Introduction to Hypothesis Testing PDF
Document Details
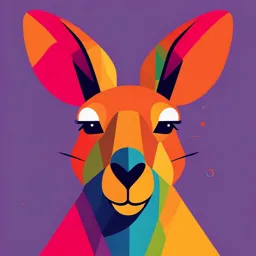
Uploaded by RefinedSilicon
JOMAR S. P. BAUDIN
Tags
Summary
This document presents an introduction to hypothesis testing. It explains the fundamental concepts, logic, and steps involved. The document covers the importance of hypothesis testing in scientific inquiry, including the role of probability, p-values, and significance levels.
Full Transcript
Chapter 5 Introduction to Hypothesis Testing by: JOMAR S. P. BAUDIN Hypothesis testing is a fundamental concept in statistical analysis and forms the backbone of scientific inquiry in psychology and other fields. It provides a systematic approach to making inferences about populations bas...
Chapter 5 Introduction to Hypothesis Testing by: JOMAR S. P. BAUDIN Hypothesis testing is a fundamental concept in statistical analysis and forms the backbone of scientific inquiry in psychology and other fields. It provides a systematic approach to making inferences about populations based on sample data. Logic and Purpose Hypothesis testing is a method of statistical inference that allows researchers to make decisions about populations based on sample data. The fundamental logic behind hypothesis testing is to assume a null hypothesis (typically a statement of no effect or no difference) and then use sample data to determine whether this assumption is likely to be true. The Need for Hypothesis Testing In psychological research, we're often interested in questions like: Does a new therapy reduce symptoms of depression more than a placebo? Is there a relationship between hours of sleep and academic performance? Do men and women differ in their attitudes towards climate change? We can't usually study entire populations, so we collect data from samples. Hypothesis testing provides a framework for deciding whether the patterns we observe in our sample data are likely to reflect true patterns in the population, or whether they might have occurred by chance. The Logic for Hypothesis Testing The logic of hypothesis testing can be summarized in a few key steps: 1. Formulate a null hypothesis (H₀) and an alternative hypothesis (H₁). 2. Collect sample data. 3. Calculate a test statistic from the sample data. 4. Determine the probability of obtaining a test statistic at least as extreme as the one calculated, assuming the null hypothesis is true. 5. Make a decision about the null hypothesis based on this probability. This process allows us to quantify the evidence against the null hypothesis and make decisions with a known error rate. The Role of Probability in Hypothesis Testing Probability plays a crucial role in hypothesis testing. We use probability theory to determine how likely our observed results would be if the null hypothesis were true. If this probability is very small, we reject the null hypothesis in favor of the alternative hypothesis. It's important to note that hypothesis testing doesn't prove or disprove hypotheses with certainty. Instead, it allows us to make decisions while controlling the long-run error rates of those decisions. The Probability Value The probability value, or p-value, is a key concept in hypothesis testing. It represents the probability of obtaining results at least as extreme as those observed, assuming the null hypothesis is true. More formally, the p-value is defined as the probability of obtaining a test statistic at least as extreme as the one calculated from the sample data, assuming the null hypothesis is true. Interpreting p-values The p-value is often misinterpreted. Here are some important points about p-values: 1. A p-value is not the probability that the null hypothesis is true. 2. A p-value is not the probability that the results occurred by chance. 3. A small p-value doesn't mean the effect is large or important. 4. A large p-value doesn't prove the null hypothesis. The p-value is a measure of the compatibility between the data and the null hypothesis. A small p-value suggests that the observed data would be unlikely if the null hypothesis were true. Common Misintepretations of p-value Researchers often fall into traps when interpreting p-values. Here are some common misinterpretations to avoid: 1. "p = 0.05 means there's a 5% chance the null hypothesis is true." Incorrect. The p-value assumes the null hypothesis is true. 2. "A non-significant result (p > 0.05) proves the null hypothesis." Incorrect. Failure to reject the null hypothesis is not the same as proving it true. 3. "A p-value of 0.05 means the effect has a 95% chance of being real." Incorrect. The p-value doesn't tell us the probability of the effect being real. 4. "If p = 0.04, the probability of replication is 96%." Incorrect. The p-value doesn't directly relate to replication probability. Understanding these common misinterpretations can help researchers avoid making incorrect conclusions based on p-values. The Null Hypothesis The null hypothesis (H₀) is a statement of no effect or no difference. It's the hypothesis that researchers try to reject in favor of an alternative hypothesis. 1. It typically represents the status quo or a statement of no change. 2. It's often a statement of equality (e.g., μ₁ = μ₂ or ρ = 0). 3. It's the hypothesis assumed to be true for the purposes of statistical testing. The Null Hypothesis (Examples) 1. In a study comparing two therapies: H₀: μ₁ = μ₂ (The mean effectiveness of therapy 1 equals the mean effectiveness of therapy 2) 2. In a correlational study: H₀: ρ = 0 (There is no correlation between the two variables in the population) 3. In a study comparing a sample mean to a population mean: H₀: x̄ = μ (The sample mean is equal to the hypothesized population mean) The Alternative Hypothesis The alternative hypothesis (H₁ or Hₐ) is the hypothesis that the researcher wants to support. It's typically the opposite of the null hypothesis and represents the presence of an effect or difference. 