SHS_08_Association PDF
Document Details
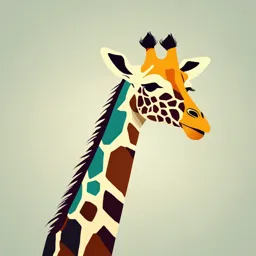
Uploaded by PraiseworthyHammeredDulcimer
UAB
Jose Barrera
Tags
Summary
This document is an academic document that discusses statistics in health sciences. It covers topics on measuring the associations between exposure and disease, and includes exercises which could have questions. It does not seem to be specifically an exam paper.
Full Transcript
B.Sc. Degree in Applied Statistics Statistics in Health Sciences 8. Measuring the association between exposure and disease Jose Barreraab [email protected] https://sites.google.com/view/josebarrera a ISGlobal Barcelona Institute for Global Health - Campus MAR b Department of Mathematics (UAB)...
B.Sc. Degree in Applied Statistics Statistics in Health Sciences 8. Measuring the association between exposure and disease Jose Barreraab [email protected] https://sites.google.com/view/josebarrera a ISGlobal Barcelona Institute for Global Health - Campus MAR b Department of Mathematics (UAB) This work is licensed under a Creative Commons “Attribution-NonCommercial-ShareAlike 4.0 International” license. Statistics in Health Sciences 1 Measures of association Introduction Comparing risks under different study designs Comparing RR, PR, OR and POR Confidence intervals 2 Measures of impact: Attributable risk, attributable fraction and attributable number Population attributable risk or fraction Exposure attributable risk or fraction Attributable fractions vs attributable numbers Jose Barrera (ISGlobal & UAB) Statistics in Health Sciences, 2023/2024 2 / 32 Measuring the association between exposure and disease: Introduction In these slides. . . • How can we assess the association between having been exposed to a given condition and suffer a given disease? • How can we compare the risk of suffering a given disease when a given exposure is present or absent? • What indicators can we consider? Under what conditions are they estimable? Concepts: Relative Risk, Odds Ratio, Attributable Risk. Smoke free bus stop in Warsaw, Poland (summer 2018). @overdispersion Jose Barrera (ISGlobal & UAB) Statistics in Health Sciences, 2023/2024 3 / 32 Comparing risks Introduction • Supose we want to explore the relationship between a binary exposure E and the presence of a given disease D, based on a 2 × 2 contingency table. • For example, supose that, at the end of a study, we have the following data: Disease Exposed No Yes Total No Yes 192 84 48 36 240 120 Total 276 84 360 • We can consider some indicator summarizing the comparison of the risk of the disease between the two groups of exposure. • This is usually done with a measure of relative risk, with the exposed group in the numerator and the non exposed group in the denominator. • The proper measure depends on the study design. . . Jose Barrera (ISGlobal & UAB) Statistics in Health Sciences, 2023/2024 4 / 32 Comparing risks under different study designs Cohort studies In cohort studies, we can compare the cumulative incidence of (i.e. probability of developing) the disease between the two exposure groups to get the risk ratio (RR) and the risk difference (RD): Risk ratio = RR = CIE , CIĒ Risk difference = RD = CIE − CIĒ . Prove that, for the following cohort study, RR = 1.50, which can be interpreted as the cumulative incidence (i.e. probability of developing) the disease among exposed being 50% higher than among non exposed. Exposed Study start End of study Disease Disease No Yes Total Exposed No Yes Total No Yes 192 84 48 36 240 120 Total 276 84 360 follow-up No Yes 240 120 0 0 240 120 Total 360 0 360 Jose Barrera (ISGlobal & UAB) −−−−→ Statistics in Health Sciences, 2023/2024 5 / 32 Comparing risks Case-control studies (1/5) In case-control studies, we can compare the prevalence of the exposure (i.e. probability of having been exposed) between the two disease status groups: P(E|D) . P(E|D̄) Study start End of study Exposed Disease No Yes Exposed Total Disease No Yes Total No (control) Yes (case) 192 48 84 36 276 84 Total 240 120 360 E assessment No (control) Yes (case) ? ? ? ? 