Develop and maintain an organized mindset....
Statistics (MAT131) LECTURE 01 Introduction and Basic Terminology Why Statistics? Deal with uncertainty in repeated scientific measurements. Draw reliable conclusions from data. Design valid experiments and draw reliable conclusions. Develop and maintain an organized mindset. Why Statistics? You study Statistics for several reasons, for example: To be able to read and understand the various statistical studies performed in your fields. You may be called on to conduct research in your field, since statistical procedures are basic to research. To accomplish this, you must be able to design experiments; collect, organize, analyze, and summarize data; and possibly make reliable predictions or forecasts for future use Basic Definition and Notations Variable: is a characteristic or attribute that can assume different values. The values themselves are called Data. For example, We can design a variable to measure: 1. The Height in cm. The data associated with the variable could be {160, 165, 185}. 2. The number of things (like shirts). The data associated with the variable could be {5, 0, 7}. 3. The grade of calculus course. The data associated with the variable could be {F, F, D}. Basic Definition and Notations A population is the entire collection of objects or outcomes about which information is sought. The total number of subjects in the population is called population size and is usually denoted by uppercase N. A sample is a subset of a population, containing the objects or outcomes that are actually observed. Likewise, the total number of subjects in the sample is called sample size and is usually denoted by lowercase n. Basic Definition and Notations Researchers use samples to collect data and information about a particular variable from a large population. Using samples saves time and money and in some cases enables the researcher to get more detailed information about a particular subject. Statisticians use different methods for sampling that include: ▪ Simple Random Sampling. ▪ Stratified Random Sampling ▪ Cluster Sampling , and ▪ Systematic Cluster Sampling Basic Definition and Notations Simple Random Sampling (SRS): samples are selected by using chance methods or random numbers. For example: Random selection of 5 students from class of 15 student. Each student has equal chance of getting selected. Here probability of selection is 1/15. Basic Definition and Notations Stratified Random Sampling: the population is divided up into subpopulations, called strata, based on the similarity in such a way that the elements within the group are homogeneous and heterogeneous among the other subgroups formed. And then the elements are randomly selected from each of these strata. Note: We need to have prior information about the population to create subgroups. Basic Definition and Notations Cluster Sampling: are obtained by dividing the population into groups called clusters by some means such as education level or type of infection then the researcher randomly selects some of these clusters and uses all members of the selected clusters as the subjects of the samples. Basic Definition and Notations Systematic Clustering Sampling: Here the selection of elements is systematic and not random except the first element. Elements of a sample are chosen at regular intervals of population. All the elements are put together in a sequence first where each element has the equal chance of being selected. Descriptive and Inferential Statistics Statistics is sometimes divided into two main areas, depending on how data are used. The two areas are: Descriptive statistics consists of the collection, organization, summarization, and presentation of data. Inferential statistics consists of generalizing from samples to populations, performing estimations and hypothesis tests, determining relationships among variables, and making predictions. Descriptive Statistics Types of Data / Variables Descriptive Statistics Qualitative variables are variables that can be placed into distinct categories, according to some characteristic or attribute. For example, if subjects are classified according to gender (male or female), then the variable gender is qualitative. Other examples of qualitative variables are hair color, nationality and geographic locations. Descriptive Statistics Quantitative variables are numerical and can be ordered or ranked. For example, the variable age is numerical, and people can be ranked in order according to the value of their ages. Other examples of quantitative variables are heights, weights, and body temperatures. Quantitative variables can be further classified into two groups: discrete and continuous. Descriptive Statistics Discrete variables can be assigned values such as 0, 1, 2, 3 and are said to be countable. Examples of discrete variables are the number of children in a family, the number of students in a classroom, and the number of cars crossing some road in a day. In simple words we say: Discrete variables assume values that can be counted. Descriptive Statistics Continuous variables, by comparison, can assume an