1. It represents the research prediction or the hypothesis of change. 2. It's typically a statement of inequality (e.g., μ₁ ≠ μ₂ or ρ ≠ 0). 3. It's accepted when the null hypothesis is rejected. Types of Alternative Hypothesis 1. Two-tailed (non-directional): The alternative hypothesis states that there is a difference, but doesn't specify the direction. Example: H₁: μ₁ ≠ μ₂ 2. One-tailed (directional): The alternative hypothesis specifies the direction of the difference. Example: H₁: μ₁ > μ₂ or H₁: μ₁ < μ₂ The choice between one-tailed and two-tailed tests depends on the research question and prior knowledge. One-tailed tests have more power but should only be used when there's a strong theoretical or practical reason to expect an effect in only one direction. Examples of Alternative Hypothesis 1. Two-tailed: H₁: The mean effectiveness of therapy 1 is different from the mean effectiveness of therapy 2. 2. One-tailed: H₁: There is a positive correlation between hours of sleep and academic performance. 3. Two-tailed: H₁: The mean anxiety score in the sample is different from the population mean. Critical values, p- values, and significance level Significance Level (α) The significance level, denoted by α (alpha), is the probability of rejecting the null hypothesis when it is actually true (Type I error). Common values for α are 0.05 and 0.01. Choosing α is a trade-off: A smaller α reduces the chance of Type I errors but increases the chance of Type II errors (failing to reject a false null hypothesis). A larger α does the opposite. Critical Values Critical values are the boundaries of the rejection region in a distribution. If the test statistic falls beyond the critical value(s), we reject the null hypothesis. For a two-tailed test with α = 0.05: In a z-distribution, the critical values are ±1.96 In a t-distribution, the critical values depend on the degrees of freedom Decision Rules If p < α, we reject the null hypothesis. If p ≥ α, we fail to reject the null hypothesis. If test statistics > critical value, reject the null hypothesis If test statistics ≤ critical value, fail to reject the null hypothesis NOTE: Critical value method in Mann-Whitney U Test is reversed Errors in Hypothesis Testing Steps in Hypothesis Testing Step 1: State the Hypotheses Clearly state both the null hypothesis (H₀) and the alternative hypothesis (H₁). Step 2: Choose the Significance Level (α) Decide on the significance level before conducting the test. Common choices are α = 0.05 or α = 0.01. Step 3: Select the Appropriate Test Statistic Choose the appropriate statistical test based on the research question, type of data, and assumptions of the test. Step 4: Calculate the Test Statistic Use the sample data to calculate the value of the test statistic. Step 5: Determine the Critical Value or p-value Find the critical value(s) for the chosen α level, or calculate the p-value associated with the test statistic. Step 6: Make a Decision Compare the test statistic to the critical value(s) or compare the p-value to α. Decide whether to reject or fail to reject the null hypothesis. Step 7: State the Conclusion Interpret the results in the context of the research question. Research Question: Do psychology students have a different average IQ than the general population? Step 1: State the Hypotheses H₀: μ = 100 (The mean IQ of psychology students is 100) H₁: μ ≠ 100 (The mean IQ of psychology students is not 100) *This is two tailed Step 2: Choose the Significance Level α = 0.05 Step 3: Select the Appropriate Test Statistic One-sample t-test Step 4: Calculate the test statistics t = 3.45 Step 5: Determine the Critical Value or p-value p-value = 0.03 Critical value = 3.06 Step 6: Make a Decision Reject the null hypothesis Step 7: Generate Conclusion Psychology students have different average IQ than the general population. Effect Size While hypothesis testing tells us whether an effect is statistically significant, it doesn't tell us about the magnitude of the effect. This is where effect size comes in. Effect size is a measure of the strength of a phenomenon. It quantifies the difference between groups or the strength of a relationship between variables. Importance of Effect Size 1. It provides information about the practical significance of a result, not just its statistical significance. 2. It allows for comparison across studies, even when sample sizes differ. 3. It's less affected by sample size than p-values. Common Measures of Effect Size 1. Cohen's d: Used for comparing two means. It represents the difference between two means divided by their pooled standard deviation. 2. Pearson's r: Used for correlations. It ranges from -1 to 1 and represents the strength and direction of a linear relationship. 3. Eta-squared (η²): Used in ANOVA. It represents the proportion of variance in the dependent variable explained by the independent variable. Interpreting effect size Cohen (1988) suggested the following guidelines for interpreting effect sizes: For Cohen's d: Small effect: d = 0.2 Medium effect: d = 0.5 Large effect: d = 0.8 For Pearson's r: Small effect: r = 0.1 Medium effect: r = 0.3 Large effect: r = 0.5 However, these guidelines should be used cautiously and in context. In some fields, even a small effect size can be practically significant. THANK YOU End of Chapter 5