276 84 Total ? ? 360 Jose Barrera (ISGlobal & UAB) −−−−−−−→ Statistics in Health Sciences, 2023/2024 6 / 32 Comparing risks Case-control studies (2/5) • In case-control studies, we can compare the prevalence of the exposure (i.e. probability of having been exposed) between the two disease status groups: P(E|D) , P(E|D̄) which is not very useful because we are interested in compare risks of D. I.e. we would like to estimate P(D|E) instead. P(D|Ē) • Using elemental theory of probability, it can be shown that P(D|E) P(E|D) 1 − P(E) = × , 1 − P(E|D) P(E) P(D|Ē) which cannot be estimated because with data from a case-control study, the prevalence of the exposure, P(E), cannot be estimated. • To solve this problem, we use the concept of odds. . . Jose Barrera (ISGlobal & UAB) Statistics in Health Sciences, 2023/2024 7 / 32 Comparing risks Case-control studies (3/5) • The odds of an event X is a monotone transformation of the probability: odds(X ) = P(X ) P(X ) = . 1 − P(X ) P(X̄ ) • The odds transforms the space P(X ) ∈ [0, 1] into the space odds(X ) ∈ [0, ∞): 10 9 8 odds(X ) 7 6 5 4 3 2 1 0 0.0 0.1 0.2 0.3 0.4 0.5 0.6 0.7 0.8 0.9 1.0 P (X ) Jose Barrera (ISGlobal & UAB) Statistics in Health Sciences, 2023/2024 8 / 32 Comparing risks Case-control studies (4/5) • Now, we can compare the prevalence of the exposure (i.e. probability of having been exposed) between the two disease status groups to get the odds ratio (OR): Odds ratio = OR = Prove that odds(E|D) . odds(E|D̄) P(E|D) P(D|E) odds(E|D) odds(D|E) ̸= while = . P(E|D̄) P(D|Ē) odds(E|D̄) odds(D|Ē) • According to the previous result, we can compute and interpret the odds ratio as ratio of odds of the disease instead of a ratio of odds of being exposed: Odds ratio = OR = Jose Barrera (ISGlobal & UAB) odds(E|D) odds(D|E) = = odds(E|D̄) odds(D|Ē) Statistics in Health Sciences, 2023/2024 P(E|D) P(Ē|D) P(E|D̄) P(Ē|D̄) = P(E|D)P(Ē|D̄) . P(E|D̄)P(Ē|D) 9 / 32 Comparing risks Case-control studies (5/5) Prove that, for the following case-control studya , odds(D|E) = 0.43, odds(D|Ē) = 0.25 and OR = 1.71, which can be interpreted as the odds of having the disease among exposed being 71% higher than among non exposed. Study start End of study Exposed Disease No Yes Exposed Total Disease No Yes Total No (control) Yes (case) 192 48 84 36 276 84 Total 240 120 360 E assessment a No (control) Yes (case) ? ? ? ? 276 84 Total ? ? 360 −−−−−−−→ In contingency tables for case-control studies, disease status groups are usually arranged in rows instead of in columns. Jose Barrera (ISGlobal & UAB) Statistics in Health Sciences, 2023/2024 10 / 32 Comparing risks Cross-sectional studies In cross-sectional studies, we can compare the prevalence of (i.e. probability of having) the disease between the two exposure groups to get the following measures of association: • Prevalence ratio: PR = P(D|E) . P(D|Ē) • Prevalence difference: PD = P(D|E) − P(D|Ē). • (Prevalence) odds ratio: = odds(D|E) . odds(D|Ē) Exercise Compute and interpret PR, PD and POR for the following cross-sectional study data. Study start End of study Disease Exposed No Yes Disease Total Exposed No Yes Total No Yes 192 84 48 36 240 120 Total 276 84 360 E and D assessment No Yes ? ? ? ? ? ? Total ? ? 