infinite number of values in an interval between any two specific values and they often include fractions and decimals. Temperature, for example, is a continuous variable, since the variable can assume an infinite number of values between any two given temperatures. Organizing Data FREQUENCY DISTRIBUTIONS Organizing Data Frequency Distributions When conducting a statistical study, the researcher must gather data for the variable under study. For example, if a researcher wishes to study the number of people who were bitten by poisonous snakes in a specific area over the past several years, he or she must gather the data from various doctors, hospitals, or health departments. To describe situations, draw conclusions, or make inferences about events, the researcher must organize the data in some meaningful way. Organizing Data Frequency Distributions The most convenient method of organizing data is to construct a frequency distribution. A frequency distribution is the organization of raw data in table form, using classes and frequencies. After organizing the data, the researcher must present them so they can be understood by those who will benefit from reading the study. The most useful method of presenting the data is by constructing statistical charts and graphs. There are many different types of charts and graphs, but we will cover them later. Organizing Data Frequency Distributions Two types of frequency distributions that are most often used are the categorical frequency distribution and the grouped frequency distribution. ▪ Categorical Frequency Distributions: used for data that can be placed in specific categories. For example, data such as skin color, nationality, blood type, or course grades. The variable is usually qualitative or discrete quantitative (with few values). ▪ Grouped Frequency Distributions: used when the range of the data is large and therefore the data must be grouped into classes that are more than one unit in width. Examples are weight of a sample of people and speed of cars detected by a radar within some time interval. The variable is usually continuous quantitative or discrete quantitative (with lots of values). Organizing Data Categorical Frequency Distributions The categorical frequency distribution (also called simple frequency distribution) is simple to construct. We only need to find out: 1. What is the variable we are studying 2. All the possible values of the variable Once done, we put a table like the one below where we count the frequencies of each category. Category / Value Tally Frequency Percent value 1 value 2 … value n Organizing Data Categorical Frequency Distributions Example: Twenty-five army soldiers were given a blood test to determine their blood type and the data set is shown below. A B B AB O O O B AB B B B O A O A O O O AB AB A O B A Construct a frequency distribution for the data. Organizing Data Categorical Frequency Distributions The variable we are studying is blood type which is categorical / qualitative so discrete classes can be used. There are four blood types: A, B, O, and AB. These types will be used as the classes for the distribution. A B B AB O Class Tally Frequency Percent O O B AB B A B B O A O B A O O O AB O AB A O B A AB Organizing Data Categorical Frequency Distributions Example: A study was performed on a sample of 20 males and females over 60 years where the number of surgeries they undergo throughout their lives is counted to help assessing the healthcare system and the results are shown below. 5 4 4 2 3 1 4 2 3 0 1 5 6 2 1 5 1 3 3 2 Construct the frequency table for the number of surgeries. Organizing Data Categorical Frequency Distributions The variable we are studying is 5 4 4 2 3 number of surgeries which is 1 4 2 3 0 quantitative but with just so few 1 5 6 2 1 values so discrete classes can be 5 1 3 3 2 used. There are seven possible values: Class Tally Frequency Percent 0, 1, 2, 3, 4, 5, and 6. These 0 values will be used as the classes 1 for the distribution. 2 3 4 5 6 Organizing Data Grouped Frequency Distributions The grouped frequency distribution needs more work to construct. We need to follow these rules … 1. There should be between 5 and 20 Classes Tally Freq. Rel. Freq. Density classes. 1 — < 11 2. It is preferable but not necessary 11 — < 21 that the class width be an odd 21 — < 31 number. 31 — < 41 41 — < 51 3. The classes must be mutually 51 — < 61 exclusive. 61 — < 71 4. The classes must be continuous. 