Hypothesis and Significance Testing Lecture 4 PDF
Document Details
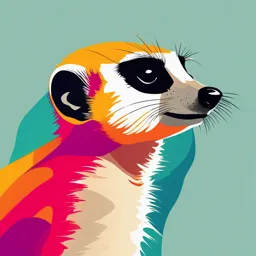
Uploaded by VictoriousElf1785
Bournemouth University
Bryan Leong
Tags
Summary
These lecture notes cover hypothesis and significance testing, focusing on inferential statistics. The document describes population means and samples, as well as the concept of sampling errors. It also touches upon standard error of mean and how it's different from standard deviation.
Full Transcript
HYPOTHESIS & SIGNIFICANCE TESTING Week 4 Experimental Methods and Bryan Leong Statistical Analysis 24/25 Aim of today’s lecture By the end of the class, students should be able to understand: 1. Inferential statistics...
HYPOTHESIS & SIGNIFICANCE TESTING Week 4 Experimental Methods and Bryan Leong Statistical Analysis 24/25 Aim of today’s lecture By the end of the class, students should be able to understand: 1. Inferential statistics 2. Hypothesis testing 3. How to write-up the ‘Methods’ section Inferential Statistics Population: Entire group that you want to make conclusions about Sample: Specific group that you collect data from When conducting a study, we take a specific sample from a specific population Inferential Statistics When conducting a study, we take a specific sample from a specific population E.g., a sample of individuals with depression; But why??? Inferential Statistics Generalize the results from the sample to the entire population We know the results in the sample We don’t know the results in the population Population Mean A psychologist designed an intervention to reduce depressive symptoms, ranging from 1 to 5. He took a sample of five depressive patients. After the intervention, on average, the patients obtained a score of 1 in a depression questionnaire. Population Mean Let’s assume that the mean of the population (all depressive people in the world) has a score of 3, and it is unlikely for a depressive patient to obtain a score of 1. If the intervention worked, we would expect that the average score of our depressive participants would be smaller than the entire depressive population (i.e., 3). But hold on… However, the mean of each sample that we collect is going to differ some from the true mean of the population, as well as from other samples. E.g., the first group of 5 participants may have a Mean = 3 Mean = 2 Mean = 4 Mean = 1 higher/lower mean than another group of 5 participants. Population Mean This difference between samples is called ‘sampling error’, and we need to characterize how serious is this for our sample! 3 2 1 0 1 2 3 Population Mean Nonetheless, if we took many Distribution of samples means samples and calculate the mean 4 for each of them, we would see 3 that the distribution of all these sample’s means follows a normal 2 distribution. 1 0 1 2 3 4 5 Standard Error of Mean Sampling error can be characterized with Standard Error of Mean (SEM). The SEM tells us how much the mean is likely to vary from one sample to another! It depends on the 1. The standard deviation 2. Number of participants used to create the sample mean. Standard Error of Mean How does SEM differ from standard deviation (SD)? Mainly, SEM takes into account the sample size. While SD examines the spread of sample from the sample mean, SEM estimates the spread of the sample means around the population mean! Population Mean If we know the SEM, we can find out Distribution of samples means the proportion (or percentage) of 4 samples that would have a score lower than my sample: 3 If this proportion is low, that would mean that the score is not very likely 2 If it is unlikely to obtain a mean score of 1 just by chance, it means that the 1 depression intervention worked! 0 1 2 3 4 5 Inferential Statistics But in real life… It is unlikely you will know the population mean to make such comparisons How to be confident that the proportion is indeed low How to infer the results in the population from the sample? Inferential Statistics How to infer the results in the population from the sample? Estimating the results in the population (i.e., what would be the means in the populations?) Inferential Statistics Estimating the results in the population (i.e., what would be the means in the populations?) If the estimated mean memory score for the population “before the pill” is smaller than the estimated mean memory score for the population “after the pill”, then I can infer that the pill works Confidence intervals CI defines the range of values that have a specific % likelihood of including the population mean (i.e., estimation) Inferential Statistics CI defines the range of values that have a specific % likelihood of including the population mean Normally, we use 95% confidence intervals Inferential Statistics Last week… The area of 1.96 SDs below and above the mean will cover 95% of your distribution. Inferential Statistics CI defines the range of values that have a specific % likelihood of including the population mean Normally, we use 95% confidence intervals In normal distributions, ±1.96 of SEM gives 95% interval 95% certain that the population mean falls within the interval* Inferential Statistics CI defines the range of values that have a specific % likelihood of including the population mean A 95% confidence interval means that if you repeat your study with a new sample in the same way 100 times, you can expect your sample mean to lie within the specified range of values 95 times. Inferential Statistics Sample mean Let’s say, in our depression experiment, =3 we know that depression score ranges from 1 to 5. We can be 100% confident that the population mean lies 1 5 somewhere between these two scores. Depression Inferential Statistics Sample mean Let’s say, in our depression experiment, =3 we know that depression score ranges from 1 to 5. We can be 100% confident that the population mean lies 1 5 somewhere between these two scores. Depression But this interval is not informative! We can use our sampling distributions to narrow this range down. Inferential Statistics Sample mean Since we know our mean and SD, as =3 well as our sample size (N = 5), we can calculate the 95% CI to narrow it down 1 5 Depression Inferential Statistics Sample mean Here, you can see that we are 95% =3 confident that the population mean lies somewhere between 2.1 to 3.9. This is obviously more precise that 1 5 stating an interval of 1 to 5. Depression 2.1 3.9 Inferential Statistics Sample mean Here, you can see that we are 95% =3 confident that the population mean lies somewhere between 2.1 to 3.9. This is obviously more precise that 1 5 stating an interval of 1 to 5. Depression We can infer that obtaining score of 1 on the depression scale is very 2.1 3.9 unlikely (outside 95% CI, means obtaining this score has