360 Jose Barrera (ISGlobal & UAB) − −−−−−−−−−− → Statistics in Health Sciences, 2023/2024 11 / 32 Ratios vs differences (1/2) Ratios vs differences • In a given study, when comparing two groups, using ratios could lead, depending on data, to different results than using differences. E.g. it can happen that PR1 > PR2 while PD1 < PD2 , or vice versa. It also applies to risks (cohort studies) and odds (case-control studies). • It is natural, since ratios and difference work in different metrics.a • Usually, ratios are more widely used than differences. • Don’t compare a study based on ratios with another study based on differences!!! a Naive example: A’s income have increased from 100 units to 120 units (difference: 20 units; percentage: 20%). B’s income have increased from 150 units to 174 units (difference: 24 units; percentage: 16%). Jose Barrera (ISGlobal & UAB) Statistics in Health Sciences, 2023/2024 12 / 32 Ratios vs differences (2/2) Ratios vs differences: example (data) Ratios vs differences: example (results) A hypothetical cross-sectional study: PR resulted higher among women while PD resulted higher among men: D̄ Women D Total D̄ Men D Total Ē E 565 280 70 43 635 323 461 246 260 161 721 407 Total 845 113 958 707 421 1128 Women Men PR PD 1.208 1.097 0.023 0.035 Ratios vs differences: generalization It can be easily shown that this example is a particular case of the general situation in which if PR♀ > PR♂ and Jose Barrera (ISGlobal & UAB) PR♀ − 1 P(D | Ē♂ ) > , then PD♀ < PD♂ . PR♂ − 1 P(D | Ē♀ ) Statistics in Health Sciences, 2023/2024 13 / 32 Example: calculations in R using the epiR package (1/4) Toy data > > > > > > > > > > > > > + > > E0D0 E0D1 E1D0 E1D1 <<<<- Manually (point estimates) 192 48 84 36 > dd ## disease no disease ## exposed 36 84 ## non exposed 48 192 E0 <- E0D0 + E0D1 E1 <- E1D0 + E1D1 D0 <- E0D0 + E1D0 D1 <- E0D1 + E1D1 n <- E0 + E1 rr <- (E1D1 / E1) / (E0D1 / E0) or <- (E0D0 * E1D1) / (E0D1 * E1D0) dd <- matrix(c(E1D1, E1D0, E0D1, E0D0), nrow = 2, byrow = TRUE) rownames(dd) <- c("exposed", "non exposed") colnames(dd) <- c("disease", "no disease") Jose Barrera (ISGlobal & UAB) > rr ## [1] 1.5 > or ## [1] 1.714286 Statistics in Health Sciences, 2023/2024 14 / 32 Example: calculations in R using the epiR package (2/4) Cohort study > library(epiR) > epi.2by2(dat = as.table(dd), method = "cohort.count") ## ## ## ## ## ## ## ## ## ## ## ## ## ## ## ## ## ## ## Exposed + Exposed Total Outcome + 36 48 84 Outcome 84 192 276 Total 120 240 360 Inc risk * 30.0 20.0 23.3 Odds 0.429 0.250 0.304 Point estimates and 95% CIs: ------------------------------------------------------------------Inc risk ratio 1.50 (1.03, 2.18) Odds ratio 1.71 (1.04, 2.83) Attrib risk in the exposed * 10.00 (0.36, 19.64) Attrib fraction in the exposed (%) 33.33 (3.25, 54.06) Attrib risk in the population * 3.33 (-3.35, 10.02) Attrib fraction in the population (%) 14.29 (-0.69, 27.03) ------------------------------------------------------------------Uncorrected chi2 test that OR = 1: chi2(1) = 4.472 Pr>chi2 = 0.034 Fisher exact test that OR = 1: Pr>chi2 = 0.047 Wald confidence limits CI: confidence interval * Outcomes per 100 population units Jose Barrera (ISGlobal & UAB) Statistics in Health Sciences, 2023/2024 15 / 32 Example: calculations in R using the epiR package (3/4) Cross-sectional study > library(epiR) > epi.2by2(dat = as.table(dd), method = "cross.sectional") ## ## ## ## ## ## ## ## ## ## ## ## ## ## ## ## ## ## ## Exposed + Exposed Total Outcome + 36 48 84 Outcome 84 192 276 Total 120 240 360 Prevalence * 30.0 20.0 23.3 Odds 0.429 0.250 0.304 Point estimates and 95% CIs: ------------------------------------------------------------------Prevalence ratio 1.50 (1.03, 2.18) Odds ratio 1.71 (1.04, 2.83) Attrib prevalence in the exposed * 10.00 (0.36, 19.64) Attrib fraction in the exposed (%) 33.33 (3.25, 54.06) Attrib prevalence in the population * 3.33 (-3.35, 10.02) Attrib fraction in the population (%) 14.29 (-0.69, 27.03) ------------------------------------------------------------------Uncorrected chi2 test that OR = 1: chi2(1) = 4.472 Pr>chi2 = 0.034 Fisher exact test that OR = 1: Pr>chi2 = 0.047 Wald confidence limits CI: confidence interval * Outcomes per 100 population units Jose Barrera (ISGlobal & UAB) Statistics in Health Sciences, 2023/2024 16 / 32 Example: calculations in R using the epiR package (4/4) Case-control study > epi.2by2(dat = as.table(dd), method = "case.control") ## ## ## ## ## ## ## ## ## ## ## ## ## ## ## ## Exposed + Exposed Total Outcome + 36 48 84 Outcome 84 192 276 Total 120 240 360 Prevalence * 30.0 20.0 23.3 Odds 0.429 0.250 0.304 Point estimates and 95% CIs: ------------------------------------------------------------------Odds ratio 1.71 (1.04, 2.83) Attrib fraction (est) in the exposed (%) 41.58 (0.11, 65.68) Attrib fraction (est) in the population (%) 17.86 (-0.43, 32.81) ------------------------------------------------------------------Uncorrected chi2 test that OR = 1: chi2(1) = 4.472 Pr>chi2 = 0.034 Fisher exact test that OR = 1: Pr>chi2 = 0.047 Wald confidence limits CI: confidence interval * Outcomes per 100 population units Jose Barrera (ISGlobal & UAB) Statistics in Health Sciences, 2023/2024 17 / 32 Comparing RR, PR, OR and POR (1/2) Comments • The names “POR” and “OR” are used in cross-sectional and case-control studies, respectively. However, they are numerically identical and can be treated equally when modeling data. • In case-control studies, neither the RR nor the PR can be estimated, and we must use the OR. • In cross-sectional studies, both POR and PR can be estimated. However, PR is preferred because it is easier to interpret. • All relative measures of association, RR, PR and OR, consider the exposed group in the numerator. Hence: • If the relative measure > 1, then the exposure E is a potential risk factor for the disease D. • If the relative measure < 1, then the exposure E is a potential protective factor for the disease D. • If the relative measure = 1, then the exposure E and the disease D are independent. Jose Barrera (ISGlobal & UAB) Statistics in Health Sciences, 2023/2024 18 / 32 Comparing RR, PR, OR and POR (2/2) RR and PR: equal but different As seen before, if both a cohort study and a cross-sectional study provided identical 2 × 2 contingency tables at the end of the study, the values of RR for the former and PR for the latter would be identical. However, the interpretation would be different. • In a cohort study, the risk ratio (RR) compares the probability of developing the disease during the follow-up between the two groups of exposure. However, it could result in a biased estimation if the follow-up time is not similar for all individuals. • In cross-sectional studies, the prevalence ratio (PR) compares the probability of having the disease between the two groups of exposure, which is useless as an indicator of a causal relationship between the exposure and the disease because we cannot guarantee that the exposure preceded the disease. Jose Barrera (ISGlobal & UAB) Statistics in Health Sciences, 2023/2024 19 / 32 RR vs OR (1/3) RR and OR use different metrics RR and OR compare risks between exposed and non exposed but using different metrics. For instance, in the previous example, RR = 1.50 while OR = 1.71. 2.0 If we denote π0 := P(D|Ē), prove that: OR 1 − π0 • = RR 1 − π0 RR • RR > 1 → OR > RR • RR < 1 → OR < RR • RR = 1 → OR = 1 OR Exercise π0 = P(D |E) 1.0 0 0.001 0.01 0.02 0.05 0.1 0.25 0.5 0.5 1.0 2.0 RR Jose Barrera (ISGlobal & UAB) Statistics in Health Sciences, 2023/2024 20 / 32 RR vs OR (2/3) RR vs OR: Error size when reporting OR as if it was RR Error(%) = OR−RR RR × 100% = π0 (OR − 1) × 100% 20 15 Error (%) 10 5 0 π0 = P(D |E) −5 0.001 0.01 0.02 0.05 0.1 0.25 −10 −15 −20 0.5 0.6 0.7 0.8 0.9 1.0 1.1 1.2 1.3 1.4 1.5 1.6 1.7 1.8 1.9 2.0 OR Jose Barrera (ISGlobal & UAB) Statistics in Health Sciences, 2023/2024 21 / 32 RR vs OR (3/3) Using (carefully) OR as an approximation for RR • As seen, the lower P(D|E) and P(D|Ē), the closer RR and OR. Hence, for rare diseases, the values of RR and OR are similar. • Usually, rare diseases are analyzed using case-control ( Why?). In those cases, as we have previously seen, OR ≈ RR. • In the logistic regression model, which is used to model a binary outcome, the β coefficients have a straightforward interpretation in terms of OR. However, such coefficients cannot be interpreted in terms of RR. Hence, despite RR is easier to interpret than OR, we are forced to use OR to interpret risks for a binary outcome when it is modeled with a logistic regression model, even in the case of cross-sectional data (i.e. modeling a prevalence). In R • RR and OR can be calculated with riskratio and oddsratio, respectively, in the epitools package. • The epi.2by2 function in the epiR provides a detailed analysis of the 2×2 contingency table, taking into account the study design. Jose Barrera (ISGlobal & UAB) Statistics in Health Sciences, 2023/2024 22 / 32 Confidence intervals for OR, RR and PR (1/3) Notation Disease Exposed Yes No Yes No n++ n−+ n+− n−− π+ := P(D|E), OR point estimate d= OR π− := P(D|Ē). n++ , n++ + n+− Jose Barrera (ISGlobal & UAB) π̂− = = n++ n−− n−+ n+− RR and PR point estimate c = PR c = π̂+ = n++ (n−+ + n−− ) RR π̂− n−+ (n++ + n+− ) Point estimates for probabilities π̂+ = π̂+ 1−π̂+ π̂− 1−π̂− n−+ . n−+ + n−− Statistics in Health Sciences, 2023/2024 23 / 32 Confidence intervals for OR, RR and PR (2/3) Confidence intervals for OR d is normally distributed, so that • Asymptotically (high sample size), log(OR) ! r d d d CI1−α (OR) ≈ OR exp ±z1−α/2 Var log(OR) , where d = n++ n−− OR n−+ n+− and −1 −1 −1 −1 d ≈ n++ d log(OR) Var + n+− + n−+ + n−− . • The exact calculation of confidence intervals for OR is based in the hypergeometric distribution. • In R, confidence intervals can be calculated using epitools::oddsratio or epiR::epi.2by2. Jose Barrera (ISGlobal & UAB) Statistics in Health Sciences, 2023/2024 24 / 32 Confidence intervals for OR, RR and PR (3/3) Confidence intervals for RR and PR c is normally distributed, so that • Asymptotically (high sample size), log(RR) ! r c d c CI1−α (RR) ≈ RR exp ±z1−α/2 Var log(RR) , where c = n++ (n−+ + n−− ) RR n−+ (n++ + n+− ) d log(RR) c Var ≈ and n+− n−− + . n++ (n++ + n+− ) n−+ (n−+ + n−− ) • The exact calculation of confidence intervals for RR is based in the multinomial distribution. • In R, confidence intervals can be calculated using epitools::oddsratio or epiR::epi.2by2. • Formulas above also apply to PR. Jose Barrera (ISGlobal & UAB) Statistics in Health Sciences, 2023/2024 25 / 32 Measures of impact: PAR Population attributable risk (PAR) • While PR, RR and OR are measures of associations, the population attributable risk is a measure of impact. • The population attributable risk (PAR), or attributable fraction among the population (AFp ), is the proportion of cases in the population which are attributable to the exposure: PAR = AFp = Risk of D − Risk of D among non exposed Risk of D = P(D) − P(D|Ē) P(D|Ē) =1− . P(D) P(D) • Previous expression can be written as PAR = AFp = Pe (RR − 1) , 1 + Pe (RR − 1) where Pe is the exposed proportion of the population (i.e. the exposure prevalence). Jose Barrera (ISGlobal & UAB) Statistics in Health Sciences, 2023/2024 26 / 32 Measures of impact: PAR Population attributable risk (PAR): comments • P(D|Ē) = 0 =⇒ PAR = 1. • P(D|Ē) = P(D) =⇒ PAR = 0. • PAR can be interpreted as the fraction of cases that could be avoided if the exposure would have been removed from the population. However, such interpretation assumes that: • • • • The exposure status among the individuals is time invariant. There is a casual relationship between E and D. Removing