71 — < 81 5. The classes must be exhaustive. Total … Organizing Data Grouped Frequency Distributions Example: A researcher wished to do a study on the ages of the top 50 wealthiest people in the world. He gathered the data on the ages of the people, and they are listed below. Construct the frequency table for the age. 49 57 38 73 81 74 59 76 65 69 54 56 69 68 78 65 85 49 69 61 48 81 68 37 43 78 82 43 64 67 52 56 81 77 79 85 40 85 59 80 60 71 57 61 69 61 83 90 87 74 Organizing Data Grouped Frequency Distributions First, we find the smallest and largest numbers: Smallest = 37 , Largest = 90 Next, we find the range of data: 𝑅 = Largest − Smallest = 90 − 37 = 53 Select the number of classes desired (usually between 5 and 20). In this case, 5 is arbitrarily chosen. Find the class width by dividing the range by the number of classes: 𝑅 53 Width = = = 10.6 ≅ 11 number of classes 5 Select a starting point for the lowest class limit. This can be the smallest data value or any convenient number less than the smallest data value. Let use 37, the smallest value. Organizing Data Grouped Frequency Distributions In summary, we will create 5 classes each has a width of 11 and the first one starts with 37. We start classifying each data point, draw the tallies, and finally count the frequencies. Class Tally Freq. Rel. Freq. Density 49 57 38 73 81 74 59 76 65 69 37 — < 48 ///// 5 54 56 69 68 78 65 85 49 69 61 48 — < 59 ///// ///// 10 48 81 68 37 43 78 82 43 64 67 59 — < 70 ///// ///// ///// / 16 52 56 81 77 79 85 40 85 59 80 70 — < 81 ///// ///// 10 81 — < 92 ///// //// 9 60 71 57 61 69 61 83 90 87 74 Total 50 Graphical Summaries Graphical Summaries After you have organized the data into a frequency distribution, you can present them in graphical form. The purpose of graphs in statistics is to convey the data to the viewers in pictorial form. It is easier for most people to comprehend the meaning of data presented graphically than data presented numerically in tables or frequency distributions. This is especially true if the users have little or no statistical knowledge. We are going to study: 1. Bar chart, 2. Pie chart, 3. Stem-and-leaf plot, 4. Dotplot, 5. Histogram, 6. Frequency polygon, 7. Cumulative frequency graph (aka: ogive). Other graphs will be studied too in later lectures like Boxplot and Scatterplot. 1. Bar chart One of the most fundamental chart types is the bar chart, and one of your most useful tools when it comes to exploring and understanding your data. A bar chart or bar graph is a chart or graph that presents categorical data with rectangular bars with heights or lengths proportional to the values that they represent. The bars can be plotted vertically or horizontally. A vertical bar chart is sometimes called a column chart. 1. Bar chart A bar chart is used when you want to show a distribution of data points or perform a comparison of metric values across different subgroups of your data. From a bar chart, we can see which groups are highest or most common, and how other groups compare against the others. 1. Bar chart Example: The grades of students in some class is listed below. Draw a bar chart to summarize the data. A D+ C C D+ A- B B+ D D D D+ B A+ D D A- C C+ A Solution: Grades 6 5 4 3 2 1 0 D D+ C- C C+ B- B B+ A- A A+ 1. Bar chart Example: Twenty-five army soldiers were given a blood test to determine their blood type and the data set is shown below. Construct a frequency distribution then draw a bar chart. A B B AB O O O B AB B B B O A O A O O O AB AB A O B A 1. Bar chart Solution: Blood Type A B B AB O 10 O O B AB B 9 B B O A O 8 A O O O AB 7 6 AB A O B A 5 4 Class Tally Frequency Percent 3 A //// 5 20% 2 B //// // 7 28% 1 0 O //// //// 9 36% A B O AB AB //// 4 16% 2. Pie chart A pie chart is a circular statistical graphic, PIE CHART which is divided into slices to illustrate Group 1 Group 2 Group 3 Group 4 Group 5 numerical proportion. In a pie chart, the arc Group 5 length of each slice is proportional to the 7% quantity it represents. Group 1 To draw a pie chart: Group 4 33% 27% 1. Calculate the percentage, 𝑃, for each group. 2. Calculate the slice angle, 𝜃, for the group: 360 ∗ 𝑃 𝜃= 100 Group 3 13% Group 2 3. Draw and label the slice. 20% 2. Pie chart Example: Suppose we have a sample of 20 blood donor in a particular blood banks. After colleting blood samples, the bank recorded the type of the blood of each donor which is shown below along with the frequency table. Find the bar and pie charts. Blood Type Frequency Percentage (%) A+ 1 5 A− 3 15 B+ 2 10 AB + B+ O+ AB + O− A− B− O+ A− O+ B− 3 15 AB + 3 15 B− O+ A+ AB − A− B− O− AB + B+ O− AB − 1 5 O+ 4 20 O− 3 15 A+ 1 5 Total 20 100 2. Pie chart Solution: Bar chart of the blood type Pie chart of the blood type 4.5 A+ A- B+ B- AB+ AB- O+ O- 4 3.5 A+ O- Frequency 3 5% 15% A- 2.5 15% 2 1.5 O+ B+ 1 20% 10% 0.5 AB- B- 0 5% 15% A+ A- B+ B- AB+ AB- O+ O- AB+ Blood Type 15% 3. Stem-and-leaf plot A stem and leaf plot looks something like a bar graph. Each number in the data is broken down into a stem and a leaf, thus the name. The stem of the number includes all but the last digit. The leaf of the number will always be a single digit. We can see that it is a simple and compact way to represent the data. It also gives us some indication of the shape of our data. 3. Stem-and-leaf plot How to draw a stem and leaf plot? Once you have decided that a stem and leaf plot is the best way to show your data, draw it as follows: ▪ On the left-hand side of the page, write down the thousands, hundreds or tens (all digits but the last one). These will be your stems. ▪ On the right-hand side, write down the ones (the last digit of a number). These will be your leaves. ▪ Stem-and-leaf plot is usually ordered. So, you will have to order the stems then the digits in each leaf. 3. Stem-and-leaf