E does no modify other potential effects on D due to other variables. The PAR estimation has been obtained after adjusting for potential confounding. Exercise Compute and interpret the PAR for the table in slide 5, assuming that, in that example, the sample is representative of the population and that the prevalence of the exposure in the whole population is 5%. Answer: 2.44%. Jose Barrera (ISGlobal & UAB) Statistics in Health Sciences, 2023/2024 27 / 32 Measures of impact: EAR Exposure attributable risk (EAR) • The exposure attributable risk, EAR, or attributable fraction among the exposed (population), AFe , is the proportion of cases among exposed (population) that are attributable to the exposure: EAR = AFe = Risk of D among exposed − Risk of D among non exposed Risk of D among exposed = P(D|E) − P(D|Ē) P(D|Ē) = 1− . P(D|E) P(D|E) • Previous expression can be written as EAR = AFe = 1 − Jose Barrera (ISGlobal & UAB) 1 . RR Statistics in Health Sciences, 2023/2024 28 / 32 Measures of impact: EAR Exposure attributable risk (EAR): comments • P(D|Ē) = 0 =⇒ EAR = 1. • P(D|E) = P(D|Ē) =⇒ EAR = 0. • The numerator of EAR is known as “excess risk”: ER = P(D|E) − P(D|Ē). Exercise Compute and interpret the EAR for the table in slide 5, assuming that, in that example, the sample is representative of the population. Answer: 33.33%. Jose Barrera (ISGlobal & UAB) Statistics in Health Sciences, 2023/2024 29 / 32 Measures of impact: attributable fractions vs attributable numbers Attributable fractions vs attributable numbers • In epidemiology, attributable fractions are usually reported as a percentage to describe the impact of the exposure. • Absolute impacts are also usually reported, which are known as attributable numbers (AN). • ANp = AFp · np , where np is the number of cases among the whole population, is the number of cases among the whole population that would not have occurred in the absence of exposure (under assumptions described previously). • ANe = AFe · np is the number of cases among the exposed population that would not have occurred in the absence of exposure (under assumptions described previously). • (Optional) further reading: Steenland and Armstrong [1] . Jose Barrera (ISGlobal & UAB) Statistics in Health Sciences, 2023/2024 30 / 32 Measures of impact: exercises Exercises 1 2 3 According to the work by Basagaña et al [2] , how the concept “heat wave” is defined? What was the overall impact estimate of heat waves on mortality? Is it PAR or EAR? Why? Interpret the result. According to the work by Khomenko et al [3] , what is the overall impact estimate of not compliance with WHO air pollution guidelines on mortality among the analyzed European cities? Is it PAR or EAR? Why? Interpret the result. Solve exercises in the document Exercises_SHS-CDA_v0_5.pdf, section “Measures of the disease”. Jose Barrera (ISGlobal & UAB) Statistics in Health Sciences, 2023/2024 31 / 32 References [1] K. Steenland and B. Armstrong. An overview of methods for calculating the burden of disease due to specific risk factors. Epidemiology, 17(5):512–519, 2006. URL https://doi.org/10.1097/01.ede.0000229155.05644.43. [2] X. Basagaña, C. Sartini, J. Barrera-Gómez, P. Dadvand, J. Cunillera, B. Ostro, J. Sunyer, and M. Medina-Ramón. Heat waves and causespecific mortality at all ages. Epidemiology, 22(6):765–772, 2011. URL https://doi.org/10.1097/EDE.0b013e31823031c5. [3] S. Khomenko, M. Cirach, E. Pereira-Barboza, N. Mueller, J. Barrera-Gómez, D. Rojas-Rueda, K. de Hoogh, G. Hoek, and M. Nieuwenhuijsen. Premature mortality due to air pollution in european cities: a health impact assessment. The Lancet Planetary Health, 5(3): e121–e134, 2021. URL https://doi.org/10.1016/S2542-5196(20)30272-2. Jose Barrera (ISGlobal & UAB) Statistics in Health Sciences, 2023/2024 32 / 32