plot Example: A teacher asked 10 of her students how many books they had read in the last 12 months. Their answers were as follows: 12, 23, 19, 6, 10, 7, 15, 25, 21, 12 Prepare a stem and leaf plot for these data. Solution: 3. Stem-and-leaf plot Example: Consider the given stem-and-leaf plot. What is the minimum value of the data? What is the maximum value of the data? Stem Leaf 3 2 3 4 4 4 6 5 0 7 5 7 9 8 2 4 9 3 Solution: 4. Dotplot A dot plot is used to represent any data in the form of dots or small circles. It is similar to a simplified histogram or a bar graph as the height of the bar formed with dots represents the numerical value of each variable. Dot plots are used to represent small amounts of data. 4. Dotplot Example: Draw a dot plot for the number of vaccinated newborns in four areas of a city, which is represented in the following table. Area 1 2 3 4 No. 7 3 5 1 Solution: Area 1 Area 2 Area 3 Area 4 4. Dotplot Example: The data which is given below shows the number of books read by a number of kids during last summer holidays. Draw a dotplot chart for the data. No.of books read 0 1 2 3 4 5 6 7 8 9 No. of Kids 4 6 3 1 2 1 0 1 1 1 Solution: 0 1 2 3 4 5 6 7 8 9 5. Histogram The histogram is a graph that displays the data by using contiguous vertical bars (unless the frequency of a class is 0) of various heights to represent the frequencies of the classes. The steps to draw a histogram are: 1. Draw and label the 𝑥 and 𝑦 axes. The 𝑥 axis is always the horizontal axis, and the 𝑦 axis is always the vertical axis. 2. Represent the frequency on the 𝑦 axis and the class boundaries on the 𝑥 axis. 3. Using the frequencies (or relative frequencies) as the heights, draw vertical bars for each class. NOTE: If the class intervals are of unequal widths, the heights of the vertical bars must be set equal to the densities, where density is the relative frequency divided by the class width. 5. Histogram Example: Draw the histogram for the given frequency table on below. Class Tally Frequency Rel. Freq. 37 —< 48 //// 5 0.1 48 —< 59 //// //// 10 0.2 59 —< 70 //// //// //// / 16 0.32 70 —< 81 //// //// 10 0.2 81 —< 92 //// //// 9 0.18 Total 50 Solution: 37 48 59 70 81 92 5. Histogram Example: The following is a frequency table, with unequal class widths, for emissions of 62 vehicles driven at high altitude. Draw the corresponding histogram. 0.15 Class Frequency Rel. Freq. Density 1 —< 3 12 0.1935 0.0968 0.1 3 —< 5 11 0.1774 0.0887 Density 5 —< 7 18 0.2903 0.1452 7 —< 9 9 0.1452 0.0726 0.05 9 —< 11 5 0.0806 0.0403 11—< 15 3 0.0484 0.0121 15 —< 25 4 0.0645 0.0065 0 1 3 5 7 9 11 15 25 Emmisions Solution: 5. Histogram Example: Use the histogram from the previous example to determine the proportion of the vehicles in the sample with emissions between 7 and 11. 0.15 Solution: ▪ The proportion is the sum of the relative 0.1 Density frequencies of the two classes spanning the range between 7 and 11. 0.05 ▪ Since the heights of the rectangles represent densities, the areas of the rectangles represent relative frequencies. The sum of 0 the areas of the rectangles is: 1 3 5 7 9 11 15 25 ▪ (2)(0.0726) + (2)(0.0403) = 0.2258 Emmisions 6. Frequency Polygon The frequency polygon is a graph that displays the data by using lines that connect points plotted for the frequencies at the midpoints of the classes. The frequencies are represented by the heights of the points. The steps to draw a frequency polygon are: 1. Find the midpoints of each class. Recall that midpoints are found by adding the upper and lower boundaries and dividing by 2. 2. Draw the x and y axes. Label the x axis with the midpoint of each class, and then use a suitable scale on the y axis for the frequencies. 3. Using the midpoints for the x values and the frequencies as the y values, plot the points. 4. Connect adjacent points with line segments. Draw a line back to the x axis at the beginning and end of the graph, at the same distance that the previous and next midpoints would be located. 6. Frequency Polygon Example: Draw the frequency polygon for the given frequency table below. Class Midpoints Frequency 37 —< 48 42.5 5 18 48 —< 59 53.5 10 16 59 —< 70 64.5 16 14 70 —< 81 75.5 10 12 81 —< 92 86.5 9 10 Total 50 8 6 Solution: 4 2 0 20 30 40 50 60 70 80 90 100 110 7. Cumulative Frequency (Ogive) The ogive is a graph that represents the cumulative frequencies for the classes in a frequency distribution. The steps to draw a frequency polygon are: 1. Find the cumulative frequency for each class. 2. Draw the x and y axes. Label the x axis with the class boundaries. Use an appropriate scale for the y axis to represent the cumulative frequencies. 3. Plot the cumulative frequency at each upper-class boundary. 4. Connect adjacent points with line segments then extend the graph to the first lower class boundary on the x axis. 7. Cumulative Frequency (Ogive) Example: Draw the ogive for the given frequency table below. Class Midpoints Frequency Cum. Freq. 37 —< 48 42.5 5 5 48 —< 59 53.5 10 15 60 59 —< 70 64.5 16 31 50 70 —< 81 75.5 10 41 81 —< 92 86.5 9 50 40 Total 50 30 Solution: 20 10 0 20 30 40 50 60 70 80 90 100 110